- 1School of Law and Business, Sanjiang University, Nanjing, China
- 2School of Management Science and Engineering, Nanjing University of Information Science & Technology, Nanjing, China
- 3School of Economics and Management, Nanjing University of Science & Technology, Nanjing, China
Agriculture has always been the focus of all countries, the rapid development of agriculture is inseparable from the strong support of finance. As a new financial model, agricultural product supply chain finance has attracted much attention. Through the analysis of the agricultural product supply chain finance model, we find that the core problem of agricultural supply chain finance is the management and control of supply chain financial risks. Based on the identification of financial risk categories of agricultural products supply chain, this paper constructs tan evaluation index system. Taking Jiangsu Province of China as the research object, this research collects relevant data of financial institutions, logistics enterprises, agricultural product production, and processing enterprises and farmers in southern and northern Jiangsu Province in 2021. The structural equation is used to establish the model. This statistical method can handle multiple potential variables at the same time, and allows observation variables and potential variables to contain measurement errors. The application of statistical methods that can handle multiple potential variables at the same time and allow observation variables and potential variables to contain measurement errors to build a model. By using the Amos calculation model, this research found that the factors affecting the financial risks of the agricultural product supply chain in Jiangsu Province from high to low are: the agricultural product pledge risks, the credit risks, the supply chain operation risks, the technical risks, the legal and policy risk. We hope this research can play a theoretical guiding role for the effective development of agricultural product supply chain finance business.
1 Introduction
Agricultural issues have always been the focus of every country. A large number of practices have shown that the development of agriculture is inseparable from the strong support of financial services. Therefore, agricultural supply chain finance has become a hot topic in recent years. Supply chain finance is a financial service model in which banks and other financial institutions manage the capital flow, logistics and information flow of upstream and downstream enterprises or individuals in the supply chain around core enterprises and transform the uncontrollable risks of a single enterprise into the controllable risks of the whole supply chain. The early recognized agricultural products supply chain financial practices, such as financial services carried out by Heilongjiang Longjiang bank in 2006, issued 90.475 billion yuan of agricultural-related loans by the end of 2018. In 2012, the People’s Bank of China carried out the supply chain finance business of agricultural product orders in Shouguang City, Shandong Province, and granted more than 900 million yuan to more than 30 new agricultural business entities and more than 3,600 farmers. In 2018, Zhongnong cooperated with 28 financial institutions to provide financial services to customers totaling 10.98 billion yuan. Generally speaking, although the practice of agricultural supply chain finance in China started late, it has developed rapidly and formed a certain scale (Huang et al., 2021; Huang and Liu, 2021).
Globally, the supply chain finance business has also developed rapidly. According to the data of Demica, a provider of supply chain electronic finance platforms since 2000, the average annual growth rate of supply chain finance has shown double-digit growth in both developed and developing countries. The rapid development of supply chain finance business benefits from two aspects: First, the government, chambers of Commerce, and industry associations have issued many relevant rules and guidelines, such as the unified rules on bank payment liability, FCI factoring general rules, etc. Second, the huge demand for foreign and domestic trade markets and the continuous progress of information technology have also promoted the rapid development of supply chain finance (Deng-Kui Sii et al., 2021; Zhou et al., 2022).
However, in the rapid development of supply chain finance, there are still many problems. For example, under the core enterprise-led mode, the core enterprises do not cooperate with the strict requirements of the bank very well. At the same time, it will also produce a dependent monopoly, which will promote the risk. The direct consequence is that small and medium-sized financing enterprises or farmers continue to have bad behaviors, such as constructing false warehouse receipts, repeated pledges, and cash arbitrage in the financing process. All these have enhanced the financial risks of the supply chain. Therefore, the difficulty and high cost of financing in agricultural production and circulation have not been effectively solved. The study of agricultural supply chain finance is extremely urgent (Zhang et al., 2022).
Therefore, in this paper, based on the identification of the financial risk categories of the agricultural product supply chain, we constructed the financial risk evaluation index system of the agricultural product supply chain. This research takes Jiangsu Province, China, as the research object and collects relevant data on financial institutions, logistics enterprises, agricultural product production, and processing enterprises and farmers in southern and northern Jiangsu in 2021. Applying the statistical method that can handle multiple potential variables at the same time and allow observation variables and potential variables to contain measurement errors to establish the model, and using Amos calculation model, the study found that the factors that affect the financial risk of agricultural product supply chain in Jiangsu Province from high to low are: agricultural product quality pledge risk, credit risk, supply chain operation risk, technical risk, legal and policy risk. We hope this research can play a theoretical role in guiding the effective development of agricultural product supply chain finance business.
2 Literature review
Since the “Macmillan gap” was proposed in 1931 (Scott and Newton, 2007), problems such as the difficult and expensive financing of small, medium-sized, and micro-enterprises have attracted extensive attention in the production and circulation of agricultural products. In the late 1990s, supply chain finance gradually became an important way to solve financing difficulties and research hotspots. The research results mainly include three areas. The first area is about the supply chain financial model; for example, JiangSu He and other scholars classify the supply chain financial model according to different standards, analyze the existing problems and deficiencies, and innovatively put forward the idea of building a visual platform, to improve the supply chain financial model and reduce the risk and cost in the implementation process (He and Tang, 2012). Zhang and other scholars take the iron and steel industry as an example to analyze the supply chain financial model from three types of customers and domestic and foreign businesses (Zhang et al., 2015). Yao Y and other scholars demonstrated the problems in the financing process of small, medium-sized, and micro-enterprises and proposed three basic modes (Yao and Liu, 2018). Deng L, Wang, and other scholars discussed the financing model with the acquirer as the core when farmers take fixed assets as collateral (Deng et al., 2022). Xu J W and other scholars analyzed the characteristics of the supply chain financing mode (Xu, 2016). The second area is about the supply chain financial risk elements. Yuan Zhang and other scholars analyzed the financial risk factors of the agricultural products supply chain (Wang and Sun, 2016; Ding et al., 2017). Wang R and other scholars use the principal-agent theory to explain the risk of electronic order pledges in online agricultural product supply chain finance (Yang et al., 2019). Vovchak O D expounded on the types of supply chain financial risks of agricultural products based on the symbiosis theory (Vovchak et al., 2018). Mou W M analyzed the credit risk in the financial process of the agricultural products supply chain (Mou et al., 2018). Li Z and other scholars take the accounts receivable factoring business as the research object and use the evolutionary game theory method to analyze the factors of the supply chain financial risk (Li et al., 2018; Bai et al., 2022; Gao, 2022). The third area is about supply chain financial risk evaluation and strategy. For example, Xuan F and other scholars applied the fuzzy preference relationship method to evaluate the financial credit risk of the supply chain (Xuan, 2021). Yu Z and other scholars applied evolutionary game theory to study the financial system of the green agricultural products supply chain under the background of covid-19 and the urban-rural income gap (Yu and Khan, 2021). Van Bergen M and other scholars believe that the products, information, and capital flow in the agricultural products supply chain are interrelated and put forward the optimization strategy from the procurement perspective (Van Bergen et al., 2019). Li X L and other scholars analyzed the role of agricultural supply chain finance on Farmers’ credit constraints, and put forward the optimization strategy from the perspective of farmers (Li and Sun, 2022).
Combing through the literature, it was found that the research results are mainly carried out from the perspectives of the supply chain financial model, supply chain financial risk elements, supply chain risk evaluation, etc. The research results provide a good idea for exploring agricultural products’ supply chain financial problems. Still, most of the existing research results are isolated, and the process is not clear enough.
Given the above analysis, combined with the current agricultural products supply chain finance situation in Jiangsu Province, China, this study applies the structural equation model (Wang et al., 2010; Hasman, 2015; Asparouhov et al., 2018; Lefcheck, 2016) (SEM) to construct the financial risk evaluation model (this quantitative research method has been widely used in psychology, education, society, and other fields). It uses the Amos method to calculate the model (Ali et al., 2019; Wan, 2021) and defines the level of factors affecting the agricultural products’ supply chain financial risk.
The novelty of this paper mainly includes two aspects: first, it analyzes the management and control of financial risk in agricultural product supply chain from a systematic perspective, while the latest research results are often carried out from a single perspective of agricultural product supply chain financial model or agricultural product supply chain financial risk factors or agricultural product supply chain financial risk evaluation; Secondly, taking Jiangsu Province as an example, the structural equation model that can handle multiple potential variables and allow observation variables and potential variables to contain measurement errors is used to evaluate the financial risk of the agricultural product supply chain. However, the existing research results on the evaluation methods of financial risk of agricultural product supply chain are more complex.
3 Materials and methods
In order to clarify the key points of financial risk management and control, it is necessary to identify the types of agricultural product supply chain risks, build an evaluation index system based on this, and then select appropriate evaluation methods for evaluation.
3.1 Risk identification
According to our research, regardless of agricultural product order financing, agricultural product inventory pledge financing, and agricultural product factoring financing mode, agricultural product supply chain financial risk is affected by many factors, including relevant laws and policies, enterprise credit, supply chain operation status, agricultural product pledge characteristics, and related technologies, thus forming different risks.
3.1.1 Legal and policy risk
From a legal point of view, the current laws on agricultural supply chain finance mainly include the Guarantee Law and the Contract Law. Compared with the complex financial process of the agricultural product supply chain, these laws are difficult to control the risks effectively (Liu and Destech Publicat, 2018). From a policy point of view, the agricultural supply chain finance policies are not yet perfect. There are many subjects involved in agricultural product supply chain finance, so it is difficult to formulate and implement policies to determine the responsibilities and powers of many subjects. For example, due to imperfect laws and regulations in the financial operation of agricultural product supply chains, the ownership of pledged property is not clearly defined, warehouse receipts are forged, and the risks of non-standard business operations often occur.
3.1.2 Credit risk
Credit risk, also known as default, refers to loss caused by the borrower’s default or credit rating decline. Compared with other types of financial risks, agricultural product supply chain finance involves many scattered subjects, including large-scale production enterprises, logistics enterprises, cooperative organizations, and many small-scale farmers. At the same, the core enterprise in the agricultural product supply chain plays a role of supervision, joint, and several liabilities for farmers’ financing. At present, the management ability of the core enterprises in the agricultural product supply chain is not strong, so the credit risk of the agricultural product supply chain finance is relatively high (Shee et al., 2019). Credit risk can be measured by indicators such as the financial status of the financing entity, solvency, enterprise characteristics (such as enterprise size and ownership nature), and profitability.
3.1.3 Supply chain operational risk
The supply chain operational risk mainly includes the control risk of the core enterprise in the supply chain, the collaboration risk between the supply chain enterprises, and the supply chain competitiveness risk. Among them, the stronger the control power of the core enterprises, the smaller the risk. On the contrary, the weaker the control power of the core enterprises, the greater the risk. Thus, smoother cooperation and communication between supply chain enterprises. The lower the risk, the higher the risk caused by poor collaboration, less communication, and poor information among supply chain enterprises. Supply chain competitiveness is inversely proportional to risk; the stronger the supply chain competitiveness, the smaller the risk. The weaker the supply chain competitiveness, the greater the risk (Huang, 2015; Xu, 2016; He et al., 2019).
3.1.4 Pledge risk
The agricultural product pledge risk refers to the risk caused by deterioration, depreciation, improper selection, and poor sales when agricultural products are used as collateral. Agricultural products are more prone to deterioration and depreciation than other collaterals, so the resulting risks need special attention. Such risks can be decomposed into agricultural product type risks, agricultural product price fluctuation risks, and agricultural product deterioration risks. Banks should be clear and specific regarding the conditions and standards for agricultural products as pledges. They should refuse to pledge agricultural products subject to deterioration, high storage conditions, and difficult-to-control transportation costs or pledges that do not meet the standards. When the value of the pledged property depreciates, the Bank shall promptly request the customer to supplement the value of the pledged property to avoid the risk of depreciation effectively.
3.1.5 Technical risk
Technical risk refers to the risk caused by a low or insufficient technical level. When agricultural products are in circulation or used as collateral, a series of control risks will arise due to low or insufficient technology for value assessment, storage, dynamic price monitoring, warehouse receipt authenticity identification, and credit identification (Kulinska and Giera, 2019).
In addition to the above-mentioned main risks, the authors believe that the macroeconomic environment will also affect agricultural supply chain finance risks. Given the current stable and improving economic environment in Jiangsu Province, China, it provides more favourable conditions for developing and operating small and medium-sized agricultural production and distribution enterprises than in other regions. Therefore, the risks that are caused by the macroeconomic environment are not considered here.
3.2 Indicator system
According to the agricultural product supply chain financial model and the types of agricultural product supply chain financial risks, the constructed evaluation index system is shown in Table 1. In the indicator system in Table 1, X2 and X4 are used to represent the internal derivative potential variables, and Y1, Y3, and Y5 are used to represent the external derivative potential variables; X2 observation variables are represented by X21, X22, X23, and X24; X4 observation variables are represented by X41, X42, and X43; Y1 observation variable is represented by Y11, Y12, and Y13; The observed variables of Y3 are represented by Y31, Y32, and Y33; The observed variables of Y5 are represented by Y51 and Y52. The meanings of the internal derivative potential variable, the external derivative potential variable, and the observation variable are further elaborated in the model construction.
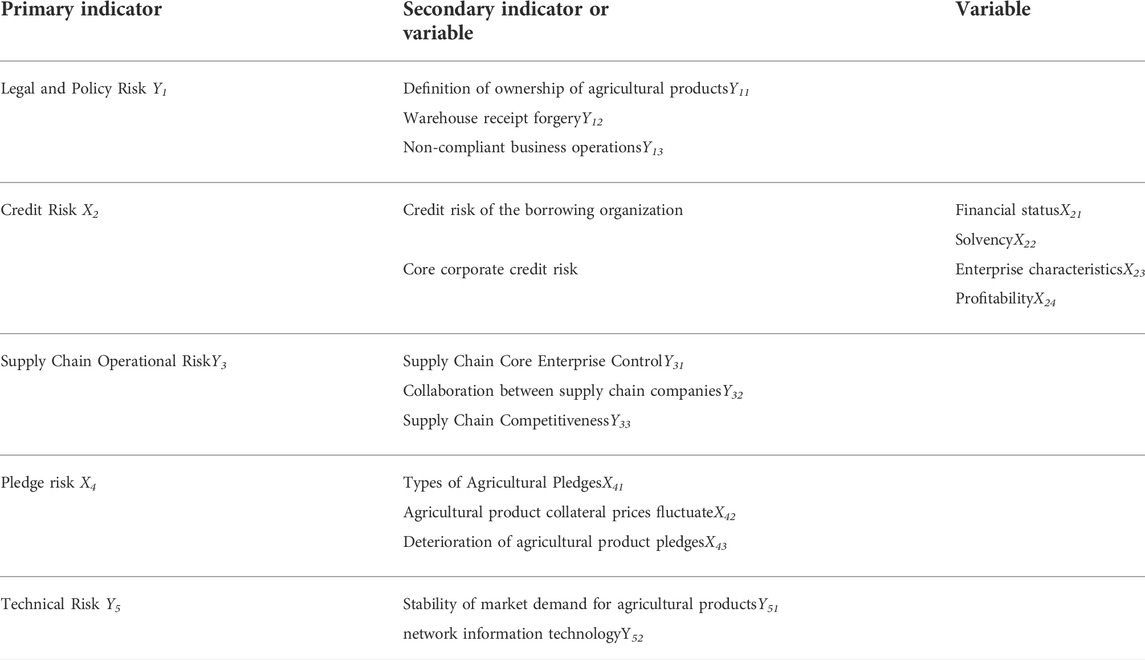
TABLE 1. Financial Risk Evaluation Index System of Agricultural Product Supply Chain (Source: sorted by the authors according to the type of risk).
To ensure the stability, representative, and consistency of the evaluation indicators, the reliability of the indicators needs to be tested after the supply chain financial risk indicator system is constructed.
3.3 Evaluation method
The evaluation method is also crucial to ensure the validity of the evaluation results and the requirements for the sample size and data itself. According to the existing literature, the main methods of supply chain financial risk evaluation are the Regression analysis method (Zhong and Su, 2016; Yang et al., 2019; Xuan, 2021), Factor analysis (Li and Destech Publicat, 2016), Structural equation modelling, etc. (Avelar-Sosa et al., 2018; Huo et al., 2018). Regression analysis is an analysis method that processes a large amount of statistical data, finds the relationship between the dependent variable and the independent variable, establishes a regression equation, and predicts the development trend of the dependent variable. The premise of regression analysis is that there is no error in the independent variable, and it is generally applicable to evaluating a single indicator or exploring correlation. Factor analysis is a statistical method for extracting common factors from multiple variables. This method is suitable for problems where there is no obvious correlation between indicators and the indicators can be directly observed. Structural equation modelling can comprehensively examine the relationship between complex multi-dimensional variables at the same time. Structural equation models include latent variables and observed variables. Generally speaking, latent variables are difficult to measure accurately, and observed variables can be measured directly. According to the different roles in the structural relationship, latent variables are divided into internal latent variables and external latent variables. The method can study the causal relationship between measurable and latent variables and apply the causal relationship between them.
The model consists of a measurement model and a structural model. The measurement model reflects the relationship between indicators and latent variables, whereas the structural model reflects the relationship between latent variables. Analysis software includes LISREL, Amos, EQS, Mplus, etc. Among them, Amos is a powerful and widely used analysis tool. This method can analyze multiple variables simultaneously and test whether the data conforms to the established model through the goodness of fit. Given the following reasons, first, the agricultural product supply chain financial risk evaluation is a comprehensive evaluation, not a single index evaluation; Second, the agricultural product supply chain financial risks evaluation needs to set latent variables for evaluation indicators; Third, there is a significant correlation between the evaluation indicators of agricultural product supply chain financial risk; Fourth, there are many and complex factors affecting the agricultural supply chain financial risk. It is necessary to examine the relationship between different index variables, between the index and latent variables, and between latent variables and latent variables. Therefore, the commonly used evaluation methods, such as regression and factor analysis, have certain limitations. Comprehensively considered, the structural equation model is an ideal supply chain financial risk assessment method.
3.4 Model assumptions and model construction
3.4.1 Model assumptions
The assumptions for applying the structural equation model are: E(ζ) = 0, E(ɛ) = 0, E(δ) = 0, E(X) = 0, E(Y) = 0; ζ、ɛ and δ Mutual independence; X and ɛ Mutual independence; Y and δ Independent of each other.
3.4.2 Model construction
To apply the SEM method, three types of variables need to be set: latent variables, observation variables, and error variables. Due to the different functions of latent variables, they are divided into internal derivative potential and external derivative potential variables. Internal derivative potential variablesrefer to the variablesaffected by other variables, which are the results of the causal relationship, that is, dependent variables; External derivative potential variablesrefer to potential variablesthat are not affected by other variables. But it will affect other variables. They are independent variables as causes in causality. According to the connotation of financial risk indicators of agricultural products supply chain, credit risk and agricultural products pledge risk are affected by laws and policies related to agricultural products supply chain finance, supply chain operation, and technical level. So the internal latent variables are: credit risk X2 and agricultural product pledge risk X4; the external latent variables are: legal and policy risk Y1, supply chain operation risk Y3, and technical risk Y5. The corresponding measures each latent variable as an observed variable, in which the internal latent variable X2 is measured by the corresponding observed variable financial status X21, solvency X22, enterprise characteristics X23, and profitability X24. The internal latent variable X4 is measured by the corresponding observation variables X41 agricultural product collateral type risk, X42 agricultural product collateral price fluctuation risk, and X43 agricultural product collateral deterioration risk. The external latent variable legal and policy risk Y1 is measured by the corresponding observation variables Y11 agricultural product ownership definition risk, Y12 warehouse receipt forgery risk, and Y13 business operation non-compliance risk. Supply chain operation risk Y3 is measured by observation variable Y31, supply chain core enterprise control risk, supply chain enterprise collaboration risk Y32, and supply chain competitiveness risk Y33. Y5 is measured by the corresponding observed variables Y51Stability of market demand for agricultural products and Y52 Network information technology.
The constructed structural equation model is as follows:
In the structural equation expression, η and n are the structural equation expressions, γ and β are the representative coefficient matrices, which are the correlation between internal latent variables and the correlation between external variables, respectively. ζ Represents the staggered term, reflecting the unexplained part of the equation. X represents the internal latent variable, Y represents the external latent variable, and m and n represent Y and X’s observed variable matrix. Am is the correlation between the variable matrix and the observed variable X, and An represents the correlation between the variable matrix and the observed variable Y. ɛ and δ are the errors existing in the observed variable matrices m and n. ζ represents an unobservable random item. As shown in Figure 1 (Ali et al., 2020; Sharma et al., 2020; Ahmadini et al., 2021; Modilbbo et al., 2022):
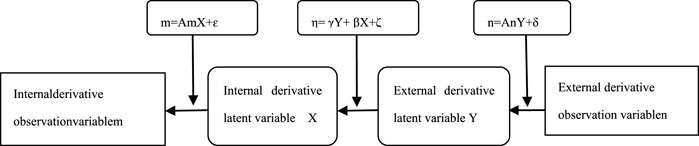
FIGURE 1. Structural equation model diagram (Figure source: by authors according to the connotation of SME).
4 Results and discussion
4.1 Data sources
Jiangsu Province is a strong economic province and a major agricultural province. Under the guidance of the relevant national policies to benefit farmers, the development of agriculture and rural areas has been continuously optimized, and the total output value of agriculture, forestry, animal husbandry, and fishery has been at the forefront of the country for many years. Judging from the output of grain, cotton, oil seeds, pork, beef, mutton and poultry, poultry eggs, and aquatic products, they are all in a growing trend. The advantages of agricultural production in Jiangsu Province are due to two factors: First, the Jiangsu provincial government has continuously increased investment in agriculture, so various types of agricultural production, processing, and logistics enterprises have developed rapidly, thus effectively guaranteeing agricultural products in Jiangsu Province. It also plays an important role in increasing farmers’ income, alleviating employment pressure in rural areas, and prospering the market. Second, the active development of various financial businesses in Jiangsu Province, especially the issuance of financial loans for agricultural products in southern Jiangsu, is increasing. Influenced by factors such as information technology, agricultural production enterprises, etc., farmers in Jiangsu Province still have difficulties in financing, such as a large gap in capital demand, high capital use costs, and few financing channels.
This study adopted a combination of questionnaires and field visits to obtain relevant information on agricultural product supply chain finance in Jiangsu Province. The design process of the questionnaire content invited government staff, business managers, scholars, and bank staff to participate. The survey objects focus on Jiangsu Province, mainly covering financial institutions, logistics enterprises, farmers, and enterprises producing and processing agricultural products in the southern and northern Jiangsu regions. In order to obtain the stability of SEM analysis, 200 research objects were selected, including eight financial institutions, all of which have carried out or are carrying out agricultural product supply chain finance business, 13 logistics enterprises, seven related agricultural enterprises, 172 farmers, and a total of 200 questionnaires were distributed, including 30 questionnaires distributed on-site, 170 questionnaires distributed by questionnaire stars, and 196 were recovered, with a recovery rate of 98%. The research group collated and analyzed the questionnaire data collected. It was found that six questionnaires were incomplete and three questionnaires answered contradictory questions, so 187 valid questionnaires and the geographical distribution of the survey objects are shown in Table 2:

TABLE 2. Distribution of the number of survey objects (Source: collated by authors according to the research process).
4.2 Discussion of results
After the model was built, according to the relevant index data of the agricultural product supply chain’s financial risk, Amos was used to calculate the model. Ideal operating results are obtained through the process of optimizing data, multiple verifications, and debugging, as shown in Table 3:
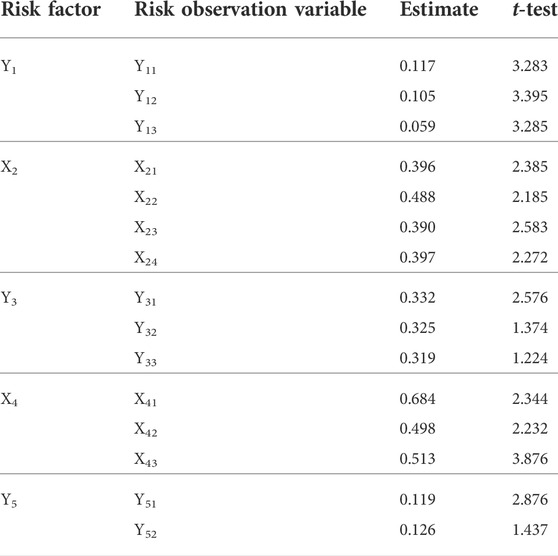
TABLE 3. Parameter estimates and reliability of model operation results (Source: calculated according to Amos software).
To put forward countermeasures and suggestions for the financial risk control of the agricultural product supply chain in Jiangsu Province, it is essential to judge the reliability and stability of the results of the model operation. Therefore, this study carried out multi-angle tests on the calculation results, as shown in Table 4:
Judging from the test results, the 15 indicators are acceptable, so it can be judged that the operation results of the indicators in the model have good reliability and stability. The evaluation results can be a theoretical basis for countermeasures and suggestions. According to the fitting test results, all latent variables have reached the standard of the significant test. Among all observed variables, the risk of agricultural product collateral type has the greatest impact on the financial business risk of the agricultural product supply chain in Jiangsu Province, with a coefficient of 0.684, the risk of deterioration, with a coefficient of 0.513, and finally the risk of changes in the price of agricultural collaterals, with a coefficient of 0.498. Therefore, when controlling the financial risk of the agricultural product supply chain in Jiangsu Province, the focus should be on the types of risks in the agricultural product pledge risk. Of course, the price fluctuation and deterioration of agricultural products in the pledge process are also key points of risk control. The second is credit risk, in which the financial status of the borrowing organization is the most important risk factor in credit risk, followed by the solvency of the borrowing company’s historical loans; it should be noted that the impact of core companies on credit risk is also higher, for example, the profitability coefficient is 0.397, so the risk control of core enterprises should also be paid attention to.
Supply chain operation risk is second only to pledged agricultural products and credit risk. Among the three indicators, the estimated value of supply chain control risk is 0.332 because the smooth progress of agricultural supply chain financial business depends on the guarantee and coordination of core enterprises. Therefore, the ability of core enterprises to coordinate and control the supply chain of agricultural products will inevitably affect the smooth development of related businesses. Technical risk is behind supply chain operation risk. Compared with collateral risk, credit risk, and supply chain operation risk, technical risk has a relatively small impact on the overall business risk. The reason lies in the continuous improvement of new technologies in recent years and the strong support of Jiangsu Province for agricultural business information technology. Therefore, the relevant technologies required in the business development process can be met, so technical risks are important to Jiangsu Province’s agricultural product supply chain finance. The business risk impact is small. The least influential factors are legal and policy risks, with risk coefficients of 0.117, 0.105, and 0.059.
5 Implications and conclusion
In recent years, under the guidance of China’s various preferential policies for agriculture, the agricultural supply chain financial business has been carried out effectively. However, compared with the traditional supply chain financial model, the agricultural supply chain financial model has the characteristics of many participants and complex business processes. According to the financial risk evaluation results of the agricultural products supply chain, the focus of financial risk management and control of the agricultural products supply chain lies in the pledge risk and credit risk of agricultural products. Therefore, the study believes that the following aspects can be used to control the financial risk of the agricultural supply chain and put forward optimization suggestions (Yang et al., 2019; Wu et al., 2021).
5.1 Risk prevention of agricultural products pledge
Supervising agricultural product pledges are the key to financial risk control in the agricultural product supply chain. Agricultural product pledges = guarantee = the source of funds for financial institutions and the driving force for financing enterprises to perform their contracts (Liu and Destech Publicat, 2018). Risks are mainly affected by factors such as agricultural product pledges, changes in the market price, and the deterioration of agricultural product pledges. The risk control of the types of agricultural pledges can be carried out from the perspective of whether it is easy to realize and sell; The risk caused by the change of the market price of agricultural products collateral can be carried out from two aspects. First, we need to pay close attention to the price elasticity of the collateral. Generally speaking, the smaller the price elasticity of the collateral, the more suitable it is to be used as agricultural product collateral. We need to grasp the trend and life cycle of the variety of the collateral, timely replace and update the collateral, and make a scientific prediction of the market demand of the collateral through big data and other technologies; Second, to prevent the risk of price fluctuations, farmers and small and medium-sized enterprises buying the corresponding insurance is an ideal means. In addition, core enterprises cooperate with futures companies to provide risk protection for the price fluctuations of agricultural products through hedging operations. For the risks caused by the deterioration of agricultural product pledges, attention should be paid to controlling the pledge’s entire process, paying attention to the form of the pledge in real-time during the shelf life, and trying to avoid selecting pledges with high regulatory conditions.
5.2 Strictly screen individuals and organizations in the agricultural product supply chain
The most basic and effective way to avoid financial risks in agricultural product supply chains is to check the access; individuals and organizations that do not qualify for loan applications are excluded. Determining whether individuals and organizations meet the loan standards requires banks to carry out based on customer credit ratings. To ensure the authenticity and effectiveness of credit ratings, banks can cooperate with professional rating agencies. Banks should strictly control loans to individuals and enterprises whose credit ratings do not meet the standards. At the same time, the core enterprises in the supply chain also need to strictly screen farmers and other node organizations. The strict selection of node farmers and organizations will reduce the income in the short term. Still, in the long run, it will provide double benefits for agricultural supply chain finance operations. Therefore, a good access system can effectively control the financial risks in the agricultural product supply chain.
5.3 Establish an effective early warning mechanism for financial risk in the agricultural supply chain
The agricultural product supply chain of any model will face a changeable and complex macro and micro-environment, and emergencies will inevitably arise. The risks brought by emergencies will quickly spread to all individuals and enterprises. The loss will be formed if the risk is dealt with and rescued after the occurrence. Therefore, it is particularly meaningful to identify the types of risks in advance, estimate the risks, quantify the losses caused by risks, and establish an effective risk early warning mechanism through technologies such as big data, the Internet of Things, and blockchain. When the risk occurs, the financial risk of the agricultural supply chain can be minimized.
5.4 Prevention of credit risk
According to the above analysis, credit risk has a high impact on agricultural supply chain finance risk. In risk control, we should focus on the solvency, profitability, financial status, and enterprise characteristics of financing customers. Whether the financial condition is good or not and whether the financial system is sound are important characteristics that reflect the solvency of enterprises. Enterprises with good financial conditions and sound financial systems show high profitability. In addition, the credit risk of core enterprises in the supply chain is mainly reflected in the comprehensive management ability. Externally, the core enterprises of the supply chain should improve the logistics environment, optimize the information platform and other contents, be able to provide professional services, and pay attention to the ability of resource integration and scheme optimization; Secondly, internally, logistics, information flow, capital flow, and business flow should be integrated. Professional personnel is also essential in business development, so we need to pay attention to the training of relevant talents.
5.5 Constantly improve relevant laws, regulations, and systems
The financial model of the agricultural products supply chain has a long cycle and complex process and is doomed to face many problems in the development process. Continuous improvement of relevant laws, regulations, and systems is a long-term and systematic process. No laws, regulations, or systems apply to all situations. From the national to the local level, we should pay attention to improving laws, regulations, and systems of agricultural products supply chain finance. Therefore, the government, cooperative organizations, and enterprises should all participate in the formulation and revision of relevant laws and regulations. It is necessary to make timely amendments and improvements to new problems, new models, and new technologies in the process of business development.
This study summarizes the risks in developing agricultural product supply chain finance using the structural equation model that can comprehensively test the relationship between complex multi-dimensional variables to evaluate the financial risks of agricultural product supply chains in Jiangsu Province and proposes countermeasures based on the evaluation results. In the research on the management and control of financial risks in the supply chain of agricultural products, there are both qualitative and quantitative evaluations. The evaluation index system is relatively comprehensive, and the evaluation method is applicable. However, there are still some imperfections in the process of research. For example, in constructing the evaluation index system, although the first-level indicators for financial risk evaluation of agricultural product supply chains have been constructed from many aspects, in the process of decomposing the first-level indicators, the constructed secondary indicators or variable settings are not comprehensive and specific. For example, the technical risk of the primary indicator is only measured by the agricultural scale level and network information technology (Ahmadini et al., 2021). It is hoped that in future research, the evaluation index system of the agricultural product supply chain can be refined, and the key points of financial risk management and control of the agricultural product supply chain can be refined.
Data availability statement
The original contributions presented in the study are included in the article/supplementary material, further inquiries can be directed to the corresponding authors.
Ethics statement
Ethical review and approval was not required for the study on human participants in accordance with the local legislation and institutional requirements. Written informed consent from the participants was not required to participate in this study in accordance with the national legislation and the institutional requirements.
Author contributions
Conceptualization, JY and DT; methodology, JY; software, JY; validation, DT, VB and HK; formal analysis, JY; investigation, JY; resources, JY; data curation, JY; writing—original draft preparation, JY; writing—review and editing, JY, DT, HK and VB; visualization, DT, VB, HK; supervision, DT; project administration, DT All authors have read and agreed to the published version of the manuscript.
Conflict of interest
The authors declare that the research was conducted in the absence of any commercial or financial relationships that could be construed as a potential conflict of interest.
Publisher’s note
All claims expressed in this article are solely those of the authors and do not necessarily represent those of their affiliated organizations, or those of the publisher, the editors and the reviewers. Any product that may be evaluated in this article, or claim that may be made by its manufacturer, is not guaranteed or endorsed by the publisher.
References
Ahmadini, A. a. H., Modibbo, U. M., Shaikh, A. A., and Ali, I. (2021). Multi-objective optimization modelling of sustainable green supply chain in inventory and production management. Alexandria Eng. J. 60 (6), 5129–5146. doi:10.1016/j.aej.2021.03.075
Ali, I., Fügenschuh, A., Gupta, S., and Modibbo, U. M. (2020). The LR-type fuzzy multi-objective vendor selection problem in supply chain management. Mathematics 8 (9), 1621. doi:10.3390/math8091621
Ali, Z., Bi, G. B., and Mehreen, A. (2019). Predicting supply chain effectiveness through supply chain finance: Evidence from small and medium enterprises. Int. J. Logist. Manag. 30 (2), 488–505. doi:10.1108/ijlm-05-2018-0118
Asparouhov, T., Hamaker, E. L., and Muthen, B. (2018). Dynamic structural equation models. Struct. Equ. Model. A Multidiscip. J. 25 (3), 359–388. doi:10.1080/10705511.2017.1406803
Avelar-Sosa, L., Garcia-Alcaraz, J. L., Maldonado-Macias, A. A., and Mejia-Munoz, J. (2018). Application of structural equation modelling to analyse the impacts of logistics services on risk perception, agility and customer service level. Adv. Prod. Eng. Manag. 13 (2), 179–192. doi:10.14743/apem2018.2.283
Bai, W., Liu, Y. B., and Wang, J. J. (2022). An intelligent supervision for supply chain finance and logistics based on internet of Things. Comput. Intell. Neurosci. 2022, 1–9. doi:10.1155/2022/6901601
Deng, L. R., Wang, S. G., Wen, Y. X., and Li, Y. (2022). Incorporating 'mortgage-loan' contracts into an agricultural supply chain model under stochastic output. Mathematics 10 (1), 85. doi:10.3390/math10010085
Deng-Kui Sii, D. K., Li, X. L., and Huang, S. J. (2021). Financial deregulation and operational risks of energy enterprise: The shock of liberalization of bank lending rate in China. Energy Econ. 93, 105047. doi:10.1016/j.eneco.2020.105047
Ding, Z. H., Wu, W. B., and Liang, X. L. (2017). “Financial risk evaluation upon agricultural products supply chain an analysis based on uncertain type Analysis of hierarchy process,” in 4th International Conference on Industrial Economics System and Industrial Security Engineering (IEIS), Kyoto, Japan, July 24–27, 2017. doi:10.1109/IEIS.2017.8078631
Gao, Z. T. (2022). Application of internet of Things and block-chain technology in improving supply chain financial risk management system. Iete J. Res., 1–10. doi:10.1080/03772063.2021.2022539
Hasman, A. (2015). “An introduction to structural equation modeling,” in 13th International Conference on Informatics, Management, and Technology in Healthcare (ICIMTH), Athens, Greece, July 9–11, 2015, 3–6.
He, X. F., Zhang, C., Jing, Y. W., and Xingzheng, A. (2019). “Risk evaluation of agricultural product supply chain based on BP neural network,” in 16th International Conference on Service Systems and Service Management (ICSSSM), Shenzhen, China, 13-15 July 2019, 7744. doi:10.1109/ICSSSM.2019.8887744
He, X. J., and Tang, L. Y. (2012). “Exploration on building of visualization platform to innovate business operation pattern of supply chain finance,” in International Conference on Medical Physics and Biomedical Engineering (ICMPBE), Qingdao, China, 2012, 1886–1893. doi:10.1016/j.phpro.2012.05.298
Huang, S., and Liu, H. (2021). Impact of COVID-19 on stock price crash risk: Evidence from Chinese energy firms. Energy Econ. 101, 105431. doi:10.1016/j.eneco.2021.105431
Huang, X., Huang, S., and Shui, A. (2021). Government spending and intergenerational income mobility: Evidence from China. J. Econ. Behav. Organ. 191, 387–414. doi:10.1016/j.jebo.2021.09.005
Huang, Y. (2015). “Based on the supply chain of agricultural products logistics operational risk assessment and avoid,” in Seventh International Conference on Measuring Technology and Mechatronics Automation (ICMTMA 2015), Nanchang, China, 13-14 June 2015, 246–254. doi:10.1109/icmtma.2015.67
Huo, X. X., Jasimuddin, S. M., Zheng, K. N., and Zhang, Z. (2018). Exploring the risks of international SupplyChain financial warehouse receipts pledge model: A structural equation Approach. Supply Chain Forum Int. J. 6, 1–12. doi:10.1080/16258312.2022.2036076
Kulinska, E., and Giera, J. (2019). Identification and analysis of risk factors in the process of receiving goods into the warehouse. Found. Manag. 11 (1), 103–118. doi:10.2478/fman-2019-0009
Lefcheck, J. S. (2016). piecewiseSEM : Piecewise structural equation modelling in r for ecology, evolution, and systematics. Methods Ecol. Evol. 7 (5), 573–579. doi:10.1111/2041-210x.12512
Li, C., and Destech Publicat, I. (2016). “The analysis on influence factors of online supply chain finance credit,” in Joint International Conference on Economics and Management Engineering (ICEME) and International Conference on Economics and Business Management (EBM), China, June 2016, 140–148. doi:10.12783/dtem/iceme-ebm2016/4145
Li, X., and Sun, Y. (2022). Network evolutionary game-based diffusion mechanism regarding the nonperformance of farmers in agricultural supply chain finance. Discrete Dyn. Nat. Soc. 2, 1–11. doi:10.1155/2022/8550974
Li, Z., Jin, G. H., and Duan, S. (2018). Evolutionary game dynamics for financial risk decision-making in global supply chain. Complexity 2018 (3), 1–10. doi:10.1155/2018/9034658
Liu, C., and Destech Publicat, I. (2018). “Research on risk control of logistics finance,” in International Conference on Education, Social Sciences and Humanities (ICESSH), Chengdu, China, March 25–26, 2018, 289–293. doi:10.12783/dtssehs/icessh2018/23848
Modilbbo, U. M., Hassan, M., Ahmed, A., and Ali, I. (2022). Multi-criteria decision analysis for pharmaceutical supplier selection problem using fuzzy topsis. Manag. Decis. 60 (3), 806–836. doi:10.1108/MD-10-2020-1335
Mou, W. M., Wong, W. K., and Mcaleer, M. (2018). Financial credit risk evaluation based on core enterprise supply chains. Sustainability 10 (10), 3699. doi:10.3390/su10103699
Scott, P., and Newton, L. (2007). Jealous monopolists? British banks and responses to the macmillan gap during the 1930s. Enterp. Soc. 8 (4), 881–919. doi:10.1093/es/khm104
Sharma, R., Shishodia, A., Kamble, S., Gunasekaran, A., and Belhadi, A. (2020). Agriculture supply chain risks and COVID-19: Mitigation strategies and implications for the practitioners. Int. J. Logist. Res. Appl., 1–27. doi:10.1080/13675567.2020.1830049
Shee, A., Turvey, C. G., and You, L. Z. (2019). Design and rating of risk-contingent credit for balancing business and financial risks for Kenyan farmers. Appl. Econ. 51 (50), 5447–5465. doi:10.1080/00036846.2019.1613502
Van Bergen, M., Steeman, M., Reindorp, M., and Gelsomino, L. (2019). Supply chain finance schemes in the procurement of agricultural products. J. Purch. Supply Manag. 25 (2), 172–184. doi:10.1016/j.pursup.2018.08.003
Vovchak, O. D., Halkiv, L. I., and Demchyshyn, M. Y. (2018). The condition and problems of ykraines agricultural enterprises banking crediting development. Financial Credit Activity-Problems Theory Pract. 3 (26), 29–37. doi:10.18371/fcaptp.v3i26.143846
Wan, Y. M. (2021). Amos-based risk forecast of manufacturing supply chain. Int. J. Simul. Model. 20 (1), 181–191. doi:10.2507/IJSIMM20-1-CO3
Wang, H. Q., Xing, Y. Q., and Wang, Y. (2010). “Structural equation model and their application,” in 3rd International Institute of Statistics and Management Engineering Symposium, 512–516. Available at: https://www.webofscience.com/wos/woscc/full-record/WOS:000298334700091.
Wang, J., and Sun, Y. J. (2016). “Financial risk and management of agricultural product supply chain,” in ISSGBM International Conference on Information, Communication and Social Sciences (ISSGBM-ICS 2016), Dubai, United Arab Emirates, October 8–09, 2016, 168–172. Available at: https://www.webofscience.com/wos/woscc/full-record/WOS:000391773100032.
Wu, Y., Li, X., Liu, Q., and Tong, G. (2021). The analysis of credit risks in agricultural supply chain finance assessment model based on genetic algorithm and backpropagation neural network. Comput. Econ. doi:10.1007/s10614-021-10137-2
Xu, J. W. (2016). “Research on agricultural supply chain finance and risk management,” in 3rd International Symposium - Management, Innovation and Development (MID2016), Beijing Jiaotong University, December 10–11, 2016, 760–764. Available at: https://www.webofscience.com/wos/woscc/full-record/WOS:000438663500121.
Xuan, F. (2021). Regression analysis of supply chain financial risk based on machine learning and fuzzy decision model. J. Intelligent Fuzzy Syst. 40 (4), 6925–6935. doi:10.3233/JIFS-189523
Yang, Q., Wang, Y., and Ren, Y. (2019). Research on financial risk management model of internet supply chain based on data science. Cognitive Syst. Res. 56, 50–55. doi:10.1016/j.cogsys.2019.02.001
Yao, Y. J., and Liu, H. (2018). “Research on financing modes of small and medium-sized enterprises on the background of supply chain finance,” in International Conf.erence on Robotics and Intelligent Syst.em (ICRIS), Changsha, China, May 26–27, 2018, 571–576. doi:10.1109/ICRIS.2018.00147
Yu, Z., and Khan, S. a. R. (2021). Evolutionary game analysis of green agricultural product supply chain financing system: COVID-19 pandemic. Int. J. Logist. Res. Appl. 1, 1115–1135. doi:10.1080/13675567.2021.1879752
Zhang, G. R., Wang, C. L., Liu, J. J., and Zhou, L. (2022). Why do consumers prefer a hometown geographical indication brand? Exploring the role of consumer identification with the brand and psychological ownership. Int. J. Consum. Stud. doi:10.1111/ijcs.12806
Zhang, L., Tian, Y., Zhu, S., and Gao, C. (2015). The financing innovation pattern based on supply chain finance – a case study in Chinese steel industry. LISS 2013, 203–208. doi:10.1007/978-3-642-40660-7_29
Zhong, B. Y., and Su, Y. L. (2016). “The credit risk assessment model of internet supply chain finance: Bayesian networks model based on relative weight,” in 1st International Conference on Economic and Business Management (FEBM), Qingdao, China, October 1, 46–52. doi:10.2991/febm-16.2016.8
Keywords: agricultural products, supply chain, financial risks, structural equation model, countermeasures and suggestions
Citation: Yang J, Tang D, Kong H and Boamah V (2022) Research on financial risk management and control of agricultural products supply chain—A case study of Jiangsu Province of China. Front. Environ. Sci. 10:1008716. doi: 10.3389/fenvs.2022.1008716
Received: 01 August 2022; Accepted: 18 October 2022;
Published: 28 October 2022.
Edited by:
Rashi Taggar, Shri Mata Vaishno Devi University, IndiaReviewed by:
Umar Muhammad Modibbo, Modibbo Adama University of Technology, NigeriaSyed Abdul Rehman Khan, Xuzhou University of Technology, China
Copyright © 2022 Yang, Tang, Kong and Boamah. This is an open-access article distributed under the terms of the Creative Commons Attribution License (CC BY). The use, distribution or reproduction in other forums is permitted, provided the original author(s) and the copyright owner(s) are credited and that the original publication in this journal is cited, in accordance with accepted academic practice. No use, distribution or reproduction is permitted which does not comply with these terms.
*Correspondence: Decai Tang, dGFuZ2RlY2FpQG51aXN0LmVkdS5jbg==; Haojia Kong, YmVzdGtvbmdAbmp1c3QuZWR1LmNu