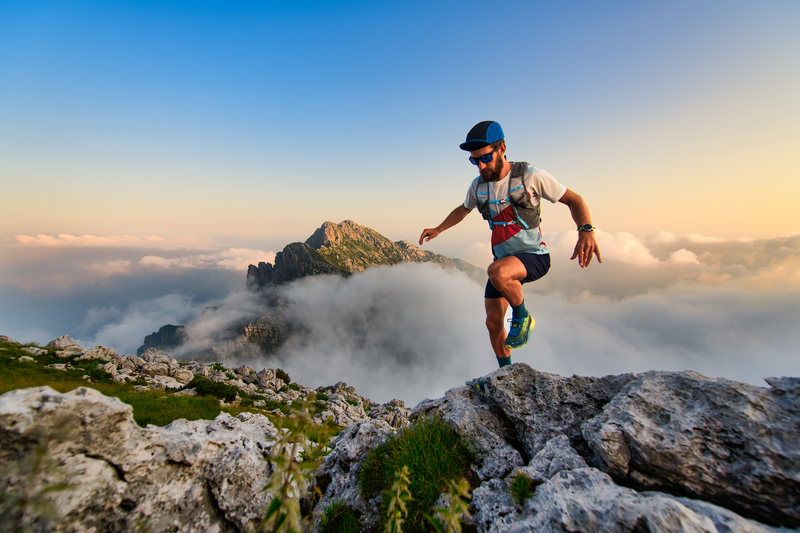
95% of researchers rate our articles as excellent or good
Learn more about the work of our research integrity team to safeguard the quality of each article we publish.
Find out more
ORIGINAL RESEARCH article
Front. Environ. Sci. , 02 November 2022
Sec. Environmental Economics and Management
Volume 10 - 2022 | https://doi.org/10.3389/fenvs.2022.1008986
Based on the data of Shanghai and Shenzhen A-share listed companies from 2015 to 2019, this paper studies the influence of air quality on the R&D investment of listed companies from the perspectives of investor sentiment and government concern. It is found that, on the whole, air quality has a significant inhibitory effect on R&D investment. Air quality significantly promotes investor sentiment, which serves as a path to further restrain the R&D investment of listed companies. Air pollution is an effective concern for the government and serves as a veil between air quality and R&D investment. Furthermore, this paper analyzes the heterogeneity of enterprises from the aspects of regional technology complexity, property right nature, whether it is a polluting enterprise or not, and whether it is a key regulated enterprise or not, and comes to relevant conclusions. This paper expands the research on air quality and enterprise R&D investment decision-making, which helps to clarify and improve the transmission mechanism and implementation effect of environmental protection policies.
“China Ecological Environment Bulletin 2019″ shows that in 2019, China’s urban air quality standards accounted for 46.6 percent of the total number of cities, an increase of 10.8% compared to 2018, and the number of city ambient air quality exceeded the standard was 180, accounting for 53.4% of the total number of cities. A total of 452 days of serious pollution occurred in 337 cities, 183 days less than in 2018; 1,666 days of serious pollution, (Figure 1). For a long time, the rapid growth of China’s economy based on energy consumption has caused a serious imbalance of China’s ecological environment, especially the air quality problem. The economic high-efficiency growth pattern with independent innovation as the core is the core path to realizing the high-quality development of China’s economy (He et al., 2020), and a significant leap in scientific and technological strength is set as one of the long-term goals in the 14th Five-Year Plan.
China’s economy has been in a period of continuous development in recent decades, while high-frequency economic activities have led to a continuous decline in air conditions (Hao et al., 2020). With regard to the current severe situation of air pollution, China has issued “the action plan for air pollution prevention” and “control and the 3-year action plan for winning the blue sky defense war” to make strategic arrangements for air pollution prevention and control. The former stipulates the task of reducing air pollutant emissions for core industries and regions and adds it to the government assessment, clarifying the consequences of failing to meet the standards. In this case, in order to transform the economy to green development, the government advocates that all enterprises should make the green transformation, promote technological progress and scientific and technological progress, and it is necessary to study whether air pollution will affect the R&D and innovation of micro-enterprises, and what path it will take to affect the R&D and innovation of enterprises.
The search for the high-quality development of both air and the economy has been the focus of many scholars’ research. The available literature on air pollution has focused on environmental pollution and social welfare (Wang et al., 2021), the formation of air pollution (Vega et al., 2021), governance mechanism and effect (Liu et al., 2021), labor supply, and resident’s happiness (Li et al., 2020; Sanduijav et al., 2021) and impact of green investment (Ren et al., 2021) and internet on environmental pollution (Ren et al., 2022). However, there are a few research studies on the impact of air pollution on micro- enterprises’ R&D and innovation behavior, and the impact paths are mainly focused on the perspectives of regulatory mechanisms (Zhang et al., 2020; Wang et al., 2021), human capital (Liu et al., 2021), and corporate social responsibility (Wang et al., 2021) and so on. On the basis of previous studies, this paper takes investor sentiment and government concern as the core paths to explore the effect of air pollution on enterprise R&D investment. The domestic and foreign references for the two are summarized as follows.
In the aspect of air pollution and investor sentiment, from the point of view of environmental psychology, the negative effect of air pollution has been concluded, and the negative effect of air pollution is the result of the interaction of many factors. Psychologists have long known that environmental factors influence people’s moods, thoughts, and judgment (Hirshleifer and Shumway 2003; Jacobsen and Marquering, 2008). The psychological effects of air pollution were not only associated with life satisfaction and subjective well-being (Dolan and Laffan, 2016), but also correlated with people’s mood (Lim et al., 2012). Emotional reactions may be the most sensitive among the possible adverse effects of air pollution (Power et al., 2015). Weather factors can directly affect individual behavior and psychological processes or indirectly affect individual behavior. In addition, weather factors will directly impact various activities within the enterprise and the enterprise relations in the industrial chain or indirectly impact the business behavior (Gao and Zhang, 2020). There is a negative relation between air pollution and an enterprise’s stock return, and the air pollution effect is particularly strong for enterprises that are more likely to be held by local investors, such as younger enterprises, enterprises with lower institutional ownership, and enterprises covered by fewer analysts (Ding et al., 2021). Severe air pollution results in low returns, turnover, volatility, and high illiquidity. The relationship between air pollution and local enterprise performance is insignificant, implying that the effects of air pollution can be attributed to the emotional bias of investors (Wu et al., 2018).
The innovation of knowledge has the characteristics of the great demand for fund support, high risk of innovation activities, and obvious uncertainty of benefits. Therefore, the R&D investment of enterprises has become the leading factor in realizing innovation activities (Song et al., 2021). In terms of investor sentiment and enterprise R&D, pessimistic individual investor sentiment reduces liquidity and volatility. The mispricing caused by individual investor sentiment cannot be eliminated using risk-factor models (Wu and Lu, 2020). Market sentiment further affects R&D investments by influencing manager sentiment (Dang and Xu. 2018). High or low market sentiment reflects investors’ general optimism or pessimism about asset prices (Brown and Cliff, 2005), and this kind of irrational sentiment will lead to mispricing of the stock and have an important influence on the capital investment decision of the enterprise (Baker and Wurgler. 2004; Polk and Sapienza. 2009). Besides, high or low investor sentiment can “shape” managers’ homogeneous sentiment and thus influence the enterprise’s investment and other decision-making behavior (Nofsinger, 2005). Based on the above facts, the adverse effects of air pollution will affect both the investment behavior of micro-individuals and the innovation activities of enterprises. Therefore, this paper tries to analyze how air quality affects enterprise R&D investment by taking individual investor behavior, namely investor sentiment, as an influence path.
In addition, as the main body of micro-development, enterprises are the focus of the government’s attention and also the foothold of implementing relevant environmental regulation policies. The implementation effect of the relevant government policies will be reflected through the overall effect of all micro-enterprises individual innovation behavior. China’s coal-based, energy-intensive development path in air quality and government supervision has led to severe air pollution problems (Du et al., 2018; Hein et al., 2019). And air pollution is a major threat to human health and life satisfaction (Han et al., 2017; Tang et al., 2018). Severe air pollution associated with rapid urbanization is a pressing issue in China. The Chinese public’s awareness of environmental protection is awakening, which poses enormous pressure on governments to enforce environmental regulations (Lu et al., 2018). The Chinese government has proposed to establish an ecological compensation (EC) mechanism for air quality to deal with the country’s serious air pollution problem (Cui et al., 2021). In government supervision and enterprise R&D investment, the Chinese government R&D subsidies significantly incentivize enterprise technology innovation investment (Jia et al., 2021). And government public support is an important policy tool to support enterprises in carrying out innovation and R&D in China (Xiang et al., 2021). But there are different conclusions. Although government support can help alleviate financial constraints and encourage funded enterprises to invest in innovative activities (Buisseret et al., 1995), such support may simply induce funded enterprises to replace their R&D investment with scarce public funds, thereby crowding out their spending (Ziga-Vicente et al., 2014).
From the above literature review of air quality and enterprise R&D investment, it can be seen that there is relatively little research on how air quality affects the R&D innovation of micro-enterprises. In previous studies, we can find the impact of air quality on investor sentiment and government attention, and the impact of both on Enterprise R&D investment has been studied by scholars. But at present, there is no article trying to study the impact of air quality on Enterprise R&D investment through investor sentiment and government attention. Therefore, the two research paths selected in this paper are feasible and fill the gap in this part.
Therefore, this paper analyzes the relationship between air governance and corporate R&D investment by using government supervision as another influence path. Government supervision plays an important role in regulating the market, building investor confidence, and relieving the financing difficulties of R&D enterprises. Therefore, based on the reality of China’s capital market and the reality of listed companies, the government supervision factor is embedded into the research category of air pollution and enterprise R&D investment, and it is necessary to further reveal how the institutional factors affect and intervene the process of air pollution’s effect on enterprise micro-R&D investment. On the one hand, under the pressure of environmental performance assessment and social and media attention, regional air pollution will force the government to pay more attention to, formulate and revise relevant environmental protection policies. Various positive fiscal and taxation policies formulated by the government can encourage technological innovation of enterprises, regulate the market using “visible hands”, maintain and boost investor confidence. In addition, it enhances the capital market’s influence on enterprise innovation and drives the innovation economy’s transformation and adjustment. The impact mechanism of this paper is shown in Figure 2. In a word, the enterprise R&D level is the key field of green and low-carbon industry transformation in China. It is the key to carrying out the innovation-driven development strategy in the context of high-quality economic development to probe into the path and main obstacles of R&D from the micro-perspective of enterprises.
As an important subject of responsibility for pollution control, enterprises are bound to be affected by air pollution in terms of investor sentiment and government attention during production and operation. On the one hand, air pollution will stimulate investors’ sentiment, affect their investment judgment ability, and then make enterprises change their R&D investment; On the other hand, the decline of air quality will make the government pay more attention to the environment and formulate and release some environmental regulation policies. The introduction of these policies not only reduced the amount of pollutants emitted by enterprises but also urged enterprises to invest more money in R&D and innovation. It can be seen that exploring the impact of air quality on the total factor productivity of enterprises has certain practical significance and theoretical value for improving air quality, promoting the improvement of total factor productivity of enterprises, and further promoting the realization of green, healthy and sustainable economic development.
According to the above analysis, this paper puts forward the following three assumptions:
Hypothesis 1. air pollution has a restraining effect on the R&D investment of enterprises.
Hypothesis 2. air quality affects investor sentiment, thus changing enterprise R&D investment.
Hypothesis 3. air quality affects the government’s attention, thus changing the R&D investment of enterprises.
This paper is mainly divided into the following parts:
The first part is the introduction. First of all, the current situation of air quality and the significance of the research are described. Secondly, the literature review is done on the impact of air quality on investor sentiment and government attention and the impact of investor sentiment and government attention on Enterprise R&D. finally, the existing literature is comprehensively reviewed; The second part is the research design part, which mainly explains the samples and data sources, constructs the mediation effect model, and defines the variables used in the article; The third part is the result analysis and conclusion, which studies the impact of air quality on the R&D investment of enterprises under the two paths of investor sentiment and government concern; The fourth part is the conclusion and suggestion. This paper expounds and summarizes the results after empirical testing, and puts forward some suggestions on the results.
This paper selected all the listed companies of Shanghai and Shenzhen A-shares as the sample of enterprises, in which the enterprises with missing data were excluded. Furthermore, the daily Air Quality (AQ) of prefecture-level cities across the country was collected from the Ministry of Ecology and Environment of the People’s Republic of China. Considering the significant changes in the air quality evaluation index system in China after 2014, this paper only selected air quality data in 2015 and later to ensure the consistency of the calculation of air pollution degree. This paper selected the sample of enterprises from 2015 to 2019 and processed the sample according to the following principles: 1) Eliminating companies with incomplete data; 2) Considering the significance of extreme weather in the study of this paper, this paper only conducted Winsorize (1%) treatment on the continuous variables of financial indicators. The data in this paper are mainly from the CSMAR database, and the data of the macro-economic level are from the Statistical Yearbook of Chinese Cities. In the city’s technical complexity data, the patent data comes from the State Intellectual Property Office.
In order to analyze the impact path of air pollution on the R&D investment of enterprises, this paper sets up an extensible benchmark measurement model 1) as follows. This paper will take the logarithm treatment with the explained variables:
The logarithmic transformation in this paper is a semi logarithmic model, that is, only the logarithm of the explained variable is taken. The reasons are as follows:
One side, for variables greater than 0, the conditional distribution usually has heteroscedasticity and skewness, because taking logarithm can weaken these two problems. On the other hand, The coefficient of the log linear model measures the elasticity of one variable (CRD) to another explanatory variables, which is the relative change rate of the explained variable (CRD) caused by the unit absolute change of the explanatory variables.
Where β0 is a constant term, β1 and β- represent regression coefficients, i represents the listed company, t represents the year, j represents the prefecture-level city where the listed company i is located. CRDit represents the R&D investment of enterprise i in the t period, AQIijt represents the degree of air pollution in period t of j where enterprise i is located. Xi represents the control variable at the enterprise and macro levels, Dummy represents the corresponding Virtual variable, and εit defines the error term.
Based on the above analysis of the impact mechanism of air quality on R&D investment of enterprises, this paper studies and discusses the possible impact path through the intermediary effect model. By referring to the step-by-step method of testing the intermediate effect proposed by Baron and Kenny (1986), the following mediating effect model for testing is constructed (Baron and Kenny, 1986):
Where yit is the explained variable, xit is the explanatory variable, and mit represents the intermediate variable, including investor sentiment (Sent) and government supervision (GS).
When the coefficient a1 is significant, if the coefficients b1 and c2 are significant, it indicates an indirect effect. At this time, when c1 is not significant, there is a complete intermediate effect. When c1 is significant, if b1*c2 and c1 have the same sign, there is a partial intermediate effect; if b1*c2 and c1 have different signs, there is a masking effect. The related variables and their symbols and definitions involved in this paper are shown in Table 1.
Referring to related research, this paper uses R&D expenditure/main business income as a substitute variable for innovation input (Hansen and Hill, 1991). At the same time, R&D personnel investment is another commonly used measurement indicator for enterprises’ investment in R&D and innovation. Therefore, this paper adopts R&D personnel investment as another substitute variable of enterprise R&D investment to ensure the robustness of the empirical results.
This paper uses the average daily air quality of the city where the enterprise is located to measure air pollution, namely the average air quality (Jans et al., 2018; Du et al., 2022).
Investor sentiment (Sent): In this paper, we consider the time effect of the momentum effect, which is usually 3–12 months. However, the momentum index of China’s stock market is significant in the half-year period, and beyond the half-year period or longer will be reversed. Therefore, this paper uses a half-year momentum index as the substitute variable of investor sentiment to carry on the follow-up study. That is, the cumulative monthly rate of return Sent which considers a cash bonus with a 6-month lag period, is used to measure investor sentiment. At the same time, the cumulative monthly rate of return Sent0, which does not consider a cash bonus with a 6-month lag period, is used to test the robustness (Gao et al., 2022).
Government supervision (GS): It is common practice at home and abroad that the government funds R&D funds, and social forces are involved. How the government encourages and promotes more social forces to participate in R&D investment is an issue that needs attention in China’s current science and technology innovation. For micro-level enterprises, this paper uses the calculation of R&D concern of regional governments for reference and uses the total of government subsidies to enterprises (total of government subsidy profits, VAT returns, subsidies, etc.) to represent the government supervision (Hein et al., 2019).
According to the research of related scholars, this paper selects asset-liability ratio (ALE), enterprise size (Size), cash stock (Cash), free cash flow (FCF), technical complexity (TCD), city year-end population (CYP), and GDP growth rate (GDP) as control variables. The regional technical complexity can be divided into large technical complexity (TCD) and small technical complexity (TCD0) according to the types of patent classification (Song et al., 2021; Peters et al., 2022).
In this paper, the heterogeneity of the research questions is analyzed by Virtual variables, which are based on whether the listed enterprise is a key pollution monitoring enterprise (key pollution monitoring enterprises are 1 and the rest are 0), whether it is a state-owned enterprise (state-owned enterprises are 1 and the rest are 0), whether it is a polluting enterprise (polluting enterprises are 1 and the rest are 0), whether the location of listed companies is a high-tech complexity area (high-tech complexity areas are 1 and the rest are 0), etc.
Table 2 shows the descriptive statistical results of the main variables. The mean value of listed companies’ R&D investment (CRD) is 4.111, and the standard deviation is 1.451, indicating that there is a large gap in the R&D investment status among different listed companies. The mean value of air quality (AQ) is 2.983, the median is 2.994, and the standard deviation is 0.708, indicating that the air quality in cities of China has been evenly distributed in recent years, and in some areas, it has been improved. The mean value of investor sentiment is -0.007, and the standard deviation is 0.342, the maximum stock return is 13.197, and the minimum is -0.044, indicating that the impact of investor sentiment on the returns of various enterprises is very different. The mean value of government supervision is 51.068, and the standard deviation is 246.852, indicating that the government’s support is obvious, but there is great heterogeneity. The statistical results of other variables are in line with the overall situation of enterprises in China. Therefore, it is very necessary to analyze the heterogeneity of the research in this paper. From the statistical results, we can see that non-key pollution monitoring companies, non-state-owned companies, and non-pollution companies accounted for a large proportion of the sample.
Table 3 shows the benchmark regression results of Formula 1, the models 1) and 2) are the regression results of fixed effect and random effect with the listed companies’ R&D investment as the explained variable, and models 3) and 4) are the regression results of fixed effect and random effect with the listed companies’ R&D personnel as the explained variable. This paper further found through Hausman test (the result is rejecting the null hypothesis, which is not shown in the text) that the fixed effect model should be used for analysis in this paper. Therefore, the analysis in this paper is based on the results of the fixed effect model. The random effect results are similar to the results, which increases the robustness of the regression results in this paper.
According to the regression results of models 1) and (3), the estimated coefficients of AQI are all negative at the significant level of 1%, and the economic implications are as follows: Taking model 1) as an example, the regression coefficient of -0.2097 indicates that an increase of 1 unit in the Air Quality (AQ) reduces the R&D investment of listed companies by 20.97% of the total asset investment at the beginning of the period. It shows that the worse the air quality is, the less the R&D investment of the listed companies is. Air pollution has led to an increase in the company’s production and operation costs, leading to a decrease in R&D funds. However, the increase in cost did not force the improvement of the company’s production process and management level in time. Thus, hypothesis 1 is tested and confirmed.
Table 4 shows the intermediary effect benchmark regression results of Formula 2, and the intermediary variable is investor sentiment (Sent). In Table 4, the models (1)–3) are the regression results of the intermediary effect with the listed companies’ R&D investment as the explained variable, and the models (4)–6) are the regression results of the intermediary effect with the listed companies’ R&D personnel as the explained variable. The results show that the explanatory variable air quality (AQ) has a significantly positive influence coefficient b1 on the intermediary variable investor sentiment (Sent) whether for R&D investment or R&D personnel. It means that air pollution can stimulate investor sentiment. At this time, the explanatory variable air quality (AQ) and the intermediary variable investor sentiment (Sent) in columns 3 and 6 have significant coefficients c1 and c2 for the explained variable R&D investment, and b1c2 and c1 have the same sign, indicating that investor sentiment has an intermediary effect between air quality and R&D investment. The former regression coefficient for investor sentiment is -0.0662, and for air quality (AQ), it is -0.2052, both of which are significant at the 1% significance level. The latter regression coefficient for investor sentiment is -0.0158, and for air quality, it is -0.0377, both of which are significant at the 10% significance level. And the regression coefficient of air quality on investor sentiment is 0.0684, which is significant at the 1% significance level. The results of models (1)–3) are consistent with those of models (4)–(6). In general, air quality significantly inhibits R&D Investment, and the inhibitory effect is slightly weakened when the intermediary variables are added. And because air quality has a significant effect on investor sentiment, this approach further restrains the R&D investment of listed companies. On the whole, with the aggravation of air pollution, the R&D investment of enterprises will decrease under the capital crowding-out effect and human resource loss effect. Especially, the high-tech talents with a stronger ability to avoid pollution and better ability to bear population flow will lead to the decline of innovation quality of enterprises in polluted areas. Thus, hypothesis 2 is tested and confirmed.
From a micro perspective, the higher the degree of air pollution is, the higher the investor sentiment is. And different from the previous research conclusion, it has an indirect inhibitory effect on the R&D investment of listed companies by using investor sentiment as the influence path. And the indirect effect of this part is -0.0045. This indicates that the upsurge of investment sentiment caused by the increase of air pollution level has not stimulated the innovation vigor of the listed companies but may hinder the financing constraints of enterprises and weaken the sensitivity of the R&D investment of the listed companies, and at the same time, the rationality of managers and the irrationality of investors have a reverse effect, which does not cater to investors and indirectly restrains the R&D Investment of listed companies.
Table 5 shows the intermediary effect benchmark regression results of Formula 2, and the intermediary variable is government supervision (GS). In Table 5, the models (1)–3) are the regression results of the intermediary effect with the listed companies’ R&D investment as the explained variable, and the models (4)–6) are the regression results of the intermediary effect with the listed companies’ R&D personnel as the explained variable. The results show that the explanatory variable air quality (AQ) has a significantly positive influence coefficient b1 on the intermediary variable government supervision (GS), whether for R&D investment or for R&D personnel. It means that air pollution will be of effective concern to the government, and measures will be taken in response. At this time, the explanatory variable air quality (AQ) and the intermediary variable government supervision (GS) in columns 4 and 6 have significant coefficients c1 and c2 for the explanatory variable R&D investment, and b1c2 and c1 have the same sign, indicating that government supervision has a masking effect between air quality and R&D investment. The former regression coefficient for government supervision is 0.0001, and for air quality (AQ), it is -0.2052, both of which are significant at the 1% significance level. The latter regression coefficient for government supervision is 0.0001, and for air quality, it is -0.0399, both of which are significant at the 10% significance level. And the regression coefficient of air quality on investor sentiment is 17.4213, which is significant at the 1% significance level. The results of models (1)–3) are consistent with those of models (4)–(6). Thus, hypothesis 3 is tested and confirmed.
The aggravation of air pollution can indeed attract the effective attention of the government and cause the government to implement the corresponding subsidy policies. The implementation of corresponding subsidy policies can also indirectly increase R&D investment of listed companies. But at the same time, it may cause enterprise managers to pay too much attention to this kind of speculative profit-seeking and expand the demand for their own interests. Enterprises may be less motivated to innovate, which in turn reduces investment in research and development. The crowding-out effect of government supervision may offset or even outweigh its promotional effect.
Since the results of the explained variables in Tables 4, 5 are consistent, the empirical results of R&D investment represented by R&D fund investment and R&D personnel are robust, and the results of R&D fund investment are more significant. Therefore, the later empirical analysis of this paper only uses R&D fund investment as the explained variable.
Among the results in Tables 4, 5, it is noteworthy that the urban technical complexity (TCD) is an indicator closely related to enterprise R&D innovation. In Tables 4, 5, the regression results of technological complexity on enterprise R&D innovation are both significant at the 1% level. Technical complexity represents the comparative advantage of urban technology. At present, enterprise innovation is based on the innovation of complex products, not the innovation of single technology, so it needs multi-technology support. Therefore, the technological complexity of the cities where the listed companies are located determines the differences in the environments in which they conduct their innovation activities. This paper classifies the technical complexity by means of average value. The cities above the average value are high technical complexity areas, marked as 1, on the contrary, low technical complexity areas marked as 0. It is a region with low technical complexity and is recorded as 0. The empirical results are shown in (Tables 6, 7).
Table 6 presents the grouped regression results of high-tech and low-tech complexity regions, with investor sentiment as the intermediary variable, where the models (1)–3) are samples of high-tech complexity regions and the models (4)–6) are samples of low-tech complexity regions. In models (1)–(3), the influence coefficient b1 of the explanatory variable air quality (AQ) on investor sentiment (Sent) of the intermediary variable was significantly positive. It means that air pollution can stir up investor sentiment. At this point, the explanatory variable air quality (AQ) and the intermediary variable investor sentiment (Sent) in column 3 are both significant for coefficients c1, c2 for the explained variable R&D investment, and b1c2 and c1 are the same number, indicating that investor sentiment still has an intermediary effect between air quality and R&D investment. However, in the models (4)–(6), the influence coefficient b1 of the explanatory variable air quality (AQ) on the investor sentiment (Sent) of the intermediary variable is significantly positive, but there is no longer an intermediary effect, indicating that investor sentiment, as the impact path of air quality and R&D investment of listed companies, needs the technical complexity of the region to reach a certain degree and comparing model 1) and model (4), air quality has stronger inhibition on R&D Investment of listed companies in low-tech complex areas. As cities become more technologically diverse, on the one hand, enterprises are more likely to have access to complementary technology support, providing a good environment and external support to undertake complex innovation activities. On the other hand, technological diversity can also enhance the possibility of technology integration, which is conducive to the formation of emerging industries, enhance the managers’ expectation of future earnings and the competitiveness of the industry, and thus can effectively encourage enterprises to increase R&D investment.
Table 7 shows the group regression results for high-tech and low-tech regions with government concerns as the intermediary variables, with the same model setting as Table 6. The coefficients c1 and c2 of the explanatory variable air quality (AQ) and the intermediary variable government concern (GS) in column 3 are significant, and the b1c2 and c1 are different, indicating that the government is concerned about the masking effect between air quality and R&D investment. However, in the models (4)–(6), the influence coefficient b1 of the explanatory variable air quality (AQ) on investor sentiment (Sent) of the intermediary variable is significantly positive, but c1 is not significant, so in low-tech complex areas, government concern cannot be the impact path of air pollution and R&D investment of listed companies. Comparing model 2) and model (5), the influence coefficient of air quality on government concern in high-tech complexity areas is 1.8849, and in low-tech complexity areas, it is 37.5347. Both are significant at a 1% level, but the difference is large. The coefficient in Table 5 is 17.4213, indicating that at present, the government is more sensitive to air pollution and more urgent to promote the improvement of regional innovation ability in the regions with low technological complexity. In this state, the government subsidy does not play a crowding-out role.
According to the nature of the actual controller, this paper defines the property right nature of listed companies (State) as the virtual variable. State takes 1 if it belongs to a state-owned enterprise and 0 if it belongs to a non-state-owned enterprise. Table 8 is a grouped regression result of the property rights nature with investor sentiment as the intermediary variable, in which the model (1)–3) is the sample of state-owned enterprises, and the model (4)–6) is the sample of non-state-owned enterprises. The estimation coefficient of AQI on investor sentiment in models 1)–3) is not significant, while the estimated coefficient of AQI in models 4)–6) is significantly positive at the level of 1%. The above results show that air pollution has an intermediary effect on the R&D investment of non-state-owned enterprises while not on the R&D investment of state-owned enterprises.
Enterprises with different property rights are confronted with different political environments. Non-state-owned enterprises face more stringent regulatory pressure than state-owned enterprises. At the same time, non-state-owned enterprises are likely to bear environmental responsibility from the government, and investor sentiment in non-state-owned polluting enterprises is notably higher when air pollution levels rise, in this case, the investors will have a “pessimistic expectation” of the expected return and effectiveness, leading to the reduction of their R&D investment.
Table 9 is a grouped regression result with the property right nature as the intermediary variables, in which the model (1)–3) is the sample of state-owned enterprises, and the model (4)–6) is the sample of non-state-owned enterprises. In column 3, the coefficient c2 of the intermediary variable government concern to the explained variable R&D investment is not significant, which indicates that there is no intermediary effect in the sample of state-owned enterprises, and the air quality is not significant to the government concern. However, in the models (4)–(6), the coefficient b1 of the impact of explanatory variable air quality on the investor sentiment of the intermediary variable is significantly positive, and the b1c2 and c1 are showing opposite signs, which indicates that there is a masking effect between the government concern on air quality and R&D investment.
In order to improve economic performance, local governments will intervene in the market competition by means of administrative monopoly to protect the state-owned holding companies. Local governments pay more attention to protecting the interests of state-owned enterprises and give more policy support to state-owned enterprises that are at a disadvantage in market competition. As a result, government concern is not on its own a path of influence for air pollution and R&D investment by listed companies, and it is not sensitive to air pollution for state-owned enterprises. As air pollution intensifies, state-owned polluters are protected even as the government sets more binding environmental policies, and their R&D investment is not significantly affected by air pollution. For the non-state-owned enterprises, government concern is a significant intermediary variable, and its crowding-out effect on R&D investment still exists.
The intermediary effect of “air quality-enterprise R&D investment” may be affected by the characteristics of the pollution degree of the enterprise itself. This article takes the “Notice on the Implementation of Special Emission Limits for Air Pollutants” issued by the Ministry of Environmental Protection as the standard. If the listed company is in the six major industries under the “Notice”, it will be defined as a heavy pollution enterprise, and the rest will be defined as light pollution enterprises. In this article, the pollution degree of an enterprise (Pollute) is defined as a virtual variable. If it is a heavily polluting enterprise, the Pollute value is 1; if it is a lightly polluting enterprise, the Pollute value is 0.
Table 10 presents the grouped regression results of heavy and light polluting enterprises with investor sentiment as the intermediary variable, in which the models (1)–3) are the samples of heavily polluting enterprises, and (4)–6) are the samples of light polluting enterprises. Both of them have a significant intermediary effect, and there is no significant difference between the two groups in the regression results of the intermediary variable of investor sentiment. However, in terms of the overall effect, compared with light polluters, heavy polluters’ R&D investment is more restrained by air quality. The above results show that air pollution has a more obvious impact on R&D investment of heavily polluting enterprises. Heavy polluting enterprises generally belong to high energy-consuming industries, most of which are local pillar industries. They should bear more responsibility for environmental protection, but they are prone to the phenomenon of ‘collusion between government and enterprises, which makes such enterprises pay less attention to environmental protection and do not implement the principle of ‘polluter pays.
Table 11 presents the grouped regression results of heavy and light polluting enterprises with government concern as the intermediary variable, in which the models (1)–3) are the samples of heavily polluting enterprises, and (4)–6) are the samples of light polluting enterprises. There is a significant intermediary effect in the sample of heavily polluting enterprises, while there is no intermediary effect in the sample of light polluting enterprises. Comparing models 2) and (5), heavy-polluting enterprises are more sensitive to air quality changes than light pollution enterprises. Most of the heavily polluting enterprises, as high energy consuming enterprises, bear more environmental protection responsibilities and receive more attention from local governments. Therefore, the increase in air pollution will attract more support and attention from relevant government departments, thus reducing the inhibitory effect of air on Enterprise R&D investment.
According to the degree of supervision of listed companies, whether listed companies are key regulatory enterprises (Control) is defined as a virtual variable. For key regulatory enterprises, the Control value is 1; for non-key regulatory enterprises, the Control value is 0. Table 12 is the grouped regression result of supervising enterprises with investor sentiment as the intermediary variable, where the models (1)–3) are the samples of key regulatory enterprises and (4)–6) are the samples of non-key regulatory enterprises. Both have a significant intermediary effect, and the intermediary effect of non-key regulatory enterprises is more significant than that of key regulatory enterprises. Table 13 shows the grouped regression results with government concern as the intermediary variable, and the model is set the same as the above. For key regulatory enterprises, government concern does not have a significant intermediary effect, but for non-key enterprises, government concern has a significant intermediary effect, which indicates that key regulatory measures for enterprises can offset the crowding-out effect of government subsidies.
Because there are many factors that affect enterprise R&D Investment, this paper may have the problem of missing variables. In addition, there may be a two-way causality between enterprise R&D investment and air quality, and enterprise R&D investment can improve air quality after achieving the expected benefits. Referring to the related research, this paper selects the two variables of rainfall and relative humidity as the instrumental variables of air pollution (Xie and Lin, 2020). The results of the instrumental variable method show that the relevant conclusions of this paper are still valid (As shown in Table 14).
In order to ensure the robustness of the results of this paper, the explained variables are replaced in this paper, and R&D personnel investment (CRP) is used to replace R&D capital investment (CRD). This paper also replaces the technical complexity of the control variable (large class) with the technical complexity of the small class (TCD0). At the same time, the investor sentiment (Sent) is replaced by the cumulative monthly return Sent0, which is 6 months behind the first period and does not consider the cash dividend. As shown in Table 15, the conclusions of this paper are still valid. Air pollution will damage health, cause brain drain, and weaken the company’s innovation investment. Relevant studies believe that the loss of human resources forces the improvement of management level and then improves the innovation level of enterprises. However, the results of this paper believe that the improvement of management level can not offset the weakening of the company’s innovation ability caused by the brain drain.
Based on the data of Shanghai and Shenzhen A-share listed companies from 2015 to 2019, this paper studies the impact path of air quality on R&D investment of listed companies from the perspectives of investor sentiment and government concern. The findings are as follows:
① In general, air quality has a significant inhibitory effect on R&D investment. Air quality has a significant role in promoting investor sentiment, which further inhibits the investment in the R&D of listed companies.
② Air pollution will cause effective government concern, and there is a masking effect between air quality and R&D investment. Furthermore, this paper draws the following conclusions from the perspectives of regional technical complexity, property rights, whether it is a polluting enterprise, and whether it is a key regulatory enterprise.
③ As the impact path of air quality and R&D investment of listed companies, investor sentiment needs the technical complexity of the region to cross a certain threshold before it can play a role. Moreover, air quality has a stronger inhibition on R&D investment of listed companies in low-tech complex areas; the government’s sensitivity to air pollution is higher in low-tech complexity areas where government concern cannot be an impact path for air pollution and R&D investment of listed companies.
④ Air pollution has an intermediary effect on R&D investment of non-state-owned enterprises but no on the R&D investment of state-owned enterprises; in the sample of state-owned enterprises, government concern has no intermediary effect, and air quality has no significant effect on government concern. But in non-state-owned enterprise samples, government concern has a masking effect between air quality and R&D investment.
⑤ In terms of the total effect, heavy polluting enterprises have a stronger inhibitory effect on enterprise R&D investment than light polluting enterprises, and air pollution has a more obvious effect on R&D investment of heavily polluting enterprises; with government concern as an intermediary variable, the sample of heavily polluting enterprises has a significant intermediary effect, but in the sample of light polluting enterprises, there is no intermediary effect. Heavy polluters are more sensitive to changes in air quality than light polluters.
⑥ With investor sentiment as the intermediary variable, there is a significant intermediary effect for both key and non-key regulatory enterprises, and the intermediary effect for the non-key regulated enterprises is more significant. For key regulatory enterprises, government concern does not have a significant intermediary effect, while for non-key enterprises, government concern has a significant intermediary effect, which indicates that key regulatory measures for enterprises can offset the crowding-out effect caused by government subsidies.
Based on the conclusions, this paper makes the following policy recommendations:
① The R&D investment of listed companies has a negative response to air quality, and for the government, it is necessary to implement different environmental protection policies for different enterprises, strengthen the management of state-owned enterprises and heavy polluting enterprises, and rectify them actively. For other enterprises, the government should stimulate the “shock potential”, so that the “polluter pays” concept will “deep into the enterprises”. We should rapidly improve the supply capacity of scientific and technological innovation and attach great importance to the system capacity and leading talents. For enterprises, it is necessary to speed up the formation of a good environment for personnel training and use, coordinate the internal relations of organizations, and accelerate the innovation of a perfect management mode.
② Government concern, that is, the government’s subsidy to enterprises, has a masking effect between air quality and R&D investment. On the one hand, the government should strengthen the mechanism of supervision and inspection, link the number of subsidies received by enterprises to their performance, restrict the use of subsidies, and enhance the innovation vitality and enthusiasm of enterprises. On the other hand, enterprises should seize the opportunity of the information technology revolution, take market demand as the fundamental driving force of scientific and technological innovation, attract global innovation resources, and expand development space.
③ Local governments should accelerate the formation of industrial technology and knowledge system, ensure the security of regional industrial chains and supply chains, and optimize the allocation of innovation factors in order to enhance the innovation vitality and innovation ability of enterprises and promote their convergence to enterprises.
④ We should rapidly improve the supply capacity of scientific and technological innovation and attach great importance to system capacity and leading talents. We should promote the transfer and transformation of scientific and technological achievements and stimulate the innovation and Entrepreneurship of scientific researchers in universities. Besides, We should focus on training and bringing up a number of strategic scientists, science and technology leading talents, and high-level innovation teams with strategic vision and able to grasp the world’s science and technology development trends.
The raw data supporting the conclusions of this article will be made available by the authors, without undue reservation.
JY and NQ: Analyzed and interpreted the Green Bond data regarding the financing cost. And analyzed and interpreted the data of Pro-Environmental Factors. DH: A major contributor in writing the manuscript and collecting the data. And Reviewing and editing the manuscript. All authors read and approved the final manuscript.
This work was supported by the National Social Science Fund of China (21BTJ050) and Jiangsu college Philosophy and social science research project (2021SJA2405).
We thank all the editors for permitting the use of the vineyards where this research was done.
The authors declare that the research was conducted in the absence of any commercial or financial relationships that could be construed as a potential conflict of interest.
All claims expressed in this article are solely those of the authors and do not necessarily represent those of their affiliated organizations, or those of the publisher, the editors and the reviewers. Any product that may be evaluated in this article, or claim that may be made by its manufacturer, is not guaranteed or endorsed by the publisher.
Baker, M., and Wurgler, J. (2004). A catering theory of dividends. J. Finance 59 (3), 1125–1165. doi:10.1111/j.1540-6261.2004.00658.x
Baron, R. M., and Kenny, D. A. (1986). The moderator-mediator variable distinction in social psychological research: Conceptual, strategic, and statistical considerations. J. Pers. Soc. Psychol. 51 (6), 1173–1182. doi:10.1037/0022-3514.51.6.1173
Brown, G. W., and Cliff, M. T. (2005). Investor sentiment and asset valuation. J. Bus. 78 (2), 405–440. doi:10.1086/427633
Buisseret, T. J., Cameron, H., and Georghiou, L. (1995). What difference does it make? Additionality in the public support of R&D in large firms. Int. J. Technol. Manag. 10 (4), 587–600. Available at: https://www.inderscienceonline.com/doi/abs/10.1504/IJTM.1995.025644. doi:10.1504/IJTM.1995.025644
Cui, L. B., Duan, H. B., Mo, J. L., and Song, M. L. (2021). Ecological compensation in air pollution governance: China's efforts, challenges, and potential solutions. Int. Rev. Financial Analysis 74, 101701–101711. doi:10.1016/j.irfa.2021.101701
Dang, T. V., and Xu, Z. X. (2018). Market sentiment and innovation activities. J. Financ. Quant. Anal. 53 (3), 1135–1161. doi:10.1017/S0022109018000078
De Pretto, L., Acreman, S., Ashfold, M. J., Mohankumar, S. K., and Campos-Arceiz, A. (2015). The link between knowledge, attitudes and practices in relation to atmospheric haze pollution in Peninsular Malaysia. PLoS One 10 (12), 1–18. doi:10.1371/journal.pone.0143655
Ding, X. Y., Guo, M. M., and Yang, T. (2021). Air pollution, local bias, and stock returns. Financ. Res. Lett. 39, 101576–6. doi:10.1016/j.frl.2020.101576
Dolan, P., and Laffan, K. (2016). Bad air days: The effects of air quality on different measures of subjective well-being. J. Benefit. Cost. Anal. 7, 147–195. doi:10.1017/bca.2016.7
Du, G. D., Shin, K. J., and Managi, S. (2018). Variability in impact of air pollution on subjective well-being. Atmos. Environ. X. 183, 175–208. doi:10.1016/j.atmosenv.2018.04.018
Du, M. Y., Hou, Y. F., Zhou, Q. J., and Ren, S. Y. (2022). Going green in China: How does digital finance affect environmental pollution? Mechanism discussion and empirical test. Environ. Sci. Pollut. Res. Int., 1–23. doi:10.1007/S11356-022-21909-0
Gao, W. H., and Zhang, J. Q. (2020). How does the weather affect individual behavior and business activities-Literature review on the influence mechanism of weather elements. Econ. Manag. 42 (1), 194–208. (in Chinese).
Gao, Y., Zhao, C. G., Sun, B. X., and Zhao, W. D. (2022). Effects of investor sentiment on stock volatility: New evidences from multi-source data in China's green stock markets. Financ. Innov. 8 (1), 77. doi:10.1186/S40854-022-00381-2
Han, X., Liu, Y. Q., Gao, H., Ma, J. M., Mao, X. X., Wang, Y. T., et al. (2017). Forecasting PM2.5 induced male lung cancer morbidity in China using satellite retrieved PM2.5 and spatial analysis. Sci. Total Environ. 607-608, 1009–1017. doi:10.1016/j.scitotenv.2017.07.061
Hansen, G. S., and Hill, C. W. L. (1991). Are institutional investors myopic? A time-series study of four technology ‐ driven industries. Strateg. Manag. J. 12 (1), 1–16. doi:10.1002/smj.4250120102
Hao, Y., Wu, Y. R., Wu, H. T., and Ren, S. Y. (2020). How do FDI and technical innovation affect environmental quality? Evidence from China. Environ. Sci. Pollut. Res. 27 (8), 7835–7850. doi:10.1007/s11356-019-07411-0
He, D., Yang, J. L., Wang, Z. M., and Li, W. C. (2020). Has the manufacturing policy helped to promote the logistics industry? Plos One 15 (7), 02352922–e235321. doi:10.1371/journal.pone.0235292
Hein, W., Wilson, C., Lee, B., Rajapaksa, D., de Moel, H., Athukorala, W., et al. (2019). Climate change and natural disasters: Government mitigation activities and public property demand response. Land use policy 82, 436–443. doi:10.1016/j.landusepol.2018.12.026
Hirshleifer, D., and Shumway, T. (2003). Good day sunshine: Stock returns and the weather. J. Finance 58 (3), 1009–1032. doi:10.1111/1540-6261.00556
Jacobsen, B., and Marquering, W. (2008). Is it the weather? J. Bank. Finance 32 (4), 526–540. doi:10.1016/j.jbankfin.2007.08.004
Jans, J., Johansson, P., and Nilsson, J. P. (2018). Economic status, air quality, and child health: Evidence from inversion episodes, air quality, and child health: Evidence from inversion episodes. J. Health Econ. 61, 220–232. doi:10.1016/j.jhealeco.2018.08.002
Jia, L. L., Nam, E., and Chun, D. (2021). Impact of Chinese government subsidies on enterprise innovation: Based on a three-dimensional perspective. Sustainability 13 (3), 1288–1322. doi:10.3390/su13031288
Li, X., Wang, Y. T., Zhou, H. C., and Shi, L. (2020). Has China's war on pollution reduced employment? Quasi-experimental evidence from the clean air action. J. Environ. Manage. 260, 109851–109910. doi:10.1016/j.jenvman.2019.109851
Lim, Y. H., Kim, H., Kim, J. H., Bae, S., Park, H. Y., and Hong, Y. C. (2012). Air pollution and symptoms of depression in elderly adults. Environ. Health Perspect. 120 (7), 1023–1028. doi:10.1289/ehp.1104100
Liu, G. X., Dong, X. C., Kong, Z. Y., and Dong, K. Y. (2021). Does national air quality monitoring reduce local air pollution? The case of PM2.5 for China. J. Environ. Manage. 296, 113232–113311. doi:10.1016/j.jenvman.2021.113232
Liu, X. X., Dong, X. Y., Li, S., Ding, Y. T., and Zhang, M. (2021). Air pollution and high human capital population migration: An empirical study based on 35 major cities in China. Sustain. Prod. Consum. 27, 643–652. doi:10.1016/j.spc.2021.01.032
Lu, Y. L., Wang, Y., Zuo, J., Jiang, H. Q., Huang, D. C., and Rameezdeen, R. (2018). Characteristics of public concern on haze in China and its relationship with air quality in urban areas. Sci. Total Environ. 637-638, 1597–1606. doi:10.1016/j.scitotenv.2018.04.382
Nofsinger, J. R. (2005). Social mood and financial economics. J. Behav. Financ. 6 (3), 144–160. doi:10.1207/s15427579jpfm0603_4
Peters, B., Roberts, M. J., and Vuong, V. A. (2022). Firm R&D investment and export market exposure. Res. Policy 51 (10), 104601. doi:10.1016/J.RESPOL.2022.104601
Polk, C., and Sapienza, P. (2009). The stock market and corporate investment: A test of catering theory. Rev. Financ. Stud. 22 (1), 187–217. doi:10.1093/rfs/hhn030
Power, M. C., Kioumourtzoglou, M. A., Hart, J. E., Okereke, O. I., Laden, F., and Weisskopf, M. G. (2015). The relation between past exposure to fine particulate air pollution and prevalent anxiety: Observational cohort study. BMJ 350, h1111. doi:10.1136/bmj.h1111
Ren, S. Y., Hao, Y., and Wu, H. T. (2022). Digitalization and environment governance: Does internet development reduce environmental pollution? J. Environ. Plan. Manag., 1–30. doi:10.1080/09640568.2022.2033959
Ren, S. Y., Hao, Y., and Wu, H. T. (2021). How does green investment affect environmental pollution? Evidence from China. Environ. Resour. Econ. 25, 1–27. doi:10.1007/S10640-021-00615-4
Sanduijav, C., Ferreira, S., Filipski, M., and Hashida, Y. (2021). Air pollution and happiness: Evidence from the coldest capital in the world. Ecol. Econ. 187, 107085–107120. doi:10.1016/j.ecolecon.2021.107085
Song, Y., Zhang, K. K., Hong, X. P., and Li, X. Y. (2021). A novel multi-objective mutation flower pollination algorithm for the optimization of industrial enterprise R&D investment allocation. Appl. Soft Comput. 109, 107530–107614. doi:10.1016/j.asoc.2021.107530
Tang, S. Y., Yan, Q. L., Shi, W., Wang, X., Sun, X. D., Yu, P. B., et al. (2018). Measuring the impact of air pollution on respiratory infection risk in China. Environ. Pollut. 232, 477–486. doi:10.1016/j.envpol.2017.09.071
Vega, G. C., Gross, A., and Birkved, M. (2021). The impacts of plastic products on air pollution-A simulation study for advanced life cycle inventories of plastics covering secondary microplastic production. Sustain. Prod. Consum. 28, 848–865. doi:10.1016/j.spc.2021.07.008
Wang, T., Peng, J. C., and Wu, L. (2021). Heterogeneous effects of environmental regulation on air pollution: Evidence from China's prefecture-level cities. Environ. Sci. Pollut. Res. 28, pages25782–25797. doi:10.1007/s11356-021-12434-7
Wang, Y. Y., Lu, T., and Qiao, Y. B. (2021). The effect of air pollution on corporate social responsibility performance in high energy-consumption industry: Evidence from Chinese listed companies. J. Clean. Prod. 280, 124345–124412. doi:10.1016/j.jclepro.2020.124345
Wang, Z. X., Qi, Z. Y., and Zhu, L. (2021). Latitudinal and longitudinal variations in the impact of air pollution on well-being in China. Environ. Impact Assess. Rev. 90, 106625–106710. doi:10.1016/j.eiar.2021.106625
Wu, Q. N., and Lu, J. (2020). Air pollution, individual investors, and stock pricing in China. Int. Rev. Econ. Finance 67, 267–287. doi:10.1016/j.iref.2020.02.001
Wu, Q. Q., Hao, Y., and Lu, J. (2018). Air pollution, stock returns, and trading activities in China. Pacific-Basin Finance J. 51, 342–365. doi:10.1016/j.pacfin.2018.08.018
Xiang, D., Zhao, T. Z., and Zhang, N. (2021). Does public subsidy promote sustainable innovation? The case of Chinese high-tech SMEs. Environ. Sci. Pollut. Res. 28, 53493–53506. doi:10.1007/s11356-021-14555-5
Xie, J., and Lin, X. C. (2020). Study on the impact of air pollution on the investment behavior of polluting enterprises-from the perspective of "pessimistic expectation. Econ. Rev. 05, 124–136.
Zhang, N., Zhao, K. K., and Yu, Y. T. (2020). The effect of environmental regulation on air pollution, productivity, and factor structure: A quasi-natural experiment evidence from China. Environ. Sci. Pollut. Res. 27, 20392–20409. doi:10.1007/s11356-020-08462-4
Keywords: investment, financial enterprise, air quality, enterprise R&D investment, investor sentiment
Citation: Yang J, He D and Qureshi NA (2022) Assessing the impact path of air quality on enterprise R&D investment: Empirical evidence from dual perspectives of investor sentiment and government concern. Front. Environ. Sci. 10:1008986. doi: 10.3389/fenvs.2022.1008986
Received: 01 August 2022; Accepted: 14 September 2022;
Published: 02 November 2022.
Edited by:
Faik Bilgili, Erciyes University, TurkeyCopyright © 2022 Yang, He and Qureshi. This is an open-access article distributed under the terms of the Creative Commons Attribution License (CC BY). The use, distribution or reproduction in other forums is permitted, provided the original author(s) and the copyright owner(s) are credited and that the original publication in this journal is cited, in accordance with accepted academic practice. No use, distribution or reproduction is permitted which does not comply with these terms.
*Correspondence: Dan He, aGVkYW5AdWpzLmVkdS5jbg==; Naveed Akhtar Qureshi, bmF2ZWVkQGliYS1zdWsuZWR1LnBr,
Disclaimer: All claims expressed in this article are solely those of the authors and do not necessarily represent those of their affiliated organizations, or those of the publisher, the editors and the reviewers. Any product that may be evaluated in this article or claim that may be made by its manufacturer is not guaranteed or endorsed by the publisher.
Research integrity at Frontiers
Learn more about the work of our research integrity team to safeguard the quality of each article we publish.