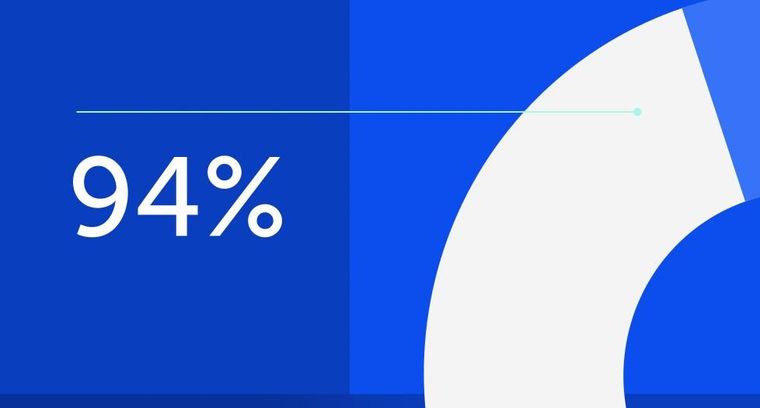
94% of researchers rate our articles as excellent or good
Learn more about the work of our research integrity team to safeguard the quality of each article we publish.
Find out more
ORIGINAL RESEARCH article
Front. Environ. Sci., 25 November 2022
Sec. Environmental Economics and Management
Volume 10 - 2022 | https://doi.org/10.3389/fenvs.2022.1017297
In a developing nation such as Pakistan, the textile sector is crucial for employment creation and poverty reduction. As a result of digitization, standard industrial operating processes have altered. The study, which is built on resource-based theory, seeks to determine how the digital supply chain (comprised of blockchain, the internet of things, and big data analytics) influences operational performance. In addition, the relationship between the innovative climate and the operational success of the digital supply chain has been analyzed. Data was collected from 270 respondents, i.e., 65 top level managers and 205 middle level managers. The results indicate that the digitization of the supply chain, which includes the application of big data analytics, the internet of things, and blockchain, greatly enhances operational performance. The innovation climate has not been proven to affect operational performance or digitalization. At the end theoretical and practical implication are highlighted.
Indeed, the current industrial markets have expanded their operations around the world and the current business segment has generated intense competition in the market. To maintain a competitive edge in these international markets, organizations need to identify emerging digital technologies that can be used to build a new business model. Currently, manufacturing activities are conducted in such a way that the world has directed companies to integrate modern manufacturing technologies such as 3D printing, rapid prototyping and the Internet of Things for data collection and evaluation. In the current economic environment, the main objective of companies is to provide the best quality and quantity of products and services to their customers at reasonable costs (Agrawal and Narain, 2018). Digitization will be hugely beneficial to supply chain organizations and operations. Many companies want to become more "digital" because they see the value and importance of digital technology in growth and operations and management support for such projects is increasing (Bughin J. et al., 2015). Supply Chain Management These challenges are efficiently addressed by SCM, which includes operations management, logistics, procurement, information technology and manufacturing. Accordingly, current developments in these areas should be provided, as well as how technology development can be used to create competitive advantage in these actions. Companies must now adapt their trade flows to changing customer needs while maintaining a highly standardized and professional supply chain (Arsyad et al., 2022). In the coming years, researchers predict a shift to the digital world and digital supply chain management. Traditional supply chains will be replaced by demand-responsive networks (Agrawal and Narain, 2018). To be sure, previous studies have discussed operational performance from different perspectives. However, these models lack the role of the digital supply chain. Indeed, the digital supply chain invented the technology, but its operational performance has not been evaluated in any studies.
The companies are transforming digitally and adopting new ways to do it (Hoberg et al., 2015). It involves doing the things differently and with an innovative way by adopting core activities of value chain. The new supply chain will adopt doing things with latest technology. The transition to a digital world is a type of change and any change must be treated with caution (Wade and Marchant, 2014). One person's effort is not enough to bring about change in digital transformation and the concerted efforts of all who work to bring about change successfully are needed (Ijaz et al., 2022).
The supply chain is the most important component of any business organization. Companies that want to thrive in today's corporate culture are constantly incorporating digitization and technological advances into their logistics efforts, while those that are not moving away from the field (Amjad et al., 2020). The business sector is undergoing rapid and dramatic development, forcing changes unlike any before (Baig et al., 2022). The Internet of Things (IoT) brings economic benefits to the real world (Borgia, 2014; Madakam and Lake, 2015; Russo et al., 2015). With its traceability, adaptability, transparency, flexibility, and scalability, his IOT adoption helps reduce costs and risks, thereby improving the overall operational process (Zhou L. et al., 2015). It has many hindrances as well (Whitmore et al., 2015). It needs to be explored more. IOT and supply chain has a great connection (Mishra et al., 2016). IoT implementation methods, facilitators, difficulties, and adoption by companies should be considered. Previously, there were no significant studies evaluating the relationship between digital supply chain technology and Pakistan’s textile sector. It has been equally applied in the service sector and logistics management. The study focused on the connection between digital SC and companies performance. The previous discussion concludes that there is still a need for empirical research on blockchain technology, big data analytics, and the relationship between the Internet of Things and the modest impact of operational performance and innovation environments. This paper fills the gap by discussing these variables. The study is conducted in relation to the textile industry in Pakistan and is based on variables such as blockchain technology, Internet of Things, big data analytics, operational performance, and innovation environment. The study adds transformative climate mitigation effects to the literature, demonstrating the need for businesses to adopt digital technologies and create dynamic cultures to improve corporate and operational performance. According to the study, effective use of digital technology requires a supply chain manager to contact her IT company to better understand the use of technology in the supply chain. Digital technology is a descriptive technology. We constantly rely on other technologies to improve the functionality of our applications. The extent of research is determined empirically. Discuss the use of digital technologies in the supply chain, such as blockchain technology, big data analytics, and IoT, and their impact on supply chain operational performance. We all know that digital technology is a wide range of technologies, but this study explored the impact of three major technologies on operational performance: blockchain technology, big data analytics, and the IOT. Importantly, this theoretical contribution to the study has improved operational performance models for the textile industry that will also benefit future researchers.
Digitization is now advancing as a new process to reinvent many aspects of life globally as reflected in a highly competitive business market and dynamic and complex environment. More than 90% of Internet users shop online and more than 40% of organizations use advanced technologies for big data analysis. SC will get benefitted with the use of IT. Create a competitive advantage that drives long-term enterprise value by digitizing legacy supply chains. Cap Gemini (2016) predicts that over the next five years, many industrial companies will propose ways to digitize their supply chains. Increasing supply chain agility, information systems integration and innovative technologies are examples of developments that improve customer service and industry performance in the long term. In fact, DSC incorporates advanced technologies (big data, augmented reality, blockchain, etc.) to reduce processes within and between organizations, have a greater impact on customers and consumers, and increase business value. To better serve their customers, organizations should consider establishing, improving, and developing DSC and related new management practices.
Blockchain technology is comprised of a peer-to-peer transmission protocol, a shared distributed database, and the irreversibility of a digital record, all of which are powered by a computational algorithm that ensures users’ transparency and pseudo-anonymity (Lakhani and Iansiti, 2017). Proponents of BCT expect a significant shift in how consumers interact with the internet. In the current environment, network applicants are involved in the “Internet of Information,” where they transfer data with the possibility of duplication (Treiblmaier, 2018). They will move to the “Internet of Value” through the creation of a blockchain that will allow for the transparent exchange of digital property rights (Tapscott, Tapscott, 2016). The organization will get befit in all their aspect with the use of blockchain (Tapscott and Tapscott, 2017).
Big data is defined as huge or complicated data collections with sizes ranging from Exabytes to Petabytes. It outperforms the technical capabilities of outmoded storage, processing, management, interpretation, and display system (Kaisler et al., 2013). Many organizations have endeavored to expand and strengthen their big data analytic capabilities to identify and get a thorough understanding of their big data hidden standards. Academics and professionals alike agree that the overflow data presents new opportunities. Big-data research is constantly growing and deepening; the “5V” concept incorporates volume, velocity, variety, verification/veracity, and value as the primary characteristics of big data (White, 2012; Addo-Tenkorang and Helo, 2016). Kambatla et al. (2014) presents the most recent hardware and software developments in big data analytics.
IoT is evident from decades. Kevin Ashton coined the term in the context of SC (Gubbi et al., 2013). It simply refers to a collection of physical devices that transmit data over web to do essential business functions (Whitmore et al., 2015; Wortmann and Flüchter, 2015; Botta et al., 2016).
Operational efficiency is defined as the actual performance of an operational strategy that represents or reflects the unique characteristics of a production system, influenced by operating conditions (Bartezzaghi and Turco, 1989). Lu et al. (2018) said the OP is a key indicator of SC performance. This is often the result of various system elements and enablers. It is need of the time to see it closely (Franco-Santos et al., 2007). Figure 2 shows the items loadings of the inner and outer constructs in the model. Ebrahimi (2015) suggests that a firm's supply chain performance should be measured using both financial and non-financial indicators such as net sales, cost of goods sold, profitability and return on investment. Operational efficiency was chosen as one of the criteria for several reasons. The performance of the organization should be seen from SC view (Behrouzi et al., 2011; Devaraj et al., 2007).
Organizational characteristics influence creativity and innovation (Amabile et al., 1996; Mumford et al., 2002). Environmental factors influence human creativity, motivation, and motivation (Redmond et al., 1993). It has many factors to c (Jung et al., 2003). Scott and Bruce (1994) defined climate as „the individuals cognitive representation of the organizational environment”. According to Mumford et al. (2002), the invention needs great culture. Jung et al. (2003) found different environments has different effects. Some cultures are more creative than other. Figure 3 shows the output model with the interaction of innovation climate.
The majority of published research has focused on traditional SCM basics and their impact on operational performance (Fynes et al., 2005; Dehning et al., 2007; Boo-itt, 2011; Wong et al., 2011; Abdallah et al., 2014). These early studies laid the groundwork for subsequent research, which has focused on a variety of innovative technologies used to characterize a digital supply chain, such as IOT, big data, blockchain cloud computing, and robotics in SCM (Akter et al., 2016; Weichhart et al., 2016; Büyüközkan Feyzioglu and Göçer, 2018; Matt et al., 2015). It is a unique investigation into the supply chains digital aspects. In terms of technological advancement, the three technologies chosen are significant to the textile industry. This is focused on societal requirements and improves the deployment of firm assets and environmental performance (Hart, 1995). The resource-based perspective of Sustainable SCM wins the advantages for the firms by developing distinctive sustainability-related talents, with the support of power, and with a traditional notion of corporate performance. Figure 1 depicts the conceptual framework.
The high speed of innovation creates opportunities for new businesses, while simultaneously increasing global competition and shortening the company's life cycle (Berg et al., 2019). Pieiro-Chousa, et al., (2020) found the determinant to bring change. Real-time information systems are a good way to go (Tian, 2017; Treiblmaier, 2018; Banerjee, 2019; Madhwal and Panfilov, 2017). Blockchain technology increases productivity, and efficiency in the business. It makes things easy, because the supply chain has the most organized competitors, and the infrastructure has product quality levels delivered at standard prices managed by the supply chain.
H1. Blockchain technology has a positive and significant impact on operational performance.
Big data is usually the most popular trend and one of the most frequently asked questions in systematic and practical problems. In general, „big data” refers to a large amount of data (Mayer-Schönberger and Cukier, 2013). Bulk data characteristics, on the other hand, are not defined by their size. Big data refers to a large collection of data that exceeds the capacity of traditional database software to track, record, manage, store, and analyze (Arunachalam et al., 2018). Big data analysts can effectively extract useful facts from large amounts of data (Mikalef et al., 2018). Instead of expanding resources, the company's goal is to acquire diverse talent to manage reputational risk and gain a competitive advantage over uncertainty. Risk management is especially important because the environmental impacts of an organization's activities can cause reputational and financial problems if they do not meet sustainability standards (Wood et al., 2018). SCM has brought many good aspects to the companies to improve their overall efficiency (Gong et al., 2018; Wang G. et al., 2016). Applying BDA to a company's supply chain sustainability approach can successfully address overall business or financial performance (Lin et al., 2018).
H2. Big data analytics has a positive and significant impact on operational performance.
According to Cortés et al. (2015), IoT is providing services electronically to achieve meaningful goals. IoT is a panacea for all diseases in the supply chain. It has many benefits, for example, monitoring and tracking products, developing intelligent delivery systems, and predicting demand. Inventory is one of the most critical places where the supply chain can save money. IoT can reduce inventory costs as well as impacts across the supply chain. This is an advantage that allows companies to actively contribute to relevant business prospects across multiple business dimensions, including distribution, procurement, and production, shorten separation between entities, reduce costs through operations, optimize inventory levels, and dynamic operational strategies (Li et al., 2015).
H3. The Internet of things has a positive and significant impact on operational performance.
Organizational environment is a strong predictor of inventive behavior (Scott and Bruce, 1994). Research shows that corporate culture is a valuable, intangible, and unique resource (Russo and Fouts, 1997). At the staff level, we focus on the perceived environment for innovation and perceived operational performance. This strategy is consistent with evaluation studies that assume that the value lies solely in the imagination of the observer (Muniesa, 2011). Such an innovation environment can be a useful organizational capability to drive open innovation offerings across the business, resulting in higher performance levels for operating companies. Organizations with thriving innovation environments provide employees with ample time to track and adopt creative ideas (Park and Jo, 2018).
H4. Innovation climate has a positive and significant impact on operational performance.
Blockchain technology has gained popularity in recent years, and it has been labeled the "trigger" for the impending information uprising. It was invented for Bitcoin and is now widely utilized in banks, education, healthcare, and other areas (Kollmann et al., 2020). Because of its unique technological qualities, blockchain technology is being used by an increasing number of businesses to provide technical support for business processes. Similarly, it serves as the foundation for truly trustless economic transactions and provides organizations with a viable strategy for managing data related to innovations. Blockchain technology, which is an emerging digital technology, provides businesses with a new way of producing and receiving value while also changing exchange mechanisms and interchange structures and forming an inventive organization. These are advantageous to improving invention quality for the following reasons. To begin, firms can use lifecycle, and blockchain technology can efficiently increase pursuing and recycling operations. Businesses that use blockchain technology can acquire a technological advantage over competitors, increasing their competitiveness and market survival (Haleem et al., 2018). Second, businesses can use blockchain technology to restructure their research and development resources. Finally, it supports cost-cutting by lowering the risks of innovation, allowing business shareholders to watch any relevant changes in the accounts in real time, and improving the organizations' data (Risius and Spohrer, 2017).
H5. Innovation climate moderates the relationship between Blockchain and operational performance.
Frankwick (1996) found beyond technological innovation has taken many forms, including processing innovations (Pisano, 1997), service innovations (Gallouj and Weinstein, 1997), and strategical innovations (Gallouj and Weinstein, 1997; Hamel, 1998). In general, innovation can be described as any ideas, processes, or material outputs perceived to be creative by the firm (Zaltman et al., 1973). The properties and functionality of big data are usually associated with creativity. According to (Gobble, 2013), the path from collecting big data to determining its worth necessitates innovation. Adopting big-data technology requires significant investment (Terziovski, 2010), and organizations that use it as the foundation of their business, such as Amazon or Google, take years to achieve profitability. Many of the firms recognized for business-to-consumer (B2C) innovation have transformed B2-B marketing.
H6. Innovation climate moderates the relationship between Big data analytics and operational performance.
In recent years, there has been a lot of buzz about the Internet of Things (IoT). The IoT is a collection of disruptive digital technologies that touch people’s and businesses’ daily lives (Kim and Kim, 2016; Scuotto et al., 2016). Firms are increasingly adept at developing, implementing, and adapting disruptive technologies in the operations of these organizations to enhance them efficiently and innovatively through information flows and data or information collection, per the paradigm (Malhotra, 2000; Vrontis et al., 2012). The extensive research and daily focus on the company’s expertise have concentrated on managing knowledge to strengthen the organization’s advantages. More research is needed in this regard (Del Giudice and Della Peruta, 2016). Because many businesses need to manage data in larger contexts, and they are continuously striving for that.
H7. Innovation climate moderates the relationship between the Internet of things and operational performance.
Research methods are founded on facts and numbers that can be gathered. The research methodology is clearly defined as the strategy utilized to efficiently answer a problem. It contains the research work plan (Rajasekar, 2013). Designing the research methodology for the specified problem is extremely crucial.
The term population refers to the whole of all persons, factors, or occurrences from which a researcher strives to draw an appropriate conclusion (Bull, 2005). The research was carried out in the context of the country’s textile companies operating in Punjab. The population of the study is all of the country’s textile mills operating in the Punjab and are listed in all Pakistan mills association APTMA. These textile businesses are classified into several groups (weaving, spinning, composite, garments, etc.). The companies’ information was obtained from the textile members’ directory. Moreover, the convenient sampling approach was used. The unit of analysis is stated as how we can initially examine the data. It demonstrates through the study who and what the study is intended to discover. We conducted a survey for the middle and top management of the textile sector as they are the most suitable people to provide us with the data. Table 2 shows the descriptive stats of the constructs.
The data was obtained from senior and middle-level textile industry executives. The questionnaire was self-administered and used a 5-point Likert scale.
According to Table 1, a total of 400 questionnaires were sent, with 303 responses received. 270 of the completed surveys were valid for further data analysis. The wrong and improper responses were 33. However, only 270 of the 303 questionnaires were used, resulting in a legitimate response rate of 67.5%.
SPSS software was used for data screening, including missing value analysis and outlier detection. Missing data is responsible for decreasing their frequency, so researchers must reduce the missing value to avoid it. As a result, the researcher demanded that preventative measures be implemented at the collection location. The researchers double-checked the completed questionnaires to ensure that the items were appropriately answered. A mean of more than 5% missing value per item is recommended (Hair et al., 2013). The study’s lack of value analysis found that no object had a missing value of more than 5%, ranging from 0.2% to 1.5%. To replace the missing value, SPSS v20 is utilized. The outlier is that other results do not support it, even though other observations are uncommon. Outliers can suggest experimental error during the variance calculating method (Churchill and Iacobucci, 2006). It can occur in any random distribution, but it always implies a population with a hardtail distribution or measurement errors. Missing the initial outlier analysis will prejudice the statistical test if it reveals outlier issues, thus an outlier inquiry is critical. To discover multivariate outliers using the linear regression approach in SPSS v20, Mahalanobis D2 is employed (Tabachnick et al., 2007). The Mahalanobis D2 values ranged from 0.081 to 18.477 but did not exceed 18.48. The study uses descriptive statistical analysis to determine the major properties of data. The overall description of the structures is used in the study. Structured equation modeling (SEM) techniques were used to analyze the screened data using SMART Pls software. SEM techniques were used to create measurement models as well as structural models. Measurement models thoroughly analyze the reliability and validity of the instruments used for data collection, as well as additional methods to ensure the data’s applicability. The structural model evaluates the links between latent components for association and causality.
The Smart PLS results are shown. The instrument’s validity and reliability were evaluated. Validity and reliability tests can be performed to ensure that constructs are correct. The inner and outer models were tested after data screening (Vinzi et al., 2010). The smart PLS is used to evaluate the study’s relationships. In the current investigation, all variables were measured reflectively. Indicator and hidden variables were mostly reflecting rather than formative. The first-order construct, not the second-order construct, underpins the study analysis. Five latent variables were composed, as represented by the relationship between variables and sequence. The current study used one multidimensional independent variable (Blockchain, Internet of Things, and big data analysts), one moderator, “Innovation Climate,” and one dependent variable (operational performance).
The PLS-SEM first stage in the current investigation is the Outer model. The measurement components with which the outer model is concerned, in theory, influence how appropriately load and associate the items with constructions. The outer model inspection reveals the structure’s research issues that are thought to be measured. The two basic metrics, validity, and reliability, are utilized to evaluate the external model in PLS-SEM (Hulland, 1999; Ramayah et al., 2011). The constructs have a comparable indicator loading that the CR does not assume (Hair et al., 2013). The threshold value must be more than or equal to 0.60, and the composite dependability scale must be between 0 and 1 (Hensler et al., 2009). The more acceptable cutoff value is 0.70 or higher (Hair et al., 2012). The average internal consistency is shown by a composite reliability value of 0.6–0.7. The most acceptable range is 0.7–0.9.
A set of characteristics linked to the demographics of the participants was chosen. The demographic profile of participants comprised age, gender, and degree of income.
Table 3 displays the gender distribution of responders. Males made up 94.5% of responders, while females made up 5.5%. 21.5% of respondents were between the ages of 20 and 30, 8.4% were between the ages of 31 and 40, 33.8% were between the ages of 41 and 50, and 36.3% were between the ages of 51 and 60. Three responders have departed the 51–60 age range. Furthermore, 76.9% of respondents were in middle-level management, while 23.1% were in senior-level management in the textile business. Furthermore, 9.1% of respondents have experience of 1 year or less, 7.8% have experience of more than 1 year up to 3 years, 6.5% have experience of more than 3 years up to 5 years, 23.3% have experience of more than 5 years up to 10 years, and 53.4% have experience of more than 10 years.
Table 4 displays the Cronbach’s alpha, CR, and AVE values. Cronbach alpha levels are more than the threshold value of 0.7. It demonstrates that the constructs utilized in the study were trustworthy. The measurements were appropriate for collecting data for the constructs. The permissible range is also reflected in the AVE and CR readings.
Construct uniqueness is based on discriminant validity, which determines whether the phenomena are taken and not symbolized by another construct in the model. The Heterotrait-Monotrait and Fornell-Larcker criteria could be used to test discriminant validity by examining construct cross-loading. The correlation ratio measures how well two variables were linked to each other (HTMT). A cross-loading analysis is more advanced for proving more significant numbers of the discriminant validity of the construct. The current study looked at outer factor loading as a critical criterion.
A systematic model analysis of the structural model was used in the study to provide a comprehensive perspective of results and to assess the hypothesis from 1 to 7. The PLS-SEM algorithm is also used to investigate the route coefficient size. A moderating model dealing with H4–H6 was used to investigate variable connections.
Table 6 shows the outcome of the direct relationship hypothesis. As a result, hypothesis 1 yields t = 8.097 and p = 0.000, indicating that there is a direct association between blockchain and operational performance as well as a strong positive relationship between blockchain and operational performance. As a result, hypothesis 1 is supported. Hypothesis 2 is further supported by the results of t = 4.005 and p = 0.000, which reveal a direct beneficial association between big data analytics and operational performance. Hypothesis 3 yields t = 2.345 and p = 0.019, indicating a direct association between the internet of things and operational performance. Therefore, hypothesis 3 is likewise supported by the aforementioned two hypotheses. Finally, the results of hypothesis 4 are t = 0.072, and p = 0.942, indicating that there is no direct association between the innovation climate and operational performance, indicating that hypothesis 4 is rejected or not supported.
Table 5 shows the discriminant validity of the constructs. Table 6 does not support the direct association of the moderator with the dependent variable, implying that there is no relationship between innovation climate and operational performance, and hypothesis 4 is not accepted and supported. Table 7 shows that the moderator does not affect independent or dependent variables, implying that the innovation climate does not affect the relationship between blockchain technology and operational success. The results are t = 0.241 p = 0.809, indicating that hypothesis 5 is unsupported. The t value is 1.252 and the p-value is 0.211, indicating that there is no moderating effect on big data analytics and operational performance. Again, hypothesis 7 is not supported because the data t = 1.297, p = 0.195 reveal that there is no moderating influence of the innovation climate on the internet of things and operational performance; hence, hypothesis 6 is unacceptable.
The special study examined the national sample. Studies show that the digital chain has an incredibly positive impact on the performance of the regions manufacturing sectors. The study examines the impact of blockchain technology, big data, and the Internet on productivity, as well as the use of new value chains. According to the results of the study, there is a direct relationship between the independent variable and the dependent variable, but there is no relationship between the moderator and the dependent variable. This shows that blockchain, big data, and the Internet of Things have an incredibly positive impact on productivity, supporting hypotheses 1, 2, and 3. These studies are supported by (Fawcett et al., 2011), who explained how digital technology can be used to facilitate data sharing and improve supply chain efficiency. In line with previous studies (Raguseo, 2018), the data presented here are relevant. He found that big data and digital technology had a positive impact on business performance.
It facilitates the introduction of new technologies, processes, and procedures. However, after the change is implemented, the innovation atmosphere no longer affects the operational performance of the business. Similarly, an environment of creativity is required for enterprises to transition to blockchain technology, but once blockchain technology is installed, it ceases to impact operational performance. Training, knowledge, and adequate facilities and equipment contribute to improved operational performance. Designing and deploying blockchain technology demands a high level of Blockchain skill and knowledge. The majority of tasks, from development to maintenance, are outsourced to firms offering specialized services. The company’s employees are solely instructed on how to utilize the programs; therefore, the innovation climate will have little effect on operational success.
The results show that there is a positive correlation between digital supply chains and blockchain, big data analytics, and the Internet of Things in terms of operational success. Implementing materials and processes in supply chains can increase company productivity. In addition, companies can generate significant revenue and add value by rapidly setting industry standards by adopting digital technology. Internet of Things, robotics, big data, blockchain, and cloud computing are the digital technologies that businesses need to explore for their supply chains. A preliminary study (Haddud and Khare, 2020) supports the results to ensure that all potential benefits of supply chain digitization are fully realized to the level by emphasizing the importance of a professional clerical heart. Digitization in the supply chain can therefore be seen as an additional phenomenon rather than an extraordinary phenomenon (Brinch and Stentoft, 2017). Due to the complex and time-consuming nature of implementing digital technologies, organizations must have specific operational capabilities and a clear understanding of their goals. Identifying the impact of digital supply chains with factors such as blockchain technology, big data analytics, internet of things, contribute significantly to understanding the systemic impact of climate innovation on the interaction between IoT, and operational success We find that digital supply chains play a critical role in performance company and have a positive impact on company performance. In addition, he explains how digital supply chains improve operational efficiency through the introduction of blockchain technology, big data, and the internet of things, but this study does not show any relationship between the new environment and performance.
This study has significant theoretical implications that enhance the body of literature with the theory of resource-based view. This study extended the theory by introducing new variables in it. This study introduced block chain technology as critical for operational performance that was not discussed by the earlier studies. In this way, this significant variable has influence on the performance. Therefore, the future researcher should consider the important role of block chain technology for data analysis. Secondly, this study introduced big data analytics as serious for operational performance that was not conferred by the previous studies. However, this significant variable has influence on the performance. Consequently, the future researcher should consider the important role of big data analysis for learner performance. Lastly, this study introduced internet of things as critical for operational performance that was not discoursed by the existing literature. On the other hand, this substantial variable has impact on the operational performance. Consequently, the scholars should consider the important role of internet of things for operational performance. Importantly, this study pointed out that innovative climate cannot moderate the relationship between digital supply chain and operational performance in the textile sector. In this way, this is the contribution of this research that highlighted the novel relationship in literature and contributed the important role of digital supply chain in operational performance.
The study’s findings have many managerial ramifications. To begin, the prior study has found a link between the digital supply chain and operational success, which broadens the information. Managers can utilize the conceptual research framework to gain a comprehensive and improved understanding of digital supply chain adoption. Second, actual evidence from the study suggests that the digital supply chain can improve operational performance. The research will assist managers in developing nations, particularly those with comparable features, in gaining a better knowledge of the factors that drive the development of the digital supply chain. Furthermore, there must be a requirement for extensive research before investing in the digital supply chain. As a result, the supply chain parameters may be redefined and reconfigured. As a result, managers must be familiar with a variety of converging technologies to make wise investments.
As with any research, the study’s limitations present possibilities to delve deeper into the phenomenon of the digital supply chain. The study’s target group includes top or middle-level management; replies from top-level management are too brief due to their busy schedules and absenteeism. The population of the study was the country’s textile industry. First, there is a limitation regarding the research context. Manufacturers in the textile sector are categorized in a particular country. As a result, the generalization of the findings is limited. As a result, future scholars should conduct additional research on economies with varying levels of technical development and other industries. Second, the information was gathered entirely using self-report questionnaires. Many scholars criticized the approach, yet it seemed appropriate to evaluate each of these characteristics separately. Thus, in the future, the research could be applied to cross-sectional data comparing organizational output before and after deploying digital supply chain technology in the textile industry. Finally, the study analyzed the digital supply chain using blockchain, IoT, and big data, but it can also be measured using robotics, cloud computing, and other technologies in the future.
The raw data supporting the conclusion of this article will be made available by the authors, without undue reservation.
The studies involving human participants were reviewed and approved by The Ethics Committee of Islamia University of Bahawalpur. Written informed consent for participation was not required for this study in accordance with the national legislation and the institutional requirements.
RuY, RaY, MF, and MI conceived the idea of the paper. RaY and MF collected the data. MI and MF wrote the first draft of the manuscript. All the authors significantly contributed to the final version of the manuscript.
This research was funded by the National Natural Science Foundation of China, grant number 92167206; and the Science and Technology Pillar Key Program of Tianjin Key Research and Development Plan, grant number 20YFZCGX00640.
FM was employed by China Automotive Technology and Research Center Co., Ltd.
The remaining authors declare that the research was conducted in the absence of any commercial or financial relationships that could be construed as a potential conflict of interest.
All claims expressed in this article are solely those of the authors and do not necessarily represent those of their affiliated organizations, or those of the publisher, the editors and the reviewers. Any product that may be evaluated in this article, or claim that may be made by its manufacturer, is not guaranteed or endorsed by the publisher.
Abdallah, A. B., Obeidat, B. Y., and Aqqad, N. O. (2014). The impact of supply chain management practices on supply chain performance in Jordan: The moderating effect of competitive intensity. Int. Bus. Res. 7 (3), 13. doi:10.5539/ibr.v7n3p13
Addo-Tenkorang, R., and Helo, P. T. (2016). Big data applications in operations/supply-chain management: A literature review. Comput. Industrial Eng. 101, 528–543. doi:10.1016/j.cie.2016.09.023
Agrawal, P., and Narain, R. (2018). Digital supply chain management: An Overview. IOP Conf. Ser. Mater. Sci. Eng. 455, 012074. doi:10.1088/1757-899X/455/1/012074
Akter, S., Wamba, S. F., Gunasekaran, A., Dubey, R., and Childe, S. J. (2016). How to improve firm performance using big data analytics capability and business strategy alignment? Int. J. Prod. Econ. 182, 113–131. doi:10.1016/j.ijpe.2016.08.018
Amabile, T. M., Conti, R., Coon, H., Lazenby, J., and Herron, M. (1996). Assessing the work environment for creativity. Acad. Manage. J. 39 (5), 1154–1184. doi:10.5465/256995
Amjad, H., Asim, M., and Manzoor, M. S. (2020). Digitization of logistics in fmcg sector of Pakistan: A new approach to strengthen corporate performance. GSJ 8, 2.
Arsyad, A. A., Widayat, I. W., and Köppen, M. (2022). Supporting farming smart documentation system by modular blockchain solutions. Decis. Mak. Appl. Manag. Eng. 5 (1), 1–26. doi:10.31181/dmame0326022022a
Arunachalam, D., Kumar, N., and Kawalek, J. P. (2018). Understanding big data analytics capabilities in supply chain management: Unravelling the issues, challenges, and implications for practice. Transp. Res. Part E Logist. Transp. Rev. 114, 416–436. doi:10.1016/j.tre.2017.04.001
Baig, M. M. U., Ali, Y., and Rehman, O. U. (2022). Enhancing resilience of oil supply chains in context of developing countries. Oper. Res. Eng. Sci. Theor. Appl. 5 (1), 69–89. doi:10.31181/oresta210322091b
Banerjee, A. (2019). Blockchain with IOT: Applications and use cases for a new paradigm of supply chain driving efficiency and cost. Adv. Comput. 115, 259–292. doi:10.1016/bs.adcom.2019.07.007
Bartezzaghi, E., and Turco, F. (1989). The impact of just-in-time on production system performance: An analytical framework. Int. J. Operations Prod. Manag. 9, 40–62. doi:10.1108/eum0000000001257
Behrouzi, F., Wong, K. Y., and Behrouzi, F. (2011). “A study on lean supply chain performance measures of SMEs in the automotive industry,” in IEEE International Conference on Industrial Engineering and Engineering Management, Singapore, 06-09 December 2011 (IEEE).
Berg, C., Davidson, S., and Potts, J. (2019). Understanding the blockchain economy: An introduction to institutional cryptoeconomics. Cheltenham Glos UK: Edward Elgar Publishing.
Boon-itt, S. (2011). Achieving product quality performance: The roles of supply chain integration and information technology. Int. J. Innovation Manag. Technol. 2 (5), 373–376.
Borgia, E. (2014). The Internet of Things vision: Key features, applications and open issues. Comput. Commun. 54, 1–31. doi:10.1016/j.comcom.2014.09.008
Botta, A., De Donato, W., Persico, V., and Pescapé, A. (2016). Integration of cloud computing and internet of things: A survey. Future gener. Comput. Syst. 56, 684–700. doi:10.1016/j.future.2015.09.021
Brinch, M., and Stentoft, J. (2017). Digital supply chains: Still more “wannabe” than practice. DILF Orienter. 54 (2), 22–28.
Bughin, J., Chui, M., and Manyika, J. (2015). An executive’s guide to the Internet of Things. McKinsey Q. 4, 92–101.
Bughin, J., Holley, A., Mellbye, A., Gottlieb, J., and Rutten, E. (2015). Cracking the digital code: McKinsey global survey results. AvaliableAt: https://www.mckinsey.com/business-functions/business-technology/our-insights/cracking-the-digital-code.
Bull, I. H. F. (2005). The relationship between job satisfaction and organisational commitment amongst high school teachers in disadvantaged areas in the Western Cape. Doctoral dissertation. University of the Western Cape.
Büyüközkan Feyzioğlu, G., and Gocer, F. (2018). Digital Supply Chain: Literature review and a proposed framework for future research. Comput. Industry 97, 157–177. doi:10.1016/j.compind.2018.02.010
CapGemini, I. (2016). GTNexus.” the current and future state of digital supply chain transformation, 112. GT Nexus.
Churchill, G. A., and Iacobucci, D. (2006). Marketing research: methodological foundations, 199. New York. Dryden Press.
Cortés, B., Boza, A., Pérez, D., and Cuenca, L. (2015). Internet of things applications on supply chain management. Int. J. Comput. Inf. Eng. 9 (12), 2486–2491.
Dehning, B., Richardson, V. J., and Zmud, R. W. (2007). The financial performance effects of IT-based supply chain management systems in manufacturing firms. J. Operations Manag. 25 (4), 806–824. doi:10.1016/j.jom.2006.09.001
Del Giudice, M., and Della Peruta, M. R. (2016). The impact of IT-based knowledge management systems on internal venturing and innovation: A structural equation modeling approach to corporate performance. J. Knowl. Manag. 20, 484–498. doi:10.1108/jkm-07-2015-0257
Devaraj, S., Krajewski, L., and Wei, J. C. (2007). Impact of eBusiness technologies on operational performance: The role of production information integration in the supply chain. J. operations Manag. 25 (6), 1199–1216. doi:10.1016/j.jom.2007.01.002
Ebrahimi, S. M. (2015). Examining the impact of supply chain integration on organization structure and operational performance in oil and gas supply chains: A contingency approach. Doctoral dissertation. University of Sheffield.
Fawcett, S. E., Wallin, C., Allred, C., Fawcett, A. M., and Magnan, G. M. (2011). Information technology as an enabler of supply chain collaboration: A dynamic-capabilities perspective. J. supply chain Manag. 47 (1), 38–59. doi:10.1111/j.1745-493x.2010.03213.x
Franco-Santos, M., Kennerley, M., Micheli, P., Martinez, V., Mason, S., Marr, B., et al. (2007). Measuring performance in a changing business environment. Int. J. operations Prod. Manag. 23, 213–229. doi:10.1108/01443570310458465
Fynes, B., Voss, C., and De Búrca, S. (2005). The impact of supply chain relationship quality on quality performance. Int. J. Prod. Econ. 96 (3), 339–354. doi:10.1016/j.ijpe.2004.05.008
Gallouj, F., and Weinstein, O. (1997). Innovation in services. Res. policy 26 (4-5), 537–556. doi:10.1016/s0048-7333(97)00030-9
Gobble, M. M. (2013). Big data: The next big thing in innovation. Research-technology Manag. 56 (1), 64–67. doi:10.5437/08956308x5601005
Gong, M., Simpson, A., Koh, L., and Tan, K. H. (2018). Inside out: The interrelationships of sustainable performance metrics and its effect on business decision making: Theory and practice. Resour. Conservation Recycl. 128, 155–166. doi:10.1016/j.resconrec.2016.11.001
Gubbi, J., Buyya, R., Maurusic, S., and Palaniswami, M. (2013). Internet of things (IoT): A vision, architectural elements, and future directions. Future Gener. Comput. Syst. 29, 1645–1660. doi:10.1016/j.future.2013.01.010
Haddud, A., and Khare, A. (2020). Digitalizing supply chains potential benefits and impact on lean operations. Int. J. Lean Six Sigma 11, 731–765. doi:10.1108/ijlss-03-2019-0026
Hair, J. F., Ringle, C. M., and Sarstedt, M. (2012). Partial least squares: The better approach to structural equation modeling? Long. range Plan. 45 (5-6), 312–319. doi:10.1016/j.lrp.2012.09.011
Hair, J. F., Ringle, C. M., and Sarstedt, M. (2013). Partial least squares structural equation modeling: Rigorous applications, better results and higher acceptance. Long. range Plan. 46 (1-2), 1–12. doi:10.1016/j.lrp.2013.01.001
Haleem, A., Kumar, S., and Luthra, S. (2018). Flexible system approach for understanding requisites of product innovation management. Glob. J. Flex. Syst. Manag. 19 (1), 19–37. doi:10.1007/s40171-017-0171-7
Hamel, G. (1998). Opinion: Strategy innovation and the quest for value. Sloan Manag. Rev. 39 (2), 7–14.
Hart, O. (1995). Corporate governance: Some theory and implications. Econ. J. 105 (430), 678–689. doi:10.2307/2235027
Henseler, J., Ringle, C. M., and Sinkovics, R. R. (2009). “The use of partial least squares path modeling in international marketing,” in New challenges to international marketing (Emerald Group Publishing Limited).
Hoberg, P., Krcmar, H., Oswald, G., and Welz, B. (2015). Research report: Skills for digital transformation. Germany: SAP SE and Technical University of Munich.
Hulland, J. (1999). Use of partial least squares (pls) in strategic management research: A review of four recent studies. Strateg. Manag. J. 20 (2), 195–204. doi:10.1002/(sici)1097-0266(199902)20:2<195::aid-smj13>3.0.co;2-7
Ijaz, A., Nabeel, M., Masood, U., Mahmood, T., Hashmi, M. S., Posokhova, I., et al. (2022). Towards using cough for respiratory disease diagnosis by leveraging Artificial Intelligence: A survey. Inform. Med. Unlock., 100832.
Jung, D. I., Chow, C., and Wu, A. (2003). The role of transformational leadership in enhancing organizational innovation: Hypotheses and some preliminary findings. Leadersh. Q. 14 (4-5), 525–544. doi:10.1016/s1048-9843(03)00050-x
Kim, M., Ahn, H., and Kim, K. P. (2016). Process-aware internet of things: A conceptual extension of the internet of things framework and architecture. KSII Trans. Internet Inf. Syst. (TIIS) 10 (8), 4008–4022.
Kollmann, T., Hensellek, S., de Cruppe, K., and Sirges, A. (2020). Toward a renaissance of cooperatives fostered by blockchain on electronic marketplaces: A theory-driven case study approach. Electron. Mark. 30 (2), 273–284. doi:10.1007/s12525-019-00369-4
Lakhani, K. R., and Iansiti, M. (2017). The truth about blockchain. Harv. Bus. Rev. 95 (1), 119–127.
Li, S., Xu, L. D., and Zhao, S. (2015). The internet of things: A survey. Inf. Syst. Front. 17 (2), 243–259. doi:10.1007/s10796-014-9492-7
Lin, K. P., Tseng, M. L., and Pai, P. F. (2018). Sustainable supply chain management using approximate fuzzy DEMATEL method. Resour. Conservation Recycl. 128, 134–142. doi:10.1016/j.resconrec.2016.11.017
Lu, D., Ding, Y., Asian, S., and Paul, S. K. (2018). From supply chain integration to operational performance: The moderating effect of market uncertainty. Glob. J. Flex. Syst. Manag. 19 (1), 3–20. doi:10.1007/s40171-017-0161-9
Madakam, S., Lake, V., Lake, V., and Lake, V. (2015). Internet of things (IoT): A literature review. J. Comput. Commun. 3 (05), 164–173. doi:10.4236/jcc.2015.35021
Madhwal, Y., and Panfilov, P. B. (2017). Blockchain and supply chain management: Aircrafts parts business case. Ann. DAAAM Proc. 28, 1051–1056.
Malhotra, Y. (2000). Knowledge management for e-business performance: Advancing information strategy to “internet time”. Inf. Strategy Executive's. J. 16 (4), 5–16. doi:10.1080/07438613.2000.10744620
Matt, C., Hess, T., and Benlian, A. (2015). Digital transformation strategies. Bus. Inf. Syst. Eng. 57 (5), 339–343. doi:10.1007/s12599-015-0401-5
Mayer-Schönberger, V., and Cukier, K. (2013). Big data: A revolution that will transform how we live, work, and think. Houghton Mifflin Harcourt.
Mikalef, P., Pappas, I. O., Krogstie, J., and Giannakos, M. (2018). Big data analytics capabilities: A systematic literature review and research agenda. Inf. Syst. E-Bus. Manage. 16 (3), 547–578. doi:10.1007/s10257-017-0362-y
Mumford, M. D., Scott, G. M., Gaddis, B., and Strange, J. M. (2002). Leading creative people: Orchestrating expertise and relationships. Leadersh. Q. 13 (6), 705–750. doi:10.1016/s1048-9843(02)00158-3
Muniesa, F. (2011). Is a stock exchange a computer solution?: Explicitness, algorithms and the Arizona Stock Exchange. Int. J. Actor-Network Theory Technol. Innovation 3 (1), 1–15. doi:10.4018/jantti.2011010101
Park, S., Jo, S. J., Kwon, Y. J., and Shim, J. Y. (2017). Association between the eating family meal and the prevalence of metabolic syndrome using data from Korea national health and nutrition examination survey (2007-2012). Korean J. Fam. Med. 39 (1), 130–134. doi:10.4082/kjfm.2017.38.3.130
Pisano, G. P. (1997). The development factory: Unlocking the potential of process innovation. Harvard Business Press.
Raguseo, E. (2018). Big data technologies: An empirical investigation on their adoption, benefits and risks for companies. Int. J. Inf. Manag. 38 (1), 187–195. doi:10.1016/j.ijinfomgt.2017.07.008
Rajasekar, S., Philominathan, P., and Chinnathambi, V. (2013). Research methodology. eprint, 1–53. arXiv preprint physics/0601009.
Ramayah, T., Lee, J. W. C., and In, J. B. C. (2011). Network collaboration and performance in the tourism sector. Serv. Bus. 5 (4), 411–428. doi:10.1007/s11628-011-0120-z
Redmond, M. R., Mumford, M. D., and Teach, R. (1993). Putting creativity to work: Effects of leader behavior on subordinate creativity. Organ. Behav. Hum. Decis. Process. 55 (1), 120–151. doi:10.1006/obhd.1993.1027
Risius, M., and Spohrer, K. (2017). A blockchain research framework. Bus. Inf. Syst. Eng. 59 (6), 385–409. doi:10.1007/s12599-017-0506-0
Russo, G., Marsigalia, B., Evangelista, F., Palmaccio, M., and Maggioni, M. (2015). Exploring regulations and scope of the internet of things in contemporary companies: A first literature analysis. J. Innov. Entrep. 4 (1), 11–13. doi:10.1186/s13731-015-0025-5
Russo, M. V., and Fouts, P. A. (1997). A resource-based perspective on corporate environmental performance and profitability. Acad. Manage. J. 40 (3), 534–559. doi:10.5465/257052
Scott, S. G., and Bruce, R. A. (1994). Determinants of innovative behavior: A path model of individual innovation in the workplace. Acad. Manage. J. 37 (3), 580–607. doi:10.5465/256701
Scuotto, V., Ferraris, A., and Bresciani, S. (2016). Internet of things: Applications and challenges in smart cities: A case study of IBM smart city projects. Bus. Process Manag. J. 22, 357–367. doi:10.1108/bpmj-05-2015-0074
Tabachnick, B. G., Fidell, L. S., and Ullman, J. B. (2007). Using multivariate statistics. Boston, MA: Harper & Row.
Tapscott, D., and Tapscott, A. (2016). Blockchain revolution: How the technology behind bitcoin is changing money, business, and the world. New York, NY: Penguin.
Tapscott, D., and Tapscott, A. (2017). How blockchain will change organizations. MIT Sloan Manag. Rev. 58 (2), 10.
Terziovski, M. (2010). Innovation practice and its performance implications in small and medium enterprises (SMEs) in the manufacturing sector: A resource-based view. Strateg. Manag. J. 31 (8), 892–902. doi:10.1002/smj.841
Tian, F. (2017). “A supply chain traceability system for food safety based on HACCP, blockchain & Internet of things,” in 2017 International conference on service systems and service management, Dalian, 16-18 June 2017 (IEEE).
Treiblmaier, H. (2018). The impact of the blockchain on the supply chain: A theory-based research framework and a call for action. Supply Chain Manag. An Int. J. 23, 545–559. doi:10.1108/scm-01-2018-0029
Vinzi, V. E., Chin, W. W., Henseler, J., and Wang, H. (2010). Handbook of partial least squares. Springer Berlin, Heidelberg: Springer.
Vrontis, D., Thrassou, A., Chebbi, H., and Yahiaoui, D. (2012). Transcending innovativeness towards strategic reflexivity. Qual. Mark. Res. An Int. J. 15, 420–437. doi:10.1108/13522751211257097
Wade, M., and Marchant, D. (2014). “Are you prepared for your digital transformation,” in Understanding the power of technology AMPS in organizational change. Tomorrow’s challenges (Switzerland: IMD Lausanne).
Wang, G., Gunasekaran, A., Ngai, E. W., and Papadopoulos, T. (2016). Big data analytics in logistics and supply chain management: Certain investigations for research and applications. Int. J. Prod. Econ. 176, 98–110. doi:10.1016/j.ijpe.2016.03.014
Weichhart, G., Molina, A., Chen, D., Whitman, L. E., and Vernadat, F. (2016). Challenges and current developments for sensing, smart and sustainable enterprise systems. Comput. Industry 79, 34–46. doi:10.1016/j.compind.2015.07.002
White, M. (2012). Digital workplaces: Vision and reality. Bus. Inf. Rev. 29 (4), 205–214. doi:10.1177/0266382112470412
Whitmore, A., Agarwal, A., and Da Xu, L. (2015). The internet of things—a survey of topics and trends. Inf. Syst. Front. 17 (2), 261–274. doi:10.1007/s10796-014-9489-2
Wong, C. Y., Boon-Itt, S., and Wong, C. W. (2011). The contingency effects of environmental uncertainty on the relationship between supply chain integration and operational performance. J. operations Manag. 29 (6), 604–615. doi:10.1016/j.jom.2011.01.003
Wood, L. C., Wang, J. X., Duong, L. N., Reiners, T., and Smith, R. (2018). Stock market reactions to auto manufacturers’ environmental failures. J. macromarketing 38 (4), 364–382. doi:10.1177/0276146718781915
Wortmann, F., and Flüchter, K. (2015). Internet of things. Bus. Inf. Syst. Eng. 57 (3), 221–224. doi:10.1007/s12599-015-0383-3
Zaltman, G., Duncan, R., and Holbek, J. (1973). Innovations and organizations. New YorkToronto: Wiley.
Keywords: digital supply chain, blockchain, internet of things, big data, innovative climate, operational performance
Citation: Men F, Yaqub RMS, Yan R, Irfan M and Fatima Me (2022) Resource-based theory perspective in the textile industry: The impact of the digital supply chain on operational performance. Front. Environ. Sci. 10:1017297. doi: 10.3389/fenvs.2022.1017297
Received: 11 August 2022; Accepted: 18 October 2022;
Published: 25 November 2022.
Edited by:
Željko Stević, University of East Sarajevo, Bosnia and HerzegovinaReviewed by:
Morteza Yazdani, Fundación de la Universidad Autónoma de Madrid, SpainCopyright © 2022 Men, Yaqub, Yan, Irfan and Fatima. This is an open-access article distributed under the terms of the Creative Commons Attribution License (CC BY). The use, distribution or reproduction in other forums is permitted, provided the original author(s) and the copyright owner(s) are credited and that the original publication in this journal is cited, in accordance with accepted academic practice. No use, distribution or reproduction is permitted which does not comply with these terms.
*Correspondence: Muhammad Irfan, ZHIubWlyZmFuQGJ6dS5lZHUucGs=
Disclaimer: All claims expressed in this article are solely those of the authors and do not necessarily represent those of their affiliated organizations, or those of the publisher, the editors and the reviewers. Any product that may be evaluated in this article or claim that may be made by its manufacturer is not guaranteed or endorsed by the publisher.
Research integrity at Frontiers
Learn more about the work of our research integrity team to safeguard the quality of each article we publish.