- 1School of Business, Guangxi University, Nanning, China
- 2College of Economics and Management, Beibu Gulf University, Qinzhou, China
- 3Trinity College of Arts and Sciences, Duke University, Durham, NC, United States
Circular economy is an important trend in the development of the world economy. The establishment of a sound green and low-carbon circular economy system is an important way and method for China to promote sustainable development. Based on the Technology-Organization-Environment (TOE) framework, this paper investigates the linkage effects of technological, organizational, and environmental conditions on the performance of the circular economy and their configuration paths using the fuzzy set qualitative comparative analysis method (fsQCA) with 30 provincial-level circular economy development levels in China as a case study. The study found that: 1) China’s circular economy performance in 2019 shows regional differences of “high in the east, medium in the centre and low in the west,” and there are also imbalances in development between different dimensions. 2) There is no single necessary condition that affects the circular economy, but rather the result of the combined influence of multiple condition variables. And the combination of different conditions has the characteristic of “different ways and the same way”. 3) There are obvious differentiations in the performance-driven paths of circular economy in eastern, central and western regions of China. Based on the research results, policy recommendations are provided for the development of China’s circular economy to promote the balanced development of the circular economy in the east, central and west.
1 Introduction
Due to rapid population growth and economic development, the negative impact of human activities on the Earth has increased significantly (Sharma et al., 2019). Human activities, especially the irrational exploitation and use of resources in the process of industrial production, have caused global environmental pollution and ecological disruption (Ahmad et al., 2020), such as land use (Lebreton and Andrady, 2019), biodiversity (Millhauser and Earle, 2022), air pollution (Miao et al., 2019), water pollution (Schwarzenbach, et al., 2010), and other issues. In addition, resource depletion and resource scarcity have become major challenges for the development of the world economy (Gao et al., 2021). All kinds of phenomena indicate that the problems faced by the Earth, such as transitional consumption of resources, the low recycling rate of resources, serious pollution emission, destruction of ecological environment and global climate and environmental changes, make the contradiction between human beings, resources, ecological environment and economic development more and more prominent. Achieving “sustainable development” of the economy and society has become more urgent (Markard et al., 2012).
The circular economy is a quality-driven economic development model characterized by resource conservation and recycling to prevent further environmental degradation (Yu et al., 2015). Compared to the traditional linear economy, the circular economy provides a sustainable circular model for economic systems (Andrews, 2015). The development of the circular economy is to establish the rules of sustainable resource management and make the socio-economic system an integral part of the ecosystem, to promote economic development and the ecological environment in harmony with each other (Mangla et al., 2018). Therefore, the circular economy, as an important way to implement sustainable development strategies, has gradually gained universal recognition in many countries (Tang et al., 2020). China, a particularly important and huge economy in the world, introduced the idea of the circular economy as early as the 1990s and quickly transformed it from a concept to a national strategy and regional practice (Tang et al., 2020). China not only promulgated the first national legislation on the global circular economy in 2008, the China Circular Economy Promotion Law (Wang N et al., 2018; Ding et al., 2020) but also integrated the development of the circular economy as a national strategy into the national development plans at different times early (Feng and Yan, 2007; Geng et al., 2012) on vigorously promoting the development of circular economy from different spatial scales: city, regional and national levels (Tang et al., 2020). In July 2021, China’s National Development and Reform Commission issued the “14th Five-Year Plan” for the development of the circular economy, which is a general arrangement for the 14th Five-Year Plan period to vigorously develop the circular economy and promote resource conservation and recycling. This shows that China has made further efforts to establish a sound “reduce, reuse, resource” green low carbon cycle development economic system.
To promote the development of the circular economy, it is necessary to research the influencing factors of the circular economy. Most papers explore the drivers and barriers to implementing the circular economy (Paletta et al., 2019; Khan et al., 2022; Neves and Marques, 2022). Lehmann et al. (2022) found that the circular economy is self-reliant; investment can promote the circular economy by reducing environmental degradation and promoting resource efficiency; innovation only has an impact on reducing environmental degradation, and human capital does not have a significant impact on the circular economy. Gusmerotti et al. (2019) conducted a logit regression to identify the drivers of the circular economy based on questionnaire data from 821 Italian companies and found that economic drivers have an important contribution to the circular economy, while the role of regulatory pressure, resource exploitation risks and environmental values remained insignificant. Robaina et al. (2020) explored the impact of environmental taxes, renewable energy rates, R&D intensity, recycling rates, population density, and services on the circular economy in Europe through an econometric model. Armeanu et al. (2018) studied the impact of higher education, business environment, infrastructure, technology, communication and media, population lifestyle and demographic changes on sustainable economic growth through a panel data regression model. Zhou et al. (2020) studied the impact of technological progress and structural change on sustainable economies and found that technological advances in pollution control, technological progress support and ecologisation of autochthonous structures are the main drivers of sustainable economic growth, and that innovation policies have a positive impact on national economic growth by promoting technological advances in pollution abatement. In addition, scholars have found that regional resource endowments, economic development, technological innovation, government environmental regulations, industrial restructuring and big data development also influence the development of the circular economy (Nobre and Tavares, 2017; Tang et al., 2020; Luo and Leipold, 2022; Shang et al., 2022). However, the impact of different factors on the performance of the circular economy is not independent, and they may be linked to each other to form different combinations to influence the development level of the regional circular economy. Therefore, a study of circular economy based on the “configuration perspective” can provide a deeper understanding of the complex mechanisms behind the development of circular economy in different regions. Based on provincial cross-sectional data for 2019, this study applies the Fuzzy Set Qualitative Comparative Analysis Method (fsQCA), and by introducing the Technology-Organization-Environment Framework (TOE) into the circular economy. The study constructs a research framework that affects the performance of the circular economy, explores the influence of technology, organization and environment factors on the performance of the circular economy, and identifies the conditional configurations and influence mechanisms that drive the improvement of the circular economy performance. Specifically, this study will attempt to address the following 3 questions: 1) What different condition sets exist to drive circular economy performance improvement? 2) Which conditions are more important for the improvement of the performance of the cycle? 3) Are there differences in the driving paths of circular economy performance in eastern, central and western China? This study helps broaden the research perspective of circular economy and deepen the understanding of the driving path and mechanism of circular economy development, providing scientific guidance to promote China’s comprehensive high-quality economic development.
The contributions of this study are as follows. 1) From a theoretical perspective, the introduction of the TOE theoretical framework into the study of circular economy performance not only enriches the research perspective of the circular economy but also broadens the scope of application of the TOE theoretical framework in the Chinese context. 2) In terms of research content, most of the existing studies have only explored the effects of individual antecedents on the circular economy (Armeanu et al., 2018; Gusmerotti et al., 2019; Robaina et al., 2020; Zhou et al., 2020), and the mechanisms of the effects of multiple interactions of antecedents on the circular economy are still rare. The present study bridges this gap by shifting the driving model of the circular economy performance from focusing on the influence of a single antecedent condition to a holistic perspective of the combined effects of technology, organization and environment, enriching the research content of the circular economy performance. It further analyzes the multiple concurrent effects of technology, organization, and environmental conditions on circular economy performance revealing different configuration paths for forming high-level circular economy performance and enhancing the relevance and accuracy of countermeasures to improve circular economy performance. 3) In terms of research methodology, most of the studies have been conducted using the research method of regression analysis. This paper introduces the fsQCA approach to the study of circular economy performance, which not only enriches the research tools of circular economy performance but also makes up for the lack of linkage in the TOE framework and provides a holistic perspective on the complex interaction mechanism behind the development of the circular economy.
2 Literature review and theoretical framework
2.1 Literature review
From the basic concept of circular economy to its practical implementation and evaluation, scholars have conducted many relevant studies and achieved rich research results. Since the 1960s, scholars have gradually carried out discussions on the relationship between ecological environment, resource utilization and human survival and development, and the idea of the circular economy has been born and enriched. The idea of the circular economy first originated in 1966 when American economist Boulding proposed the “spaceship economic theory” (Boulding, 1966). The theory is that the Earth’s resources are finite, and if resources are not developed and used rationally, it will eventually lead to resource depletion and environmental damage, and destruction like a spaceship (Boulding, 1966). In 1990, the British environmental economists Pearce and Turner formally introduced the concept of “circular economy,” arguing that economic systems should contain ecosystems to achieve the economical use of resources and reduce environmental pollution (Pearce and Turner, 1990). Since then, the concept of circular economy has evolved. Kirchherr et al. (2017) collected 114 definitions of the circular economy and systematically examined the core principles and differences between them. Blomsma and Brennan (2017) argue that the concept of the circular economy should include extending the productive life of resources through waste and resource management strategies. Geissdoerfer et al. (2017) consider sustainability as the basic concept and circular economy as the practical application. Korhonen et al. (2018) classify the circular economy as a “collaborative economy”. In addition, other scholars have studied the specific application of the concept of circular economy and the construction of circular economy models (Genovese et al., 2017; Ferronato et al., 2019).
Evaluation of the circular economy performance. Evaluation of circular economy performance helps to understand the effectiveness of national, regional and local circular economy development strategies (De Pascale et al., 2021). The evaluation method of the circular economy mainly includes material flow analysis (MFA) (Moriguchi, 2007; Chen, 2009), life-cycle assessment (LCA) (Daddi et al., 2017; Sommerhuber et al., 2017) and energy analysis (Geng et al., 2013; Pan and Li, 2016; Fan et al., 2017) and other methods. In terms of levels, scholars’ evaluation of circular economy performance can be divided into micro, meso, and macro levels. At the micro level, Franklin-Johnson et al. (2016) construct new performance metrics to measure circular economy performance from a resource utilization lifetime perspective and provide a management and organizational level tool that can be used to measure the impact of business decisions on the lifetime of precious materials. Park and Chertow (2014) constructed a material reuse potential indicator (RPI) to indicate the reuse potential of material at the current level of technology. Cayzer et al. (2017) designed a system of indicators for assessing product recyclability through expert consultation and questionnaires. At the meso level, Geng et al. (2010) discussed the energy value analysis method at the industrial park level and proposed an industrial park energy value index, and its potential was verified in the case of the Dalian Economic Development Zone. Fan et al. (2017) constructed energy value indicators that can evaluate the overall performance of industrial parks and sought to implement industrial coexistence in a circular economy framework. Wen and Meng (2015) used a material flow analysis and substance flow analysis combined with resource productivity indicators to assess the contribution of industrial co-production to the circular economy. Kayal et al. (2019) constructed a system of indicators to measure the recyclability of the wastewater industry based on the three Rs (reduce, reuse and recycle) principle. Chen et al. (2022) proposed an integrated assessment method for quantifying the benefits of industrial co-production in parks by combining resource productivity with energy value analysis that considers the impact of emissions. Liang et al. (2022) combined an ecological network approach and environmental and economic analysis to dynamically monitor and assess Eco-industrial park performance and further analyzed the changes in environmental and economic flows observed at the park level. At the macro level, the main focus is on evaluating the level of circular economy performance of cities, provinces and countries. At the city level, Wang N et al. (2018) proposed an urban circular economy performance evaluation index system based on expert scoring and entropy weight method and used it to evaluate and analyze the circular economy level of 40 pilot circular economy cities in China from 2012 to 2016. Ding et al. (2020) proposed a new extended Malmquist index to evaluate changes in industrial circular economy performance in 41 cities in the Yangtze River Delta region of China. Wang et al. (2021) constructed a fully fuzzy DEA method to assess the circular economy performance of 264 cities in China in the context of big data. Gao et al. (2021) constructed a framework for assessing the performance of circular economy based on ecological network analysis as a way to explore the performance of urban circular economy. At the provincial and national levels, Fan and Fang (2020) used data envelopment analysis to evaluate the level of circular economy development in 31 Chinese provinces in 2017. Geng et al. (2013) proposed an energy value indicator system to measure China’s circular economy performance. Li and Su (2012) comprehensively evaluated the development level of the circular economy of Chinese chemical enterprises from five aspects: economic development, resource development, pollution reduction, eco-efficiency and development potential. Jacobi et al. (2018) assess the level of circular economy in Austria in 2014 based on a large-scale flow of inputs of material resources linked to emissions and outputs of waste. Giannakitsidou et al. (2020) used data envelopment analysis to evaluate the performance of managing and utilizing municipal solid waste as a measure of environmental and circular economy performance in 26 EU countries.
Factors influencing the circular economy. Research on the factors influencing the circular economy has been conducted at the individual, household, community, industry level, and regional levels. The main focus at the individual, household and societal levels is on the recycling of resources. For example, Akil et al. (2015) analyzed the waste recycling of 600 households in an emerging city in Malaysia and showed that socioeconomic factors contribute to waste recycling. Mahmud and Osman (2010) studied the effects of specific attitudes, subjective norms and perceived behavioural control on recycling behaviour of Malaysian secondary school students based on the AMOS structural model test, and the results showed that the greatest effect on waste recycling behaviour. Starr and Nicolson (2015), using municipal recycling in Massachusetts, United States, found that charging by volume of waste was the most significant influencing factor. At the industry level, this includes an analysis of the barriers and drivers to implementing a circular economy in industries such as automotive, construction, and solid waste recycling. Khan et al. (2022) empirically analyze the drivers and barriers of the circular economy based on the Pakistani automotive industry, and the results show that resource efficiency, parent company support, social responsibility and international competition and promotion can drive the circular economy, while government policies, industrial support, lack of supply chain integration and supply chain complexity hinder the development of the circular economy. Giorgi et al. (2022) identified barriers and drivers for the implementation of the circular economy in the construction sector through interviews with construction industry stakeholders in five European countries. Upadhyay et al. (2021) reviewed several circular economy initiatives undertaken by the mining industry and assessed the barriers, drivers and triggers of these circular economy initiatives. Taghipour et al. (2022) investigated the mechanism of the role of government policies on sustainable management in the circular economy of steel recycling manufacturing companies through structural equation modelling and found that government policy solutions such as financial assistance, logistics and financing guidance related to the steel industry can promote the performance of steel recycling companies. At the regional level, Wright et al. (2019) explored the risks and opportunities of circular economy implementation in low- and middle-income economies, and the impact on environmental health. Neves and Marques (2022) empirically analyzed the role of economic, social and environmental factors on the circular economy and found that environmental regulation and environmental awareness can drive the circular economy, while GDP per capita, Gini index and poverty hurt the circular economy. Hong Nham and Ha (2022) empirically explored the two-way interaction between digital discourse and the circular economy based on data from 20 European countries and showed that the initial development of digitalization can promote the development of the circular economy in European countries, but the excessive development of digitalization has a hindering effect on the circular economy. Kumar et al. (2021) explored barriers to the adoption of Industry 4.0 and circular economy in agricultural supply chains and found that the lack of policies and protocols were significant barriers to the adoption of Industry 4.0 and circular economy in agricultural supply chain organizations, while strategic planning was the opposite. Shang et al. (2022) studied the role and influence mechanism of environmental regulations on the circular economy based on panel data of 286 cities in China and found that environmental regulations promote circular economy mainly through the “catch-up effect”. Zhou et al. (2020) studied the impact of technological progress and structural change on a sustainable economy and found that technological progress in pollution control, technological progress support and decolonization of autochthonous structures are the main drivers of sustainable economic growth and that innovation policies positively affect national economic growth by promoting technological progress in pollution reduction. Ryen and Babbitt (2022) explore the impact of current approaches on the adoption of circular economy solutions for waste food management by analyzing the state of federal and state policies in the United States. Kayikci et al. (2021) proposed the concept of a smart and sustainable circular economy at the macro level and addressed the barriers to a smart and sustainable circular economy in four areas: technology, producers, consumers, and policy.
2.2 Theoretical framework
The TOE analysis framework was initially used to study the factors influencing the use of technology in the enterprise, and it divides the factors influencing the use of technology into three dimensions: technology, organization and environment (Baker, 2012). As the TOE framework continues to evolve, scholars have given it new connotations and enhanced its research applicability, and it has been applied in some research areas, such as risk management (Ullah et al., 2021). In this paper, the theoretical model framework affecting the performance of the circular economy is constructed based on the TOE analysis framework in the context of the Chinese scenario, as shown in Figure 1.
2.2.1 Technical conditions
Technical conditions include the level of technological innovation and the level of development of big data. The circular economy is a new economic system that effectively integrates material, value and information flow, with the core idea of reducing waste and extending the life of materials while maintaining their value (Lehmann et al., 2022). Technological innovation is the key to achieving closed-loop material flows at all stages of production, distribution and consumption. In the 3Rs principles of circular economy, each of them requires technological support (Su et al., 2013). The emergence of new technologies and products caused by technological innovation gradually replaces the use of energy-intensive technologies and products, which can reduce resource consumption in production and life, reduce environmental pollution, improve resource reuse while enhancing production efficiency and quality of life, and thus promote the development of the economic cycle. Big data helps to open up the whole circular economy system. Big data technologies can track the flow of materials and obtain complete information about the product life cycle (Jayaraman et al., 2008), and the information collected is connected to stakeholders across the value chain through the Big Data-based Internet of Things, providing a data basis for assessing the behavioural outcomes of material flows at each stage (Pagoropoulos et al., 2017), establish a good and transparent communication mechanism between supply and demand to reduce friction and improve the efficiency of the material circulation. Therefore, technological innovation can solve the technical difficulties in all aspects of the circular economy system, and big data can stimulate and release the potential in circular economy management (Lieder and Rashid, 2016), the two synergize with each other to promote the healthy development of the circular economy system.
2.2.2 Organizational conditions
The organizational level includes industrial structure upgrading and factor endowments. Industrial structure upgrading and optimization are conducive to reducing environmental pollution (Wang Z et al., 2018). The transfer of industrial structure from heavy industry to service and high-tech industries has promoted the rational distribution and optimization of various resource factors among industries, so that resources can be fully utilized, thus reducing environmental pollution (Pasche, 2002) and improving the overall quality of the environment. In addition, the optimization and upgrading of industrial structure, as well as intra-industry and inter-industry synergistic innovation, promote the development of the tertiary industry, continuously improve the quality and level of the service industry, drive the transformation and upgrading of consumer demand, and thus promote the development of the circular economy. Neo-structuralist economics argues that the structural transformation of the economy by upgrading the factor endowment structure is a better model for regional economies to achieve sustainable and efficient development (Lin, 2011). The rational allocation of factor resources is an important means to improve technical efficiency, which not only enhances the quality of industrial development but also helps promote green total factor productivity, making enterprises more competitive in the domestic market and international market. The coordinated balance of factor endowment and industrial structure provides good inherent support for the development of the circular economy.
2.2.3 Environmental conditions
The environmental dimension includes environmental regulation and the level of economic development. In pollution control, environmental regulation is an important way to effectively compensate for the difficulty of regulating market mechanisms (Schneider, 2019). On the one hand, environmental regulation will directly reduce the generation and emission of pollution by raising product environmental standards, closing outdated production capacity, restricting the production of high pollution and high emission enterprises, and limiting emissions. On the other hand, the higher environmental pressure will promote the development of the circular economy by “innovation compensation,” which motivates enterprises to strengthen the research, development and innovation of green technologies (Thiel et al., 2016), change production methods, improve the utilization rate and treatment rate of pollutants, and reduce environmental pollution while promoting production. In addition, the development of the circular economy requires a large amount of capital investment, and the ability to invest capital depends on the regional economic situation (Tang et al., 2020). A high level of regional economic development means richer knowledge resources, human resources and financial resources, which can provide more support for resource recycling and pollutant management, thus facilitating the adoption and diffusion of the circular economy. At the same time, when the level of economic development reached a certain level, environmental pressure increased significantly, which prompted the development of regulations and technologies to improve efficiency and curb environmental pollution (Li et al., 2022).
3 Research design
3.1 Variable selection and measurement
Result Variables: China’s National Development and Reform Commission, together with the National Bureau of Statistics and other departments, released two versions of the circular economy indicator system in 2007 and 2017 to comprehensively assess the effectiveness of the development of the circular economy (Geng et al., 2012; Wang N et al., 2018). Based on the two circular economy indicator systems in China, and also drawing on the research results of Geng et al. (2009), Jia and Jun (2011), Li et al. (2011), Wang N et al. (2018) and other scholars, this paper constructs a regional circular economy indicator system based on the entropy weight method in three aspects: resource consumption intensity, waste emission intensity, and waste recycling and disposal (see Table 1), to measure the level of circular economy performance in each province.
Conditional variables: The level of technological innovation is expressed by the number of patent applications granted per 10,000 people. Patent application authorization has a high technical threshold, which requires regions to have high requirements in technology development, promotion and application. Patent application authorization has a high technical threshold, which requires regions to have high requirements in technology development, promotion and application. Therefore, the number of patent applications granted per 10,000 people can reflect the technological innovation capability of the region. Big data development level, this paper adopts the Big Data Development Index measured in the White Paper on the Assessment of China’s Big Data Regional Development Levels (2020) published by the China Electronics Information Industry Development Institute (CCID), a unit directly under the Chinese Ministry of Industry and Information Technology. This index provides a comprehensive measure of a region’s level of big data development. The level of development of the regional industrial structure is expressed as the proportion of the value added by the tertiary sector to the GDP (Cheng et al., 2018). The ratio of tertiary sector added value to GDP reflects the process of industrial structure moving to a higher level. Regional factor endowment is expressed as the ratio of capital to labour (Cole and Elliott, 2003), where capital is the local stock of fixed assets and labour is expressed as the total number of employees in each province. China currently suffers from the problem of over-allocation of labour and under-allocation of capital. Therefore, the ratio of capital to labour at this stage can better measure the regional factor endowment structure. Environmental regulation is expressed by the ratio of the total cost of operating wastewater and waste gas facilities to the GDP, and the larger the value of this indicator, the greater the effort of environmental management and the higher the intensity of environmental regulation. The level of economic development is measured by GDP. The size of GDP is a good indicator of the level of economic development of a region.
3.2 Qualitative comparative analysis method
Qualitative Comparative Analysis (QCA) was created by sociologist Ragin (Ragin, 1987). Unlike traditional analysis techniques, QCA is a case-oriented analysis approach that does not recognize constant causality or assume symmetry of causality, but rather decomposes each case into a series of characteristics and combines case analysis with cross-case comparisons to explore the logical relationships between different sets of conditions and outcome variables. In addition, when using the QCA method, the units of each variable are not required to have homogeneity, and there is no need to treat different levels of variables (Lacey and Fiss, 2009). The fuzzy set qualitative comparison method (fsQCA) is one of the QCAs, which avoids dichotomizing variables. The fsQCA method can fully explore the role of antecedent variables on the results, reduce the loss of information in cases during data processing, and make the study conclusions more refined and reliable (Krogslund et al., 2015). Therefore, this paper adopts fsQCA to conduct a histological analysis of variables of different nature in this study and tries to analyze the multiple and complex mechanisms of action behind the performance of China’s circular economy from a histological perspective, combining the advantages of qualitative and quantitative analysis, and focusing on deeply exploring the complexity of antecedent conditions and causal asymmetry, rather than just considering the impact of individual antecedent conditions on the circular economy. This method can effectively explore the synergies and interactions among the preconditions, i.e., it can be used to explore the drivers of the circular economy and explore the paths to improve the performance of the circular economy from a group perspective (Mendel and Korjani, 2013).
3.3 Data source
In this paper, 30 provinces, cities and autonomous regions in China (except Hong Kong, Macao, Taiwan and Tibet) in 2019 are taken as the research objects, and the research data are mainly obtained from the national database on the official website of the National Bureau of Statistics, China Statistical Yearbook 2020, China Environmental Statistical Yearbook 2020, China Energy Statistical Yearbook 2020, the White Paper on the Assessment of the Regional Development Level of Big Data in China (2020) and the Statistical Yearbook of each province in 2020.
3.4 Data calibration
Data calibration is an important step in conducting fsQCA analysis, and in this paper, the direct calibration method was used to set the 75%, 50% and 25% quantile values of all continuous variables as the three thresholds for the membership levels of full input, intermediate, and full output, respectively (Fiss, 2011; Coduras et al., 2016), which in turn transformed the original variable data into fuzzy affiliation values from 0 to 1. At the same time, there is a situation where the sample data is equal to 0.5 after the intersection calibration, which makes this part of the data directly ignored by the software and thus affects the accuracy of the conclusion, so the true value of 0.5 is corrected to 0.5001 for calculation (Fiss, 2011). The calibration anchor points for all condition variables are shown in Table 2.
4 Empirical analysis
4.1 Comprehensive evaluation of China’s circular economy development
The composite score of the circular economy performance of 30 provinces in China in 2019 was calculated based on the entropy weighting method, as shown in Figure 2. It can be seen that the average development level of China’s circular economy in 2019 is 0.6810, achieving better development, but showing regional differences of “high in the east, medium in the middle and low in the west”, with the average development level of circular economy in the east, middle and west regions being 0.7682, 0.6814 and 0.5936 respectively. This indicates that there are regional differences and uncoordinated development problems in the process of circular economy development in China. Specifically, the top ten circular economy performance rankings in 2019 are Zhejiang, Tianjin, Shandong, Hubei, Guangdong, Beijing, Jiangsu, Henan, Anhui and Shanghai. Except for Hubei, Henan and Anhui, which are located in the central region, all others are located in the economically developed eastern region. Seven of the bottom ten provinces are located in the western region, which shows that the economic growth in the western region is generally dominated by a rough and sloppy approach. Xinjiang, Heilongjiang, Neimenggu, Qinghai, and Ningxia’s circular economy performance is more than 20% below the national average circular economy performance. Xinjiang lags mainly due to the greater intensity of resource consumption and waste emissions, and its waste recycling and disposal levels are relatively high. While Heilongjiang, Neimenggu, Qinghai and Ningxia are lagging in all dimensions, especially Ningxia’s waste emission intensity is particularly prominent.
Figure 3 shows the circular economy sub-dimension scores by the province in China in 2019. From the three dimensions, the resource consumption intensity score and the waste recycling and disposal score are relatively higher, and the waste emission score is relatively low, which shows that China has made certain achievements in resource conservation and waste recycling, but there is still the problem of high pollution emission intensity in the process of economic development. By province, the top five provinces in terms of resource consumption intensity score are Beijing, Zhejiang, Guangdong, Shaanxi and Henan, the top five provinces in terms of waste emission intensity score are Henan, Chongqing, Tianjin, Hubei and Shandong, and the top five provinces in terms of waste recycling and disposal score are Tianjin, Zhejiang, Hubei, Shandong and Jiangsu.
4.2 Single condition necessity analysis
Before conducting the conditional group analysis, it is necessary to check the “Necessity” of each conditional variable. In fsQCA, when the occurrence of a result variable depends on a single condition, then that condition is a necessary condition for the result variable. In this paper, we test whether a single condition (including its non-set) constitutes a necessary condition for the performance of the cycle via the software fsQCA 3.0. Consistency is an important test for necessary conditions, and in general when consistency is greater than 0.9, then the condition variable is considered necessary for the outcome variable (Schneider and Wagemann, 2012). Table 3 shows the results of the necessity test for high and non-high levels of circular economy performance analyzed using fsQCA 3.0 software (the symbol “∼” indicates the absence of the given variable). The results show that the consistency level for all conditions is less than 0.9. Therefore, there is no single necessary condition that affects high or low levels of circular economy performance.
4.3 Analysis of configuration conditions
By determining the adequacy of the antecedent condition grouping, the grouping analysis can reveal the differentiated pathways affecting the performance of the circular economy. Based on the characteristics of the case data, and to avoid contradictory grouping situations, the original consistency threshold, the PRI consistency threshold and the frequency threshold are set to 0.80, 0.80 and 1 respectively. The specific configuration results are shown in Table 4. There are five driving paths for high circular economy performance (configurations 1–5), and their consistency is 0.9318, 0.9127, 0.9778, 0.9778 and 0.9661, respectively, and the consistency of the overall solution reaches 0.9422, which is much higher than the minimum acceptable standard of 0.75 for both individual solutions (configuration) and the overall solution, indicating that all five paths are all sufficient conditions for the formation of high circular economy performance.
Configuration 1 (TI-BD-FE-ED): This configuration indicates that when the presence of big data and economic development plays a central role and the presence of technological innovation and factor endowments plays a supporting role, it will lead to a high level of circular economic performance. The level of technological innovation provides the technical basis for the recycling and full utilization of materials, while the development of big data opens up the circular economy system, promotes the extension of the industrial chain and reduces intermediate links. The high factor endowment represents a reasonable and efficient allocation of resources, improves the efficiency of material circulation and enhances economic efficiency, and the high level of economic development provides financial and knowledge support for the circular economy and promotes the healthy development of the circular economy system. A high level of big data development and a high level of economic development are the two core conditions for achieving high circular economy performance under this path. At the same time, complemented by a high level of technological innovation and high factor endowment, it can further optimize the allocation of relevant resources and stimulate the willingness of enterprises and individuals to participate in the circular economy, jointly promoting the generation of high circular economic performance. According to the “Environmental Kuznets Curve (EKC)” hypothesis, the level of environmental pollution starts to decrease when the economy continues to grow and breaks through the so-called “inflexion point” (Grossman and Krueger, 1995). Technological progress and optimization of industrial structure brought about by economic development reduce environmental pollution, improve the efficiency of resource utilization, and thus enhance the performance of the circular economy. Since the driving path consists of technological innovation (technology), big data development (technology), factor endowment (organization) and economic development (environment), we named this grouping “technology-organization-environment”. The unique coverage of this configuration is 0.1803 and the original coverage is 0.4604. This path explains about 46.04% of the circular economy performance cases and is the main path that generates a high level of circular economy performance, with typical cases in Beijing, Shanghai and Jiangsu, as shown in Figure 4A. In addition, about 18.03% of the circular economy performance cases can be explained by this path only. Taking Beijing as an example, the GDP in 2021 is 4,026.96 billion yuan, which provides a better economic foundation for the development of the circular economy, while in Beijing there are world-class universities such as Peking University and Tsinghua University with research institutes such as the Beijing Institute of Big Data, as well as having the Beijing Chaoyang Circular Economy Industrial Park, which provides all-round support for the development of the circular economy in terms of technology, organization and environment.
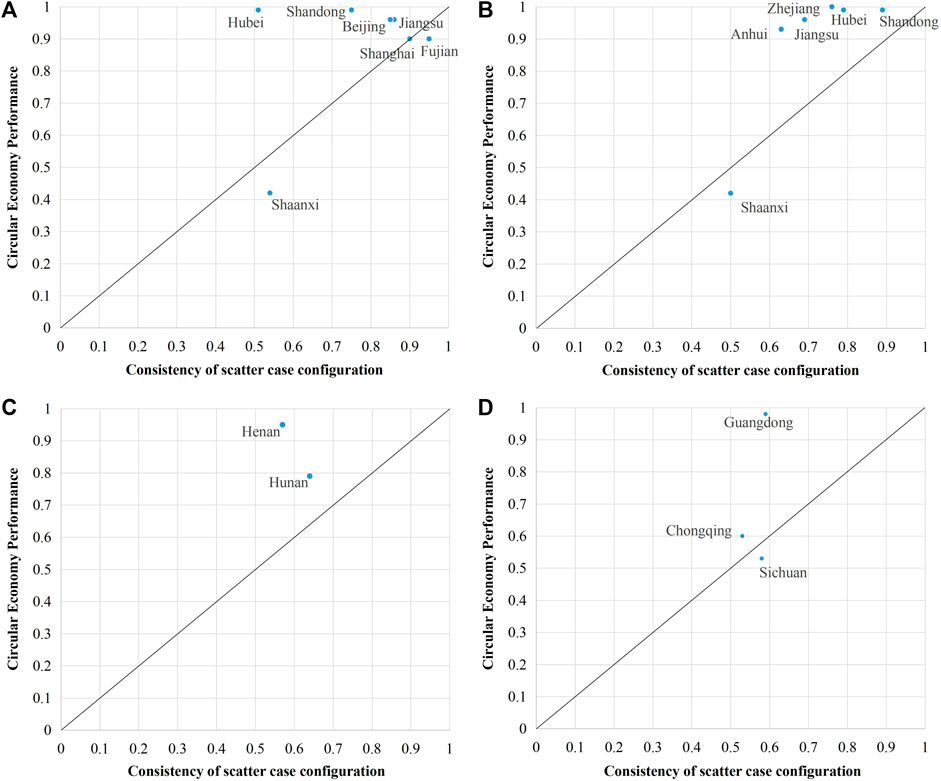
FIGURE 4. Scatter plot of high-level circular economy performance configuration cases: (A) Configuration 1, (B) Configuration 2, (C) Configuration 3 and 5, (D) Configuration 4.
Configuration 2 (TI-BD-ER-ED): The presence of big data development and economic development plays a central role, and the presence of technological innovation and environmental regulation plays a supporting role. This configuration is more similar to configuration 1. A high level of big data development and a high level of economic development are the two core conditions for achieving high circular economy performance under this path, complemented by a high level of technological innovation and strong environmental regulation to work together with the core conditions. In contrast to configuration 1, when there is a high level of technological innovation, a high level of big data development and a high level of economic development, environmental regulation (environment) can form a substitution relationship with factor endowment (organization), i.e., when there is a high level of technology (with a high level of technological innovation and a high level of big data development) and a high level of economic development, environmental regulation can substitute the role of factor endowment to promote regional circular economy performance by constraining the pollution emissions of enterprises and forcing them to undertake technological innovation. Since this driving path consists of technological innovation (technology), big data development (technology), environmental regulation (environment) and economic development (environment), we named this grouping “technology-environment”. The unique coverage of this configuration is 0.0779 and the original coverage is 0.3520. This path can explain about 35.20% of the circular economy performance cases and about 7.79% of the circular economy performance cases can be explained by this path only, as shown in Figure 4B.
Configuration 3 (∼TI-BD-∼IS-∼FE-∼ER-ED): The presence of big data and economic development plays a central role. This means that when high levels of big data development and high economic development are present, the presence or absence of other conditions is not important for high levels of circular economy performance, and that big data development (technology) and economic development (environment) are particularly important for high levels of circular economy performance compared to other conditions. Since this driving path consists of big data development (technology) and economic development (environment), we also name this configuration as “technology-environment”. This means that the central role of big data and economic development can effectively break the constraints of organizational and other conditions on the performance of the provincial circular economy. The unique coverage of this configuration is 0.0258 and the original coverage is 0.1453. This path can explain about 14.53% of the circular economy performance cases, and about 2.58% of the circular economy performance cases can only be explained by this path. In Figure 4C, Henan’s GDP in 2021 is 58887.41 billion, ranking fifth in the country, while there is a Henan big data industrial park, which actively cultivates and grows the big data industry clusters to provide economic and big data support for the construction of circular economy.
Configuration 4 (TI-BD-IS-∼FE-∼ER): The presence of technological innovation and the absence of environmental regulation play a central role, and the presence of big data and industrial structure upgrading play a complementary role. The absence of environmental regulation as a core condition means that “punitive” policies in the government institutional environment are weak, while the presence of technological innovation as a core condition indicates that technological innovation is playing a central role. According to the “New Growth Theory”, technological change is an important tool for dealing with environmental problems (Weitzman, 1997). Technological innovation improves the quality of the environment while promoting the economy, thus contributing to the achievement of sustainable economic development (Omri, 2020). A high level of technological innovation can effectively compensate for the lack of motivation for the development of the circular economy that may exist in a relaxed institutional environment, and actively reduce resource consumption and improve resource utilization in the production and consumption process through advanced technology level. A high level of technological innovation and weak environmental regulation are the two core conditions for achieving high circular economy performance under this path, which can be complemented by a high level of big data development and a high level of industrial structure upgrading to promote high circular economy performance. This means that provinces with a high level of industrial structure upgrading, when environmental conditions are absent, and with the support of technological conditions, enterprises in the region can also quickly adjust their resource utilization methods and waste discharge, recycling and disposal, thus improving the level of circular economy performance. Since the driving path consists of technological innovation (technology), big data development (technology) and industrial structure upgrading (organization), we named this configuration “technology-organization”. The unique coverage of this configuration is 0.0410 and the original coverage is 0.2041. This path can explain about 20.41% of the circular economy performance cases and about 4.10% of the circular economy performance cases can be explained by this path only, as shown in Figure 4D.
Configuration 5 (∼TI-∼BD-IS-∼FE-∼ER-ED): The presence of industrial structure upgrading and economic development plays a central role. This indicates that industrial upgrading (organization) and economic development (environment) are particularly important for high levels of circular economy performance compared to other conditions. According to gradient shift theory, the development of a regional economy depends on the status of its industrial structure. The high level of industrial structure upgrading implies a higher level of tertiary industry development than the less polluting service industry and high-tech industry and enhances the performance of the circular economy by driving intra-industry and inter-industry synergistic innovation and transformation and upgrading of consumer demand. Since this driving path is driven by both industrial structure upgrading (organization) and economic development (environment), we also name this configuration “organization-environment”. This implies that when other conditions are absent, the central role of industrial structure upgrading and economic development can effectively break the constraints of technology and other conditions on the performance of the provincial circular economy, and thus promote the development of the regional circular economy. The unique coverage of this configuration is 0.0502, and the original coverage is 0.1129. This path can explain about 11.29% of the circular economy performance cases and about 5.02% of the circular economy performance cases can be explained by this path only, as shown in Figure 4C.
4.4 Comparison between eastern, central and western regions
Due to the influence of development mode, geographical location, resource endowment, and institutional environment, the level of circular economy development in different regions of China has obvious heterogeneity. At the same time, These factors may also lead to different effects on the level of technological innovation, the level of big data development, industrial structure upgrading, factor endowment, environmental regulation, and the level of economic development on circular economy performance. Therefore, this paper divides the full sample data into eastern, central, and western regions according to the way most scholars divide them1, and then conducts a comparative analysis of the circular economy performance in each region to explore the differentiation of the impact of technological, organizational, and environmental factors on the circular economy performance in different regions.
As can be seen from Table 5, there are four paths to achieve a high level of circular economy performance in the eastern region of China, of which configurations 1–3 are “technology-organization-environment” and configuration 4 is “technology-environment”. Specifically, configuration 1 as a more comprehensive development path, regardless of the level of industrial structure upgrading, can also achieve a high level of circular economy performance when technological innovation and environmental regulation exist as core conditions, and big data development, factor endowment and economic development exist as auxiliary conditions. Configuration 2 shows that the circular economy can also be driven by the support of technological innovation as a core condition and the constraints of environmental regulations, complemented by industrial structure upgrading and factor endowments. In Configuration 3, big data and economic development exist as core conditions, and technological innovation and industrial structure upgrading exist as supporting conditions. In Configuration 4, big data development and economic development appear as core conditions, and environmental regulation appears as a secondary condition. The eastern region of China belongs to the more economically developed regions and has better basic conditions at the technological, organizational and environmental levels. Among the four configurations of high-level circular economy performance, configurations 1–2 all exist with technological innovation and environmental regulation as core conditions, and configurations 3–4 all exist with big data and economic development as core conditions. The two different sets of core conditions suggest that the combination of technological innovation and environmental regulation or big data development and economic development in the eastern region can achieve a high level of circular economy performance in a “different way” with different combinations of marginal conditions.
As can be seen from Table 6, there are four paths to achieve a high level of circular economy performance in the central region of China, with configuration 1 being “technology-environment” and configurations 2–4 being “technology-organizational-environment”. Specifically, in configuration 1, big data development and economic development exist as core conditions, and technological innovation appears as a secondary condition. In configuration 2, big data development and economic development exist as core conditions, and industrial structure upgrading appears as auxiliary conditions. In Configuration 3, big data development and economic development exist as core conditions, and technological innovation, industrial structure upgrading and environmental regulation appear as auxiliary conditions. In configuration 4, big data development and economic development exist as core conditions, and technological innovation, factor endowment and environmental regulation appear as supporting conditions. The central region of China has “east to west, through the north and south” location advantages, as well as abundant human resources, infrastructure and other factors such as the advantages of being more complete. The results of the analysis for the central region show that the level of big data development and the level of economic development in the central region are the core conditions for the development of a high level of circular economy performance in the central region, which can achieve a high level of circular economy performance by combining with different marginal conditions. The better level of economic development in the central region and the construction of big data drives the development of the circular economy.
As can be seen from Table 7, there are four paths to achieve a high level of circular economy performance in the western region of China, with configuration 1 being “technology-environmental”, configuration 2 being “technology-based” which is driven by technical conditions only, and configurations 3–4 being “technology -organization-environment”. Specifically, in configuration 1, the level of industrial structure upgrading and economic development exist as auxiliary conditions, indicating that a high level of circular economy performance can be achieved by overcoming the shortcomings of other conditions, such as the lack of environmental regulations, driven by both industrial structure upgrading and economic development. In configurations 2–4, the level of technological innovation and the development of big data exist as core conditions, which contribute to a high level of circular economy performance when combined with different auxiliary conditions. The western region does not have advantages at the technological, organizational, and environmental levels, and has certain gaps compared with the central and eastern regions. Enhancing the circular economy performance in the western region can be achieved by strengthening technological innovation and developing big data, complemented by a combination of organizational and environmental factors, as well as by promoting an alternative path to industrial structure upgrading and economic development. Technology as support to bridge the gap between resource endowment and development status is the main way to promote circular economy development in the western region.
4.5 Robustness tests
This paper uses a robustness test by adjusting the consistency threshold to ensure the robustness of the high-level circular economy performance histogram pathways obtained from the study. This is done by increasing the consistency threshold by 0.05 without changing the number of cases frequency (Zhou, et al., 2022). By observing the results of the robustness test on the overall sample it can be found (results omitted) that there is no change between the results of the study before and after the consistency threshold adjustment. At the same time, the same method was used to test the conditional configuration of the eastern, central and western regions, and still, the same results and consistent conclusions were obtained. Therefore, the conclusions of this paper can be judged to be robust.
5 Conclusion
5.1 Conclusion
This paper constructs a TOE integrated analysis framework for the circular economy, measures China’s circular economy performance based on relevant data from 30 provinces in China, and for the first time uses the fsQCA method for conditional conclusions analysis to explore the linkage effects and driving paths of technology, organization and environmental factors on multiple concurrent circular economy performance, revealing the complex interaction mechanisms affecting circular economy performance. The main research findings are as follows:
(1) China’s 2019 circular economy performance has achieved better development but shows a “high in the east, the central middle, the western low” regional differences, and in different dimensions, there is also an imbalance between the development of the phenomenon.
(2) In general, there is no single necessary condition, circular economy performance is influenced by a combination of conditional variables, and technical, organizational, and environmental factors cannot be considered as necessary conditions for circular economy performance alone. There are 5 driving paths to high levels of circular economy performance. Specifically, it can be summarized as a driving model in which technology, organization and environment act together, a driving model in which technology and environment act together, a driving model in which technology and organization act together, and a driving model in which organization and environment act together. The effective combination and synergy of the different factors behind the circular economy can lead to a high level of circular economy performance in a “different way”.
(3) Due to the different location conditions and resource endowments of each region, there are obvious differentiations in the circular economy performance driving paths in eastern, central and western regions of China. The eastern and central regions have a balanced “technology-organization-environment” driven development strategy, while the western region also has a “technology-based” driven strategy. The significant differences in the histological paths of circular economy performance between eastern, central and western provinces further indicate the asymmetric causality that contributes to the heterogeneity of circular economy performance.
5.2 Policy implications
A circular economy system is a complex and dynamic system, not determined by a single condition, but by a combination of multiple technical, organizational, and environmental factors. Therefore, the policy implications of this paper are as follows:
(1) Provinces should strengthen the linkage and integration between technical, organizational and environmental conditions to develop a circular economy. Regions should effectively combine multi-faceted factors, not only focus on technology level upgrading, but also accelerate the promotion of industrial structure upgrading, actively adjust the factor endowment structure, and develop good government environmental regulations to restrain market behaviour and get support from economic growth, and then enhance the fit between market mechanisms and government regulation to achieve effective synergistic integration and joint efforts among multiple conditions of technology, organization and environment. Each region should explore the most optimal adaptation relationship of each element to lay a good development foundation for promoting the construction of the circular economy system.
(2) Each region should take into account local conditions, based on their development bottlenecks, focus on mining local resources, find the best configuration for the development of the province’s circular economy, and thus improve the level of performance of the circular economy. Specifically, according to their development status, location conditions and resource endowment, different provinces should find their positioning, give full play to their advantages, break their development constraints, explore effective differentiated diversified paths, formulate targeted differentiated development strategies to enhance the performance of the circular economy, promote the balanced development of the circular economy in the eastern, central and western regions of China, and then comprehensively build a high-level circular economy system. For the eastern region, a more comprehensive circular economy development can be achieved by relying on the developed level of economic development, the level of big data development, and the level of technological innovation. The provinces in the central region should focus on promoting the development of a circular economy with the support of big data and with the help of economic development. In the western region, on the other hand, the main focus should be on improving the technology level by introducing advanced technology to enhance the circular economy performance.
(3) Enhance the strength of regional technological innovation and big data development to provide strong technical support for the development of the regional circular economy. Regional government departments should set up innovation service platforms and formulate innovation incentive policies, accelerate key technology research and development and innovation, strengthen the innovation-driven role of circular economy development; improve the circular economy data and information disclosure and sharing mechanism, and improve the basic database of circular economy information resources, promote the optimization of information resources and maximize resource utilization, and provide strong protection for the development of the circular economy.
(4) Each region should adjust its industrial policy according to its actual situation, optimize the regional factor endowment structure, and improve the efficiency of factor allocation. The region’s resource endowment and production support system should be utilized to accelerate technological innovation and transformation of innovation results, enhance the role of the tertiary industry in promoting the circular economy, and at the same time, establish a sound regional coordination mechanism for the circulation and sharing of resource elements to promote the orderly flow and rational allocation of resource elements, thereby improving the quality of economic development and enhancing the performance of the circular economy.
(5) To take advantage of economic development, promote the adoption and spread of the circular economy, and develop and implement environmental regulations to enhance the overall effect of environmental management. All regions should strengthen the role of economic development to support the circular economy and make good use of human, financial and material resources to promote the development of the circular economy. At the same time, it is also necessary to develop differentiated environmental regulations according to their development characteristics and to grasp the applicable intensity of environmental regulation implementation to increase the willingness of enterprises and individuals to develop a circular economy.
Data availability statement
The raw data supporting the conclusion of this article will be made available by the authors, without undue reservation.
Author contributions
The contribution of LL is conceptualization, formal analysis, investigation, resources, and writing—original draft; the contribution of FZ is methodology, investigation, supervision, funding acquisition, and writing—review and editing; the contribution of ZZ is validation, visualization, writing—review and editing; the contribution of XZ writing—original draft. All authors contributed to manuscript revision and read and approved the submitted version.
Funding
This research was funded by the National Natural Science Foundation of China (71662026); Guangxi Development Strategy Research Institute of Guangxi Key Research Base of Humanities and Social Sciences in Guangxi Universities Youth Cultivation Grant Project (2022GDSIQM13).
Conflict of interest
The authors declare that the research was conducted in the absence of any commercial or financial relationships that could be construed as a potential conflict of interest.
Publisher’s note
All claims expressed in this article are solely those of the authors and do not necessarily represent those of their affiliated organizations, or those of the publisher, the editors and the reviewers. Any product that may be evaluated in this article, or claim that may be made by its manufacturer, is not guaranteed or endorsed by the publisher.
Footnotes
1East including Liaoning, Beijing, Tianjin, Hebei, Shandong, Jiangsu, Shanghai, Zhejiang, Fujian, Guangdong, and Hainan; Central including Shanxi, Heilongjiang, Jilin, Anhui, Henan, Jiangxi, Hubei, Hunan; West including Neimenggu, Shaanxi, Gansu, Qinghai, Ningxia, Xinjiang, Sichuan, Chongqing, Yunnan, Guizhou, Guangxi.
References
Ahmad, M., Jiang, P., Majeed, A., Umar, M., Khan, Z., and Muhammad, S. (2020). The dynamic impact of natural resources, technological innovations and economic growth on ecological footprint: an advanced panel data estimation. Resour. Policy 69, 101817. doi:10.1016/j.resourpol.2020.101817
Akil, A. M., Foziah, J., and Ho, C. S. (2015). The effects of socio-economic influences on households recycling behaviour in iskandar malaysia. Procedia - Soc. Behav. Sci. 202, 124–134. doi:10.1016/j.sbspro.2015.08.215
Andrews, D. (2015). The circular economy, design thinking and education for sustainability. Local Econ. 30 (3), 305–315. doi:10.1177/0269094215578226
Armeanu, D. Ş., Vintilă, G., and Gherghina Ş, C. (2018). Empirical study towards the drivers of sustainable economic growth in eu-28 countries. Sustainability 10 (1), 4. doi:10.3390/su10010004
Baker, J. (2012). “The technology–organization–environment framework,” in Information systems theory: explaining and predicting our digital society. Editors Y. K. Dwivedi, M. R. Wade, and S. L. Schneberger (New York, NY: Springer New York), 1, 231–245. doi:10.1007/978-1-4419-6108-2_12
Blomsma, F., and Brennan, G. (2017). The emergence of circular economy: a new framing around prolonging resource productivity. J. Ind. Ecol. 21 (3), 603–614. doi:10.1111/jiec.12603
Boulding, K. (1966). The economics of coming spaceship earth. Baltimore: The Johns Hopkins University Press. doi:10.4324/9781315064147
Cayzer, S., Griffiths, P., and Beghetto, V. (2017). Design of indicators for measuring product performance in the circular economy. Int. J. Sustain. Eng. 10 (4-5), 289–298. doi:10.1080/19397038.2017.1333543
Chen, J. Z. (2009). Material flow and circular economy. Syst. Res. Behav. Sci. 26 (2), 269–278. doi:10.1002/sres.968
Chen, X., Dong, M., Zhang, L., Luan, X., Cui, X., and Cui, Z. (2022). Comprehensive evaluation of environmental and economic benefits of industrial symbiosis in industrial parks. J. Clean. Prod. 354, 131635. doi:10.1016/j.jclepro.2022.131635
Cheng, Z., Li, L., and Liu, J. (2018). Industrial structure, technical progress and carbon intensity in China's provinces. Renew. Sustain. Energy Rev. 81, 2935–2946. doi:10.1016/j.rser.2017.06.103
Coduras, A., Clemente, J. A., and Ruiz, J. (2016). A novel application of fuzzy-set qualitative comparative analysis to gem data. J. Bus. Res. 69 (4), 1265–1270. doi:10.1016/j.jbusres.2015.10.090
Cole, M. A., and Elliott, R. J. R. (2003). Determining the trade–environment composition effect: the role of capital, labor and environmental regulations. J. Environ. Econ. Manag. 46 (3), 363–383. doi:10.1016/S0095-0696(03)00021-4
Daddi, T., Nucci, B., and Iraldo, F. (2017). Using life cycle assessment (lca) to measure the environmental benefits of industrial symbiosis in an industrial cluster of smes. J. Clean. Prod. 147, 157–164. doi:10.1016/j.jclepro.2017.01.090
De Pascale, A., Arbolino, R., Szopik-Depczyńska, K., Limosani, M., and Ioppolo, G. (2021). A systematic review for measuring circular economy: the 61 indicators. J. Clean. Prod. 281, 124942. doi:10.1016/j.jclepro.2020.124942
Ding, L., Lei, L., Wang, L., and Zhang, L. (2020). Assessing industrial circular economy performance and its dynamic evolution: an extended malmquist index based on cooperative game network dea. Sci. Total Environ. 731, 139001. doi:10.1016/j.scitotenv.2020.139001
Fan, Y., and Fang, C. (2020). Circular economy development in China-current situation, evaluation and policy implications. Environ. Impact Assess. Rev. 84, 106441. doi:10.1016/j.eiar.2020.106441
Fan, Y., Qiao, Q., Fang, L., and Yao, Y. (2017). Emergy analysis on industrial symbiosis of an industrial park – a case study of hefei economic and technological development area. J. Clean. Prod. 141, 791–798. doi:10.1016/j.jclepro.2016.09.159
Feng, Z., and Yan, N. (2007). Putting a circular economy into practice in China. Sustain. Sci. 2 (1), 95–101. doi:10.1007/s11625-006-0018-1
Ferronato, N., Rada, E. C., Gorritty Portillo, M. A., Cioca, L. I., Ragazzi, M., and Torretta, V. (2019). Introduction of the circular economy within developing regions: a comparative analysis of advantages and opportunities for waste valorization. J. Environ. Manage. 230, 366–378. doi:10.1016/j.jenvman.2018.09.095
Fiss, P. C. (2011). Building better causal theories: a fuzzy set approach to typologies in organization research. Acad. Manage. J. 54 (2), 393–420. doi:10.5465/amj.2011.60263120
Franklin-Johnson, E., Figge, F., and Canning, L. (2016). Resource duration as a managerial indicator for circular economy performance. J. Clean. Prod. 133, 589–598. doi:10.1016/j.jclepro.2016.05.023
Gao, H., Tian, X., Zhang, Y., Shi, L., and Shi, F. (2021). Evaluating circular economy performance based on ecological network analysis: a framework and application at city level. Resour. Conservation Recycl. 168, 105257. doi:10.1016/j.resconrec.2020.105257
Geissdoerfer, M., Savaget, P., Bocken, N. M. P., and Hultink, E. J. (2017). The circular economy – a new sustainability paradigm? J. Clean. Prod. 143, 757–768. doi:10.1016/j.jclepro.2016.12.048
Geng, Y., Fu, J., Sarkis, J., and Xue, B. (2012). Towards a national circular economy indicator system in China: an evaluation and critical analysis. J. Clean. Prod. 23 (1), 216–224. doi:10.1016/j.jclepro.2011.07.005
Geng, Y., Sarkis, J., Ulgiati, S., and Zhang, P. (2013). Measuring China's circular economy. Science 339 (6127), 1526–1527. doi:10.1126/science.1227059
Geng, Y., Zhang, P., Ulgiati, S., and Sarkis, J. (2010). Emergy analysis of an industrial park: the case of dalian, China. Sci. Total Environ. 408 (22), 5273–5283. doi:10.1016/j.scitotenv.2010.07.081
Geng, Y., Zhu, Q., Doberstein, B., and Fujita, T. (2009). Implementing China’s circular economy concept at the regional level: a review of progress in dalian, China. Waste Manag. 29 (2), 996–1002. doi:10.1016/j.wasman.2008.06.036
Genovese, A., Acquaye, A. A., Figueroa, A., and Koh, S. C. L. (2017). Sustainable supply chain management and the transition towards a circular economy: evidence and some applications. Omega 66, 344–357. doi:10.1016/j.omega.2015.05.015
Giannakitsidou, O., Giannikos, I., and Chondrou, A. (2020). Ranking European countries on the basis of their environmental and circular economy performance: a dea application in msw. Waste Manag. 109, 181–191. doi:10.1016/j.wasman.2020.04.055
Giorgi, S., Lavagna, M., Wang, K., Osmani, M., Liu, G., and Campioli, A. (2022). Drivers and barriers towards circular economy in the building sector: stakeholder interviews and analysis of five European countries policies and practices. J. Clean. Prod. 336, 130395. doi:10.1016/j.jclepro.2022.130395
Grossman, G. M., and Krueger, A. B. (1995). Economic growth and the environment. Q. J. Econ. 110 (2), 353–377. doi:10.2307/2118443
Gusmerotti, N. M., Testa, F., Corsini, F., Pretner, G., and Iraldo, F. (2019). Drivers and approaches to the circular economy in manufacturing firms. J. Clean. Prod. 230, 314–327. doi:10.1016/j.jclepro.2019.05.044
Hong Nham, N. T., and Ha, L. T. (2022). Making the circular economy digital or the digital economy circular? Empirical evidence from the European region. Technol. Soc. 70, 102023. doi:10.1016/j.techsoc.2022.102023
Jacobi, N., Haas, W., Wiedenhofer, D., and Mayer, A. (2018). Providing an economy-wide monitoring framework for the circular economy in austria: status quo and challenges. Resour. Conservation Recycl. 137, 156–166. doi:10.1016/j.resconrec.2018.05.022
Jayaraman, V., Ross, A. D., and Agarwal, A. (2008). Role of information technology and collaboration in reverse logistics supply chains. Int. J. Logist. Res. Appl. 11 (6), 409–425. doi:10.1080/13675560701694499
Jia, C., and Jun, Z. (2011). Evaluation of regional circular economy based on matter element analysis. Procedia Environ. Sci. 11, 637–642. doi:10.1016/j.proenv.2011.12.099
Kayal, B., Abu-Ghunmi, D., Abu-Ghunmi, L., Archenti, A., Nicolescu, M., Larkin, C., et al. (2019). An economic index for measuring firm’s circularity: the case of water industry. J. Behav. Exp. Finance 21, 123–129. doi:10.1016/j.jbef.2018.11.007
Kayikci, Y., Kazancoglu, Y., Lafci, C., and Gozacan, N. (2021). Exploring barriers to smart and sustainable circular economy: the case of an automotive eco-cluster. J. Clean. Prod. 314, 127920. doi:10.1016/j.jclepro.2021.127920
Khan, S. A., Mubarik, M. S., and Paul, S. K. (2022). Analyzing cause and effect relationships among drivers and barriers to circular economy implementation in the context of an emerging economy. J. Clean. Prod. 364, 132618. doi:10.1016/j.jclepro.2022.132618
Kirchherr, J., Reike, D., and Hekkert, M. (2017). Conceptualizing the circular economy: an analysis of 114 definitions. Resour. Conservation Recycl. 127, 221–232. doi:10.1016/j.resconrec.2017.09.005
Korhonen, J., Honkasalo, A., and Seppälä, J. (2018). Circular economy: the concept and its limitations. Ecol. Econ. 143, 37–46. doi:10.1016/j.ecolecon.2017.06.041
Krogslund, C., Choi, D. D., and Poertner, M. (2015). Fuzzy sets on shaky ground: parameter sensitivity and confirmation bias in fsqca. Polit. Anal. 23 (1), 21–41. doi:10.1093/pan/mpu016
Kumar, S., Raut, R. D., Nayal, K., Kraus, S., Yadav, V. S., and Narkhede, B. E. (2021). To identify industry 4.0 and circular economy adoption barriers in the agriculture supply chain by using ism-anp. J. Clean. Prod. 293, 126023. doi:10.1016/j.jclepro.2021.126023
Lacey, R., and Fiss, P. C. (2009). in Comparative organizational analysis across multiple levels: A set-theoretic approach. Editors B. G. King, T. Felin, and D. A. Whetten (Bingley: Emerald Group Publishing Limited), 91–116. doi:10.1108/S0733-558X(2009)0000026006
Lebreton, L., and Andrady, A. (2019). Future scenarios of global plastic waste generation and disposal. Palgrave Commun. 5 (1), 6. doi:10.1057/s41599-018-0212-7
Lehmann, C., Cruz-Jesus, F., Oliveira, T., and Damásio, B. (2022). Leveraging the circular economy: investment and innovation as drivers. J. Clean. Prod. 360, 132146. doi:10.1016/j.jclepro.2022.132146
Li, N., Ding, S., and Zhao, W. (2011). Spatial divergence and optimization of China's circular economy development. China Popul. Resour. Environ. 21 (05), 157–163. doi:10.3969/j.issn.1002-2104.2011.05.025
Li, R. H., and Su, C. H. (2012). Evaluation of the circular economy development level of Chinese chemical enterprises. Procedia Environ. Sci. 13, 1595–1601. doi:10.1016/j.proenv.2012.01.151
Li, W., Qiao, Y., Li, X., and Wang, Y. (2022). Energy consumption, pollution haven hypothesis, and environmental kuznets curve: examining the environment–economy link in belt and road initiative countries. Energy 239, 122559. doi:10.1016/j.energy.2021.122559
Liang, D., Taka, G. N., Lee, D., Park, Y., and Park, H. S. (2022). Tracking industrial symbiosis performance with ecological network approach integrating economic and environmental benefits analysis. Resour. Conservation Recycl. 185, 106454. doi:10.1016/j.resconrec.2022.106454
Lieder, M., and Rashid, A. (2016). Towards circular economy implementation: a comprehensive review in context of manufacturing industry. J. Clean. Prod. 115, 36–51. doi:10.1016/j.jclepro.2015.12.042
Lin, J. Y. (2011). New structural economics: A framework for rethinking development. World Bank Res. Observer 26 (2), 193–221. doi:10.1093/wbro/lkr007
Luo, A., and Leipold, S. (2022). Chinese lessons on upscaling environmental policy concepts? A review of policy-oriented circular economy research. J. Clean. Prod. 333, 130047. doi:10.1016/j.jclepro.2021.130047
Mahmud, S. N. D., and Osman, K. (2010). The determinants of recycling intention behavior among the malaysian school students: an application of theory of planned behaviour. Procedia - Soc. Behav. Sci. 9, 119–124. doi:10.1016/j.sbspro.2010.12.123
Mangla, S. K., Luthra, S., Mishra, N., Singh, A., Rana, N. P., Dora, M., et al. (2018). Barriers to effective circular supply chain management in a developing country context. Prod. Plan. Control 29 (6), 551–569. doi:10.1080/09537287.2018.1449265
Markard, J., Raven, R., and Truffer, B. (2012). Sustainability transitions: an emerging field of research and its prospects. Res. Policy 41 (6), 955–967. doi:10.1016/j.respol.2012.02.013
Mendel, J. M., and Korjani, M. M. (2013). Theoretical aspects of fuzzy set qualitative comparative analysis (fsqca). Inf. Sci. (N. Y). 237, 137–161. doi:10.1016/j.ins.2013.02.048
Miao, Z., Baležentis, T., Shao, S., and Chang, D. (2019). Energy use, industrial soot and vehicle exhaust pollution—China's regional air pollution recognition, performance decomposition and governance. Energy Econ. 83, 501–514. doi:10.1016/j.eneco.2019.07.002
Millhauser, J. K., and Earle, T. K. (2022). Biodiversity and the human past: lessons for conservation biology. Biol. Conserv. 272, 109599. doi:10.1016/j.biocon.2022.109599
Moriguchi, Y. (2007). Material flow indicators to measure progress toward a sound material-cycle society. J. Mat. Cycles Waste Manag. 9 (2), 112–120. doi:10.1007/s10163-007-0182-0
Neves, S. A., and Marques, A. C. (2022). Drivers and barriers in the transition from a linear economy to a circular economy. J. Clean. Prod. 341, 130865. doi:10.1016/j.jclepro.2022.130865
Nobre, G. C., and Tavares, E. (2017). Scientific literature analysis on big data and internet of things applications on circular economy: a bibliometric study. Scientometrics 111 (1), 463–492. doi:10.1007/s11192-017-2281-6
Omri, A. (2020). Technological innovation and sustainable development : does the stage of development matter? Environ. Impact Assess. Rev. 83, 106398. doi:10.1016/j.eiar.2020.106398
Pagoropoulos, A., Pigosso, D. C. A., and McAloone, T. C. (2017). The emergent role of digital technologies in the circular economy: a review. Procedia CIRP 64, 19–24. doi:10.1016/j.procir.2017.02.047
Paletta, A., Leal Filho, W., Balogun, A., Foschi, E., and Bonoli, A. (2019). Barriers and challenges to plastics valorisation in the context of a circular economy: Case studies from italy. J. Clean. Prod. 241, 118149. doi:10.1016/j.jclepro.2019.118149
Pan, Y., and Li, H. (2016). Sustainability evaluation of end-of-life vehicle recycling based on emergy analysis: a case study of an end-of-life vehicle recycling enterprise in China. J. Clean. Prod. 131, 219–227. doi:10.1016/j.jclepro.2016.05.045
Park, J. Y., and Chertow, M. R. (2014). Establishing and testing the “reuse potential” indicator for managing wastes as resources. J. Environ. Manage. 137, 45–53. doi:10.1016/j.jenvman.2013.11.053
Pasche, M. (2002). Technical progress, structural change, and the environmental kuznets curve. Ecol. Econ. 42 (3), 381–389. doi:10.1016/S0921-8009(02)00135-0
Pearce, D. W., and Turner, R. K. (1990). Economics of natural resources and the environment. Baltimore: Johns Hopkins University Press. doi:10.2307/1242904
Ragin, C. C. (1987). Moving beyond qualitative and quantitative strategies. California: University of California Press. Available at: http://www.jstor.org/stable/10.1525/j.ctt1pnx57.
Robaina, M., Villar, J., and Pereira, E. T. (2020). The determinants for a circular economy in Europe. Environ. Sci. Pollut. Res. 27 (11), 12566–12578. doi:10.1007/s11356-020-07847-9
Ryen, E., and Babbitt, C. W. (2022). The role of u.s. Policy in advancing circular economy solutions for wasted food. J. Clean. Prod. 369, 133200. doi:10.1016/j.jclepro.2022.133200
Schneider, C. Q., and Wagemann, C. (2012). Set-theoretic methods for the social sciences: a guide to qualitative comparative analysis. Cambridge: Cambridge University Press. doi:10.1017/CBO9781139004244
Schneider, P. (2019). An anatomy of the market return. J. Financial Econ. 132 (2), 325–350. doi:10.1016/j.jfineco.2018.10.015
Schwarzenbach, R. P., Egli, T., Hofstetter, T. B., von Gunten, U., and Wehrli, B. (2010). Global water pollution and human health. Annu. Rev. Environ. Resour. 35 (1), 109–136. doi:10.1146/annurev-environ-100809-125342
Shang, Y., Song, M., and Zhao, X. (2022). The development of China's circular economy: from the perspective of environmental regulation. Waste Manag. 149, 186–198. doi:10.1016/j.wasman.2022.05.027
Sharma, Y. K., Mangla, S. K., Patil, P. P., and Liu, S. (2019). When challenges impede the process: for circular economy-driven sustainability practices in food supply chain. Manag. Decis. 57 (4), 995–1017. doi:10.1108/MD-09-2018-1056
Sommerhuber, P. F., Wenker, J. L., Rüter, S., and Krause, A. (2017). Life cycle assessment of wood-plastic composites: analysing alternative materials and identifying an environmental sound end-of-life option. Resour. Conservation Recycl. 117, 235–248. doi:10.1016/j.resconrec.2016.10.012
Starr, J., and Nicolson, C. (2015). Patterns in trash: factors driving municipal recycling in massachusetts. Resour. Conservation Recycl. 99, 7–18. doi:10.1016/j.resconrec.2015.03.009
Su, B., Heshmati, A., Geng, Y., and Yu, X. (2013). A review of the circular economy in China: moving from rhetoric to implementation. J. Clean. Prod. 42, 215–227. doi:10.1016/j.jclepro.2012.11.020
Taghipour, A., Akkalatham, W., Eaknarajindawat, N., and Stefanakis, A. I. (2022). The impact of government policies and steel recycling companies' performance on sustainable management in a circular economy. Resour. Policy 77, 102663. doi:10.1016/j.resourpol.2022.102663
Tang, J., Tong, M., Sun, Y., Du, J., and Liu, N. (2020). A spatio-temporal perspective of China's industrial circular economy development. Sci. Total Environ. 706, 135754. doi:10.1016/j.scitotenv.2019.135754
Thiel, C., Nijs, W., Simoes, S., Schmidt, J., van Zyl, A., and Schmid, E. (2016). The impact of the eu car co2 regulation on the energy system and the role of electro-mobility to achieve transport decarbonisation. Energy Policy 96, 153–166. doi:10.1016/j.enpol.2016.05.043
Ullah, F., Qayyum, S., Thaheem, M. J., Al-Turjman, F., and Sepasgozar, S. M. E. (2021). Risk management in sustainable smart cities governance: a toe framework. Technol. Forecast. Soc. Change 167, 120743. doi:10.1016/j.techfore.2021.120743
Upadhyay, A., Laing, T., Kumar, V., and Dora, M. (2021). Exploring barriers and drivers to the implementation of circular economy practices in the mining industry. Resour. Policy 72, 102037. doi:10.1016/j.resourpol.2021.102037
Wang, N., Lee, J. C. K., Zhang, J., Chen, H., and Li, H. (2018). Evaluation of urban circular economy development: an empirical research of 40 cities in China. J. Clean. Prod. 180, 876–887. doi:10.1016/j.jclepro.2018.01.089
Wang, S., Lei, L., and Xing, L. (2021). Urban circular economy performance evaluation: a novel fully fuzzy data envelopment analysis with large datasets. J. Clean. Prod. 324, 129214. doi:10.1016/j.jclepro.2021.129214
Wang, Z., Jia, H., Xu, T., and Xu, C. (2018). Manufacturing industrial structure and pollutant emission: an empirical study of China. J. Clean. Prod. 197, 462–471. doi:10.1016/j.jclepro.2018.06.092
Weitzman, M. L. (1997). Sustainability and technical progress. Scand. J. Econ. 99 (1), 1–13. doi:10.1111/1467-9442.00043
Wen, Z., and Meng, X. (2015). Quantitative assessment of industrial symbiosis for the promotion of circular economy: a case study of the printed circuit boards industry in China's suzhou new district. J. Clean. Prod. 90, 211–219. doi:10.1016/j.jclepro.2014.03.041
Wright, C. Y., Godfrey, L., Armiento, G., Haywood, L. K., Inglesi-Lotz, R., Lyne, K., et al. (2019). Circular economy and environmental health in low- and middle-income countries. Glob. Health 15 (1), 65. doi:10.1186/s12992-019-0501-y
Yu, F., Han, F., and Cui, Z. (2015). Evolution of industrial symbiosis in an eco-industrial park in China. J. Clean. Prod. 87, 339–347. doi:10.1016/j.jclepro.2014.10.058
Zhou, G., Zhang, Z., and Fei, Y. (2022). How to evaluate the green and high-quality development path? An fsqca approach on the China pilot free trade zone. Int. J. Environ. Res. Public Health 19, 547. doi:10.3390/ijerph19010547
Keywords: circular economy, entropy method, empirical analysis, China, fuzzy set qualitative comparative analysis, technology-organization-environment
Citation: Zhu F, Lai L, Zhu Z and Zhang X (2022) A study on the path of improving the performance of China’s provincial circular economy—An empirical study based on the fsQCA method. Front. Environ. Sci. 10:1006170. doi: 10.3389/fenvs.2022.1006170
Received: 29 July 2022; Accepted: 24 August 2022;
Published: 12 September 2022.
Edited by:
Irfan Ullah, Nanjing University of Information Science and Technology, ChinaReviewed by:
Chenglin Li, Southwestern University of Finance and Economics, ChinaJianyi Zhong, Guangzhou Huali Science and Technology Vocational College, China
Copyright © 2022 Zhu, Lai, Zhu and Zhang. This is an open-access article distributed under the terms of the Creative Commons Attribution License (CC BY). The use, distribution or reproduction in other forums is permitted, provided the original author(s) and the copyright owner(s) are credited and that the original publication in this journal is cited, in accordance with accepted academic practice. No use, distribution or reproduction is permitted which does not comply with these terms.
*Correspondence: Liangrong Lai, bGFpbGlhbmdyb25nQHN0Lmd4dS5lZHUuY24=