- 1Business School, Jiangsu Normal University, Xuzhou, China
- 2School of Management, Hainan University, Haikou, China
- 3School of Economics and Management, Leshan Normal University, Leshan, China
In the digital economy era, as a new production factor, digital technology can break through the information blocking constraints on farmers’ behavior, become an important driving force affecting farmers’ low-carbon production behavior, and provide new opportunities for agricultural green low-carbon transformation and the realization of carbon emission peak and carbon neutrality goals. Based on the field survey data of 571 farm households in Jiangsu Province, China, this paper employs the Ordered Probit model and the mediating effect test method, and takes risk cognition as the mediating variable to empirically analyze the impact of digital technology use on farmers’ low-carbon production behavior and its path. The study results show that environmental risk cognition, health risk cognition, agricultural product safety risk cognition, and pesticide residue risk cognition have a significant positive impact on farmers’ low-carbon production behavior. Study findings also reveal that, on one side, the use of digital technology has a direct effect on the farmers’ low-carbon production behavior, on the other side, it indirectly affects the farmers’ low-carbon production behavior by affecting their risk cognition, that is, risk cognition plays a partial mediation role in this relationship. Furthermore, the results indicate that among the control variables, joint cooperative membership, food security knowledge, agricultural income, technical guidance, and following instructions have a significant and positive impact on farmers’ low-carbon production behavior. Based on study findings, the variable “number of the labor force” has a negative and significant impact on farmers’ low-carbon production behavior. Based on study findings, to effectively realize carbon emission peak and carbon neutrality goals and promote sustainable and high-quality agriculture development, agricultural policy makers should pay attention to the role of digital technology to actively promote low-carbon production behavior.
1 Introduction
In recent years, with the rapid development of the global economy, extreme climate problems caused by dioxide emissions and other greenhouse gas emissions have become increasingly serious, which seriously threaten the economic development, ecological balance and sustainable development of human society (Chen et al., 2020; Su et al., 2021a; Song et al., 2022a; Isik et al., 2022). According to the report issued by the World Meteorological Organization, the debate on global greenhouse gases is expected to increase and the global temperature is observed to be 1.2°C higher than before industrialization. Therefore, implementing carbon emission reduction to cope with climate change has become a global consensus. Around 175 countries signed the Paris Agreement to regulate global temperature within 2 C. As the world’s largest emitter of greenhouse gases, China plays a key role in carbon emission reduction. In 2020, President Xi solemnly announced at the seventy-fifth UN General Assembly that “China will augment the state’s independent involvement and adopt more effective strategies and measures, by 2030, CO2 emissions will reach the highest, while by 2060, the goal of carbon neutrality will be achieved” (Fahad et al., 2022a; Yu et al., 2021; Yang et al., 2021).
Agriculture is an important source of greenhouse gas emissions. China is the country with the highest agricultural carbon emissions in the world (Alvarado et al., 2021; Zhang et al., 2019; Zhang and Wang 2020; Fatima et al., 2022). Over the past 40 years of reform and opening up, China’s grain production has achieved “eighteen consecutive harvests”, which has made great contributions to world food security (Fahad et al., 2022b; Fahad et al., 2022c; Wang et al., 2020). However, this achievement mainly depend on the excessive use of chemical fertilizers, pesticides, and other relevant factors. This traditional mode of extensive agricultural production has produced a large amount of carbon emissions, resulting in serious ecological and environmental problems (Li et al., 2011; Hu and Wang, 2022; Su et al., 2021b; Song et al., 2022b), which directly threatens China’s food security and agricultural sustainable development (Xu et al., 2016; Chen et al., 2017; Yang et al., 2022). Therefore, under the background of carbon emission peak and carbon neutrality goals, it is urgent to change the mode of agricultural production, promote low carbon agriculture transformation and accelerate the pace of agricultural carbon emission reduction. This will be not only a specific action to implement the new development concept but also an inevitable choice to realize ecological civilization and promote agriculture high-quality and sustainable development (Yu 2018; Jin et al., 2020; Isik et al., 2021; Han and Yuan, 2022). In order to better explore the green and low-carbon transformation of agriculture, more and more scholars have begun to devote themselves to the research of agricultural carbon emissions and other related environmental issues. At the macro level, domestic and foreign scholars have mainly carried out systematic discussions on the measurement, characteristics, and influencing factors of agricultural carbon emissions (Johnson et al., 2007). Research shows that agricultural mechanization, operation scale, agricultural support policy, technology diffusion, industrial agglomeration, and other factors affect agricultural carbon emissions (Ali et al., 2021; Ismael et al., 2018).
Currently, there are 260 million farmers in China, of which 230 million are contracted farmers (Yang et al., 2021). For a long time, the concept of “large country and small farmers” has been the basic pattern of Chinese agricultural. China’s national conditions determine that ordinary farmers will remain the basic subject of agricultural production for a long time in the future (Han 2018; Hossain et al., 2022; Wang et al., 2022). Taking into account their importance, farmers are the basic subject of low-carbon agricultural production. Agriculture green transformation depends on the active participation of farmers, and promoting farmers to actively implement low carbon production behavior is of great significance to cope with climate change, improving the ecological environment, and promoting sustainable and high-quality development. The government has taken a series of effective measures to encourage farmers to reduce the input of production factors. However, in reality, farmers’ agricultural production mode is still extensive, the input of chemical fertilizers, pesticides, and other production factors is still high, which makes the effect of agricultural carbon emission reduction not ideal and threatens agriculture sustainable and high-quality development. In recent years, scholars at home and abroad have conducted a lot of research on the factors that influence farmers’ low-carbon production behavior. According to the existing research, farmers’ low-carbon production behavior is mainly affected by their own endowment characteristics, family endowment characteristics, production and management characteristics, psychological cognition and external environment (Tian et al., 2015; Fan et al., 2017; Jiang et al., 2018; Tian 2019; Pata and Isik 2021). According to the planned behavior theory, farmers’ behavior logic follows the influence path of “cognition→willingness→behavior”. Farmers’ cognition and behavior decision-making are constrained by information blocking, which makes farmers’ behavior insufficient, and makes agriculture green and low-carbon transformation in a dilemma.
At present, China is moving toward the digital economy era. Various new technologies, new products, and new business formats are constantly emerging. The digital economy has been advancing steadily in various fields and has become an important instrument for driving economic growth and a new way to drive industrial transformation. Digital empowerment has become a common feature of the new round of scientific and technological revolution that attracted extensive attention of the society (Zhang et al., 2021; Khan et al., 2022a; Su et al., 2022). Promoting the deep integration of digital technology and agricultural economy can further improve resource allocation efficiency and accelerate green and low carbon development, which is generally conducive to reducing carbon emissions (Ding 2020). With the establishment of China’s “digital village strategy”, the scale of rural Internet users is expanding. In June 2021, according to the 48th statistical report on China’s Internet Development announced by the China Internet Network Information Center, the internet users in rural China were 297 million, and the Internet penetration rate in rural areas was 59.2%. It can be seen that with the rapid development of the digital economy, digital technologies such as mobile phone and the internet have generally penetrated the daily life and agricultural production of farmers. At the same time, the digital technology represented by mobile phones has broadened the farmers’ access to information. It also effectively reduced information costs, facilitated rural farmers to obtain effective agricultural production information in time, strengthened farmers’ risk cognition, and promoted farmers’ pro-environmental behavior (Fahad and Wang, 2018; Fahad and Wang, 2019; Shi et al., 2018; Khan et al., 2022b). Digital technology can break through the restriction of information blocking on farmers’ behavior decisions, inject new power into the behavior logic of “cognition→willingness→behavior”, become an important driving force affecting farmers’ low-carbon production behavior, and provide new opportunities for agricultural green and low-carbon transformation.
Throughout the existing studies, the following questions have not been answered.1) Under the background of carbon emission peak and carbon neutrality goals, how does digital technology use affect farmers’ low carbon production behavior? 2) Does the use of digital technology affect farmers’ low-carbon production behavior through risk cognition? 3) What policies and measures should be designed to promote farmers’ low-carbon production behavior? In order to answer these questions, this paper builds a theoretical framework of the relationship between digital technology use and farmers’ low-carbon production behavior. Based on the field survey data of 571 farm households in Jiangsu Province, China, this paper employs the ordered Probit model and the mediating effect test method, and takes risk cognition as the mediating variable to empirically analyze the impact of digital technology use on farmers’ low-carbon production behavior and its path. This research is unique and contributes to academia by answering the above questions. The innovation and contribution of this paper are as follows: 1) Bring the use of digital technology and farmers’ low-carbon production behavior into the same analysis framework for empirical analysis and reveal its impact on farmers’ low-carbon production behavior and its mechanism; 2) Test the use of digital technology affects farmers’ low-carbon production behavior through the impact mechanism of risk cognition, that is, to test the mediating effect of risk cognition; 3) From the perspective of digital technology, put forward policy suggestions to promote farmers’ low-carbon production behavior. This research fills the existing research and entices academic attention to this unique issue. This paper studies the impact and mechanism of digital technology use on farmers’ low-carbon production behavior from both theoretical and empirical perspectives, which has important theoretical and practical significance. It can supplement the research content of farmer behavior theory. It can provide a path choice for realizing the green and low-carbon transformation of agriculture under the background of carbon emission peak and carbon neutrality goals. It can provide practical guidance for rural digital transformation under the background of digital economy.
The remainder of this study is ordered as follows: The relevant theoretical framework is discussed in Section 2. Section 3 specifies the methodology and model specification, while Section 4 provides empirical results and discussion. Section 5 provides concludes the study and discusses limitations and propose the policy implications.
2 Theoretical framework and research hypothesis
2.1 Impact of digital technology use on farm households’ low carbon production behavior
Digital technology has the advantages of high growth, wide coverage, strong permeability, cross-border integration, and intelligent sharing. It plays an important role in spreading rural ecological civilization and affecting farmers’ environmental behavior (Jin and Bian 2015). Agricultural digitization can guide the rational allocation and flow of fertilizer and effectively reduce the environmental pollution caused by the use of nitrogen fertilizer (Mei 2001). The Internet provides an information platform for farmers’ production activities. Farmers can timely obtain information about crops, seeds, chemical fertilizers, pesticides, disease prevention and control through mobile phone apps/software, which can help farmers reasonably plan their agricultural production activities, optimize factor input, and reduce excessive use of pesticides (Mittal and Tripathi 2009; Zhang and Zhang 2020). By watching the video of agricultural production activities on mobile phones, farmers will learn the operation specifications of pesticide reduction, guide farmers to standardize the application behavior, and achieve the purpose of pesticide reduction (Suchiradipta and Raj 2018; Wyckhuys et al., 2018). Farmers who can effectively use Internet resources have stronger safety production capacity and are more likely to reduce pesticide application (Goncalves et al., 2018). It can be noticed that the use of digital technology can change the behavior of farmers, reduce pesticide application, promote farmers’ low carbon production behavior and reduce agricultural carbon emissions. Through digital technology, farmers can know the country’s carbon emission peak and carbon neutrality goals and believe that they have the responsibility to adopt low-carbon production behavior and reduce agricultural carbon emissions. Based on the above, the following research hypothesis is constructed:
Hypothesis (H1): the use of digital technology has a significant positive impact on farmers’ low-carbon production behavior.
2.2 Impact of the use of digital technology on farmers’ risk cognition
In general, digital technology has the characteristics of universality, permeability, and innovation. It can spread information about the ecological environment to all farmers and constantly change the cognition of farmers about the ecological environment. With the use of the Internet, farmers not only improve their cognition of relevant agricultural information, but also change their conventional ideas, formulate farming strategies, and form a modern agricultural production literacy (Cole and Fernando 2012). Information on agricultural pollution and food safety through Internet sources will encourage farmers’ emotional reverberation and catastrophe consciousness, animate farmers’ green production sense of responsibility, shape green production consciousness, and improve their risk cognition (Monica et al., 2019; Peng et al., 2019). It can also be noticed that digital technology not only broadens farmers’ information acquisition channels but also improves farmers’ information acquisition ability, so that they have more scientific knowledge and risk cognition. Through digital technology, farmers can watch videos of disasters caused by climate change. Farmers can intuitively feel the harm of carbon emissions, which will encourage farmers to adopt low-carbon production behavior and reduce agricultural carbon emissions. Based on the above following hypothesis is proposed:
Hypothesis (H2): The use of digital technology has a significant positive impact on farmers’ risk cognition.
2.3 Impact of risk cognition on farmers’ low-carbon production behavior
Risk cognition includes environmental risk cognition, health risk cognition, agricultural product safety risk cognition, and pesticide residue cognition. Farmers who do not know the harm of pesticide residues will unreasonable application of pesticides and random treatment of pesticide packaging (Jetiyanon and Wittaya-areekul 2009). Farmers who are conscious about food safety pay proper attention to their production behavior and avoid using pesticides with high toxicity and high residue (Zhou and Xu, 2008). Psychological cognition has a significant impact on farmers’ rational application of pesticides. Farmers who understand the harm of carbon emissions can increase their risk cognition level and promote them to adopt low-carbon production behavior. The following research hypothesis is constructed accordingly:
Hypothesis (H3): Risk cognition and its different dimensions have a significant positive impact on farmers’ low-carbon agricultural production behavior.
2.4 Mediating effect of the risk cognition
Farmers will obtain a large amount of carbon emission reduction information through the Internet, which will increase farmers’ knowledge and thus promote farmers to reduce pesticide application (Zhao 2021). Farmers’ cognition has a partial mediating effect in the impact of information utilization on farmers’ adoption of Integrated Pest Management (IPM) technology (Yan et al., 2020). Based on the above analysis and from the perspective of causal logic, the use of digital technology can affect farmers’ low-carbon production behavior by affecting farmers’ risk cognition. Farmers can obtain a large amount of carbon emission information through digital technology, which can improve farmers’ risk cognition level, and then promote farmers’ low-carbon production behavior. Therefore, the following hypothesis is proposed:
Hypothesis (H4): Risk cognition and its different dimensions play a mediating effect in the relationship between the use of digital technology and farmers’ low-carbon production behavior.
Consequently, this paper constructs a model including the impact of digital technology use and risk cognition on farmers’ low-carbon production behavior (as shown in Figure 1).
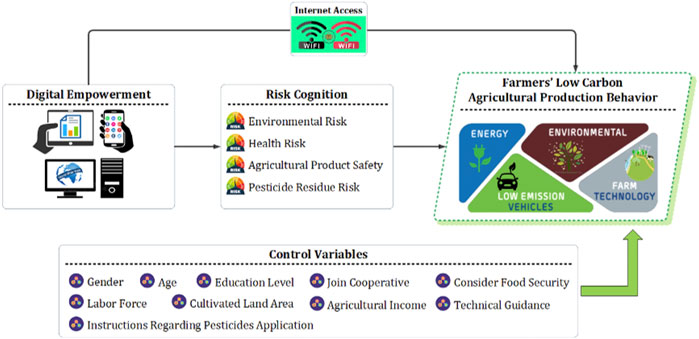
FIGURE 1. Impact mechanism of digital technology use and risk cognition on farmers’ low-carbon production behavior.
3 Materials and methods
3.1 Data sources
A household survey was conducted during September to November 2021 in rural villages in Xuzhou and Yangzhou Jiangsu province. Primary data farmers were collected using the stratified random sampling method, for which a structured questionnaire was used. In the first phase, Xuzhou and Yangzhou were purposely chosen, and in the second phase, 2-4 districts were randomly selected from each city. In the third phase, 1-2 towns randomly chosen from each county (district), and in the fourth phase, 4-5 villages were selected from each town, and finally 12–16 farmers were targeted for a households survey from each village. A total of 580 questionnaires were distributed, and after sorting of missing questionnaires, 571 valid questionnaires were obtained with an effective rate of 98.45% (as shown in Figure 2). The questionnaire consisted of questions regarding the basic characteristics of farmers and their families, farmers’ risk cognition, low-carbon production behavior, and the use of digital technology. The socioeconomic characteristics of the respondents are presented in Table 1 and Figure 3. According to the household survey, 73.91% of the farmers sampled were over 50 years old, which shows that the aging of the farmers involved in agricultural production is obvious. The education level of the surveyed farmers is generally low, 86.51% of the sampled farmers had junior middle school, and below education. Men accounted for 55.87% of the total sampled farmers. Families with a total income of 20000–50000 accounted for 40.63% of the total sample. The research sample basically conforms to the current rural reality.
The main grain producing areas are important agricultural areas in China, which play the most important supporting role for the national food security. Agricultural carbon emissions in the main grain producing areas are relatively high. Therefore, the main grain producing areas have great potential for carbon emission reduction. It is of great significance to study farmers’ low-carbon production behavior in main grain producing areas. Jiangsu is an important grain production province in China, and its agricultural production value and agricultural modernization level are in the forefront of the country. Therefore, Jiangsu province is selected as the research area; Xuzhou and Yangzhou cities are accelerating the construction of digital villages. They are key demonstration areas for agricultural green development and pilot areas for digital village construction. It is of practical significance to study the impact of digital technology use on farmers’ low-carbon production behavior in this area. Therefore, Xuzhou City and Yangzhou city are selected as the research sites, which are representative.
3.2 Variables selection
Dependent variable: In this paper, the low carbon agricultural production behavior of farmers is used as a dependent variable. China is the largest producer and consumer of pesticides in the world. The pesticide is overused, and the effective utilization rate is less than 35%. The excessive application of pesticides is an important source of agricultural carbon emissions. Therefore, this paper takes pesticide reduction application as an example to study farmers’ low-carbon production behavior. The question is: In recent years, the amount of pesticide application per hectare in your family is decreasing?
Core explanatory variables: Digital technology use is used as core explanatory variable. The question is: whether farmers obtain agricultural related information through digital technologies such as mobile phones, such as WeChat group, app or web browsing. When farmers use one of the platforms to obtain agricultural related information, the value is 1, otherwise it is 0.
Mediation variables (risk cognition): Excessive use of pesticides can cause serious damage to the environment (soil, water, atmosphere, ecological balance, etc.), produce a lot of carbon emissions, damage to farmers’ own health, lead to excessive residues of agricultural products, and seriously threaten the quality and safety of agricultural products. This paper comprehensively focused on the farmers’ risk cognition (e.g., farmers’ environmental risk cognition, health risk cognition, agricultural product safety risk cognition, and pesticide residue risk cognition) as a mediator variable.
Control variables: Following the existing relevant literature, gender, age, level of education, joint cooperative membership, food security cognition, number of the labor force, agricultural income, cultivated land area, technical guidance were used as control variables in this study. Table 2 shows the descriptive statistics of the variables used in this study.
3.3 Model specification
3.3.1 Benchmark regression model
The hierarchical five-likert scale technique was used to evaluate farmers’ pesticide reduction application behavior. Farmers’ pesticide reduction application behavior was ranked from 1 to 5, where 1 = strongly disagree, 2 = not quite agree,3 = general, 4 = relatively agree,5 = strongly agree. For this kind of discrete variable, probability model is usually used for estimation. As the discrete variable of farmers’ pesticide reduction application behavior has exceeded two categories and is in an orderly state, so, ordered Probit model approach was used to estimate farmers’ pesticide reduction application behavior. The basic form of the Ordered Probit model is as follows:
The selection rule is:
Assuming
In Eq. 1,
3.3.2 Mediating effect model
In the field of psychology and other social sciences, a large number of empirical articles have established mediating effect models to analyze the influence process and mechanism of independent variables on dependent variables. Among them, the most representative is the mediating effect test proposed by Wen et al. (2004). This paper uses this method to verify that risk cognition plays an intermediary role in the relationship between digital technology use and farmers’ low-carbon production behavior. The details are as follows:
In the above equations, X represents digital technology use;
4 Results and discussion
4.1 Impact of digital technology use
In Table 3, the results of Model 1 and 2 revealed that the use of digital technology has a positive and significant impact on farmers’ low-carbon production behavior. This indicates that with the rapid development of digitization and the popularization of the Internet networks, digital networks have become the main information sources of farmers. Through the digital network platform, farmers will receive more and more professional pesticide-related information and understand that excessive application of pesticides leads to increased carbon emissions and climatic and environmental problems. In this regard, farmers will carry out agricultural low-carbon production and reduce the usage of pesticides and hence it verifies the Hypothesis (H1). In Table 3, the use of digital technology has a significant and positive impact (at a 1% significance level) on the environmental risk cognition of farmers, agricultural product safety risk cognition, pesticide residue risk cognition, the use of digital technology has a significant and positive impact (at a 5% significance level) on health risk cognition, which verifies Hypothesis H2. The dissemination and education function of digital technology can increase the knowledge level of farmers, improve risk cognition.
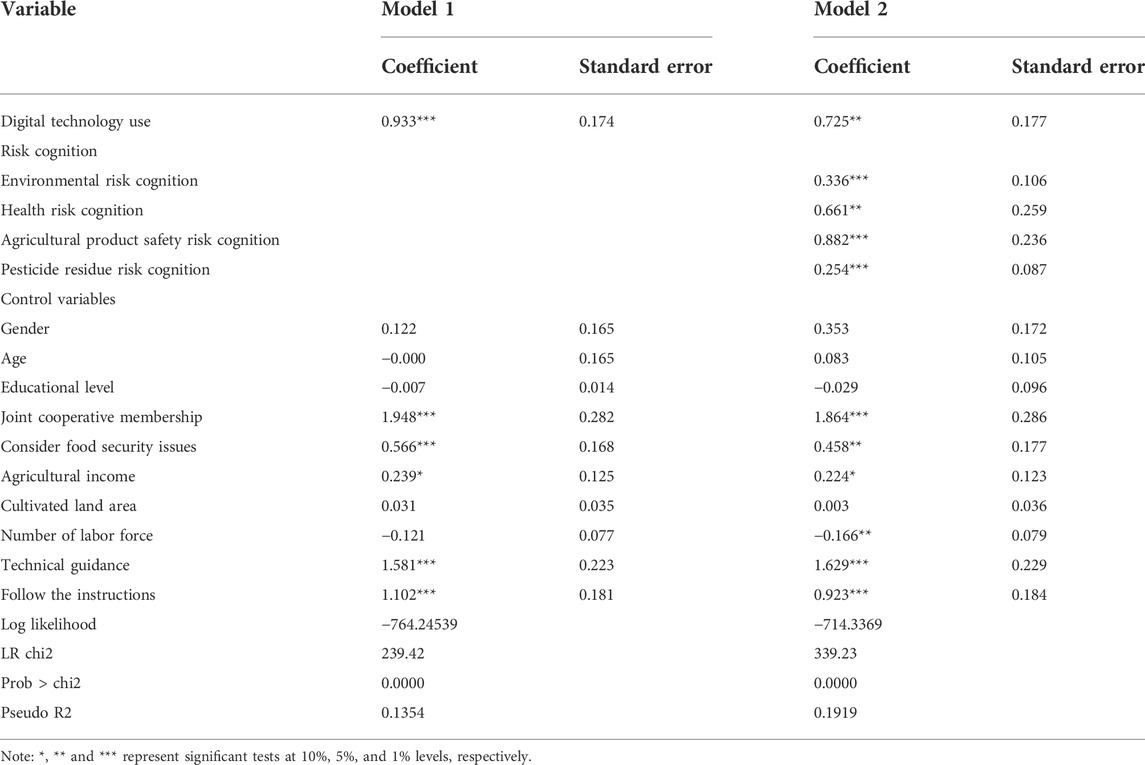
TABLE 3. Impact of digital technology use and risk cognition on farmers’ pesticide reduction behavior.
4.2 Impact of risk cognition
The results show that environmental risk cognition has a positive and significant impact on farmers’ low-carbon production behavior. If farmers assume that excessive pesticide use has an adverse effect on the environment, they are more likely to reduce the amount of pesticide use, consequently reducing agricultural carbon emissions. According to the findings, health risk cognition also showed a positive and significant relationship with farmers’ pesticide reduction behavior (at 5% significance level), indicating that when farmers think excessive spraying of pesticides has adverse impacts on their health, they are more likely to reduce pesticide use of pesticides. Likewise, agricultural product safety risk cognition showed a positive and significant association with farmers’ pesticide reduction behavior, when farmers assume that excessive pesticide use has an adverse impact on the safety of their agricultural product income and pesticide residues in agricultural products, they are more likely to avoid pesticide use. Similarly, risk cognition of pesticide residues also revealed a positive and significant relationship with farmers’ pesticide reduction behavior, indicating that When farmers are worried that the pesticide residues in agricultural products are too high and affect their income, they are more likely to reduce pesticide usage, so that hypothesis (H3) is verified here.
4.3 Mediating effect of risk cognition in the relationship between the use of digital technology and farmers’ low-carbon agricultural production behavior
According to the results of model 1 in Table 3, the impact of digital technology use on farmers’ low-carbon agricultural production behavior shows significant at a level of 1% with coefficient 0.933. It can be seen from the results in Tables 3, 4, that the use of digital technology has a significant and positive impact on risk cognition. According to the results of model 2 in Table 3, after the introduction of risk cognition, the impact of risk cognition on the pesticide reduction behavior of farmers showed a significantly positive relationship. At the same time, the impact of digital technology use on farmers’ pesticide reduction behavior still shows a significantly positive association, but the coefficient is reduced to 0.725. Therefore, risk cognition plays the role of a mediator in the relationship between digital technology use and farmers’ pesticide reduction behavior, which verifies hypothesis (H4). With the popularity of the Internet, the Internet has become one of the main channels for farmers to obtain relevant agricultural information and knowledge. This led farmers to understand that the excessive use of pesticides will increase carbon emissions and cause climatic and environmental problems. Farm households will also realize that the excessive application of pesticides will lead to pesticide residues, affect the safety of agricultural products, affect their health, and increase the levels of farmers’ risk cognition. This will promote farmers’ low-carbon production behavior.
4.4 Impact of control variable
Among control variables, joint cooperative membership has a positive and significant effect on farmers’ low-carbon agricultural production behavior. In practice, cooperatives provide standardized technical guidance and training services to their members through technical service teams, invited agricultural experts, and agricultural technology extension personnel, which promote farmers’ pesticide reduction behavior (Cai et al., 2019). Farmers considering food security showed a positive and significant association with farmers’ pesticide reduction behavior, indicating that farmers who are more aware about their food security are more likely to reduce the use of pesticide. Based on the findings of the study, agricultural income has shown a positive relationship with farmers’ pesticide reduction behavior at a significance level of 1%. This indicates that the higher the agricultural income, the more farmers rely on agricultural production and the more they can realize the losses due to the excessive use of pesticides. Therefore, farmers are more likely to reduce the use of pesticides. Likewise, the variable number of labor force has revealed a negative but significant impact on the farmers’ pesticide reduction behavior, pointing that the higher the number of labor force, the more they rely on non-agricultural income and pay less attention to the agricultural production activities. Therefore, farmers are unlikely to reduce pesticide application. Technical guidance from extension services has shown a positive and significant impact on farmers’ pesticide reduction behavior, showing that farmers with more access to extension sources obtained guidance from agricultural extension services are more likely to receive a reduction in pesticide reduction behavior. The more they know about pesticide residues, the more they know about pesticide usage norms, and the more likely they are to reduce pesticide usage. Farmers who have received training on pesticide usage are more likely to choose environmentally friendly pesticide application behavior (Li and Guan, 2013). This also reflects the importance of training in pesticide use in rational application. Results further showed a positive and significant association with farmers’ pesticide reduction behavior, indicating that the instructions regarding the use of pesticides through scientific sources led farmers to more likely reduce the use of pesticides. Relevant research shows that the size of the farm has a very important influence on the adoption of eco-friendly fertilization technology adoption. However, the regression results of this paper show that the land scale did not pass the significance test. The possible explanation is that the sample farmers’ land management scale is small, they may rely more on non-agricultural employment income, pay little attention to agricultural production, and adopt low-carbon production behavior which has no scale economy effect, which inhibits farmers’ low-carbon production behavior.
5 Conclusions and policy implications
5.1 Conclusions
Based on the field survey data of 571 farm households in China, and employs the Ordered Probit model and the mediating effect test method, this study empirically analyzes the impact of digital technology use on farmers’ pesticide reduction behavior by taking risk cognition as mediating variable. Based on the findings of the study, the following are the concluding points. Environmental risk cognition, health risk cognition, agricultural product safety risk cognition, and pesticide residue risk cognition have shown a significant and positive affect on farmers’ pesticide reduction behavior. The use of digital technology has a direct impact on farmers’ pesticide reduction behavior, while it indirectly affects farmers’ pesticide reduction behavior by influencing farmers’ risk cognition, which indicates that risk cognition plays a part of the mediating effect in this influence relationship. Among the control variables, joining cooperatives, considering food security, agricultural income, technical guidance, and following the instructions have shown positive and significant impact on the farmers’ pesticide reduction behavior. Furthermore, the number of labor force showed a negative and significant impact on farmers’ pesticide reduction behavior.
5.2 Policy implications
Based on the results of our study and the above conclusion, the following policy recommendations are proposed.
a. Governments and other authorities should formulate relevant policies to support digital development in rural areas, accelerate the construction of digital technology infrastructure, improve the level of rural digitization, and improve the availability of digital infrastructure for farm households. Improve the digital literacy of farmers and promote the development of low-carbon agriculture through digitization. Promote the rapid transformation of the mode of agricultural development by means of digital technology, in order to further promote the green and low carbon transformation and sustainable development of agriculture.
b. In the promotion of the current rural digital strategy, it is necessary to pay attention to the role of digital technology in the education of farmers’ ecological knowledge, make full use of the education and communication function of digital technology to disseminate ecological knowledge to farmers, improve farmers’ awareness of environmental protection and ecological risk cognition, enhance farmers’ pesticide residue hazards cognition, and enhance farmers’ skills in scientific and safe use of pesticides, so as to promote farmers’ pesticide reduction behavior.
c. Governments and responsible authorities should provide farmers with pesticide guidance and technical training about pesticide usage, which will improve their cognitive level, prevent excessive pesticide application, reduce uncertain behavior in pesticide usage, and fundamentally reduce agricultural carbon emissions.
d. We should explore and build a sound policy system to support the development of cooperatives, guide the healthy development of cooperatives, promote cooperatives to strengthen service guidance and training for farmers, and drive farmers’ low-carbon production behavior.
This study has few limitations as follows: First, due to limited time and funds, we only selected 571 farm households in Jiangsu province as the research object, which could not reflect the general situation of low-carbon agricultural production behavior in China. Due to the limited objective conditions, we used only 1 year data, that is, cross-sectional data of the peasant household survey, which cannot reflect the dynamic change process of the peasant household’s low-carbon agricultural production behavior. Therefore, future research should focus on expanding the research area and research objects and enhance the representativeness and persuasion of research conclusions. We should also use the tracking survey data for many years to investigate the dynamic change trend of farmers’ low-carbon production behavior.
Second, digital technology use can be affected by the personal characteristics of farmers, and it is a kind of self-selection behavior. Therefore, there may be endogenous problems. However, there is no appropriate tool variable in the questionnaire, so this problem is not well solved, which is also a defect of the paper. It will be improved in future research. With the continuous development of digital services, the digital literacy and digital skills of farmers are constantly improving, and the participation of farmers in digital production, digital logistics, digital marketing, digital finance, digital life and digital rural governance is getting higher and higher. Therefore, in the future, we should measure digital technology with a diversified indicator system. Low carbon production behaviors include chemical fertilizer reduction application, pesticide reduction application, conservation tillage technology, soil testing formula fertilizer technology, green pest prevention and control technology, water-saving irrigation technology, straw returning to the field, agricultural film recycling and so on. In this paper, the low-carbon production behavior expressed by pesticide reduction application has certain limitations. In the future, the low-carbon production behavior of farmers should be investigated from multiple dimensions.
Data availability statement
The original contributions presented in the study are included in the article/Supplementary Material, further inquiries can be directed to the corresponding author.
Author contributions
XH: Writing—original draft. FY Conceptualization, Supervision. SF Writing—review and editing and Data curation, Visualization, Investigation.
Funding
This research study is supported by the National Natural Science Foundation of China Grant No. (72203080); National Natural Science Foundation of China (NSFC) “Research Fund for International Young Scientists” Grant No. (72250410374); Jiangsu University Natural Science Research Project Grant No. (21KJB630006); Jiangsu University Philosophy and Social Science Research Project Grant No. (2022SJYB1145); General Project of Key R&D Plan (Soft Science) in Shandong Province Grant No. (2021RKY07130).
Conflict of interest
The authors declare that the research was conducted in the absence of any commercial or financial relationships that could be construed as a potential conflict of interest.
Publisher’s note
All claims expressed in this article are solely those of the authors and do not necessarily represent those of their affiliated organizations, or those of the publisher, the editors and the reviewers. Any product that may be evaluated in this article, or claim that may be made by its manufacturer, is not guaranteed or endorsed by the publisher.
References
Ali, S., Yan, Q., Hussain, M. S., Irfan, M., Ahmad, M., Razzaq, A., et al. (2021). Evaluating green technology strategies for the sustainable development of solar power projects: Evidence from Pakistan. Sustainability 13, 12997. doi:10.3390/su132312997
Alvarado, R., Tillaguango, B., Dagar, V., Ahmad, M., Isik, C., Mendez, P., et al. (2021). Ecological footprint, economic complexity and natural resources rents in Latin America: Empirical evidence using quantile regressions. J. Clean. Prod. 318, 128585. doi:10.1016/j.jclepro.2021.128585
Cai, R., Wang, Z., Qian, L., and Du, Z. (2019). Do cooperatives promote family farms to choose environmental-friendly production practices? An empirical analysis of fertilizers and pesticides reduction. China Rural. Surv. 1, 51–65. Available at: https://kns.cnki.net/kcms/detail/11.3586.f.20190117.1620.008.html.
Chen, R., He, Y., Zhu, S., and Xu, J. (2020). Duality of agricultural carbon emissions and coordination with economic development in China. Soft Sci. 234-1, 132–138. doi:10.13956/j.ss.1001-8409.2020.01.21
Chen, Y., Wen, X., Wang, B., and Nie, P. (2017). Agricultural pollution and regulation: How to subsidize agriculture. J. Clean. Prod. 164, 258–264. doi:10.1016/j.jclepro.2017.06.216
Cole, S., and Fernando, A. (2012). The value of advice: Evidence from mobile phone-based agricultural extension. Harv. Bus. Sch. Work. Pap., 13–47. doi:10.2139/ssrn.2179008
Ding, Z. (2020). Research on the mechanism of digital economy driving high-quality economic development: A theoretical analysis framework. Mod. Econ. Res. 04, 85–92. doi:10.13891/j.cnki.mer.2020.01.011
Fahad, S., Dong, B., Liu, L., and Dagar, V. (2022a). Comprehending the environmental regulation, biased policies and ofdi reverse technology spillover effects: A contingent and dynamic perspective. Environ. Sci. Pollut. Res. 29, 33167–33179. doi:10.1007/s11356-021-17450-1
Fahad, S., and Wang, J. (2018). Evaluation of Pakistani farmers’ willingness to pay for crop insurance using contingent valuation method: The case of Khyber Pakhtunkhwa province. Land Use Policy 72, 570–577. doi:10.1016/j.landusepol.2017.12.024
Fahad, S., and Wang, J. (2019). Farmers’ risk perception, vulnerability, and adaptation to climate change in rural Pakistan. Land Use Policy 79, 301–309. doi:10.1016/j.landusepol.2018.08.018
Fahad, S., Dong, B., Liu, L., and Baloch, Z. A. (2022b). Heterogeneous impacts of environmental regulation on foreign direct investment: Do environmental regulation affect FDI decisions? Environ. Sci. Pollut. Res. 29, 5092–5104. doi:10.1007/s11356-021-15277-4
Fahad, S., Faisal, A., Su, F., and Deng, J. (2022c). Adoption of green innovation practices in SMEs sector: Evidence from an emerging economy. Econ. Research-Ekonomska Istraživanja 35, 5486–5501. doi:10.1080/1331677X.2022.2029713
Fan, X., Zang, J., Wang, H., and Liu, M. (2017). Influence of household endowment on farmers' low-carbon agricultural production behavior- based on investigation in dasheng town, shandong province. Res. Soil Water Conservation 24-1, 265–271. doi:10.13869/j.cnki.rswc.2017.01.035
Fatima, N., Li, Y., Li, X., Abbas, W., Jabeen, G., Zahra, T., et al. (2022). Households’ perception and environmentally friendly technology adoption: Implications for energy efficiency. Front. Energy Res. 10, 830286. doi:10.3389/fenrg.2022.830286
Goncalves, G., Oliveira, T., and Cruz-Jesus, F. (2018). Understanding individual-level digital divide: Evidence of an African country. Comput. Hum. Behav. 87, 276–291. doi:10.1016/j.chb.2018.05.039
Han, C. (2018). Actively promote the organic connection between small farmers and the development of modern agriculture. Agric. Eng. Technol. 38 (3), 8–10. CNKI:SUN:NSGJ.0.2018-03-002.
Han, M., Yuan, Q., Fahad, S., and Ma, T. (2022). Dynamic evaluation of green development level of ASEAN region and its spatio-temporal patterns. J. Clean. Prod. 362, 132402. doi:10.1016/j.jclepro.2022.132402
Hossain, S., Alam, G. M., Fahad, S., Sarker, T., Moniruzzaman, M., and Rabbany, M. G. (2022). Smallholder farmers’ willingness to pay for flood insurance as climate change adaptation strategy in northern Bangladesh. J. Clean. Prod. 338, 130584. doi:10.1016/j.jclepro.2022.130584
Hu, G. Y., Wang, J., Fahad, S., and Li, J. (2022). Influencing factors of farmers’ land transfer, subjective well-being, and participation in agri-environment schemes in environmentally fragile areas of China. Environ. Sci. Pollut. Res. Int. doi:10.1007/s11356-022-22537-4
Isik, C., Aydın, E., Dogru, T., Rehman, A., Sirakaya-Turk, E., and Karagöz, D. (2022). Innovation research in tourism and hospitality field: A bibliometric and visualization analysis. Sustainability 14, 7889. doi:10.3390/su14137889
Isik, C., Ongan, S., Bulut, U., Karakaya, S., Irfan, M., Alvarado, A., et al. (2021). Reinvestigating the Environmental Kuznets Curve (EKC) hypothesis by a composite model constructed on the Armey curve hypothesis with government spending for the US States. Environ. Sci. Pollut. Res. 29, 16472–16483. doi:10.1007/s11356-021-16720-2
Ismael, M., Srouji, F., and Boutabba, M. A. (2018). Agricultural technologies and carbon emissions: Evidence from Jordanian economy. Environ. Sci. Pollut. Res. 25 (11), 10867–10877. doi:10.1007/s11356-018-1327-5
Jetiyanon, K., and Wittaya-areekul, S. (2009). Pesticide use patterns among small-scale farmers: A case study form phitsanulok, Thailand. Southeast Asian J. Trop. Med. Public Health 2, 401–410. doi:10.1097/OLQ.0b013e31819cd800
Jiang, L., Zhang, L., Zhang, J., and Wang, H. (2018). The influence mechanism of rice farmers' low-carbon production behaviors: An analysis based on in-depth interviews with 102 rice farmers in hubei province. China Rural. Surv. 4, 86–101. Available at: https://kns.cnki.net/kcms/detail/11.3586.f.20180712.1520.014.html.
Jin, M., and Bian, T. (2015). The role of mass media in the dissemination of rural ecological civilization and Countermeasures. Dongyue Trib. 36 (11), 179–183. doi:10.15981/j.cnki.dongyueluncong.2015.11.029
Jin, S., Niu, K., and Han, D. (2020). The path of agricultural green development and its orientation in the 14th five- year plan period. Reform 2, 30–39. doi:10.22617/BRF210192-2
Johnson, J. M. F., Franzluebbers, A. J., Weyers, S. L., and Reicosky, D. C. (2007). Agricultural opportunities to mitigate greenhouse gas emissions. Environ. Pollut. 150 (1), 107–124. doi:10.1016/j.envpol.2007.06.030
Khan, I., Zakari, A., Dagar, V., and Singh, S. (2022a). World energy trilemma and transformative energy developments as determinants of economic growth amid environmental sustainability. Energy Econ. 108, 105884. doi:10.1016/j.eneco.2022.105884
Khan, I., Zakari, A., Zhang, J., Dagar, V., and Singh, S. (2022b). A study of trilemma energy balance, clean energy transitions, and economic expansion in the midst of environmental sustainability: New insights from three trilemma leadership. Energy 248, 123619. doi:10.1016/j.energy.2022.123619
Li, B., Zhang, J., and Li, H. (2011). Research on spatial-temporal characteristics and affecting factors decomposition of agricultural carbon emission in China. China Popul. Resour. Environ. 21 (8), 80–86. doi:10.4028/www.scientific.net/AMM.291-294.1385
Li, X., and Guan, Z. (2013). Analysis on the safety behavior in pesticide application and its influencing factors: A case study of anxi tea farmers. Fujian J. Xidian Univ. Soc. Sci. Ed. 23 (6), 84–93. doi:10.16348/j.cnki.cn61-1336/c.2013.06.003
Mei, F. (2001). Strategic analysis of agricultural informatization driving agricultural modernization. Chin. Rural. Econ. 12, 22–26. Available at: https://kns.cnki.net/kcms/detail/detail.aspx?FileName=ZNJJ200112003&DbName=CJFQ2001.
Mittal, S., and Tripathi, G. (2009). Role of mobile phone technology in improving small farm productivity. Agric. Econ. Res. Rev. 22, 451–459. doi:10.22004/ag.econ.57502
Monica, K. K., Alawy, A., Allen, C., Subharwal, M., Jadhav, A., and Parr, M. (2019). Effectiveness of mobile agri-advisory service extension model. World Dev. Perspect. 13, 25–33. doi:10.1016/j.wdp.2019.02.007
Pata, U. K., and Isik, C. (2021). Determinants of the load capacity factor in China: A novel dynamic ardl approach for ecological footprint accounting. Resour. Policy 74, 102313. doi:10.1016/j.resourpol.2021.102313
Peng, D., Li, Y., and Li, C. (2019). The influence of internet use on environmental attitude and literacy. Finance Econ. 8, 97–109. 10.1000-8306(2019)06-0097-13.
Shi, Z., Jin, R., Mu, H., and Qin, L. (2018). Study on farmers' pro-environment behavior based on the perspective of media education function. J. Arid Land Resour. Environ. 10, 76–81. doi:10.13448/j.cnki.jalre.2018.303
Song, J., Peng, R., Qian, L., Yan, F., Ozturk, I., and Fahad, S. (2022a). Households production factor mismatches and relative poverty nexus: A novel approach. Pol. J. Environ. Stud. 31, 3797–3807. doi:10.15244/pjoes/146987
Song, J., Geng, L., Fahad, S., and Liu, L. (2022b). Fiscal decentralization and economic growth revisited: An empirical analysis of poverty governance. Environ. Sci. Pollut. Res. 29, 28020–28030. doi:10.1007/s11356-021-18470-7
Su, F., Liang, X., Cai, S., Chen, S., and Fahad, S. (2021b). Assessment of parent-subsidiary companies’ geographical distance effect on corporate social responsibility: A case of A-share listed companies. Econ. Research-Ekonomska Istraživanja 35, 4922–4946. doi:10.1080/1331677X.2021.2019597
Su, F., Song, N., and Shang, H. (2022). Research on the impact of economic policy uncertainty on corporate social responsibility: A case of A-share listed companies as an example. PLOS One. doi:10.1371/journal.pone.026916s
Su, F., Song, N., Ma, N., Sultanaliev, A., Ma, J., Xue, B., et al. (2021a). An assessment of poverty alleviation measures and sustainable livelihood capability of farm households in rural China: A sustainable livelihood approach. Agriculture 11, 1230. doi:10.3390/agriculture11121230
Suchiradipta, B., and Raj, S. (2018). The online culture of agriculture: Exploring social media readiness of agricultural professionals. CSI Trans. ICT 6 (3-4), 289–299. doi:10.1007/s40012-018-0205-0
Tian, Y. (2019). Cognition Degree, future expectation and farmers' low-carbon willingness in agricultural production: Based on the survey data of farmers in Wuhan. J. Huazhong Agric. Univ. Soc. Sci. Ed. 1, 77–84+166. doi:10.13300/j.cnki.hnwkxb.2019.01.009
Tian, Y., Zhang, J., He, K., and Feng, J. (2015). Analysis on Farmers' agricultural low-carbon production behavior and its influencing factors - taking the application of chemical fertilizer and pesticide as an example. China Rural. Surv. 4, 61–70. Available at: https://kns.cnki.net/kcms/detail/detail.aspx?FileName=ZNCG201504008&DbName=CJFQ2015.
Wang, W., Rahman, M. A., and Fahad, S. (2022). The dynamic influence of renewable energy, trade openness, and industrialization on the sustainable environment in G-7 economies. Renew. Energy 198, 484–491. doi:10.1016/j.renene.2022.08.067
Wang, X., He, Y., and Jiang, H. (2020). China's food security during the 14th five-year plan period: Situation, problems and countermeasures. Reform 9, 27–39. doi:10.40000/chinaperspectives.819
Wen, Z., Chang, L., and HauLiu, H. (2004). Esting and application of the mediating effects. Acta Psychol. Sin. 5, 614–620. Available at: https://kns.cnki.net/kcms/detail/detail.aspx?FileName=XLXB200405016&DbName=CJFQ2004.
Wyckhuys, K., Bentley, J., Lie, R., Nghiem, L. T. P., and Fredrix, M. (2018). Maximizing farm-level uptake and diffusion of biological control innovations in today's digital era. Biocontrol (Dordr). 63 (1), 133–148. doi:10.1007/s10526-017-9820-1
Xu, Z., Zhang, J., and Qiu, H. (2016). Effects of reputation demands on farmers' pro-environmental behavior: Taking the farmers' disposal behavior of poultry waste as an example. China Popul. Resour. Environ. 26 (10), 44–52. doi:10.3969/j.issn.1002-2104.2016.10.006
Yan, B., Zhang, Q., and Liu, T. (2020). Can mobile phone promote the adoption of IPM technology by farmers? J. Agrotechnical Econ. 5, 45–59. doi:10.13246/j.cnki.jae.20190807.001
Yang, C., Tian, Y., and Xu, X. (2021). The path of price and tax mechanism to achieve carbon peak and carbon neutrality. Price Theory & Pract. 1, 20–26+65. doi:10.19851/j.cnki.cn11-1010/f.2021.01.22
Yang, K., Fahad, S., and He, H. (2022). Assessing the cooking oil fume exposure impacts on Chinese women health: An influential mechanism analysis. Environ. Sci. Pollut. Res. 29, 53860–53872. doi:10.1007/s11356-022-19368-8
Yu, B., Zhao, G., An, R., Chen, J., Tan, J., and Li, X. (2021). Research on China's CO2 emission pathway under carbon neutral target. J. Beijing Inst. Technol. Soc. Sci. Ed. 23 (2), 17–24. doi:10.15918/j.jbitss1009-3370.2021.7380
Yu, F. (2018). An analysis of the reasons, core and countermeasures of agricultural green development in the new era. Chin. Rural. Econ. 5, 19–34. Available at: https://kns.cnki.net/kcms/detail/detail.aspx?FileName=ZNJJ201805002&DbName=CJFQ2018.
Zhang, G., Du, P., and Chen, M. (2021). Digital empowerment and enterprise technology innovation—empirical research from Chinese manufacturing enterprises. Mod. Econ. Sci., 1–18. doi:10.1016/j.jclepro.2022.134261
Zhang, J., and Wang, H. (2020). Environmental regulation, agricultural technology innovation and agricultural carbon emission. J. Hubei Univ. (Philosophy Soc. Sci. 47 (4), 147–156. doi:10.13793/j.cnki.42-1020/c.2020.04.018
Zhang, J., and Zhang, X. (2020). The impact of internet use on the decision-making of farmland transfer and its mechanism: Evidence from the CFPS data. Chin. Rural. Econ. 3, 57–77. Available at: https://kns.cnki.net/kcms/detail/detail.aspx?FileName=ZNJJ202003004&DbName=CJFQ2020.
Zhang, Y., Tian, Y., Wang, Y., Wang, R., and Peng, Y. (2019). Rural human capital, agricultural technology progress and agricultural carbon emissions. Sci. Technol. Manag. Res. 39 (14), 266–274. doi:10.3969/j.issn.1000-7695.2019.14.035
Zhao, Q. (2021). Influence of technology promotion on pesticide reduction behavior of vegetable growers and the effect of pesticide reduction. Northwest A&F Univ. J. doi:10.27409/d.cnki.gxbnu.2021.000040
Keywords: digital technology use, farmers’ low-carbon production behavior, carbon emission peak, carbon neutrality, risk cognition, mediating effect
Citation: Huang X, Yang F and Fahad S (2022) The impact of digital technology use on farmers’ low-carbon production behavior under the background of carbon emission peak and carbon neutrality goals. Front. Environ. Sci. 10:1002181. doi: 10.3389/fenvs.2022.1002181
Received: 24 July 2022; Accepted: 27 September 2022;
Published: 12 October 2022.
Edited by:
Cem Işık, Anadolu University, TurkeyReviewed by:
Umer Shahzad, Anhui University of Finance and Economics, ChinaSufyan Ullah Khan, University of Stavanger, Norway
Syed Hussain Gillani, National Textile University, Pakistan
Copyright © 2022 Huang, Yang and Fahad. This is an open-access article distributed under the terms of the Creative Commons Attribution License (CC BY). The use, distribution or reproduction in other forums is permitted, provided the original author(s) and the copyright owner(s) are credited and that the original publication in this journal is cited, in accordance with accepted academic practice. No use, distribution or reproduction is permitted which does not comply with these terms.
*Correspondence: Shah Fahad, c2hhaC5mYWhhZEBtYWlsLnhqdHUuZWR1LmNu