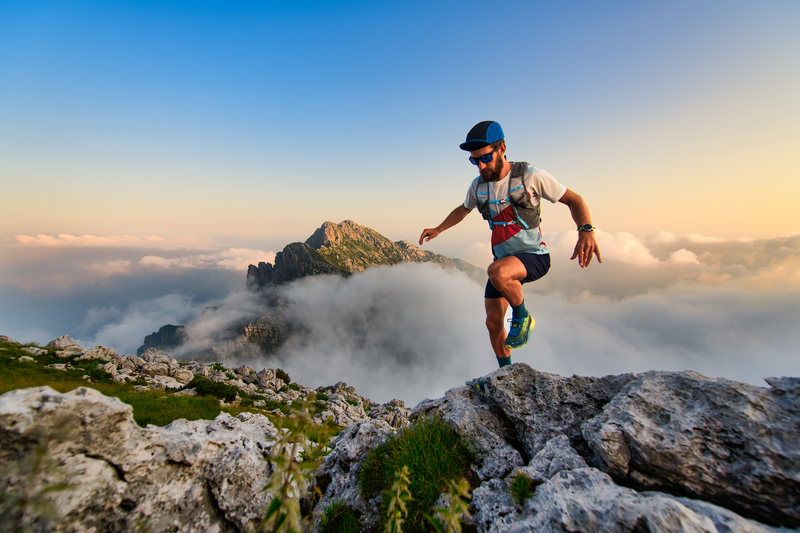
94% of researchers rate our articles as excellent or good
Learn more about the work of our research integrity team to safeguard the quality of each article we publish.
Find out more
ORIGINAL RESEARCH article
Front. Environ. Sci. , 28 October 2022
Sec. Environmental Economics and Management
Volume 10 - 2022 | https://doi.org/10.3389/fenvs.2022.1018540
With the rise of “Internet + Agriculture”, existing related research has focused on the impact of Internet use on agricultural productivity. However, few studies have considered the impact on agricultural green production efficiency. This paper employed the SBM model to calculate the agricultural green production efficiency using the observation data of 459 farmers in Shaanxi, Shanxi, and Henan provinces. Further, the influencing factors, their nonlinear impact, and even the effect of using the internet on agricultural green production efficiency were analyzed. The results show that corn’s average agricultural green production efficiency is 0.44, with the main peak distributed in a low-level range. The impact of the Internet use on agricultural green production efficiency is positively moderated by rural infrastructure, household assets, and educational attainment. Age and arable land area significantly impact agricultural green production efficiency. The endogenous switching regression model (ESR) shows that the agricultural green production efficiency of farmers who do not use the internet will increase by 41.45% if they use the internet. Internet use has a “U-shaped” impact on agricultural green production efficiency, and the threshold value of the farmers’ expenditure to income ratio is 0.0886. The policy aimed at improving agricultural green production efficiency and popularizing Internet use should, therefore, focus on strengthening the emphasis on increasing agricultural green production, increasing investment in rural human capital, providing green production subsidies and green production skills training, improving infrastructure construction in rural areas, integrating and enriching agricultural-related information on the Internet.
Agriculture in China is characterized by extensive production, such as high input of agricultural production resources, high emission of pollutants, backward agricultural production technology in some areas, and asymmetric production information, which have jointly led to a low utilization rate of agricultural production resources and deterioration of agricultural production environment (Zhang et al., 2022a; Zhang et al., 2022b). Besides, agriculture production in China has long faced the problem of resource constraints such as less per capita arable land, which has led to increasing pressure on agricultural production. Balancing the relationship between development and the deterioration of the ecological environment and promoting the transformation of green agricultural development has attracted more and more attention from all walks of life (Chandio et al., 2020; Chandio et al., 2022a). As an essential evaluation indicator of agricultural green production, agricultural green production efficiency (GPE) is the key to promoting the transformation of agricultural and rural green development (Chen et al., 2021), which guarantees national food security to a great extent. At the same time, implementing the political strategy of “Internet + agriculture” provides a new opportunity for the transformation of China’s agricultural development (Shen et al., 2022). At the same time, the effect of the internet on improving the agricultural production environment has been controversial. Due to the differences in the current rural environment and geographical conditions, as well as the existence of factors such as the low degree of Internet penetration, the acceptance of the internet by different households, and its effect on agricultural green production efficiency are different. Therefore, examining the agricultural green production efficiency in China and its influencing factors, especially the influence of Internet use, are worthy of further academic exploration.
A number of studies have analyzed agricultural green production efficiency from three aspects. One is the research on the measurement method of agricultural green production efficiency, which mainly includes the non-parametric method represented by data envelopment analysis (DEA) and the parametric method represented by stochastic frontier analysis (SFA) (Chen et al., 2021; Huang et al., 2022; Li and Lin, 2016; Xu et al., 2019; Cui et al., 2020; Yang et al., 2022; Zhai et al., 2022). Adetutu and Ajayi (2020) used stochastic frontier analysis (SFA) to calculate agricultural productivity in Sub-Sahara Africa, and Chen et al. (2021) used the three-stage DEA model and SBM model to calculate agricultural green total factor productivity in China. The second is the selection of measurement indicators of agricultural green production efficiency. The advantage of green production efficiency is that environmental factors are considered in the measurement system. Most studies have little difference in the selection of variables, mainly taking fertilizer and pesticide as input variables, crop yield as expected output variables, and non-point source pollution as undesirable output variables (Cole and Fernando, 2012; Kaila and Tarp, 2019; Xu et al., 2019; Chen et al., 2021; Huang et al., 2022). The third is the selection of models on the impact of subject behavior on production efficiency. The endogenous switching regression model (ESR) and propensity score matching model (PSM) are mainly applied to explore the relationship between the internet and technical efficiency, as well as the relationship between risk perception and farmers’ fertilizer use (Tang et al., 2018; Zheng et al., 2021; Zhu et al., 2021), using DID model to explore the impact of policies on grain green production efficiency (Li and Lin, 2022), models such as PSM-DID was used to explore the impact of urban energy policies on green production efficiency (Zhuo et al., 2022), or use PSM model to classify respondents for correlation analysis (Li et al., 2020; Mario et al., 2014). For example, Zhu et al. (2021) applied the endogenous switching regression model to explore the Internet’s impact on Apple’s production technical efficiency. In contrast, few studies analyze the impact of Internet use on agricultural green production efficiency.
The research on the impact of Internet use on production efficiency is relatively rich, mainly focusing on two aspects. On the one hand, it has been found that Internet use can promote production efficiency (Chandio et al., 2022b). Specifically, the use of the internet can improve technical efficiency (Zheng et al., 2021; Zhu et al., 2021) and total factor production efficiency (Gao et al., 2022), promoting industrial green production efficiency (Zhang et al., 2018), and promote agricultural green production efficiency (Kaila and Tarp, 2019; Zheng et al., 2022a). On the other hand, some scholars have divided the impact of Internet use on production efficiency into two parts: technical progress and technical efficiency. Fare et al. (1994) held that the improvement of agricultural green production efficiency could be further decomposed into agricultural technical progress-driven and technical efficiency-driven. Some scholars have pointed out the promoting effect of Internet use on agricultural green production efficiency, mainly derived from the improvement of agricultural technical efficiency (Zhang et al., 2018), Zheng et al. (2021) pointed out that using the internet can improve the technical efficiency of banana production. Zhu et al. (2021) explored the influence of Internet use on the technical efficiency of apple production. They found that the use of the internet played a significant role in promoting the technical efficiency of apple production. Sun and Shu (2021) showed that Internet use had a significant impact on improving agricultural supply efficiency and logistics efficiency. Finally, the technical efficiency of agriculture can be improved. On the other hand, some scholars conclude that the promoting effect of Internet use on agricultural green production efficiency mainly comes from the progress of agricultural technology (Chi et al., 2021; Tao et al., 2016). Liu et al. (2022) pointed out that agricultural green production efficiency’s main growth driver was agricultural technical progress. Mario et al. (2014) also pointed out that the main role of Internet use in affecting agricultural production efficiency is to promote the progress of agricultural technology while reducing the efficiency of agricultural technology. Some studies have also pointed out that Internet use has a significant promoting effect on green production efficiency in the short term, but in the long run, the impact of Internet use on green production efficiency has a nonlinear relationship (Liu et al., 2021; Liu et al., 2022; Wu and Zhang, 2020; Zhou et al., 2019). As Hu et al. (2020) pointed out in their study, China’s agricultural total factor productivity has undergone three stages, accelerated growth, negative growth, and fluctuation. The first stage accelerates the growth of agricultural total factor productivity and is also a stage where technical efficiency and progress are jointly driven. In the second stage, the total factor productivity of agriculture entered the decline period, mainly due to the deterioration of technical progress and technical efficiency, but mainly dominated by technical progress. The fluctuation of technical efficiency mainly causes the fluctuation of agricultural total factor productivity in the third stage.
Although studies have contributed to analyzing the relationship between Internet use and green production efficiency, some limitations remain in the research. First, most of the research focuses on the impact of Internet use on industrial green production efficiency, total factor production efficiency, agricultural technology efficiency, ecological efficiency etc., or take wheat and rice as well as economic crops such as bananas and apples as the main research objects. However, there is a lack of research on the impact of Internet use on agricultural green production efficiency, with corn as the main research object. Second, there are some limitations in the research conclusions. Existing studies are controversial about the relationship between Internet use and production efficiency, and most of them only explore whether Internet use can improve green production efficiency. However, there is a lack of further extension. To address the above limitations, this paper has the following important contributions: First, to the best of our knowledge, this paper is the first study to explore the influence of Internet use on agricultural green production efficiency by taking corn as the research object. According to the requirements of the Chinese No.1 central document “On Making Every Effort to Improve Food Production and Supply of Important Agricultural Products,” released in 2022, taking corn, China’s main grain, as the research object to explore whether the Internet use can promote agricultural green production efficiency, improve the agricultural production environment, and promote sustainable agricultural development, is necessary and has great significance. Second, based on existing research, this paper will further explore whether there is a nonlinear relationship between Internet use and agricultural green production efficiency and analyze the development stage of China’s agricultural development in combination with the actual situation. Thirdly, the endogenous switching regression model is used to analyze the effect of Internet use on agricultural green production efficiency. This model can effectively solve the endogeneity problems. At the same time, the threshold regression model is used to explore whether Internet use in the agricultural production process has a nonlinear impact on the agricultural green production efficiency and to analyze the heterogeneity of farmers to explore the green production efficiency of different types of farmers using the internet. Finally, according to the research conclusions, the paper puts forward targeted suggestions to provide theoretical support for improving the agricultural production environment, ensuring national food security, and promoting sustainable agricultural development.
The rest of this article is arranged as follows. The second section introduces the theoretical framework and research hypothesis, and the model construction and variable design are presented in section three. The fourth section summarizes the empirical results, followed by the discussion for the results, and concludes with the conclusions and policy implications.
The traditional theory of agricultural production behavior holds that farmers’ behavior is often accompanied by complete information. However, it is difficult for farmers to obtain complete information when making production decisions. Internet use can reduce farmers’ information costs, improve farmers’ cognition of agricultural technology and market, and realize the optimal allocation of resources. The key to high-quality agricultural development is optimizing production resources and reducing excessive investment in production factors such as pesticides and fertilizers. Agricultural green production efficiency is the key to dealing with the serious negative externalities caused by the long-term investment of a large number of chemical fertilizers and pesticides. Improving the agricultural green production efficiency is conducive to improving the agricultural production environment, helping agriculture realize the transformation of economic structure and promoting high-quality agricultural development.
The improvement of agricultural green production efficiency lies in introducing new production factors. The Internet use, as a new production factor providing production information and technology, changes the traditional agricultural production mode through information transmission, which is conducive to adjusting and optimizing the input structure of agricultural production, thereby promoting the spillover effect of technology and promoting the common development of economic progress and ecological environment improvement. This study believes that Internet use mainly affects agricultural green production efficiency in two ways. The first way mainly plays a role in the progress of agricultural technology (Tao et al., 2016; Chi et al., 2021; Liu et al., 2022). Economist Kuznets explained on economic growth that the economic growth of a country is inseparable from advanced technology and the corresponding ideology. By building an information transmission platform (Ogutu et al., 2014), Internet use spreads modern technologies and knowledge to more farmers and promotes the adoption of advanced agricultural technologies (Zheng et al., 2022b). At the same time, the Internet use, as a dissemination platform for knowledge capital, effectively connects agricultural producers, scientific research institutes, and front-end and back-end enterprises in the agricultural industry chain and applies advanced agricultural production technology to the whole process of agricultural production, which is conducive to promoting the progress of agricultural technology and promoting agricultural technology progress, improving integrated agricultural production, effectively reducing the undesired output of agricultural production, thereby improving agricultural green production efficiency. The second approach plays a role in agricultural technical efficiency (Zhang et al., 2018; Zhu et al., 2021). The development of Internet use is conducive to improving the phenomenon of information asymmetry and reducing the cost of information transmission. Internet use has penetrated information technology into all stages of agricultural production and changed the mode of agricultural production in many aspects. Internet use has made full use of labor and other production resources to achieve more optimal resource allocation, thereby improving agricultural technical efficiency and making agricultural production more sustainable (Liu et al., 2021). According to the research of Fare et al. (1994), agricultural production efficiency is decomposed into agricultural technical progress and agricultural technical efficiency. Agricultural technical progress and agricultural technical efficiency will improve agricultural green production efficiency.
On this basis, the following hypothesis is put forward.
H1. Internet use can promote agricultural green production efficiency.Users are demanded to improve themselves for increasingly advanced Internet technology, and the development of the rural internet is also limited by the ability of farmers to use it. At present, the education level of agricultural operators is generally low, and their understanding of the internet is not deep enough. At the same time, due to the large differences between the traditional agricultural business model and the “Internet + agriculture” business model, such as differences in transaction channels, methods, platforms, etc., and the incomplete construction of the “first-kilometer” of agricultural products leaving the village and entering the city, to a certain extent, it restricts the circulation of agricultural products, reduces the total output value of agricultural products, and leads to a decline in green production efficiency. At the initial stage when the internet was put into rural areas, it was difficult for the internet to fully play a role and achieve the purpose of optimizing resource allocation due to the insufficient professional and technical level of agricultural operators and the corresponding inadequate infrastructure in rural areas. Accordingly, in the initial stage of internet development, the effect of Internet use on green production efficiency will show a downward trend with professional technology going to the countryside, agricultural technology training, and infrastructure improvement. The Internet use has begun to promote the progress of agricultural technology and the improvement of agricultural technical efficiency, to better realize the allocation of agricultural resources (Zhou et al., 2019; Wu and Zhang, 2020; Wu et al., 2021b; Shen et al., 2022). Furthermore, Internet use will promote the improvement of agricultural green production efficiency.Accordingly, the following assumption is made.
H2. There is a nonlinear relationship between Internet use and agricultural green production efficiency.
For instance, the existing calculation of green production efficiency mainly focuses on cash crops such as bananas and apples (Zhu et al., 2021; Zheng et al., 2021) or measurement of grain eco-efficiency (Liu et al., 2021), or using SPF and SBM models for analysis (Mario, 2014; Cui et al., 2020; Li and Chen, 2021). Considering the limitations of the measurement scope and research objects and that agricultural production is greatly affected by the external environment, the traditional DEA model cannot accurately measure the output (Chen et al., 2021), and SPF cannot effectively solve the problem of undesired output. Therefore, this paper takes corn as the research object and uses the SBM model, including undesired output, to measure agricultural green production efficiency.
Taking each respondent’s family as the unit of analysis, according to Xu et al. (2019) and Huang et al. (2022), the inputs of fertilizer, pesticide, irrigation, machinery, and labor per unit area were selected as inputs indicator, and output value per unit area is taken as the desired output indicator. Carbon emission per unit area was taken as the undesired output indicator. The agricultural green production efficiency of 459 samples was calculated by DEA software, and the calculation results are shown in Figure 1.
It can be found from Figure 1 that the main peak is located in the low green production efficiency range. Except for some farmers with high agricultural green production efficiency, most agricultural green production efficiency is located in the low agricultural green production efficiency range, which means there is still much room for improvement in agricultural green production efficiency.
This study uses the green production efficiency calculated by each sample as the dependent variable. Due to the censored characteristics of the dependent variable, the parameters will be seriously biased and inconsistent if estimated by traditional OLS regression. Thus the Tobit model, which can solve this problem well, is used to analyze the influencing factors (Chen and Zhou, 2011). The Tobit model constructed in this paper is as follows:
Among them,
In the existing literature, the instrumental variable or PSM method is mostly used to explore the difference in effects of whether the same event occurs with cross-section data (Zheng et al., 2021). However, the former method will ignore the heterogeneity problem, while the latter will be unable to deal with the endogeneity problem caused by unobserved factors (Zhu et al., 2021). Therefore, this paper, referring to the study of Tang et al. (2018), selects the endogenous switching regression model for analysis and deals with the measurement errors caused by unobservable factors in the model. This paper constructs a production efficiency growth model as follows:
In Eq. 3, it indicates whether the farmers use the internet, if the farmers use the internet
The endogenous switching regression model results can reflect the differential impact of various variables on agricultural green production efficiency with and without internet use. Further, this paper will use the counterfactual hypothesis to explore the change in the expected value of green production efficiency when farmers use the internet and do not use the internet. To analyze the average treatment effect of Internet use on agricultural green production efficiency.
Agricultural green production efficiency expectations using the internet:
The expected value of agricultural green production efficiency without the use of the internet:
Considering the counterfactual hypothesis in both cases, the following is the expected value of agricultural green production efficiency level of farmers using the internet under the scenario of not using the internet:
The expected value of agricultural green production efficiency of farmers who do not use the internet in the scenario of using the internet:
Taking the difference between Eqs. 4, 6, the treatment effect of using the internet on GPE is:
Similarly, by making the difference between Eqs. 5, 7, the treatment effect of not using internet on GPE can be obtained as follows:
In conclusion, the average value of ATT and ATU is used in this paper to measure the average treatment effect of Internet use decision making on GPE of two types of farmers.
Due to many factors, such as human capital and infrastructure, have huge differences in agricultural green production efficiency in different stages of internet development (Mario, 2014). Thus, this paper uses a threshold regression model to explore the relationship between the internet development stage and agricultural green production efficiency by referring to Wu and Zhang (2020).
According to the theory of Hansen, (2000), the following threshold model is established:
Among them, i = 1,2, j = 1,2,3,4,5,6; P indicates whether the farmers use the internet.
Dependent variable: Agricultural green production efficiency is determined as the dependent variable in this paper, which is calculated with the input and output of farmers.
Treatment variable: Whether farmers use the internet is taken as the treatment variable in this study. In the survey process, the farmers were asked whether they used the internet to learn about agricultural modernization knowledge, which was used as the criterion to judge whether they used the internet. According to statistics, 65% of the farmers used the internet in 459 valid data, indicating that there is still great room for improvement in the popularization of the internet in rural areas.
Matching variables: This paper refers to the research of Zhu et al. (2021), Bravo-Ureta et al. (2020) and Xie et al. (2021), combined with the actual situation of the survey, and then selects respondents’ gender, age, years of education, cultivated land area, total assets, level of rural infrastructure, and total expenditure as the main independent variables. Whether the family’s province belongs to Shaanxi Province and whether it belongs to Shanxi Province are used as regional control variables.
Instrumental variable: This paper selects whether to accept technical guidance as an instrumental variable, because whether to accept technical guidance has an important impact on whether farmers can obtain more useful information through the internet, but it has no direct impact on agricultural green production efficiency. Therefore, whether farmers accept technical guidance is selected as an instrumental variable.
The data in this paper are from the survey in Shaanxi, Henan, and Shanxi provinces in 2021. The data are collected by stratified sampling in each province to make the research conclusion more universal. We randomly chose three counties in each province, three townships in each county, and then three villages in each township, with six households in each village; households that produce wheat and corn, in turn, were selected as the research objects, and face-to-face interviews were conducted with family decision-makers, which mainly involved household internet use, fertilizer, pesticide, seed, irrigation, labor input, machinery input and output in the process of agricultural production and other issues. A total of 480 questionnaires were distributed, and 470 were recovered, of which 459 were valid, with an effective rate of 97.66%. The sample selection of this paper has the following characteristics: First, Shaanxi, Shanxi, and Henan, as the major agricultural production provinces in the Yellow River basin, have the highest grain output in the country and a high degree of agricultural production modernization, which is representative to a certain extent. Second, the three provinces of Shaanxi, Shanxi, and Henan rotate the planting of corn and wheat each year, and the planting scale is relatively large, which is also the area of serious agricultural non-point source pollution, facing greater pressure of agricultural environmental protection. Third, the samples cover agricultural ecosystems in different geographical environments, such as Guanzhong Plain, Loess Plateau, and North China Plain. Regional differences exist between rural households’ Internet use and green agricultural development. The above characteristics reflect the good representativeness of the samples in this paper.
Table 1 shows the descriptive statistical results of each variable for the total sample and farmers with and without Internet use. In all samples, we can find that the mean value of agricultural green production efficiency is 0.44. There are more males in the sample area, and the respondents are older and have lower years of education. 95% of the respondents were male; the average age was 60.46. The average distance to the distribution station is 1.46 km. The average year of education was 6.99, the average arable area of the families was 8.49 mu (1/15ha), the average total household assets were 21,100 CNY, and the average expenditure was 41,200 CNY.
According to the descriptive statistical results of the farmers who use the internet and those who do not use the internet in Table 1, it can be concluded that the agricultural green production efficiency is both 0.44, and there is no significant difference; in terms of matching variables, there are significant differences in gender, age, education level and the family arable land area between farmers who use the internet and those who do not. Regarding gender, the proportion of men who use the internet is higher than that of men who do not. Compared with those who did not use the internet, the farmers who used the internet were younger, better educated, and more cultivated area. However, there is no significant difference between the two groups of farmers in terms of total assets and expenditures.
The Tobit model is used to explore the influence factors of the Internet on agricultural green production efficiency by using Stata16.0. The analysis results are shown in Table 2. Column 1) contains only the core explanatory variable of Internet use. Column 2) adds control variables, infrastructure, and interaction terms with Internet use based on Column (1). Column 3) adds control variables, assets, and their interaction terms with Internet use based on Column (1). Based on Column (1), the control variable, education level, and its interaction with Internet use are added to Column (4). All variables are added to Column 5) for regression based on Column (1). According to the regression results in Table 2, from the horizontal comparison, the symbols of the regression coefficients of variables in columns 1) to 5) are consistent, indicating that the model is stable.
TABLE 2. Analysis of influencing mechanism of the Internet on agricultural green production efficiency.
The results in Table 2 show that the impact of Internet use on agricultural green production efficiency is significant. The coefficients are all positive in the five regression results, which indicates that Internet use can improve agricultural green production efficiency. This conclusion is consistent with the study of (Zheng et al., 2022a), who shows that the Internet can promote the adoption of agricultural technologies, thereby increasing agricultural production efficiency. Hypothesis H1 is valid. From the perspective of horizontal comparison, after adding various explanatory and control variables, the regression coefficient of Internet use shows increasing characteristics. Specifically, the coefficient of Internet use is significant in Column (1), with a value of 0.041. Since the influence of other factors is not considered, this result may be biased. Therefore, more explanatory variables are introduced. In Column (5), the coefficient of Internet use is significant, and the value increases to 0.0925, indicating that Internet use positively impacts agricultural green production efficiency. Meanwhile, Column 5) shows that age, education level, rural infrastructure, and household assets significantly positively affect agricultural green production efficiency. Whereas the arable land area significantly negatively affects agricultural green production efficiency. For example, Shen et al. (2022) pointed out that human capital has a positive impact on agricultural production efficiency.
The coefficients of the interaction of Internet use and infrastructure, Internet use and household assets, and Internet use and education, are all positive significant at a 5% significance level, indicating the complementary relationships exist between them. In other words, improving the level of rural infrastructure, household assets and education level of householders, can strengthen the positive impact of Internet use on agricultural green production efficiency.
It can be seen from Table 3 that Internet use has a promoting effect on agricultural green production efficiency. To explore growth effects, The ESR model is used to analyze Eq. 3 using Stata16.0. Regression results are shown in Table 3. According to the likelihood ratio test results and goodness of fit test, the hypothesis that decisions and outcome equations are independent can be significantly rejected, and the model is significant at 1%.
TABLE 3. The results of the simultaneous analysis using the internet and the impact model of agricultural green production efficiency.
The regression results of the decision model of farmers using the internet are shown in the column of decision equations in Table 3. As mentioned in the previous variable selection, the treatment variable selected in this paper is whether farmers use the internet. If the regression result of the variable is significantly positive, it indicates that this variable has a significant positive impact on farmers’ use of the internet and vice versa. It can be seen from Table 3 that age, education level, cultivated land area, whether they are in Shanxi Province, and whether they receive technical guidance have a significant impact on their Internet use.
The education level of farmers who do not use the internet has a negative impact on agricultural green production efficiency. The higher education level of the farmers in the case of not using the internet, the traditional agricultural management mode would be used, which inputs a large number of chemical fertilizers, pesticides, and other production resources that may have a negative impact on the agricultural environment. Therefore, it has a negative impact on agricultural green production efficiency. Both cultivated land area and household assets have a negative impact on agricultural green production efficiency. The main reason may be that the larger the cultivated land area and the richer the household assets, the greater the input of agricultural production, which is not conducive to the optimization of resource allocation, thus reducing the efficiency of agricultural green production. Expenditure has a positive impact on agricultural green production efficiency. Current household expenditures include production and operation expenditures, basic living, and gift money expenditures. The higher the current expenditure, the smaller the agricultural production and operation expenditure share under the same conditions. Therefore, the allocation of resources can be optimized, which is conducive to improving agricultural green production efficiency.
The regression results of the growth effect of agricultural green production efficiency are shown in the result equation column in Table 3. The results of the comparative analysis are as follows: the gender of farmers who use the internet and those who do not use the internet have a negative impact on agricultural green production efficiency, and the age of farmers has a positive impact on agricultural green production efficiency. According to the survey, most of the young male labor force in rural areas shows the mode of part-work and part-farming. In contrast, the female and elderly labor are mainly responsible for the production and operation of family agriculture. Young men do not have enough energy to plan the input and output of agricultural production, which has a negative impact on agricultural green production efficiency. For farmers who use the internet, the regression result of educational level is positive and significant at the 10% level. The higher the level of education, the higher the acceptance and cognitive efficiency of new things, which can promote the use of the internet to obtain sufficient information to make full use of production resources, optimize the allocation of resources, and improve agricultural green production efficiency (Deng et al., 2022). Household assets positively impact agricultural green production efficiency and are significant at the 5% significance level. The more household assets mean more capital to use the internet to learn and apply modern technologies, promoting agricultural green production efficiency (Wang, 2018). Expenditure positively impacts agricultural green production efficiency, and it is significant at 10%. It may be explained that more expenditure means more opportunities to use the internet to learn more knowledge, which can help to optimize the allocation of input and output and rationally plan the input and expenditure of productive resources such as fertilizers and pesticides, thereby improving agricultural green production efficiency (Xie et al., 2021; Jasper et al., 2019). The regression coefficient of the cultivated land area is positive, indicating that the cultivated land area positively impacts agricultural green production efficiency. The larger the cultivated land area of the family, the more need to use the internet to learn modern technologies, carry out large-scale production, improve the efficiency of agricultural technology, and promote the progress of agricultural technology as a whole, thereby improving agricultural green production efficiency.
In this paper, the endogenous switching regression model is used to explore the processing effect of Internet use on agricultural green production efficiency. The analysis results are shown in Table 4. Among them, the contents of 1) and 2) respectively represent the factual results of agricultural green production efficiency in the context of using the internet or not; Similarly, the contents of 3) and 4) respectively represent the fact hypothesis results contrary to the preceding.
According to Table 4, the average treatment effect of household Internet use on agricultural green production efficiency has a positive impact and is significant at the 1% level. From the perspective of the average expected agricultural green production efficiency, based on the background of the counterfactual hypothesis that if using the internet, farmers in the case not using the internet, its green production efficiency will decrease by 0.333, which fell by 73.67%. The agricultural green production efficiency of farmers who do not use the internet will increase by 0.456 if they use the internet, which rose by 41.45%. Through the above comparison, it is found that the use of the internet can significantly improve agricultural green production efficiency.
To explore the effect of heterogeneous farmers’ Internet use on agricultural green production efficiency. Farmers with different education levels were selected to analyze the average treatment effect in this study. According to Table 1, the average years of education for all the samples is 6.99 years. Therefore, this paper divides farmers into primary school and below level and middle school and above level. The analysis results are shown in Table 5. The results show that the average treatment effect level of agricultural green production efficiency for farmers at the primary school level and below after using the internet is 0.103, which is significant at the level of 1%. The average treatment effect of agricultural green production efficiency for farmers at the middle school level and above after using the internet is 0.313 and is significant at 1%. It shows that farmers with different educational levels can improve agricultural green production efficiency after using the internet. Farmers with high education levels are more receptive to new things and have a stronger ability to learn; they are more effective in acquiring and mastering agricultural information and modernization technologies. Thus, farmers with a high education degree can easily get information from the internet and reasonably control the input and output of agricultural production, optimize resource allocation and improve agricultural green production efficiency. Although farmers with low education levels may be deficient in learning ability, due to the particularity of rural agglomeration living, the behavior of people around them will have a particular impact on the production and management decisions of farmers, especially to learn more advanced agricultural production information and modern technology through the internet. After discussion and communication with neighbors, agricultural production input can be planned, and undesired output can be controlled scientifically to improve the agricultural production environment and increase agricultural green production efficiency (Deng et al., 2022).
TABLE 5. The average treatment effect of different educated farmers using the internet on agricultural GPE.
In this section, MATLAB14.0 was applied to perform regression analysis on the threshold model mentioned above, and the ratio of expenditure to income was selected as the threshold variable. Through 10,000 bootstrap simulations, the LR value is calculated to be 16.5141, the single threshold value is 0.0886, and is significant at 10%, which indicates that the expenditure-to-income ratio of farmers has a significant threshold role in the impact of Internet use on agricultural green production efficiency. The test results are shown in Table 6.
In Table 6, column 1) represents the general linear regression model, and columns 2) and 3) represent the regression results of the threshold model, where model 2) represents the regression result of the expenditure-to-income ratio lower than the threshold value, and model 3) represents the regression result of the expenditure-to-income ratio higher than the threshold value. When the ratio of farmers’ expenditure to income is lower than the threshold value, the use of the internet has a significant negative impact on agricultural green production efficiency, which means that when the ratio of farmers’ expenditure to income is lower than the threshold value of 0.0886, the use of the internet by farmers will have a negative impact on the agricultural production environment. It is not conducive to optimizing the allocation of agricultural production resources, thereby reducing agricultural green production efficiency. When the ratio of farmers’ expenditure to income is higher than the threshold value, the use of the internet positively impacts agricultural green production efficiency, and it is significant at a significant level of 10%. It shows that when the ratio of farmers’ expenditure to income is higher than 0.0886, internet use can effectively plan agricultural production’s input and output, thereby promoting green production efficiency. The main reason for this result lies in the poor infrastructure conditions in rural areas at the initial stage. Coupled with the lack of professional technology and knowledge, most farmers adopt the traditional extensive production mode, which leads to the low utilization rate of agricultural resources. The continuous improvement of infrastructure and the increase of informatization degrees in rural areas, and the arrival of professional and technical personnel in rural areas, to help rural areas improve their production and management models, improve the utilization rate of agricultural resources, and promote sustainable development of agriculture, indicating that there is a nonlinear relationship between Internet use and agricultural green production efficiency (Wu and Zhang, 2020; Wu et al., 2021a; Zhu et al., 2021).
This paper firstly selects the method of replacing the core explanatory variables with reference to the research of Shen et al. (2022) for the robustness test, taking the number of visits to agricultural websites as the core explanation, without changing the estimation method, and re-analyze its effect on agricultural green production efficiency. The results are shown in Table 7.
By comparing the results in Tables 3, 7, it pointed out that the direction of the regression results has not changed, the changes in the magnitude of the coefficient values are also small, and the results of variables that passed the significance test are the same. Taking the decision equation as an example, the variables that passed the significance test are still age, education level, area of arable land, and whether they received technical guidance. The effects of these four variables are also consistent with Table 3, which shows that the model is robust.
The PSM method is selected further to test the robustness, and the average treatment effect results are shown in Table 8. By comparing Tables 4, 8, it can be found that the coefficients estimated by PSM and ESR models are in the same direction, and the magnitude of the values only changes in a small range, indicating the robustness of the regression model.
There are three important discoveries through the empirical part of the present: 1) The Internet can significantly improve agricultural green production efficiency. 2) Rural infrastructure, household assets, and education level play a moderating role in the impact of the Internet on agricultural green production efficiency. 3) There is a nonlinear relationship between the Internet and agricultural green production efficiency.
According to the regression results in Table 2, the Internet can significantly positively impact agricultural green production efficiency. Table 4 shows that the agricultural green production efficiency of farmers who do not use the Internet has increased by 41.45% after using the Internet, indicating that the Internet significantly promotes agricultural green production efficiency. The main reason is that the Internet spreads modernization information and technology to farmers by building an information transmission platform, which breaks the barrier of traditional agricultural information asymmetry. At the same time, the Internet connects farmers with scientific colleges, consumers, and suppliers of raw materials. After obtaining more information and resources, farmers can improve the production mode, change the amount of production input, optimize the allocation of agricultural resources, scientifically reduce the undesired output, and effectively improve the agricultural production environment to improve agricultural green production efficiency (Liu et al., 2021; Zhu et al., 2021; Zheng et al., 2021). This conclusion is consistent with the study of Zheng et al. (2022b). Ogutu et al. (2014) pointed out that the Internet use can help farmers change the amount of fertilizer and pesticide input, change the production behavior of farmers, and optimize the allocation of resources.
The regression results in Table 2 show that the impact of the Internet use on agricultural green production efficiency is moderated by rural infrastructure, household assets, and educational attainment. Rural infrastructure plays a positive regulating role in the impact of the Internet use on agricultural green production efficiency. Rural infrastructure is an important non-productive driver of agricultural productivity. With the improvement of infrastructure construction, the Internet use can facilitate farmers to adopt more modern transaction modes in the agricultural production process, promote the adoption of emerging green production technologies, and promote technological progress in rural areas, thereby improving agricultural green production efficiency. This conclusion is similar to the research of Onofri and Fulginiti. (2008).
Household assets play a positive moderating role in the impact of the Internet use on agricultural green production efficiency. The main reason is that the more household assets, the more abundant the funds to use the Internet to learn agricultural modernization technology, and the more apt to apply modern green technology to agricultural production, promote the progress of agricultural technology, and then improve agricultural green production efficiency (Wang, 2018).
Education level positively moderates the impact of the Internet use on agricultural green production efficiency. The reason is that the higher the education level of farmers, the stronger their ability to accept new technologies and knowledge. They can learn more agricultural modernization green production knowledge through the Internet and apply it to the agricultural production process. Farmers with higher education levels can learn to rationally plan the input of agricultural production resources and optimize the allocation of production resources to improve the agricultural technical efficiency. By scientifically reducing undesired output, the agricultural production environment is improved, the sustainable development of agriculture is promoted, and finally, the goal of improving agricultural green production efficiency can be achieved (Deng et al., 2022). Shen et al. (2022) pointed out that Internet use can improve agricultural productivity by promoting the improvement of human capital. In conclusion, it can be concluded that the Internet use can improve agricultural green production efficiency mainly by affecting agricultural green technological progress and technical efficiency improvement.
The results of the threshold regression model show that there is a nonlinear relationship between Internet use and agricultural green production efficiency. From a dynamic perspective, Internet use and agricultural green production efficiency have a “U-shaped” relationship. When the household expenditure-income ratio of farmers is bigger than the threshold value, the Internet use will improve agricultural green production efficiency. Some scholars have come to the same conclusion (Hu et al., 2020; Liu et al., 2020). For example, in the study of the impact of agricultural innovation on agricultural green production efficiency, He et al. (2021) found that technical progress showed a trend of decreasing first and then increasing. As the main driving method of production efficiency, agricultural green production efficiency also shows the same “U-shaped” trend of change; the main reason for this phenomenon is that when the ratio of expenditure to income is below the threshold value, the corresponding infrastructure in rural areas is not perfect, the human capital in rural areas is poor, and there are fewer professional and technical personnel, and household expenditure accounts for a small proportion of income at this stage, farmers would choose to use most of the spending in agricultural production, extensive production mode is still adopted. The above reasons lead to the negative impact of the use of the Internet on the progress of agricultural technology and the improvement of technical efficiency, which will further reduce agricultural green production efficiency. When the household expenditure-income ratio of farmers is beyond the threshold value, farmers can obtain sufficient agricultural production information through the Internet use and purchase modern machinery and equipment with their extra expenditures, which can help farmers to change their production behavior. Optimizing the allocation of agricultural resources is conducive to the progress of agricultural technology and the improvement of agricultural technical efficiency. Some studies have pointed to similar conclusions (Deng et al., 2022; Shen et al., 2022; He et al., 2021). Lu et al. (2022) pointed out that labor input can improve agricultural green production efficiency by studying the nonlinear relationship between agricultural insurance and agricultural green production efficiency. He et al. (2021) believed that the degree of agricultural mechanization and the education level of farmers would significantly impact the “U-shaped” change of agricultural green production efficiency. Shen et al. (2022) believe rural infrastructure construction will improve agricultural green production efficiency. Based on the above empirical analysis results, it shows that the development of the rural Internet is currently in the right half of the “ U ” shape, in the growth period, and has passed the initial period of fake and inferior products.
In this paper, the SBM model is used to calculate the agricultural green production efficiency by using the observation data of 459 farmers in Shaanxi, Shanxi, and Henan provinces. The Tobit model is used to analyze the influencing factors of agricultural green production efficiency. In addition, considering the endogeneity and heterogeneity, the paper applies the endogenous switching regression model to explore the effect of using the Internet on agricultural green production efficiency. Finally, the threshold regression model is used to discuss the nonlinear impact of Internet use on agricultural green production efficiency in different stages. The main conclusions are as follows:
First, the main peak of agricultural green production efficiency is distributed in a low-level range, indicating much room for improvement in agricultural green production efficiency. Second, based on the Tobit analysis and counterfactual assumptions, the agricultural green production efficiency of farmers who do not use the Internet will increase by 41.45% if they use the Internet, indicating that the use of the Internet can improve agricultural green production efficiency. The impact of the Internet on agricultural green production efficiency is positively moderated by rural infrastructure, household assets, and educational attainment. Age and arable land area significantly impact agricultural green production efficiency. Thirdly, the threshold regression results show that the impact of internet use on agricultural green production efficiency is nonlinear, and the threshold value of the ratio of farmers’ expenditure to income is 0.0886.
In order to strengthen the effect of Internet use on agricultural green production efficiency and promote sustainable development of agriculture, we put forward the following policy recommendations:
First, strengthening the emphasis on increasing agricultural green production efficiency. Agricultural green production efficiency is a key indicator for evaluating the agricultural environment and development level. At present, agricultural green production efficiency in rural areas is low, which needs more attention from many aspects. At the institutional level, we should build a production concept dominated by green production. At the developer level, it is necessary to accelerate the research and development of green production technology. At the level of farmers, it is necessary to improve the cognitive level of green production to promote the adoption of green production technology.
Second, increase investment in rural human capital, especially in the education level of farmers. The transformation of green agricultural development requires higher human capital, and improving the quality of rural labor is urgent. It is suggested to improve human capital by encouraging farmers to receive higher education and organizing farmers to participate in skills training.
Third, the government should provide relevant support to ensure the development of agricultural green production. The government should provide green production subsidies for agricultural producers and encourage farmers to adopt green production skills. Strengthen supervision and ensure the quality and safety of agricultural products. At the same time, improve infrastructure construction in rural areas, improve the “first-kilometer” system for products in rural areas to leave villages and enter cities, and promote rural economic development.
Last but not least, the government should integrate and enrich agricultural-related information on the Internet to ensure the accuracy and accessibility of the information and encourage farmers to use the Internet to learn agricultural green production skills.
Besides, the research is preliminary and has some limitations. First, the research only focuses on corn production, and thus, the conclusion should be applied to other crops with cautions. Further research should pay more attention to other crops. Second, the sample size in this study remains relatively small due to the limitation of research funds. All sample farm households were selected in only three provinces. In the context, a degree of caution is important when extrapolating the conclusion of this study to other regions of China. Third, using cross-section data makes it difficult to examine the dynamic impact of Internet use on agricultural green production efficiency. Further research can be analyzed using panel data from all regions of China.
The original contributions presented in the study are included in the article/Supplementary Material, further inquiries can be directed to the corresponding author.
HY: Methodology, Conceptualization, Writing-original draft, writing-review & editing. XB: Methodology, Conceptualization, Writing-review & revision, Supervision, Project administration. HZ: Writing-revision, supervision.
This study was funded by National Key R&D Program of China (2022YFE0115300), Ministry of Education of Humanities and Social Science Foundation (22YJA790001), Chinese Universities Scientific Fund (2452022061; 2452019157; 2452021222), Shaanxi Soft Science Foundation (2022KRM068), Youth Innovation Team of Shaanxi University.
The authors would like to thank the referees for their helpful suggestions and corrections on the earlier draft of our paper.
The authors declare that the research was conducted in the absence of any commercial or financial relationships that could be construed as a potential conflict of interest.
All claims expressed in this article are solely those of the authors and do not necessarily represent those of their affiliated organizations, or those of the publisher, the editors and the reviewers. Any product that may be evaluated in this article, or claim that may be made by its manufacturer, is not guaranteed or endorsed by the publisher.
Adetutu, M. O., and Ajayi, V. (2020). The impact of domestic and foreign R&D on agricultural productivity in sub-Saharan Africa. World Dev. 125, 104690. doi:10.1016/j.worlddev.2019.104690
Bravo-Ureta, B. E., Moreira, V. H., Troncoso, J. L., and Wall, A. (2020). Plot-level technical efficiency accounting for farm-level effects: Evidence from Chilean wine grape producers[J]. Agric. Econ. (United Kingdom) 51, 811–824. doi:10.1111/agec.12593
Chandio, A. A., Akram, W., Sargani, G. R., Twumasi, M. A., and Ahmad, F. (2022b). Assessing the impacts of meteorological factors on soybean production in China: What role can agricultural subsidy play? [J]. Ecol. Inf. 71, 101778. doi:10.1016/j.ecoinf.2022.101778
Chandio, A. A., Jiang, Y. S., Rehman, A., and Rauf, A. (2020). Short and long-run impacts of climate change on agriculture: An empirical evidence from China. Int. J. Clim. Chang. Strateg. Manag. 12, 201–221. doi:10.1108/IJCCSM-05-2019-0026
Chandio, A. A., Sethi, N., Dash, D. P., and Usman, M. (2022a). Towards sustainable food production: What role ICT and technological development can play for cereal production in asian-7 countries? [J]. Comput. Electron. Agric. 202, 107368. doi:10.1016/j.compag.2022.107368
Chen, Y., Miao, J., and Zhu, Z. (2021). Measuring green total factor productivity of China's agricultural sector: A three-stage SBM-DEA model with non-point source pollution and CO2 emissions. J. Clean. Prod. 318 (18), 128543. doi:10.1016/j.jclepro.2021.128543
Chen, S. N., and Zhou, X. B. (2011). Semiparametric estimation of a bivariate Tobit model. J. Econom. 165 (2), 266–274. doi:10.1016/j.jeconom.2011.07.005
Chi, Y. Y., Xu, Y. M., Wang, X., Jin, F., and Li, J. L. (2021). A win–win scenario for agricultural green development and farmers’ agricultural income: An empirical analysis based on the EKC hypothesis. Sustainability 13, 8278. doi:10.3390/su13158278
Cole, S. A., and Fernando, A. N. (2012). The value of advice: Evidence from mobile phone-based agricultural extension. doi:10.22004/ag.econ.160520
Cui, Y., Liu, W. X., Khan, S. U., Cai, Y., Zhu, J., Deng, Y., et al. (2020). Regional differential decomposition and convergence of rural green development efficiency: Evidence from China. Environ. Sci. Pollut. Res. 27, 22364–22379. doi:10.1007/s11356-020-08805-1
Deng, H. Y., Jing, X. N., and Shen, Z. Y. (2022). Internet technology and green productivity in agriculture. Environ. Sci. Pollut. Res. Int 6, 22. doi:10.1007/s11356-022-21370-z
Fare, R., Grosskoprf, S., and Norris, M. (1994). Productivity growth, technical progress, and efficiency change in industrialized countries[J]. Am. Econ. Rev. 84 (1), 66–83.
Gao, Q., Cheng, C. M., Sun, G. L., and Li, J. F. (2022). The impact of digital inclusive finance on agricultural green total factor productivity: Evidence from China. Front. Ecol. Evol. 10, 905644. doi:10.3389/fevo.2022.905644
Hansen, B. E. (2000). Sample splitting and threshold estimation. Econometrica 68 (3), 575–603. doi:10.1111/1468-0262.00124
He, W. C., Li, E. L., and Cui, Z. Z. (2021). Evaluation and influence factor of green efficiency of China’s agricultural innovation from the perspective of technical transformation. Chin. Geogr. Sci. 31, 313–328. doi:10.1007/s11769-021-1192-x
Hu, J. F., Wang, Z., and Huang, Q. H. (2020). Factor allocation structure and green-biased technological progress in Chinese agriculture[J]. Econ. Research-Ekonomska Istraz. 34, 2034–2058. doi:10.1080/1331677X.1860795
Huang, X. Q., Feng, C., Qin, J. H., Wang, X., and Zhang, T. (2022). Measuring China's agricultural green total factor productivity and its drivers during 1998-2019[J]. Sci. Total Environ. 829, 154477. doi:10.1016/j.scitotenv.2022.154477
Jasper, B., Andrea, C., and Wilko, L. (2019). Energy performance and capital expenditures in manufacturing industries. Energy Effic. 12 (8), 2011–2038. doi:10.1007/s12053-019-09779-x
Kaila, H. D., and Tarp, F. (2019). Can the internet improve agricultural production? Evidence from Viet Nam[J]. Agric. Econ. 50, 675–691. doi:10.1111/agec.12517
Li, J. D., and Lin, Q. N. (2022). Can the adjustment of China's grain purchase and storage policy improve its green productivity? [j]. Int. J. Environ. Res. Public Health 19, 6310. doi:10.3390/ijerph19106310
Li, J., Ma, W., Renwick, A., and Zheng, H. (2020). The impact of access to irrigation on rural incomes and diversification: Evidence from China. China Agric. Econ. Rev. 12, 705–725. doi:10.1108/caer-09-2019-0172
Li, K., and Lin, B. Q. (2016). Impact of energy conservation policies on the green productivity in China's manufacturing sector: Evidence from a three-stage DEA model[J]. Appl. Energy 168, 351–363. doi:10.1016/j.apenergy.2016.01.104
Li, Y., and Chen, Y. Y. (2021). Development of an SBM-ML model for the measurement of green total factor productivity: The case of pearl river delta urban agglomeration. Renew. Sustain. Energy Rev. 145, 111131. doi:10.1016/j.rser.2021.111131
Liu, D. D., Zhu, X. Y., and Wang, Y. F. (2020). China's agricultural green total factor productivity based on carbon emission: An analysis of evolution trend and influencing factors. J. Clean. Prod. 278, 123692. doi:10.1016/j.jclepro.2020.123692
Liu, H. M., Wen, S. B., and Zhang, Z. (2022). Agricultural production agglomeration and total factor carbon productivity: Based on NDDF–MML index analysis. China Agric. Econ. Rev. 14, 709–740. doi:10.1108/CAER-02-2022-0035
Liu, S., Chen, X. M., and Shang, J. (2021). Research on the degree of coupling of the internet development level and agricultural——ecological efficiency based on 2009–2018 data from 13 major grain-producing areas in China. Plos One 16 (7), e0254078. doi:10.1371/journal.pone.0254078
Lu, F., Wang, W. W., Liu, M. H., Liu, M., and Qi, D. Y. (2022). The non-linear effect of agricultural insurance on agricultural green competitiveness[J]. Technol. Analysis Strategic Manag 7, 8. doi:10.1080/09537325.2022.2098102
Mario, G. F., Boris, E. B. U., Daniel, S., and Paul, W. (2014). The impact of high value markets on smallholder productivity in the ecuadorean sierra: A stochastic production frontier approach correcting for selectivity bias. Food policy 44, 237–247. doi:10.1016/J.foodpol.2013.09.014
Ogutu, S. O., Okello, J. J., and Otieno, D. J. (2014). Impact of information and communication technology-based market information services on smallholder farm input use and productivity: The case of Kenya. World Dev. 64 (104482), 311–321. doi:10.1016/j.worlddev.2014.06.011
Onofri, A., and Fulginiti, L. E. (2008). Public inputs and dynamic producer behavior: Endogenous growth in U.S. Agriculture. J. Product. Anal. 30 (1), 13–28. doi:10.1007/s11123-008-0093-7
Shen, Z. Y., Wang, S. K., Boussemart, J. P., and Hao, Y. (2022). Digital transition and green growth in Chinese agriculture[J]. Technol. Forecast. Soc. Change 181, 121742. doi:10.1016/j.techfore.2022.121742
Sun, X., and Shu, K. L. (2021). Application research of perception data fusion system of agricultural product supply chain based on Internet of things. EURASIP J. Wirel. Commun. Netw. 2021, 138. doi:10.1186/s13638-021-02014-1
Tang, L. Q., Zhou, J. H., Ihtiyor, B. J., Zhang, Y. J., and Thomas, G. (2018). Induce or reduce? The crowding-in effects of farmers’ perceptions of climate risk on chemical use in China[J]. Clim. Risk Manag. 28, 27–37. doi:10.1016/j.crm.2018.02.001
Tao, F., Zhang, H. Q., Hu, J., and Xia, X. H. (2016). Dynamics of green productivity growth for major Chinese urban agglomerations[J]. Appl. Energy 196, 170–179. doi:10.1016/j.apenergy.2016.12.108
Wang, C. S. (2018). An analysis of rural household livelihood change and the regional effect in a western impoverished mountainous area of China. Sustainability 10 (6). doi:10.3390/su10061738
Wu, H. T., Yan, X., Yu, H., and Ren, S. (2021a). How does internet development affect energy- saving and emission reduction? Evidence from China[J]. Energy Econ. 103, 105577. doi:10.1016/j.eneco.2021.105577
Wu, H. T., Yu, H., Ren, S. Y., Yang, X., and Xie, G. (2021b). Does internet development improve green total factor energy efficiency? Evidence from China[J]. Energy Policy 153, 112247. doi:10.1016/j.enpol.2021.112247
Wu, L., and Zhang, Z. G. (2020). Impact and threshold effect of Internet technology upgrade on forestry green total factor productivity: Evidence from China. J. Clean. Prod. 271, 122657. doi:10.1016/j.jclepro.2020.122657
Xie, F. M., Zhang, B., and Wang, N. (2021). Non-linear relationship between energy consumption transition and green total factor productivity: A perspective on different technology paths. Sustain. Prod. Consum. 28, 91–104. doi:10.1016/j.spc.2021.03.036
Xu, X. C., Huang, X. Q., Huang, J., Gao, X., and Chen, L. H. (2019). Spatial-temporal characteristics of agriculture green total factor productivity in China, 1998–2016: Based on more sophisticated calculations of carbon emissions. Int. J. Environ. Res. Public Health 16, 3932. doi:10.3390/ijerph16203932
Yang, Y., Ma, H., and Wu, G. S. (2022). Agricultural green total factor productivity under the distortion of the factor market in China. Sustainability 14, 9309. doi:10.3390/su14159309
Zhai, X. Q., Xue, R., He, B., Yang, D., Pei, X. Y., Li, X., et al. (2022). Dynamic changes and convergence of China's regional green productivity: A dynamic spatial econometric analysis. Adv. Clim. Change Res. 13, 266–278. doi:10.1016/j.accre.2022.01.004
Zhang, H. Q., Chandio, A. A., Yang, F., Tang, Y. S., Twumasi, M. A., and Sargani, G. R. (2022b). Modeling the impact of climatological factors and technological revolution on soybean yield: Evidence from 13-major provinces of China. Int. J. Environ. Res. Public Health 19, 5708. doi:10.3390/ijerph19095708
Zhang, H. Q., Tang, Y. S., Abbas Ali Chandio, A. A., Sargani, G. R., and Twumasi, M. A. (2022a). Measuring the effects of climate change on wheat production: Evidence from northern China. Int. J. Environ. Res. Public Health 19, 12341. doi:10.3390/Ijerph191912341
Zhang, J. D., Qu, X. Y., and Sangaiah, A. K. (2018). A study of green development mode and total factor productivity of the food industry based on the industrial internet of things. IEEE Commun. Mag. 56 (5), 72–78. doi:10.1109/MCOM.2018.1700789
Zheng, H. Y., Ma, W. L., Wang, F., and Li, G. (2021). Does internet use improve technical efficiency of banana production in China? Evidence from a selectivity-corrected analysis[J]. Food Policy 102, 102044. doi:10.1016/j.foodpol.2021.102044
Zheng, Y. Y., Fan, Q. Q., and Jia, W. (2022a). How much did internet use promote grain production? -Evidence from A survey of 1242 farmers in 13 provinces in China[J]. Foods 11, 1389. doi:10.3390/Foods11101389
Zheng, Y. Y., Zhu, T. H., and Jia, W. (2022b). Does internet use promote the adoption of agricultural technology? Evidence from 1449 farm households in 14 Chinese provinces[J]. J. Integr. Agric. 21 (1), 282–292. doi:10.1016/s2095-3119(21)63750-4
Zhou, Y. Y., XuLiuFang, Y. R. C. Z. Z. Q., Fu, X. Y., He, M. Z., and He, M. (2019). The threshold effect of China’s financial development on green total factor productivity. Sustainability 11, 3776. doi:10.3390/su11143776
Zhu, X. K., Hu, R. F., Zhang, C., and Shi, G. (2021). Does internet use improve technical efficiency? Evidence from apple production in China[J]. Technol. Forecast. Soc. Change 166, 120662. doi:10.1016/j.techfore.2021.120662
Keywords: agricultural green production efficiency, internet use, endogenous switching regression model, threshold regression model, nonlinear relationship
Citation: Yu H, Bai X and Zhang H (2022) Strengthen or weaken? Research on the influence of internet use on agricultural green production efficiency. Front. Environ. Sci. 10:1018540. doi: 10.3389/fenvs.2022.1018540
Received: 13 August 2022; Accepted: 10 October 2022;
Published: 28 October 2022.
Edited by:
Lin Zhang, City University of Hong Kong, Hong Kong SAR, ChinaReviewed by:
Yao An, Tsinghua University, ChinaCopyright © 2022 Yu, Bai and Zhang. This is an open-access article distributed under the terms of the Creative Commons Attribution License (CC BY). The use, distribution or reproduction in other forums is permitted, provided the original author(s) and the copyright owner(s) are credited and that the original publication in this journal is cited, in accordance with accepted academic practice. No use, distribution or reproduction is permitted which does not comply with these terms.
*Correspondence: Xiuguang Bai, YmFpeGc5NjBAbndzdWFmLmVkdS5jbg==
Disclaimer: All claims expressed in this article are solely those of the authors and do not necessarily represent those of their affiliated organizations, or those of the publisher, the editors and the reviewers. Any product that may be evaluated in this article or claim that may be made by its manufacturer is not guaranteed or endorsed by the publisher.
Research integrity at Frontiers
Learn more about the work of our research integrity team to safeguard the quality of each article we publish.