- 1School of Foreign Studies, Suzhou University, Suzhou, Anhui, China
- 2Business School, Henan University, Jinming Campus, Kaifeng, China
- 3Department of Economics and Management, Université Félix Houphouët-Boigny, Abidjan, Cote d’Ivoire
- 4School of Accounting, Dongbei University of Finance and Economics, Dalian, China
- 5Office of People’s Bank of China (PBC), Suzhou, Anhui, China
The International Energy Agency revealed in its report in 2021 that the world energy-CO2 emission reached its highest peak during that year. This statistic shows the need to set new environmental protection policies and improve the current ones. Hence, our study aims to highlight the leading role of human capital in the fight against climate change. To do so, by using the Feasible Generalized Least Squares and the Two-Stage Least Squares estimators, we analyze the moderating impact of human capital on the link between renewable energy, nonrenewable energy, economic growth, and CO2 emissions in the case of the 20 newly emerging market economies for the period 1990–2021. We find negative effects of renewable energy consumption, industrialization and trade openness on CO2 emissions. We also find positive effects of nonrenewable energy consumption, economic growth, and human capital on CO2 emissions. In addition, our findings reveal that renewable energy consumption and human capital are complementary levers for reducing CO2 emissions, whereas human capital mitigates the detrimental effect of nonrenewable energy consumption on environmental quality. Besides, the results underline that human capital has an inverted U-shaped effect on CO2 emissions. Regarding policy implications, policymakers should emphasize the complementarity between human capital and renewable energy consumption by facilitating the accumulation of human capital towards productive investments and the use of renewable energy technologies in these countries. They should also raise people’s environmental awareness by implementing educational reforms, effective environmental awareness campaigns, and government regulatory pressures that help people understand global warming issues and adopt environmentally friendly practices in their daily activities. Finally, policymakers should promote the use of renewable energy rather than non-renewable energy sources in the production of goods and services by obliging countries to integrate green policies into their industrialization and trade openness strategies.
1 Introduction
In 2021, global energy-related CO2 emissions reached their highest level (IEA, 2021), which consequently has a negative impact on the environment. This statistic recalls the urgency of the climate situation and the necessity to manage sustainable development. Indeed, it is clear that the world is facing a deterioration of ecological conditions, mainly due to the evolution of people’s lifestyles. In order to combine changing lifestyles and economic practices with environmental preservation, economic growth, and social welfare (sustainable development), it is vital to educate the population about the importance of climate change. In effect, as the population grows, its impact on the environment increases. This growth of the population means an increase in the urbanization level, the need for water and more valuable resources, and the need for land, infrastructures, industry, and energy. Thus, human activities play a huge role in environmental degradation. Government should therefore implement appropriate policies to facilitate the transition from development to sustainable development. One policy should be the improvement of human capital which will help understand the environmental pollution issues and improve energy efficiency (Pablo-Romero and Jesus, 2015). Another policy should encourage renewable energy instead of nonrenewable or fossil fuel energy, especially in urban areas. In fact, urbanization is responsible for 70% of energy-related global CO2 emissions (IEA, 2017). This is because the urban population consumes more energy in transportation, infrastructure development, sanitation, sewages, and houses.
Looking for the best way to manage energy consumption and CO2 emissions, we analyze the link between renewable energy, nonrenewable energy, economic growth, industrialization, trade openness, human capital, and CO2 emissions in the case of the newly emerging market economies. In effect, we consider these countries in our study because these newly emerging market economies1 have witnessed economic improvements and transformation in the current century. This improvement mainly includes strong economic growth, high per capita income, industrialization, and urbanization. According to the World Development Report (2019), countries could not achieve sustained economic growth without strengthening their human capital. Thus, we also study the effect of human capital on these variables. Although many studies have analyzed the role of renewable energy, nonrenewable energy, governance quality, and human capital on economic growth and CO2 emissions (Yao et al., 2020; Kassi et al., 2020; Shahnazi and Shabani, 2021; Kassi et al., 2021), none of them have combined human capital with the main studied variables to analyze its conditional and nonlinear impacts on the relationships between renewable energy, nonrenewable energy, economic growth, and environmental quality. Therefore, this investigation explores the association between various energy sources, human capital, economic growth, and CO2 emissions for sustainable development in the newly emerging market economies. In so doing, we contribute to the literature on environmental protection and sustainable development in several aspects. First, we examine this relationship in the newly emerging market economies. Second, we include human capital in this analysis, highlighting its conditional effect on the relationship between renewable energy, economic growth and CO2 emissions. Third, this paper examines the mitigating effect of human capital on the nonrenewable energy-economic growth-CO2 emissions nexus. Fourth, we analyze the nonlinear effect of human capital on environmental quality. This study also examines the impacts of urbanization, industrialization, and trade openness on environmental quality, given their growing influences on sustainable development across countries. Finally, we use two econometric techniques, i.e., the feasible generalized least squares (FGLS) and the two-stage least squares (2SLS) estimators. The former generates robust estimates in the presence of cross-sectional dependence and serial correlation, while the latter overcomes endogeneity issues in the results.
We focus on the newly emerging market economies because of their dynamic economic growth and abundant natural resources in terms of renewable energies, but also because of the environmental challenges posed by the increasing urbanization of these countries to meet the needs of their galloping population. Thus, the policy implications of this study will contribute to the sustainable development of these countries and improve the quality of the environment around the world. The remainder of this study is structured as follows. The literature review is presented in Section 2. Section 3 describes the material and methods. In Section 4, we present and discuss our empirical results. Section 5 concludes the paper by providing policy recommendations and areas for future studies.
2 Literature review
The link between energy, especially renewable energy, nonrenewable energy and CO2 emissions, has been deeply analyzed through different studies. Nevertheless, the moderating impact of human capital on the relationship between these variables, which is the main contribution of our study, has not been analyzed in previous studies. In fact, empirical studies mainly highlighted the nexus between energy-CO2 emissions (Timmons et al., 2014; Bilan et al., 2019; Mohsin et al., 2021). Another research gap in the literature is that most previous authors failed to take the newly emerging market economies into account in their analyses, but also they did not consider the conditional and nonlinear effects of human capital on the nexus between renewable energy, nonrenewable energy, economic growth and CO2 emissions. For instance, Shahnazi, and Shabani (2021) considered the European Union in their analysis, while Mahalik et al. (2021) focused on BRICS countries. Lastly, the authors did not or hardly consider cross-dependence analysis in their studies. They used methods such as the ARDL (Pata, 2018), PMG-ARDL (Berkun et al., 2019), the dynamic fixed effect and GMM (Muhammad et al., 2021), the FMOLS and Markov switching regression model (Feng, 2022), and the DOLS (Dogan and Seker, 2016).
Timmons et al. (2014) considered that the higher price of renewable energy contributes to the population’s dependence on fossil fuels. Thus, policymakers should implement policies to reduce the price of renewable energy and encourage the development of pro-environmental technologies. The study by Chen et al. (2019) shows a negative link between renewable energy, trade, and CO2 emissions. Using a Kuznets Curve hypothesis, Dong et al. (2018) found that renewable energy negatively affects carbon emissions. For Shahnazi and Shabani (2021), renewable energy mitigates CO2 emissions for the period 2000–2017. Studying the 50 largest countries, Mendonça et al. (2020) found that growth and population contribute to CO2 emissions, unlike renewable energy. Pata (2018) also showed the existence of an inverted U-shaped relationship between GDP/k and CO2 emissions. He also found that renewable energy does not affect CO2 emissions, and urbanization contributes to environmental degradation. For Awosusi et al. (2022), globalization and renewable energy mitigated CO2 emissions in Colombia. Using quarterly data, Adebayo and Kirikkaleli (2021) found that renewable energy mitigates CO2 emissions in Japan. Through a dynamic fixed effect and GMM estimators, Muhammad et al. (2021) showed that renewable energy contributes to environmental quality. Analyzing BRICS countries, Dingru et al. (2021) found that renewable energy reduces CO2 emissions. For Mohsin et al. (2021), it will be possible to mitigate environmental degradation using continuously renewable energy. Feng (2022), using FMOLS and Markov switching regression model, found a two-way causal relationship between renewable energy and trade openness. They also found that green energy and trade openness contribute to environmental protection.
Concerning the nonrenewable energy-CO2 emissions nexus, Dogan and Seker (2016) employed dynamic ordinary least squares and found that renewable energy and trade mitigates CO2 emissions. Also, nonrenewable energy positively affects CO2 emissions, supporting the EKC hypothesis.
Shafiei and Salim (2014) used the STIRPAT model to analyze OECD countries and found that nonrenewable energy increases CO2 while renewable energy decreases CO2. They also highlight the existence of EKC between urbanization and CO2. For Awodumi and Adewuyi (2020), there is a positive relationship between nonrenewable energy and carbon emissions. Studying BRICS countries from 1990 to 2015, Mahalik et al. (2021) found a positive link between nonrenewable energy consumption, primary education, economic growth and CO2 emissions, while renewable energy consumption, urbanization, and secondary education reduce carbon emissions. Oke et al. (2021) found the same results while analyzing 51 African countries from 1990 to 2015. In their work, Sarkodie and Ozturk (2020) provided strong support for EKC. They also showed the positive impact of energy consumption on CO2 emissions and a negative between renewable energy and CO2 emissions. Raza and Shah (2018) investigated the role of renewable energy consumption in the context of the EKC hypothesis in a panel of G-7 countries from 1991 to 2016. While investigating the EKC hypothesis, the study employed the dynamic ordinary least squares (DOLS), fully modified ordinary least squares (FMOLS), and the fixed effects ordinary least squares regression (FE OLS) to establish evidence of cointegration. The study found that, in the case of renewable energy consumption, the development of renewable energy in the panel of G7 countries was a significant factor for long-term decarbonization policy. While incorporating trade indicators together with renewable energy consumption and per capita GDP, the empirical results supported the validity of the EKC hypothesis in the G-7 countries.
Among other studies that have examined the link between renewable energy-CO2, we note Zoundi (2017), Ito (2017), Cai et al. (2018), and Lau et al. (2019), among others. The results of these studies largely support the CO2- mitigating effect of renewable energy consumption. However, most of these studies failed to include the role of human capital, which may have far-reaching implications for environmental quality. Szetela et al. (2022) analyze the relationship between renewable energy and CO2 emissions in top natural resource-depending countries over the period 2000–2015. An essential contribution of this study is to assess the role of Governance. The Ordinary Least Squares Fixed Effects Generalized Least Squares methods and two-step GMM estimators are used for panel data. The empirical results show that renewable energy has a significant negative impact on per capita CO2 emissions. They also find that renewable energy consumption decreases CO2 emissions faster in countries with higher rule of law and voice and accountability. Besides, Gross domestic product per capita has an inverted U-shaped relationship with CO2 emissions. In an earlier study, Qi et al. (2014) for China found that renewable energy targets may lead to a nearly 1.8% decrease in CO2 emissions from 2010 to 2020. Inglesi-Lotz and Dogan (2018) assess the relationship between renewable energy and CO2 emissions in the top 10 electricity-producing countries in Sub-Saharan Africa for 1980–2011. The study documents the long-run relationship between GDP, renewable energy, nonrenewable energy and CO2 emissions. Moreover, there is causality running from renewable energy to CO2 emissions and from CO2 emissions to trade. Fatima et al. (2021) contribute to extant research by exploring the relationship between GDP, renewable energy, and CO2 emissions using global panel data. Using various econometric methods, the study shows that GDP moderates the relationship between renewable energy and CO2 emissions. At the same time, GDP has effect on nonrenewable energy consumption, which in turn also increases CO2 emissions.
Regarding the human capital-CO2 emissions link, we found a very few studies. Desha et al. (2015) stated that human capital increases renewable energy consumption through education. It can also help produce fewer polluting goods (Hartman and Kwon, 2005). Bano et al. (2018) found that improving human capital will reduce carbon emissions without decreasing economic growth. Besides, in a company, highly educated employees use new technologies for cleaner production and make practical efforts in environmental management and compliance (Dasgupta et al., 2000). Wang et al. (2021) considered that human capital contributes to reducing emissions in an economy. Studying 11 European Union countries, Bayar et al. (2022) showed a negative link between human capital and carbon dioxide in Croatia, the Czech Republic, Hungary, and Slovenia, while its impact is positive in Latvia and Lithuania. For Yao et al. (2020), advanced human capital linked to the years of schooling, negatively impacts CO2 emissions. They also found that this relationship was positive before the 1950s and negative after 1950. Li and al. (2022), examining the nexus human capital-CO2, found that an increase in education reduces CO2, and a decrease in education increase CO2 emissions.
Based on these analyses, we formulate the following hypotheses:
H1: Renewable energy consumption and human capital are complementary levers for reducing CO2 emissions
H2: Human capital mitigates the positive effect of nonrenewable energy consumption on CO2 emissions
H3: Human capital has an inverted U-shaped effect on CO2 emissions
3 Materials and methods
3.1 Database
In this work, we examine the data of the 20 newly emerging market economies2 for the period 1990–2021. These data presented in Table 1 are from the World Development Indicators (World Bank, 2021) and the Penn World Table, version 10.0 (Feenstra, Inklaar Timmer, 2015) and have been used according to their availability. Our dataset includes the following variables:
• CO2 emissions (metric tons per capita), which is considered as the level of pollution per inhabitant in the studied countries;
• Human capital index, based on years of schooling and returns to education;
• Gross domestic product per capita (GDP/k), which is the sum of gross value added by all resident producers in the economy plus any product taxes and minus any subsidies not included in the value of the products;
• Renewable energy consumption (RENEW), which is the share of renewable energy in total final energy consumption;
• Nonrenewable energy consumption (NONRENE), which is fossil fuel energy consumption in the percentage of total consumption;
• Urban population (URBAN), which is the urban population as a percentage of the total population. It is used as the indicator of urbanization;
• Industry (including construction), value-added in the percentage of GDP, which is considered as the industrialization rate in the studied countries;
• Trade openness (TRAD) refers to the outward or inward orientation of a country’s economy in international trade.
3.2 Theoretical model
The following equation is based on the Stochastic Impact by Regression on Population, Affluence, and Technology (STIRPAT) model developed by Dietz and Rosa (1997). This model is inspired by the IPAT model of Ehrlich and Holdren (1971), in which I represents the environmental impact, p, the population size, A the affluence and T, the level of technology.
Where: the parameter a is the constant term, and b, c and d denote, the parameters of p, A and T respectively. The variable
As urbanization is one of the main variables influencing CO2 emissions (Shahbaz et al. (2015), we include this variable to the STIRPAT model. We obtain the following model:
Where: the subscript i (i = 1, 2, …, n) refers to countries. Meanwhile, the subscript t (t = 1, 2, …, T) refers to the time period. By taking the logarithmic form of both sides of model (1), the new model (2) is a linear specification which reduces the correlation between variables. In our empirical models, in line with Assamoi et al. (2020), we adopt carbon dioxide per capita as a dependent variable to describe the environmental impact.
Affluence is represented by per capita GDP (GDP/k), technology, by renewable (RENE) and nonrenewable (NONRE) energy consumption, whereas human capital (HC) is used to denote population. Then, we add some control variables to our theoretical model, such as: trade openness (TRAD), following Dogan and al. (2015); industrialization (IND), following Butkiewicz and Yanikkaya (2011). Trade openness contributes to CO2 emissions through technology transfer and industrialization. Industrialization can increase CO2 emissions through nonrenewable energy consumption and can also reduce it through renewable energy.
After including the studied variables, we obtain the following model:
Where a is the constant term, and
In addition, several studies have highlighted the impact of the energy mix (renewable energy versus nonrenewable energy) on CO2 emissions.
Most studies show that renewable energy is one of the key drivers of sustainable development by decreasing carbon emissions (Dingru et al., 2021; Kassi D. F. et al., 2022; Feng, 2022), unlike the detrimental impact of nonrenewable energy consumption on environmental quality (Dogan and Seker, 2016; Le et al., 2020; Ibrahim and Ajide, 2021).
We argue that human capital remains one of the main factors in transforming a country’s energy mix by facilitating the development of technological capabilities, new energy transformation processes emphasizing the green economy, and the efficient management of energy resources.
Its development also contributes to the renewal of mentalities and the emergence of a highly qualified workforce capable of implementing green policies toward greater use of renewable energies in the production of goods and services to fight against environmental degradation.
This study extends the findings of previous studies showing the beneficial effect of human capital accumulation on the reduction of CO2 emissions (Yao et al., 2020; Wang and Xu, 2021; Li and Ullah, 2022).
Unlike these studies, we particularly analyze the conditional effect of human capital accumulation on the link between renewable energy, nonrenewable energy, and CO2 emissions in multivariate models as follows:
Where
Thus, we examine whether human capital and renewable energy consumption are complementary
However, human capital accumulation may hinder any reducing effect of renewable energy if
Likewise, we analyze whether human capital accumulation annihilates any contributing impact of nonrenewable energy i.e., if
Moreover, we compute the marginal effects of renewable energy consumption and nonrenewable energy consumption on CO2 emissions as follows:
We estimate the marginal effects of renewable energy consumption
Given the average level of human capital, the negative marginal effects of renewable energy consumption and nonrenewable energy consumption on CO2 emissions will occur only if human capital accumulation exceeds the following thresholds:
Where:
Finally, we test the EKC hypothesis in the case of human capital by adding its quadratic term
Where:
Following model (10), we also compute the marginal effect of human capital
Where:
3.3 Cross-sectional dependence and panel unit root tests
In the process of analyzing the relationship between the different variables, we use the cross-sectional dependence test proposed by Breusch-and Pagan (1980) and Pesaran (2004, 2007) to determine if we can use the conventional panel unit root tests or not.
The null hypothesis of the cross-dependence ADF panel unit root test (CADF) assumes the independence of the cross-section unit. In the case of the cross-dependence IPS panel unit root test (CIPS), the null hypothesis assumes that variables have unit root while the alternative hypothesis expresses stationarity.
3.4 Estimation methods
In the econometrical model, we apply the feasible generalized least squares (FGLS) estimator from Parks (1967) and Kmenta (1986) and the two-stage least squares (2SLS) estimator. The 2SLS estimator is used to address the endogeneity problem. This estimator is also used when the dependent variable error term is supposed to be correlated with the independent variables. Besides, this estimator is also robust to panel-specific autocorrelation and heteroscedasticity and permits correcting endogeneity and eliminating panel bias. Regarding the FGLS, it is used to control for slope heterogeneity and cross-sectional dependence. Moreover, this estimator is asymptotically efficient among the linear models because, in the presence of heteroskedasticity, serial and cross-sectional correlations, it consistently estimates the large error covariance matrix. However, this estimator is not suitable when checking endogeneity among variables.
4 Results and discussion of the study
4.1 Results of the study
4.1.1 Preliminary analysis
Table 2 provides a detailed description of the studied variables. In this work, we analyze 20 countries for 31 years. On average, these countries’ CO2 emissions rate is 0.53, while the urbanization rate is 1.765. In addition, the average levels of nonrenewable energy (1.902), industrialization (1.532), and trade openness (1.111) are higher than those of renewable energy (0.954) and human capital (0.374).
The results also show that, on average, the level of nonrenewable energy consumption (1.902) is higher than that of renewable energy consumption (0.954) in these countries. Besides, the median of urbanization (1.806) is three times higher than that of human capital (0.375). In addition, economic growth (GDP/k) is positive and above 2% in the studied countries (min = 2.722; max = 4.767). The low standard deviations of all the variables (less than 1) indicate a low level of dispersion around their mean between countries.
Table 3 shows that the variables lnNONRE, lnGDP/k, lnURB, lnHC, lnIND, lnTRAD have a positive and significant correlation with lnCO2. It also shows that the correlation between lnRENE and lnCO2 is negative and significant. The correlation between lnRENE and lnCO2 is −0.743, which indicates that these variables are strongly negatively correlated. Hence, more use of lnRENE reduces lnCO2.
Moreover, the correlation between lnNONRE, and lnCO2, and lnGDP/k and lnCO2 is 0.771 and 0.750. These values indicate that the correlation between these variables is positively strong.
The results of the multicollinearity test (Table 4) show that the variance inflation factor (VIF) is less than 10. This result confirms that there is no multicollinearity among these variables (El-Bannany, 2017).
The results of Table 5 show that there is a cross-sectional dependence among the panel time series. And the probability values of the three test statistics, which are less than 0.01, reject the null hypothesis of cross-sectional independence. Besides, the second-generation tests CADF and CIPS results (Table 6) confirm the stationarity of the variables.
4.1.2 Results of the FGLS and 2SLS estimators
In this analysis, as shown in the tables below, we used two estimators, the feasible generalized least squares (FGLS) and the two-stage least squares (2SLS). The first estimator overcame the cross dependence and serial correlation, while the second overcame the endogeneity problem. Besides, the FGLS estimator is suitable for this analysis because the number of countries (20) is lower than the analysis period (31).
The results of the different estimations are presented in Tables 7–Tables 10. The coefficients estimated from the FGLS and 2SLS methods can be interpreted as elasticity as all the variables were expressed in natural logarithms. The results provided by all the estimators are similar considering the coefficient signs and the significance level. The FGLS estimator findings show that the renewable energy consumption (lnRENE) has a negative impact on carbon dioxide emissions (lnCO2) and a 1% increase in lnRENE decreases lnCO2 by 0.27%.Also, industrialization (lnIND) and trade openness (lnTRAD) have a negative effect on CO2 emissions. That means a 1% increase in lnIND and lnTRAD decrease lnCO2 by 0.11 and 0.03%, respectively.
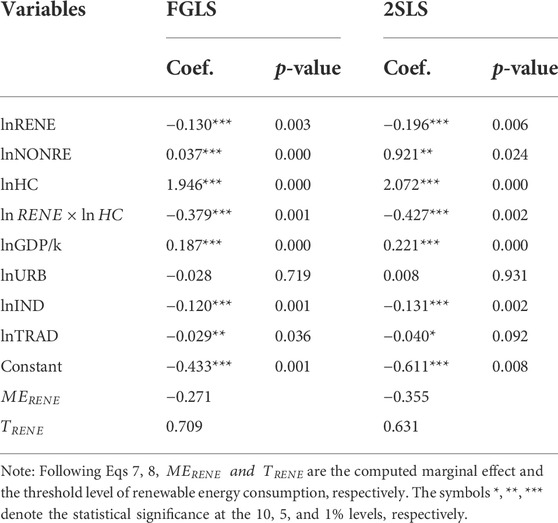
TABLE 8. Results of the moderating effect of human capital on the renewable energy-CO2 emissions nexus.
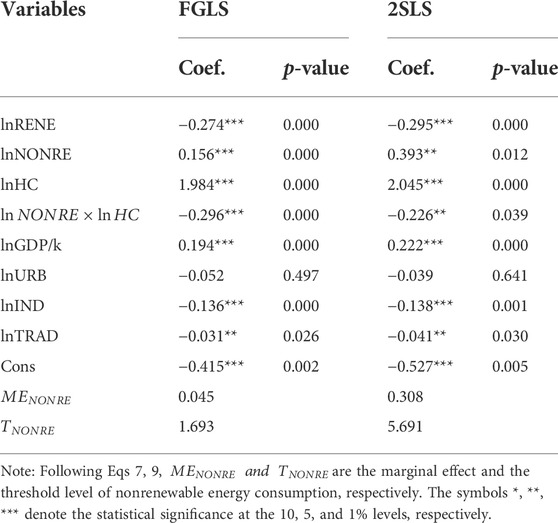
TABLE 9. Results of the moderating effect of human capital on the nonrenewable energy-CO2 emissions nexus.
In the cases of lnNONRE, lnGDP/k, and lnHC, the results show that these variables have a positive effect on lnCO2. And a 1% increase of these variables increases CO2 emissions by 0.05, 0.18, and 1.82%, respectively.
The results of the 2SLS estimator indicate the robustness of the main findings of this research, such as nonrenewable energy consumption, human capital, and GDP per capita, have a positive influence on CO2 emissions while renewable energy consumption, industrialization, and trade openness reduce CO2 emissions in the studied countries.
The next tables (Tables 8–Tables 10) highlight the effect of human capital on the different studied variables. Table 8 shows the joint effect of lnHC and lnRENE on lnCO2. We found that the combination of lnHC and lnRENE (lnRENE*lnHC) has a negative and significant effect on lnCO2 at the 1% level. The effect of lnRENE*lnHC (−0.379) is higher than those of lnRENE (−0.130) in terms of absolute values. This finding supports our hypothesis (H1), revealing that renewable energy consumption and human capital accumulation are complementary levers
Besides, Table 9 shows the joint effect of lnNONRE and lnHC on lnCO2.
We found that the combination of lnHC and lnNONRE (lnNONRE*lnHC) influence negatively lnCO2. And a 1% increase of lnNONRE*lnHC reduces lnCO2 by approximately 0.30%.
So, human capital accumulation annihilates the positive effect of nonrenewable energy consumption
Moreover, Table 10 presents the nonlinear effect of human capital on CO2 emissions. We found that the square of human capital (lnHC2) significantly negatively affects CO2 emissions at 10% level. A 1% increase in lnHC2 reduces lnCO2 by 0.80% approximately. This result corroborates our hypothesis (H3), showing that human capital has an inverted U-shaped effect on CO2 emissions in the studied countries. Therefore, our findings confirm the EKC hypothesis’s validity in the relationship between human capital and CO2 emissions.
Although this result is similar to the results of Yao et al. (2020), Li and Ullah (2022), our study extends these previous studies by revealing the nonlinear effect of human capital on CO2 emissions. Most previous studies ignore the nonlinear impact of human capital on CO2 emissions. Therefore, our conclusion reconciles the results of previous studies indicating the mixed effects of human capital accumulation on CO2 emissions.
The results of the effects of all independent variables on CO2 emissions are robust and consistent across the different models (Tables 7–Tables 10).
4.1.3 Robustness check
We use the fixed effects (FE), and random effects approaches to analyze the robustness of our results. The main advantage of the fixed effects model is that it allows controlling all time invariant omitted variables. Besides, we can use the fixed effects model to avoid omitted variable bias; however, to do so, we have to estimate a number of additional parameters. Concerning the random effects (RE), it assumes that the individual-specific effects are uncorrelated with the independent variables, and assists in controlling for unobserved heterogeneity when the heterogeneity is constant over time and not correlated with independent variables. It also allows making inference on the population data based on the assumption of normal distribution. However, one of the limits of the random effect model is that its coefficients are more likely to be biased.
Based on the Hausman test, we find that the random effect approach is appropriate for our analysis. The random effect results of Tables 11, 12, 13, and 14 show that the signs of the coefficients are the same as those of the FGLS, 2SLS, and Driscoll-Kraay estimators. Hence, our findings are consistent and robust across the different estimators.
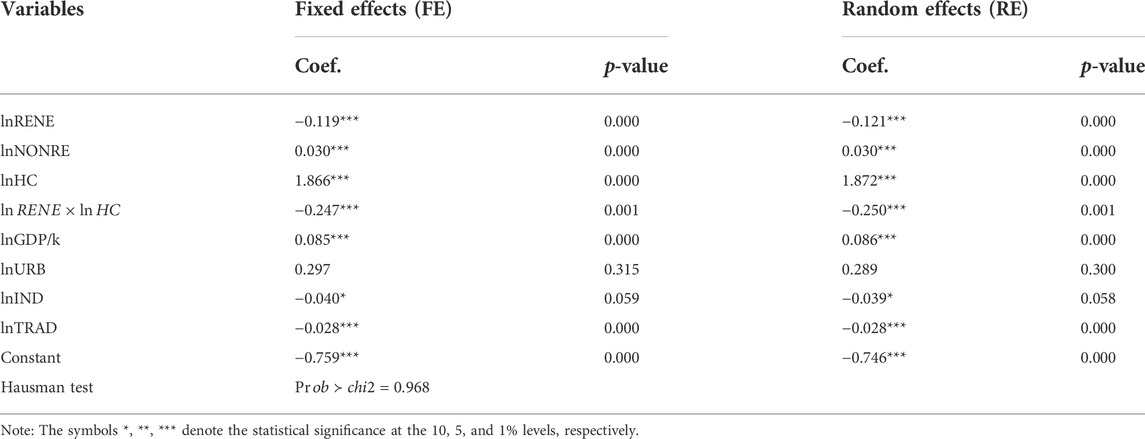
TABLE 12. Results of the moderating effect of human capital on the renewable energy-CO2 emissions nexus using the FE and RE estimators.
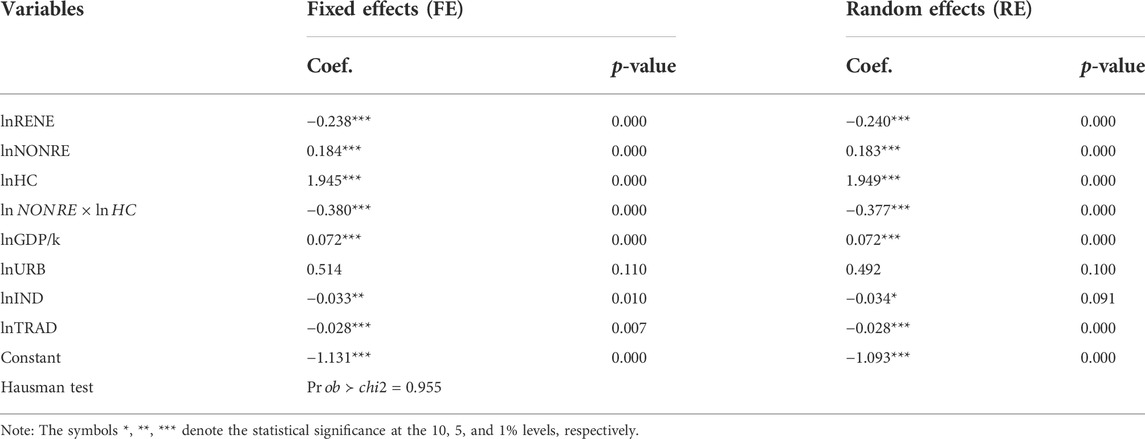
TABLE 13. Results of the moderating effect of human capital on the nonrenewable energy-CO2 emissions nexus using the FE and RE estimators.
4.2 Discussion of the findings
Concerning the marginal efficiency analysis, we find that the average level of human capital magnifies the negative effect of renewable energy consumption
For instance, at the average level of human capital, a 1% increase in lnRENE decreases lnCO2 by 0.271 and 0.355%, following the FGLS and 2SLS estimators, respectively (Table 8).
Under the conditional effect of human capital, renewable energy consumption has a greater marginal impact than its autonomous effect on environmental quality (i.e.,
These countries’ average level of human capital falls below its required threshold,
By considering the conditional effect of human capital in this analysis, our results extend those of most previous studies, which relied mainly on the linear nexus renewable energy consumption-nonrenewable energy consumption-CO2 emissions (Kassi et al., 2017; Berkun et al., 2019; Bilan et al., 2019; Mahalik et al., 2021; Kassi D. F. et al., 2022).
The negative impact of lnRENE on lnCO2 suggests an improvement in the quality of the environment when these countries use renewable energy in their production of goods and services, as this energy (solar, wind, nuclear energy) does not emit carbon dioxide and greenhouse gases that contribute to global warming.
Hence, these countries must offer incentives to households and the private sector to accelerate the adoption of renewable energy technologies. These incentives include providing low-interest loans or grants to renewable companies and communities using renewable electricity generation devices in their daily operations.
The positive link between nonrenewable energy and CO2 emissions is due to traditional energy resources. In effect, using traditional energy resources daily boost CO2 emissions through smoke expulsion from factories and households. Thus, these countries should invest in clean energy and set policies to encourage their population to use pro-environmental energy.
Furthermore, our results show a nonlinear relationship between human capital and CO2 emissions, meaning that human capital decreases CO2 emissions after only reaching a certain level.
The positive marginal effect of human capital on CO2 emissions
The curriculum in most emerging countries does not cover environmental topics. Thus, a low level of human capital initially increases CO2 levels in these countries. There is a need to educate people on climate change mitigation strategies, environmentally friendly practices, and efficient management of natural resources through using renewable energy in daily activities. Starting with the education system, policymakers should raise awareness about climate change and environmental protection.
In addition, the positive link between economic growth and CO2 emissions is in accordance with the findings of Dogan and Seker (2016), and Mahalik et al. (2021). This is because many countries have initially relied on the use of nonrenewable energies to drive their economic growth through fossil fuel-intensive industrialization policies financed by revenues from the trade of petroleum products.
Thus, an increased economic growth can increase CO2 emissions unless regulatory and green policies are applied to promote a green economy across countries. Accordingly, governments are urged to establish legal and regulatory frameworks that stimulate green financing of economic activities in industries and countries to ensure efficient management of energy sources and CO2 emission trading systems within limited thresholds that enable the transition to cleaner technologies and sustainable development (Kassi et al., 2021; Kassi D. F. et al., 2022).
Due to growing concern over climate change, countries have gradually considered environmentally friendly practices and technologies in their industrialization and trade openness to reduce CO2 emissions. Companies are attracted by a healthy economic environment and an optimal tax policy that favors green investments.
Countries initially exposed to greater trade openness might not have the technological capacity to compete in the international market. As a result, their unsophisticated, often fossil-based products are likely to pollute the environment. Over time, the transfer of green technologies and inflows of foreign capital will facilitate the implementation of green policies and the effective management of natural resources for sustainable development in all countries. Enforcing trade agreements on climate change can help countries better address sustainability issues (Alekseichuk et al., 2016; Hanslmayr et al., 2016).
Finally, our results highlight the importance of human capital to promote the beneficial effects of renewable energy consumption on the quality of the environment. Human capital also mitigates the negative impact of nonrenewable energy consumption on environmental quality. Therefore, higher levels of education can significantly help people better understand the importance of renewable energy in protecting the environment, which leads to the use of energy-efficient household appliances, solar energy, and other renewable sources in daily activities. Highly educated people also consider using green transportation (smart technology, energy-efficient hybrid vehicles) rather than fossil fuel-based means of transportation, which reduce CO2 emissions.
This finding also confirms the results of Lan et al. (2012), who consider human capital as a key driver for the adoption of environmentally friendly technologies that are mainly derived from renewable energy sources.
5 Conclusion
This study highlights the conditional effect of human capital on the relationships between renewable energy, nonrenewable energy, economic growth, and CO2 emissions in the 20 newly emerging market economies for the period 1990–2021. Using the FGLS and the 2SLS estimators, we found that renewable energy consumption negatively affects CO2 emissions. Also, industrialization and trade openness have negative effects on CO2 emissions. The results show that nonrenewable energy consumption, economic growth, and human capital positively affect CO2 emissions.
Besides, our findings confirm that renewable energy consumption and human capital are complementary levers for reducing CO2 emissions, whereas human capital mitigates the positive effect of nonrenewable energy consumption on CO2 emissions. Finally, this study reveals that human capital has an inverted U-shaped effect on CO2 emissions across countries.
As for the policy implications, policymakers should foster the use of renewable energy and continue to push companies that do so by offering incentives such as tax breaks or subsidies. They should also increase trade openness by creating a good economic environment for business. In addition, they should encourage these countries to invest and/or facilitate human capital accumulation to promote the use of renewable energy technologies over nonrenewable energy sources in producing goods and services. More emphasis should be placed on legal and regulatory policies that enhance the complementarity between human capital and renewable energy consumption for sustainable development in all countries. Thus, policymakers must implement educational reforms that help people understand environmental issues. These reforms could include environmental sustainability content in the curriculum, promoting the sustainable use of resources. In addition, social media should be used for effective environmental awareness campaigns, to teach the workforce about the importance of sustainable work and living habits, and to educate them on methods to reduce pollution and excessive consumption of natural resources. Raising citizens’ awareness of government regulatory pressures may be a possible solution to the problem of environmental degradation. People should be aware of the consequences of using poor-quality fossil products and encouraged to use energy-efficient gadgets and facilities such as solar-powered household items, LPG for cooking, and energy-saving light bulbs. When these green items are expensive, governments must provide them at subsidized rates. In addition, emission standards must be established for industries, and monitoring strategies must be developed to ensure that they comply. Governments should reduce energy consumption in urban cities through green means of transportation and eco-friendly urban transport network. The governments of these countries should also support national and international institutions (via financial and fiscal support) to innovate in renewable energies.
Moreover, cooperation between countries should be strengthened in renewable energy development, such as in the transfer of technology, equipment, and talents. These countries have diverse cultures, beliefs, and orientations. Therefore, it will be challenging to put in place a “global policy” parallel to the oddity of each country. Thus, policymakers in each of these countries should take into account their particularities in terms of cultural models, consumer responsibility, respect for nature, and environmental education of citizens when launching policies related to environmental sustainability. A sure way to improve environmental quality and energy efficiency in these countries is to strengthen policy coordination in the energy and economic sectors.
Finally, this study has some limitations that leave room for future studies. It would be interesting to examine the role of the private sector, the quality of governance, and foreign direct investment that may condition the effects of renewable energies on CO2 emissions across countries. Other studies may analyze the role of green finance and/or inclusive finance in this relationship. Also, instead of analyzing renewable energy holistically, future studies could consider a disaggregated approach highlighting the different types of renewable energy sources such as solar, wind, and nuclear, among others. Another strand of studies may also focus on the role of infrastructures that promote green energy vehicles.
Data availability statement
The datasets generated and/or analyzed in this study are from the World Development Indicators 2021 and the Penn World Table, version 10.0.
Author contributions
TYG and DFK made significant contributions in the conceptualization, methodology, analysis of results, discussion, and review. AJ-RE wrote the introduction and the literature review. OK made a significant contribution to the administration, and editing of the project. DY made a significant contribution to the conclusion and policy recommendations. All authors contributed to the article and approved the submitted version.
Funding
This study is supported by grant number 2021XJPT45, Suzhou University, Suzhou, Anhui Province, P.R. China.
Conflict of interest
The authors declare that the research was conducted in the absence of any commercial or financial relationships that could be construed as a potential conflict of interest.
Publisher’s note
All claims expressed in this article are solely those of the authors and do not necessarily represent those of their affiliated organizations, or those of the publisher, the editors and the reviewers. Any product that may be evaluated in this article, or claim that may be made by its manufacturer, is not guaranteed or endorsed by the publisher.
Footnotes
1Argentina, Brazil, Chile, China, Colombia, Egypt, Hungary, India, Indonesia, Iran, Malaysia, Mexico, the Philippines, Poland, Russia, Saudi Arabia, South Africa, Thailand, Turkey, and the United Arab Emirates.
2Argentina, Brazil, Chile, China, Colombia, Egypt, Hungary, India, Indonesia, Iran, Malaysia, Mexico, Philippines, Poland, Russian Federation, Saudi Arabia, South Africa, Thailand, Turkey, United Arab Emirates.
References
Adebayo, T. S., and Kirikkaleli, D. (2021). Impact of renewable energy consumption, globalization, and technological innovation on environmental degradation in Japan: Application of wavelet tools. Environ. Dev. Sustain. 2021, 16057–16082. doi:10.1007/s10668-021-01322-2
Alekseichuk, I., Turi, Z., Amador de Lara, G., Antal, A., and Paulus, W. (2016). Spatial working memory in humans depends on theta and high gamma synchronization in the prefrontal cortex. Curr. Biol. 26 (12), 1513–1521. doi:10.1016/j.cub.2016.04.035
Assamoi, G. R., Wang, S., Liu, Y., Gnangoin, Y. T. B., Kassi, D. F., and Edjoukou, A. J. R. (2020). Dynamics between participation in global value chains and carbon dioxide emissions: Empirical evidence for selected asian countries. Environ. Sci. Pollut. Res. 27, 16496–16506. doi:10.1007/s11356-020-08166-9
Awodumi, O. B., and Adewuyi, A. O. (2020). The role of nonrenewable energy consumption in economic growth and carbon emission: Evidence from oil producing economies in Africa. Energy Strategy Rev. 27, 100434. doi:10.1016/j.esr.2019.100434
Awosusi, A. A., Mata, M. N., Ahmed, Z., Coelho, M. F., Altuntaş, M., Martins, J. M., et al. (2022). How do renewable energy, economic growth and natural resources rent affect environmental sustainability in a globalized economy? Evidence from Colombia based on the gradual shift causality approach. Front. Energy Res. 9, 739721. doi:10.3389/fenrg.2021.739721
Bano, S., Zhao, Y., Ahmad, A., Wang, S., and Liu, Y. (2018). Identifying the impacts of human capital on carbon emissions in Pakistan. J. Clean. Prod. 183, 1082–1092. doi:10.1016/j.jclepro.2018.02.008
Bayar, Yilmaz, Smirnov, Vladimir, Danilina, Marina, and Kabanova, Natalia (2022). Impact of institutions and human capital on CO2 emissions in E.U. Transition economies. Sustainability 14, 353. doi:10.3390/su14010353
Berkun, F. V., Emir, F., and Sarkodie, S. A. (2019). Another look at the relationship between energy consumption, carbon dioxide emissions, and economic growth in South Africa. Sci. Total Environ. 655, 759–765. doi:10.1016/j.scitotenv.2018.11.271
Bilan, Y., Streimikiene, D., Vasylieva, T., Lyulyov, O., Pimonenko, T., and Pavlyk, A. (2019). Linking between renewable energy, CO2 emissions, and economic growth: Challenges for candidates and potential candidates for the E.U. Membership. Sustainability 11 (6), 1528. doi:10.3390/su11061528
Breusch, T. S., and Pagan, A. (1980). The Lagrange multiplier test and its applications to model specification in econometrics. Rev. Econ. Stud. 47 (1), 239–253. doi:10.2307/2297111
Butkiewicz, J. L., and Yanikkaya, H. (2011). Institutions and the impact of government spending on growth. J. Appl. Econ. 14, 319–341.
Cai, Y., Sam, C. Y., and Chang, T. (2018). Nexus between clean energy consumption, economic growth and CO2 emissions. J. Clean. Prod. 182, 1001–1011. doi:10.1016/j.jclepro.2018.02.035
Chen, Y., Wang, Z., and Zhong, Z. (2019). CO2 emissions, economic growth, renewable and non-renewable energy production and foreign trade in China. Renew. Energy 131, 208–216. doi:10.1016/j.renene.2018.07.047
Dasgupta, Susmita, Hettige, Hemamala, and Wheeler, David (2000). What improves environmental compliance? Evidence from Mexican industry.
Desha, C., Robinson, D., and Sproul, A. (2015). Working in partnership to develop engineering capability in energy efficiency. J. Clean. Prod. 106, 283–291. doi:10.1016/j.jclepro.2014.03.099
Dietz, T., and Rosa, E. A. (1997). Effects of population and affluence on CO2 emissions. Proc. Natl. Acad. Sci. U. S. A. 94 (1), 175–179. doi:10.1073/pnas.94.1.175
Dingru, L., Ramzan, M., Irfan, M., Gülmez, Ö., Isik, H., Adebayo, T. S., et al. (2021). The role of renewable energy consumption towards carbon neutrality in BRICS nations: Does globalization matter? Front. Environ. Sci. 9. doi:10.3389/fenvs.2021.796083
Dogan, E., and Seker, F. (2016). Determinants of CO2 emissions in the European Union: The role of renewable and nonrenewable energy. Renew. Energy 94 (C), 429–439. doi:10.1016/j.renene.2016.03.078
Dong, K., Sun, R., Jiang, H., and Zeng, X. (2018). CO2 emissions, economic growth, and the environmental Kuznets curve in China: What roles can nuclear energy and renewable energy play? J. Clean. Prod. 196, 51–63. doi:10.1016/j.jclepro.2018.05.271
El-Bannany, M. (2017). Factors influencing accounting conservatism in banks: The UAE case. J. Gov. Regul. 6 (2), 14–21.
Fatima, Tehreem, Shahzad, Umer, and Cui, Lianbiao (2021). Renewable and nonrenewable energy consumption, trade and CO2 emissions in high emitter countries: Does the income level matter? J. Environ. Plan. Manag. 64 (7), 1227–1251. doi:10.1080/09640568.2020.1816532
Feenstra, R. C., Inklaar, R., and Timmer, M. P. (2015). The next generation of the Penn world table. Am. Econ. Rev. 105 (10), 3150–3182. doi:10.1257/aer.20130954
Feng, H. (2022). The impact of renewable energy on carbon neutrality for the sustainable environment: Role of green finance and technology innovations. Front. Environ. Sci. 10. doi:10.3389/fenvs.2022.924857
Hanslmayr, S., Staresina, B. P., and Bowman, H. (2016). Oscillations and episodic memory: Addressing the synchronization/desynchronization conundrum. Trends Neurosci. 39 (1), 16–25. doi:10.1016/j.tins.2015.11.004
Hartman, R., and Kwon, O-S. (2005). Sustainable growth and the environmental Kuznets curve. J. Econ. Dyn. Control 29 (10), 1701–1736. doi:10.1016/j.jedc.2004.10.001
Ibrahim, R. L., and Ajide, K. B. (2021). The dynamic heterogeneous impacts of nonrenewable energy, trade openness, total natural resource rents, financial development and regulatory quality on environmental quality: Evidence from BRICS economies. Resour. Policy 74, 102251. doi:10.1016/j.resourpol.2021.102251
Inglesi-Lotz, R., and Dogan, E. (2018). The role of renewable versus non-renewable energy to the level of CO2 emissions a panel analysis of Sub- saharan africa's ßig 10 electricity generators. Renew. Energy 123, 36–43. doi:10.1016/j.renene.2018.02.041
International Energy Agency (2017). Cities are at the frontline of the energy transition. Available at https://www.iea.org/news/cities-are-at-the-frontline-of-the-energy-transition.
International Energy Agency (2021). Global Co2 emissions rebounded to their highest level in history. Available at https://www.iea.org/news/global-co2-emissions-rebounded-to-their-highest-level-in-history-in-2021.
Ito, K. (2017). CO2 emissions, renewable and non-renewable energy consumption, and economic growth: Evidence from panel data for developing countries. Int. Econ. 151, 1–6. doi:10.1016/j.inteco.2017.02.001
Kassi, D. F., Li, Y., and Dong, Z. (2021). The mitigating effect of governance quality on the finance-renewable energy‐growth nexus: Some international evidence. Int. J. Fin. Econ. doi:10.1002/ijfe.2423
Kassi, D. F., Francois, D., Li, Y., Gnahe, F. E., Shaikh, R., and Tuo, S. J. (2022b). Green credits, green securities, and environmental quality: A comparative analysis across Chinese provinces. SSRN Electron. J.. doi:10.2139/ssrn.4063864
Kassi, D. F., Li, Y., Riaz, A., Wang, X., and Batala, L. K. (2022a). Conditional effect of governance quality on the finance-environment nexus in a multivariate EKC framework: Evidence from the method of moments-quantile regression with fixed-effects models. Environ. Sci. Pollut. Res. 29 (35), 52915–52939. doi:10.1007/s11356-022-18674-5
Kassi, D. F., Nasiri, A., and Edjoukou, A. J. R. (2017). Financial development, economic growth and energy consumption Nexus in Cote d’Ivoire. Int. J. Finance Bank. Stud. 6 (2147-44863), 1–21. doi:10.20525/ijfbs.v6i3.746
Kassi, D. F., Sun, G., and Ding, N. (2020). Does governance quality moderate the finance-renewable energy-growth nexus? Evidence from five major regions in the world. Environ. Sci. Pollut. Res. 27 (11), 12152–12180. doi:10.1007/s11356-020-07716-5
Lan, J., Kakinaka, M., and Huang, X. (2012). Foreign direct investment, human capital and environmental pollution in China. Environ. Resour. Econ. (Dordr). 51, 255–275. doi:10.1007/s10640-011-9498-2
Lau, L. S., Choong, C. K., Ng, C. F., Liew, F. M., and Ching, S. L. (2019). Is nuclear energy clean? Revisit of environmental Kuznets curve hypothesis in OECD countries. Econ. Model. 77, 12–20. doi:10.1016/j.econmod.2018.09.015
Le, T.-H., Chang, Y., and Park, D. (2020). Renewable and nonrenewable energy consumption, economic growth, and emissions: International evidence. Energy J. 41 (2). doi:10.5547/01956574.41.2.thle
Li, X., and Ullah, S. (2022). Caring for the environment: How CO2 emissions respond to human capital in BRICS economies? Environ. Sci. Pollut. Res. 29 (12), 18036–18046. doi:10.1007/s11356-021-17025-0
Mahalik, M. K., Mallick, H., and Padhan, H. (2021). Do educational levels influence the environmental quality? The role of renewable and nonrenewable energy demand in selected BRICS countries with a new policy perspective. Renew. Energy 164 (C), 419–432. doi:10.1016/j.renene.2020.09.090
Mendonça, A. K. d. S., de Andrade Conradi Barni, G., Moro, M. F., Bornia, A. C., Kupek, E., and Fernandes, L. (2020). Hierarchical modeling of the 50 largest economies to verify the impact of GDP, population and renewable energy generation in CO2 emissions. Sustain. Prod. Consum. 22, 58–67. doi:10.1016/j.spc.2020.02.001
Mohsin, M., Kamran, H. W., Atif Nawaz, M., Sajjad Hussain, M., and Dahri, A. S. (2021). Assessing the impact of transition from nonrenewable to renewable energy consumption on economic growth-environmental nexus from developing asian economies. J. Environ. Manage. 284, 111999. doi:10.1016/j.jenvman.2021.111999
Muhammad, B., Khan, M. K., Khan, M. I., and Khan, S. (2021). Impact of foreign direct investment, natural resources, renewable energy consumption, and economic growth on environmental degradation: Evidence from BRICS, developing, developed and global countries. Environ. Sci. Pollut. Res. 28 (17), 21789–21798. doi:10.1007/s11356-020-12084-1
Oke, D. M., Ibrahim, R. L., and Bokana, K. G. (2021). Can renewable energy deliver African quests for sustainable development? J. Dev. Areas 55 (1). doi:10.1353/jda.2021.0022
Pablo-Romero, M. D., and Jesus, J. D. (2015). Economic growth, and energy consumption: The energy-environmental Kuznets curve for Latin America and the caribbean. Renew. Sustain. Energy Rev. 60, 1343–1350. doi:10.1016/j.rser.2016.03.029
Parks, R. (1967). Efficient estimation of a system of regression equations when disturbances are both serially and contemporaneously correlated. J. Am. Stat. Assoc. 62, 500–509. doi:10.1080/01621459.1967.10482923
Pata, U. K. (2018). Renewable energy consumption, urbanization, financial development, income and CO2 emissions in Turkey: Testing EKC hypothesis with structural breaks. J. Clean. Prod. 187, 770–779. doi:10.1016/j.jclepro.2018.03.236
Pesaran, M. H. (2007). A simple panel unit root test in the presence of cross-section dependence. J. Appl. Econ. 22 (2), 265–312.
Pesaran, M. H. (2004). General diagnostic tests for cross section dependence in panels. CESifo Working Paper Series No. 1229. Bonn, Germany: IZA Discussion Paper Series, 1240.
Qi, T., Zhang, X., and Karplus, V. J. (2014). The energy and CO2 emissions impact of renewable energy development in China. Energy Policy 68, 60–69. doi:10.1016/j.enpol.2013.12.035
Raza, Syed Ali, and Shah, Nida (2018). Testing environmental Kuznets curve hypothesis in G7 countries: The role of renewable energy consumption and trade. Environ. Sci. Pollut. Res. 25, 26965–26977. doi:10.1007/s11356-018-2673-z
Sarkodie, S. A., and Ozturk, Ilhan (2020). Investigating the environmental Kuznets curve hypothesis in Kenya: A multivariate analysis. Renew. Sustain. Energy Rev. 117 (C), 109481. doi:10.1016/j.rser.2019.109481
Shafiei, S., and Salim, R. (2014). Nonrenewable and renewable energy consumption and CO2 emissions in OECD countries: A comparative analysis. Energy Policy 66 (C), 547–556. doi:10.1016/j.enpol.2013.10.064
Shahbaz, M., Loganathan, N., Sbia, R., and Afza, T. (2015). The effect of urbanization, affluence and trade openness on energy consumption: A time series analysis in Malaysia. Renew. Sustain. Energy Rev. 47, 683–693. doi:10.1016/j.rser.2015.03.044
Shahnazi, R., and Shabani, Z. D. (2021), The effects of renewable energy, spatial spillover of CO2 emissions and economic freedom on CO2 emissions in the E.U, Renew. Energy, 169 293–307. doi:10.1016/j.renene.2021.01.016
Szetela, B., Majewska, A., Jamroz, P., Djalilov, B., and Salahodjaev, R. (2022). Renewable energy and CO2 emissions in top natural resource rents depending countries: The role of governance. Front. Energy Res. 10, 872941. doi:10.3389/fenrg.2022.872941
Timmons, D., Harris, J.M., and Roach, B. (2014). The Economics of Renewable Energy. Global Development and Environment Institute, Tufts University, 52 Boston, USA.
Wang, J., and Xu, Y. (2021). Internet usage, human capital and CO2 emissions: A global perspective. Sustainability 13 (15), 8268. doi:10.3390/su13158268
Wang, S., Yang, C., and Li, Z. (2021). Spatio-temporal evolution characteristics and spatial interaction spillover effects of new-urbanization and green land utilization efficiency. Land 10, 1105. doi:10.3390/land10101105
World Development Report, (2019). The changing nature of work. Available at https://www.worldbank.org/en/publication/wdr2019.
Yao, Y., Ivanovski, K., Inekwe, J., and Smyth, R. (2020). Human capital and CO2 emissions in the long run. Energy Econ. 91, 104907. doi:10.1016/j.eneco.2020.104907
Keywords: renewable energy, non-renewable energy, human capital, CO2 emissions, emerging market economies
Citation: Gnangoin TY, Kassi DF, Edjoukou AJ-R, Kongrong O and Yuqing D (2022) Renewable energy, non-renewable energy, economic growth and CO2 emissions in the newly emerging market economies: The moderating role of human capital. Front. Environ. Sci. 10:1017721. doi: 10.3389/fenvs.2022.1017721
Received: 12 August 2022; Accepted: 22 August 2022;
Published: 26 September 2022.
Edited by:
Mihaela Simionescu, Romanian Academy, RomaniaReviewed by:
Umer Shahzad, Anhui University of Finance and Economics, ChinaMuhammad Zahid Rafique, Center for Economic Research, Shandong University, China
Copyright © 2022 Gnangoin, Kassi, Edjoukou, Kongrong and Yuqing. This is an open-access article distributed under the terms of the Creative Commons Attribution License (CC BY). The use, distribution or reproduction in other forums is permitted, provided the original author(s) and the copyright owner(s) are credited and that the original publication in this journal is cited, in accordance with accepted academic practice. No use, distribution or reproduction is permitted which does not comply with these terms.
*Correspondence: Thierry Yobouet Gnangoin, Z3RoaWVycnkuZ2JAZ21haWwuY29t, dGhpZXJyeWJAYWhzenUuZWR1LmNu