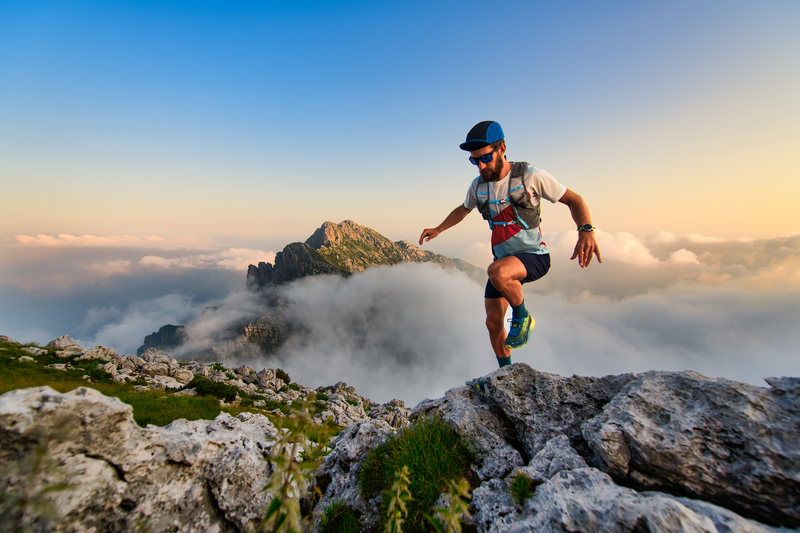
94% of researchers rate our articles as excellent or good
Learn more about the work of our research integrity team to safeguard the quality of each article we publish.
Find out more
COMMUNITY CASE STUDY article
Front. Environ. Sci. , 03 February 2022
Sec. Environmental Economics and Management
Volume 9 - 2021 | https://doi.org/10.3389/fenvs.2021.826838
The concept of agricultural and environmental sustainability refers to minimizing the degradation of natural resources while increasing crop productions; assessment of inflow and outflow energy resources is helpful in highlighting the resilience of the system and maintaining its productivity. In this regard, the current study evaluated the amount of energy input–output of cotton productions and their environmental interventions. Data are randomly collected from 400 cotton farmers through face-to-face interview. Results suggested that the major energy is consumed by three culprits, i.e., chemical fertilizer, diesel fuel, and irrigation water (11,532.60, 11,121.54, and 4,531.97 MJ ha−1, respectively). Total greenhouse gas (GHG) emission is 1,106.12 kg CO2eq ha−1 with the main share coming from diesel fuel, machinery, and irrigation water. Stimulating data of energies, e.g., energy use efficiency (1.53), specific energy (7.69 MJ kg−1), energy productivity (0.13 kg MJ−1), and net energy gained (16,409.77 MJ ha−1). Further analysis using data envelopment analysis (DEA) showed that low technical efficiency, i.e., 69.02%, is the most probable cause of poor energy use efficiency. The impermanent trend in growth of energy efficiency has been witnessed with plausible potential of energy savings from 4,048.012 to 16,194.77 MJ ha−1 and a reduction of 148.96–595.96 kg CO2eq ha−1 in GHG emission. Cobb–Douglas production function is further applied to discover the associations of energy input to output, which inferred that chemical fertilizer, diesel fuel, machinery, and biocides have significant effect on cotton yield. The marginal physical productivity (MPP) values obliged that the additional use in energy (1 MJ) from fuel (diesel), biocides, and machinery can enhance cotton yield at the rate of 0.35, 1.52, and 0.45 kg ha−1, respectively. Energy saving best links with energy sharing data, i.e., 55.66% (direct), 44.34% (indirect), 21.05% (renewable), and 78.95% (nonrenewable), further unveiled the high usage of nonrenewable energy resources (fossil fuels) that ultimately contributes to high emissions of GHGs. We hope that these findings could help in the management of energy budget that we believe will reduce the high emissions of GHGs.
The Pakistan agricultural food basket is dominated by production of grain and cash crops such as wheat, rice, sugarcane, and cotton that is currently being deteriorated by the traditional farming approaches (Rehman et al., 2016; Elahi et al., 2019b; Elahi et al., 2019c). However, due to the high export value of cotton in the global market, its production stands with significant contribution to the national economy that accounts for 6.9% of the agricultural added value and about 1.4% of the gross domestic product (GDP) (Hayat et al., 2020). Pakistan is the fifth largest cotton-producing country of the world (Nadeem et al., 2014), bookkeeping for 9.80% of worldwide cotton productions (Zulfiqar et al., 2021). Amid the same period Pakistan’s yarn and garment exports revenue accounted for around 26% and 14% of the global market (Ullah et al., 2020), respectively. Cotton share was 46% of exports revenue of the country's total exports, employing 35% of industrial labor force at the national level (Rehman et al., 2019a; Rehman et al., 2019b).
The quantity and quality of cotton farming and its industrial by-products have a significant contribution towards national economic growth (Zulfiqar et al., 2021). Despite extensive efforts and other motivating factors, the cotton yield in Pakistan remained lower in comparison to other cotton-producing regions (Rehman et al., 2016). The efforts to enhance cotton production leads to the excessive application of resources, i.e., irrigation water, chemical fertilizer, and pesticide that are ultimately deteriorating the environment, public health, and financial return (Elahi et al., 2020). Severe runoff of agricultural inputs result in an unsustainable agriculture production path (Imran et al., 2019). As a result, researchers and policy makers are pushing and focusing on advance energy-efficient agricultural resource utilization, which would ultimately lead to environmental, social, and financial sustainability (Alluvione et al., 2011; Elahi et al., 2019a; Tayyab et al., 2020; Shah et al., 2021). The accelerating process of agricultural modernization increased the quantity of fossil fuel consumption; therefore, optimization is a way to reduce fossil energy and greenhouse gas (GHG) emissions (Rokicki et al., 2021; Zhao et al., 2021). The increased consumption of energy not only intensifies the environmental pollution but also brings serious threat to human life (Gu et al., 2019; Gu et al., 2020a; Gu et al., 2020b; Niu et al., 2020; Mir et al., 2021; Sepehri et al., 2021). Therefore, it is an important task to improve energy efficiency of production systems to strengthen the comprehensive management of ecological environment (Steinbuks and Hertel, 2014; Peng et al., 2019b; Zhao et al., 2020). Energy efficiency is considered to be an important factor for the agricultural sector that comprehensively analyzes the energy-saving potential and simulates the energy input–output profile of production systems to improve the regional environmental efficiency (Abbas et al., 2018; Sheng et al., 2019; Tu et al., 2019; Zhong et al., 2020). Determination of energy efficiency includes the evaluation and comparison of the geographical and temporal efficiency of farming systems that can help to compare and improve energy managements (Pellegrini and Fernández, 2018; Zhong et al., 2021). There are two kinds of energies involved in agriculture production systems, i.e., direct and indirect. The term “direct energy” refers to the energy associated with inputs and resources directly used at a farm to carry out different activities, whereas “indirect energy” involves the energy associated with the resources used during manufacturing, packing, and delivering the inputs (such as fertilizer, chemicals, and machinery) at the farm gate (Walters et al., 2016). Direct and indirect energies provide a system boundary of the whole production cycle to quantify environmental impacts, i.e., life cycle assessment, aligned with International Standards Organization (ISO) standards of environmental management (Finkbeiner, 2014b; Finkbeiner, 2014a). The literature on energy use is well documented; numerous studies have been conducted in different regions across the world over different agricultural crops, for instance, energy efficiency assessment of rice (Muazu et al., 2015; Soni and Soe, 2016), wheat (Imran and Ozcatalbas, 2021), corn (Banaeian and Zangeneh, 2011), sugar beet (Kazemi et al., 2015), soybean (Mousavi-Avval et al., 2011c), canola (Mousavi-Avval et al., 2011b), faba bean (Kazemi et al., 2015), alfalfa (Asgharipour et al., 2016), peach and cherry (Aydın and Aktürk, 2018), apple (Çelen et al., 2017), and vegetables (Heidari and Omid, 2011). However, there are few studies on energy input–output of cotton production; Kazemi et al. (2018) analyzed the energy use efficiency of cotton cultivations in two climatic regions of Iran (Darab and Gorgan). The cotton cultivation in Darab requires 36,189.03 MJ ha−1 energy to produce 34,090.07 MJ ha−1, and Gorgan consumes 31,860.6 MJ ha−1 energy to produce 35,237.82 MJ ha−1. Gorgan consumes less energy and produces more; thus, with average energy use efficiency of 1.106, Gorgan is found to be a more efficient and profitable region in cotton cultivations than Darab. Gokdogan et al. (2016) considered the energy balance of cotton cultivations in Turkey. The production of 4,750 kg of cotton from 1 ha requires 29,138.11 MJ energy (75.5% from fuel and fertilizer). Bonou-zin et al. (2019) studied energy flow and environmental emissions from cotton cultivations at conventional and organic farms. Organic farmers consumed less energy than conventional farmers; similarly, the quantity of GHG emission produced by organic farmers is relatively lower than the conventional farmers, but organic farmers are still environmentally inefficient compared to conventional farmers. Pishgar-Komleh et al. (2012) explored the stakeholders of energy consumption in cotton productions. The major share comes from indirect energy resources (60%) and non-renewable energy resources (71%). The heavy usage of fossil fuels is not only deteriorating the environment and depleting natural resources but also negatively impacting on climate change (Peng et al., 2019a; Shen et al., 2019; Peng et al., 2021). Consequently, climate change has adverse impacts on crop productivity (Elahi et al., 2021a). Pellegrini and Fernández (2018) studied the energy use efficiency and suggested possible remedies for preserving natural resources, so that the intensive demand and excessive utilization can be revived with suitable production structure, proper farm management, and adoption of new strategies and technologies. However, a comprehensive assessment of the farm production process is to do what is required to enhance energy use efficiency of crop inputs and develop innovative techniques for sustainable development (Rezvani Moghaddam et al., 2011; Asgharipour et al., 2012; Mondani et al., 2017). Efficient use of energy resources will decrease environmental pollution to save natural resources (Owusu and Asumadu-Sarkodie, 2016; Odhiambo et al., 2020; Nassani et al., 2021; Shittu et al., 2021). Increasing the usage of renewable energy resources (Sarkar and Seo, 2021) and monitoring energy efficiency in supply allocation of agricultural production system could make a valuable contribution towards sustainable energy development targets (Gielen et al., 2019; Kumar and Majid, 2020). Therefore, the purpose of this study is to assess energy efficiency at operational level (farm level) of agronomic inputs, GHG emissions, and utilization of renewable energy and non-renewable energy resources at cotton farms of Pakistan. Furthermore, regression and sensitivity analysis are performed to see the relationship between energy inputs and output. The findings of this study can be helpful to achieve the fundamental principles of sustainability, i.e., dynamic balance and integrated management.
This study is performed in Punjab, Pakistan (a province that is considered a necessary backbone of the country economy), producing 80% of the total cotton production of the country (Wei et al., 2020). From Punjab province, five cotton-producing districts named Vehari, Dera Gazi Khan, Lodhran, Rahim Yar khan, and Multan are successively selected to collect, analyze, and alleviate the data regarding agricultural practices (Figure 1).
FIGURE 1. (A) The selected area for good execution of this study which clearly indicates the high density of cotton growers, strongly concentrated in specific regions only (districts). (B) The geographical information and percentage of cotton production in selected districts.
Multistage sampling technique was used to collect data from farmers (Elahi et al., 2021b; Elahi et al., 2018a). Eighty farmers from each district are randomly selected; thus, a total of 400 cotton farmers are interviewed from five selected districts. A well-structured questionnaire containing the information regarding use of seeds, chemical fertilizers, pesticides, FYM (farm yard manure), irrigation water, labor, diesel fuel, and machinery is used in the survey. The quantities of inputs used are calculated per acre (laterally converted to hectare) then multiplied with the coefficient of energy equivalent. The energy equivalent coefficients are determined from literature and given in Table 1; a detailed description about the calculation of energy equivalents is provided in the reference by Kitani (1999), and the same protocol has been followed in this study. Similarly, the conversion coefficients for GHG emission are also derived from previous studies, and the equivalent amount of CO2 is determined by multiplying the amount of input with equivalent factor; equivalent coefficients are provided in Table 1.
The energy associated with all cotton inputs except machinery are calculated directly from energy equivalent Table 1, and the energy associated with agricultural machinery is calculated by using Eq. 1.
where ME is the machine energy (MJ ha−1), Wm is the weight of machine (kg), eq is the energy equivalent (MJ unit−1), tm is the machine working time in the field (h ha−1), and Tm is the economic/useful life of machine (h). The calculated values are presented in Table 1.
The individual energy against every input is calculated by multiplying their corresponding energy equivalents; the total quantity of energy input is then determined by adding all the energy equivalences. For energy output, the quantity of cotton yield is multiplied with the relevant energy equivalent. Based on the energy input–output profile, the energetic variables like energy use efficiency, specific energy, energy productivity, and net energy gain are calculated. Energy use efficiency is the ratio of energy output to energy input and the average energy consumed (input) and produced (output) during the year 2018–2019. Specific energy is the amount of energy consumed to produce a unit mass of cotton yield, while energy productivity exhibits the quantity of cotton yield for each MJ of energy. The net energy gain then summarizes the energy flow of the production system; higher net indicates more energy gain (more profitable), and vice versa. The following Eqs 2–5 are used (Walters et al., 2016):
where Eue is the energy use efficiency, Eo is the energy output (MJ ha−1), Ei is the energy input (MJ ha−1), Esp is the specific energy (MJ kg−1), Yc is the cotton yield (kg ha−1), Epr is the energy productivity (kg MJ−1), and Neg is the net energy gain (MJ ha−1).
Data envelopment analysis (DEA) is further used to estimate the technical efficiency of contending farmers. DEA is an extensively used non-parametric approach for estimation of productive efficiency. Based on linear programing, DEA measures the relative efficiency of different entities known as decision-making units (DMUs). DEA is explained by numerous authors (Elhami et al., 2016; Nabavi-Pelesaraei et al., 2016; Singh et al., 2019; Wei et al., 2020), so the details are not provided here. DEA was first introduced by Charnes, Cooper, and Rhodes (CCR) and characterized as constant return to scale and variable return to scale. DEA is further categorized as input and output oriented. In input-oriented analysis, a unit is made efficient by reducing the level of inputs while maintaining the level of output, and the output-oriented deals to gain the increased level of output with the same level of inputs. Input-oriented analysis seems more suitable for agriculture systems, as a farmer has more grip on inputs as compared to output (Walters et al., 2016). So we considered input-oriented DEA expressed in Eqs 6 (the standard form) 7 (the explanatory form) (Mohammadi et al., 2014). As DEA measures the ratio between weighted output to weighted input, DEA efficiency score usually ranges from 0 to 1. The DEA solver professional release 4.1 is used to evaluate the efficiency score of a particular DMU in Pakistan.
where η is efficiency; Wso, weighted sum of outputs; and Wsi, weighted some of inputs. Consider S1, S2 … SN are weights given to output, O1J*, O2J*… ONJ* are the amount of outputs of DMU J*, and r1, r2 … rM are weights given to input, and I1J*, I2J*… IMJ* are the number of inputs of DMU J*.
Cobb–Douglas is used to study the input–output relationship of energy used and gained during cotton production; the production function of Cobb–Douglas is expressed as follows (Singh et al., 2004), Eq. 8.
where, Yi is cotton yield of ith farmer, a and aj are the values of function (a is constant, while aj is derived through regression model) (coefficient of jth input), Xij is jth input of ith farmer, and the error term of ith farmer is ui, which is normally distributed with zero mean value and constant variance (Elahi et al., 2017). We assume that if X is 0, then Y is also 0 (Hatirli et al., 2005; Rafiee et al., 2010). Similarly, the Cobb–Douglas production function for direct and indirect energy is described in Eqs 9 and 10 and is used for renewable and non-renewable energy (Mousavi-Avval et al., 2011a; Pishgar Komleh et al., 2011). Yield is considered as the dependent variable in both cases.
where Yi and ui are cotton yield and error terms of ith farmer, ß1, ß2, γ1, and γ2 are coefficients of regression model, DE stands for direct energy, IDE indirect energy, RE renewable energy, and NRE for non-renewable energy.
The sensitivity of energy inputs to output is determined through marginal physical productivity (MPP). The MPP value indicates the variations in cotton yield with a unit increase/decrease in inputs; using the response coefficients of the inputs, MPP calculates the output change with a unit change in the input when all other inputs remain constant at their geometric mean levels. The following equation is used to calculate the MPP value of jth energy inputs. MPP is based on the response coefficients of the inputs, the MPP value of any input indicates the output change with a unit change in the input when all other inputs are constant at their geometric mean level. The following Eq. 11 is used to calculate the MPP values (Singh et al., 2004).
where MPPxj is the marginal physical productivity of jth energy input, GM(Y) is geometric mean of cotton yield, GM(Xj) is jth input geometric mean, and aj is the regression coefficient. As MPP is the ratio of geometric means of yield and inputs, positive MPP indicates increase in yield with an increase in specific input; negative MPP exhibits negative contribution to yield, i.e., decrease in yield with further increase in that specific input. The MPP values provide a threshold for individual inputs in which further increment is not only harming the yield but also deteriorating the resources (Singh et al., 2004; Rafiee et al., 2010; Pishgar Komleh et al., 2011).
The average of energy input–output in cotton production during 2018–2019 and their energy equivalents are presented in Table 2. In order to gain more realistic results, the average values of 400 farmers are considered. The results revealed that total energy input and output are 30,740.99 and 47,150.76 MJ ha−1, respectively. It can be seen from Table 2 that machinery used in cotton production is just for 52.65 h ha−1, which indicates a lower level of mechanization. Cotton picking is the most labor-intensive operation in cotton cultivations; not a single farmer (out of the selected 400 farmers) is using cotton pickers (cotton picking machine), which made cotton the highest labor-consuming crop. The average labor consumption is determined as 744.05 h ha−1. The quantities of other inputs like irrigation water, chemical fertilizer, machinery, seed, and biocide are 4,443.10 m3 ha−1, 195.71 kg ha−1, 52.65 h ha−1, 16.95 kg ha−1, and 3.74 L ha−1.
Moreover, percentages of individual inputs to the total energy input (%) are also presented in Table 2; chemical fertilizers (mainly nitrogen) consume 37% of total energy input followed by diesel fuel that utilized 36%. Chemical fertilizer is used to enhance the soil and plant productivity, while fuel energy is consumed during cultural operations in the field, land and seedbed preparations, goods transportation, and pumping of irrigation water. Moreover, seed, manure, and biocides are the least demanding energy input for cotton production (only 1% of the total sequestered energy), while human labor and machinery contributed about 5% and 4%, respectively. Cotton is an important cash crop that gained more value in recent years; consequently, cultivation area and number of farmers have been increased. Thus, excessive utilization of inputs has been observed. Additionally, there is a common belief among Pakistani farmers that excessive application of chemical fertilizers leads to increased crop yield (Elahi et al., 2018b). Moreover, less involvement of technological innovations in agricultural productions also raises inefficiency, and thus subsidies and passive provision of commercial credit to technological innovation will help farmers in precision agriculture (Liu, 2021). The application of farmyard manure and cultivation of legume crops is an excellent alternative solution to control the higher usage of chemical fertilizers.
Furthermore, the energy use efficiency, energy productivity, specific energy, and net energy gain of cotton is calculated using Eqs 2–5 and presented in Table 2. The energy use efficiency and specific energy is determined to be 1.53 and 7.69 MJ kg−1, respectively. The value of energy use efficiency indicated that the energy output of cotton production is 1.53 times higher than that of total energy input, which implies that cotton production is an energy-efficient crop in the studied region. Energy use efficiency and specific energy are integrative indices that are representative of the potential environmental impacts associated with the production of crops. Gokdogan et al. (2016) and Tsatsarelis (1991) reported the energy use efficiency as 1.92 and 0.66 in Turkey and Greece, respectively; the difference refers to higher level of mechanization and production in Turkey, while farmers in Greece use more inputs and gain less yield. Energy productivity and net energy gain are calculated as 0.13 kg MJ−1 and 16409.77 MJ ha−1. Energy productivity means that 0.13 kg of cotton is obtained per unit of energy (MJ). Energy productivity of Pakistani cotton farming systems is found lower than Turkish which is 0.16 kg MJ−1, which indicates a lower productive level of inputs or less soil productivity that resulted in less yield and ultimately lower net energy gain values (i.e., 1.64 times less than Turkey). Results revealed that 93% share of total energy consumed in cotton production comes from chemical fertilizer, diesel fuel, irrigation water, and human labor. A wide range of these resources indicated an inefficient resource utilization. This implies that the right amount of chemical fertilizer together with improved level of mechanization and involvement of precision agriculture could significantly enhance energy use efficiency in cotton production.
The collective share of different inputs, the equivalent amount of GHG emission from individual inputs, technical efficiency, and plausible potential of resource savings is shown in Figure 2. The results of Figure 2A revealed that the average energy values for direct and indirect energy are 17,111.86 and 13,629.14 MJ ha−1, while renewable and non-renewable energy are 6,471.56 and 24,269.44 MJ ha−1, respectively. The shares of direct energy and indirect energy to total energy are 55.66% and 44.34%, and the renewable and nonrenewable shares are 21.05% and 78.95%, respectively. Our results are consistent with Gokdogan et al. (2016); they reported 50.15%, 49.85%, 12.72%, and 87.28%, respectively. It can be seen that the non-renewable energy resources (fossil fuels) are the major source of energy consumption and GHG emissions in cotton productions.
FIGURE 2. (A) Share of direct, indirect, renewable, and nonrenewable energies. (B) The equivalent amount of GHG emissions. (C) Efficiency and inefficiency score for each district at DMU1, Vehari; DMU2, Dera Ghazi Khan; DMU3, Lodhran; DMU4, Rahim Yar khan; and DMU5, Multan. (D) Percentage of plausible potential of resource savings in each district.
Figure 2B depicts the determined quantity of GHG emissions. The total quantity of GHG emission is 1,106.12 kg CO2eq ha−1 from cotton productions, with leading share of diesel fuel accounting for 58% followed by irrigation water for 23%. Chemical fertilizer and agriculture machinery contribute 9% and 8%, respectively, while biocides are the least deteriorating input to the environment. The results of this study showed that diesel fuel and irrigation water with the contribution of 642.16 and 253.26 kg CO2eq ha−1 are the most important inputs in GHG emission for cotton production, as cotton is a water-intensive crop that ultimately leads to higher GHG emission. While diesel fuel is mainly consumed in field operations, and tractor-mounted implements are used for field operations, the improper matching of equipment's and worn-out tractors are the reasons of high fuel energy and GHG emission. The quantity of GHG produced from chemical fertilizer is 105.01 kg CO2eq ha−1. The GHG from chemical fertilizer is not only causing air pollution but also harmful to soil and water. Organic farming, crop rotation, cultivation of legume and alfa crops, and green manuring are suitable and potential alternatives to increase soil fertility and organic matter and to reduce consumption of chemical fertilizer and GHG emissions. The GHG emission from agricultural machinery usage and biocide is calculated to be 86.59 253.26 and 19.10 kg CO2eq ha−1, respectively. Koga et al. (2003) reported that 15–29% GHG emission could be reduced with alternative tillage systems. So better management techniques are viable for energy security and environment friendly agriculture production in Pakistan.
Input-oriented CCR model is used to assess the technical efficiency of cotton farmers. As discussed earlier, chemical fertilizer, diesel fuel, and irrigation water are the three major contributing stakeholders of energy consumption; thus, we use these three stakes as input variables and cotton yield as output to DEA input datasheet, and selected districts of Punjab province are considered as DMUs. Malana and Malano (2006) used a similar pattern in their study to estimate the productive efficiency of wheat farmers in India and Pakistan; chemical fertilizer, seed, and irrigation water are used as the inputs and wheat yield as the output variable to DEA input dataset. The selected districts, Vehari, Dera Gazi Khan, Lodhran, Rahim Yar khan, and Multan are followed by DMUs (DMU1–DMU5). Furthermore, the CCR-based technical efficiency score of contending DMUs is calculated and presented in Figure 2C. Based on DEA results, the average technical efficiency score is 69.02% of cotton cultivation, with DMU5 (Multan) the most efficient district in cotton production. That indicates DMU5 performed at the frontier than other DMUs and exhibits a higher level of technical efficiency.
In other words, DMU5 had less use of energy or had excess in the yield. So improvement can be made in technically inefficient DMUs by raising their performance to DMU5. For instance, DMU1, Vehari district, exhibits technical efficiency of 85.11% indicating that DMU1 is 14.89% inefficient relative to DMU5. In another way the same level of output can be received from DMU1 if it operates at frontiers. Similarly, for DMU2, Dera Gazi Khan possessed the technical efficiency of 40.43% demonstrating almost 60% inefficiency compares to DMU5. The dual interpretation of technical efficiencies of DMUs shows the level of inefficiency and potential of resource savings by raising the performance of less efficient DMUs to DMU5 (operating at frontier). The potential of resource savings in different DMUs has been illustrated in Figure 2D.
The association between energy inputs and cotton yield is further explored through regression analysis and presented in Table 3. The positive value of regression coefficient indicates an increasing impact, and negative values demonstrate decreasing impact on cotton yield. It can be seen from Table 3 that all the independent variables except chemical fertilizer possessed positive coefficient and reveal a significant impact on cotton yield. The rate of positive and negative impacts of energy inputs on cotton yield show that about 10% increase in biocides energy can cause 4.0% increase in cotton yield. Similarly, the cotton yield can be increased by 2.5% and 2.4% with 10% increase in machinery and diesel fuel energy input, respectively, while the cotton yield will decrease by 4.4% with 10% increase in the applications of chemical fertilizers. Results show that biocides possessed the highest positive impact; agricultural machinery and diesel fuel exhibited the second and third most impactful inputs to cotton yield, while irrigation water, labor, and cotton seeds are the least impacting inputs. Hatirli et al. (2006) studied the sensitive inputs to tomato cultivations in Antalya, Turkey. They found that the farm labor and irrigation water have significant impact on tomato yield. In another study, Mohammadi et al. (2010) investigated the energy use efficiency and associated inputs to kiwi fruit; inputs like irrigation water, machinery, and chemical fertilizer have significant impact on kiwi yield. The MPP values further described that with 1 MJ increase in each input of biocide, machinery, and diesel fuel energy, an additional increase of 1.52, 0.45, and 0.35 kg ha−1 would happen in cotton yield, while cotton yield will decrease at the rate of 1.78 kg ha−1 for every additional increase in fertilizer energy. Additionally, regression coefficients for direct energy, indirect energy, renewable energy, and non-renewable energy are 0.14, 0.68, 0.53, and 0.39, respectively. Furthermore, all the regression coefficients for collective energy inputs (direct, indirect, renewable, non-renewable) are positive (Table 3), indicating an increase in input energies can increase output; MPP values further exhibit that increasing 1 MJ of direct energy, indirect energy, renewable energy, and non-renewable energy can increase cotton yield by 0.51, 1.02, 2.78, and 0.54 kg ha−1, respectively. Moreover, the highest increment in cotton yield with an additional 1 MJ of energy is observed in renewable energy resources.
The current assessment of energy use and GHG emissions in cotton production resulted in the following conclusions.
• The total energy input–output for cotton production is 30,740.99 and 47,150.76 MJ ha−1, respectively.
• The total GHG emission is calculated as 1,106.12 kg CO2eq ha−1, with leading share of diesel fuel (58%) followed by irrigation water (23%) and chemical fertilizer (9%).
• The DEA results further revealed an average of 69.02% technical efficiency with plausible potential of energy savings from 4,048.012 to 16,194.77 MJ ha−1 and reduction in GHG emission from 148.96 to 595.96 kg CO2eq ha−1.
• A key message emerging from this study is that there is considerable scope for increasing the efficiency of cotton production of individual farms in Pakistan.
• Farm size, financial resources, type of farmer enterprise, experience, education, and other socioeconomic factors of farmers can be incorporated for future studies.
The original contributions presented in the study are included in the article/supplementary material, further inquiries can be directed to the corresponding author.
AA: overall conceptualization, methodology, investigation, analysis, and writing. CZ: supervision, data curation, and conceptualization. MW and KA: data curation and draft editing. RA: review and editing and formal analysis.
The authors of this study would like to express their appreciation to the key project of the National Natural Science Foundation (42130405), the Innovative and Entrepreneurial Talent Program of Jiangsu Province (R2020SC04), and the Strategic Priority Research Program of the Chinese Academy of Sciences (XDA2006030201) for their sponsorship.
The authors declare that the research was conducted in the absence of any commercial or financial relationships that could be construed as a potential conflict of interest.
All claims expressed in this article are solely those of the authors and do not necessarily represent those of their affiliated organizations or those of the publisher, the editors, and the reviewers. Any product that may be evaluated in this article, or claim that may be made by its manufacturer, is not guaranteed or endorsed by the publisher.
We would like to thank our survey team members for conducting the field survey.
Abbas, A., Yang, M., Yousaf, K., Ahmad, M., Elahi, E., and Iqbal, T. (2018). Improving Energy Use Efficiency of Corn Production by Using Data Envelopment Analysis (A Non-parametric Approach). Fresenius Environ. Bull. 27, 4725–4733.
Alluvione, F., Moretti, B., Sacco, D., and Grignani, C. (2011). EUE (Energy Use Efficiency) of Cropping Systems for a Sustainable Agriculture. Energy 36, 4468–4481. doi:10.1016/j.energy.2011.03.075
Asgharipour, M. R., Mondani, F., and Riahinia, S. (2012). Energy Use Efficiency and Economic Analysis of Sugar Beet Production System in Iran: A Case Study in Khorasan Razavi Province. Energy 44, 1078–1084. doi:10.1016/j.energy.2012.04.023
Asgharipour, M. R., Mousavinik, S. M., and Enayat, F. F. (2016). Evaluation of Energy Input and Greenhouse Gases Emissions from Alfalfa Production in the Sistan Region, Iran. Energ. Rep. 2, 135–140. doi:10.1016/j.egyr.2016.05.007
Aydın, B., and Aktürk, D. (2018). Energy Use Efficiency and Economic Analysis of Peach and Cherry Production Regarding Good Agricultural Practices in Turkey: A Case Study in Çanakkale Province. Energy 158, 967–974. doi:10.1016/J.ENERGY.2018.06.087
Banaeian, N., and Zangeneh, M. (2011). Study on Energy Efficiency in Corn Production of Iran. Energy 36, 5394–5402. doi:10.1016/j.energy.2011.06.052
Bonou-zin, R. D. C., Allali, K., and Fadlaoui, A. (2019). Environmental Efficiency of Organic and Conventional Cotton in Benin. Sustainability 11, 3044. doi:10.3390/SU11113044
Çelen, İ. H., Baran, M. F., Önler, E., and Bayhan, Y. (2017). Determination of Energy Balance of Apple (Malus Domestica) Production in Turkey: A Case Study for Tekirdag Province. Anadolu J. Agric. Sci. 32, 40. doi:10.7161/Omuanajas.289604
Dyer, J. A., and Desjardins, R. L. (2006). Carbon Dioxide Emissions Associated with the Manufacturing of Tractors and Farm Machinery in Canada. Biosyst. Eng. 93, 107–118. doi:10.1016/j.biosystemseng.2005.09.011
Dyer, J. A., and Desjardins, R. L. (2003). Simulated Farm Fieldwork, Energy Consumption and Related Greenhouse Gas Emissions in Canada. Biosyst. Eng. 85, 503–513. doi:10.1016/S1537-5110(03)00072-2
Elahi, E., Zhang, L., Abid, M., Javed, M. T., and Xinru, H. (2017). Direct and Indirect Effects of Wastewater Use and Herd Environment on the Occurrence of Animal Diseases and Animal Health in Pakistan. Environ. Sci. Pollut. Res. Int. 24, 6819–6832. doi:10.1007/s11356-017-8423-9
Elahi, E., Abid, M., Zhang, H., Cui, W., and Ul Hasson, S. (2018a). Domestic Water Buffaloes: Access to Surface Water, Disease Prevalence and Associated Economic Losses. Prev. Vet. Med. 154, 102–112. doi:10.1016/j.prevetmed.2018.03.021
Elahi, E., Abid, M., Zhang, L., ul Haq, S., and Sahito, J. G. M. (2018b). Agricultural Advisory and Financial Services; Farm Level Access, Outreach and Impact in a Mixed Cropping District of Punjab, Pakistan. Land use policy 71, 249–260. doi:10.1016/j.landusepol.2017.12.006
Elahi, E., Khalid, Z., Tauni, M. Z., Zhang, H., and Lirong, X. (2021a). Extreme Weather Events Risk to Crop-Production and the Adaptation of Innovative Management Strategies to Mitigate the Risk: A Retrospective Survey of Rural Punjab, Pakistan. Technovation, 102255. doi:10.1016/j.technovation.2021.102255
Elahi, E., Khalid, Z., Weijun, C., and Zhang, H. (2020). The Public Policy of Agricultural Land Allotment to Agrarians and its Impact on Crop Productivity in Punjab Province of Pakistan. Land Use Policy 90, 104324. doi:10.1016/j.landusepol.2019.104324
Elahi, E., Weijun, C., Jha, S. K., and Zhang, H. (2019a). Estimation of Realistic Renewable and Non-renewable Energy Use Targets for Livestock Production Systems Utilising an Artificial Neural Network Method: A Step towards Livestock Sustainability. Energy 183, 191–204. doi:10.1016/j.energy.2019.06.084
Elahi, E., Weijun, C., Zhang, H., and Abid, M. (2019b). Use of Artificial Neural Networks to rescue Agrochemical-Based Health Hazards: a Resource Optimisation Method for Cleaner Crop Production. J. Clean. Prod. 238, 117900. doi:10.1016/j.jclepro.2019.117900
Elahi, E., Weijun, C., Zhang, H., and Nazeer, M. (2019c). Agricultural Intensification and Damages to Human Health in Relation to Agrochemicals: Application of Artificial Intelligence. Land Use Policy 83, 461–474. doi:10.1016/j.landusepol.2019.02.023
Elahi, E., Zhang, H., Lirong, X., Khalid, Z., and Xu, H. (2021b). Understanding Cognitive and Socio-Psychological Factors Determining Farmers' Intentions to Use Improved Grassland: Implications of Land Use Policy for Sustainable Pasture Production. Land Use Policy 102, 105250. doi:10.1016/j.landusepol.2020.105250
Elhami, B., Akram, A., and Khanali, M. (2016). Optimization of Energy Consumption and Environmental Impacts of Chickpea Production Using Data Envelopment Analysis (DEA) and Multi Objective Genetic Algorithm (MOGA) Approaches. Inf. Process. Agric. 3, 190–205. doi:10.1016/j.inpa.2016.07.002
Finkbeiner, M. (2014a). Product Environmental Footprint-Breakthrough or Breakdown for Policy Implementation of Life Cycle Assessment? Int. J. Life Cycle Assess. 19, 266–271. doi:10.1007/S11367-013-0678-X
Finkbeiner, M. (2014b). The International Standards as the Constitution of Life Cycle Assessment: The ISO 14040 Series and its Offspring. LCA Compendium–The Complete World of Life Cycle Assessment,Background and Future Prospects in Life Cycle Assess., 85, 106. doi:10.1007/978-94-017-8697-3_3
Gielen, D., Boshell, F., Saygin, D., Bazilian, M. D., Wagner, N., and Gorini, R. (2019). The Role of Renewable Energy in the Global Energy Transformation. Energ. Strategy Rev. 24, 38–50. doi:10.1016/J.ESR.2019.01.006
Gokdogan, O., Erdogan, O., Eralp, O., and Zeybek, A. (2016). Energy Efficiency Analysis of Cotton Production in turkey: a Case Study from Aydin Province. Fresenius Environ. Bull. 25, 4959–4964.
Gu, H., Bian, F., and Elahi, E. (2020a). Effect of Air Pollution on Female Labor Supply: An Empirical Analysis Based on Data of Labor Force Dynamic Survey of China. Soc. Work Public Health 35, 187–196. doi:10.1080/19371918.2020.1764433
Gu, H., Cao, Y., Elahi, E., and Jha, S. K. (2019). Human Health Damages Related to Air Pollution in China. Environ. Sci. Pollut. Res. Int. 26, 13115–13125. doi:10.1007/s11356-019-04708-y
Gu, H., Yan, W., Elahi, E., and Cao, Y. (2020b). Air Pollution Risks Human Mental Health: an Implication of Two-Stages Least Squares Estimation of Interaction Effects. Environ. Sci. Pollut. Res. 27, 2036–2043. doi:10.1007/s11356-019-06612-x
Hatirli, S. A., Ozkan, B., and Fert, C. (2005). An Econometric Analysis of Energy Input–Output in Turkish Agriculture. Renew. Sust. Energ. Rev. 9, 608–623. doi:10.1016/J.RSER.2004.07.001
Hatirli, S. A., Ozkan, B., and Fert, C. (2006). Energy Inputs and Crop Yield Relationship in Greenhouse Tomato Production. Renew. Energ. 31, 427–438. doi:10.1016/J.RENENE.2005.04.007
Hayat, N., Hussain, A., and Lohano, H. D. (2020). Eco-labeling and Sustainability: A Case of Textile Industry in Pakistan. J. Clean. Prod. 252, 119807. doi:10.1016/j.jclepro.2019.119807
Heidari, M. D., and Omid, M. (2011). Energy Use Patterns and Econometric Models of Major Greenhouse Vegetable Productions in Iran. Energy 36, 220–225. doi:10.1016/J.ENERGY.2010.10.048
Houshyar, E., Azadi, H., Almassi, M., Sheikh Davoodi, M. J., and Witlox, F. (2012). Sustainable and Efficient Energy Consumption of Corn Production in Southwest Iran: Combination of Multi-Fuzzy and DEA Modeling. Energy 44, 672–681. doi:10.1016/j.energy.2012.05.025
Imran, M. A., Ali, A., Ashfaq, M., Hassan, S., Culas, R., and Ma, C. (2019). Impact of Climate Smart Agriculture (CSA) through Sustainable Irrigation Management on Resource Use Efficiency: A Sustainable Production Alternative for Cotton. Land use policy 88, 104113. doi:10.1016/j.landusepol.2019.104113
Imran, M., and Ozcatalbas, O. (2021). Optimization of Energy Consumption and its Effect on the Energy Use Efficiency and Greenhouse Gas Emissions of Wheat Production in Turkey. Discov. Sustain. 2, 28. doi:10.1007/s43621-021-00035-w
Kazemi, H., Shahbyki, M., and Baghbani, S. (2015). Energy Analysis for Faba Bean Production: A Case Study in Golestan Province, Iran. Sustainable Prod. Consumption 3, 15–20. doi:10.1016/J.SPC.2015.07.004
Kazemi, H., Shokrgozar, M., Kamkar, B., and Soltani, A. (2018). Analysis of Cotton Production by Energy Indicators in Two Different Climatic Regions. J. Clean. Prod. 190, 729–736. doi:10.1016/J.JCLEPRO.2018.04.195
Kitani, O. (1999). CIGR Handbook of Agricultural Engineering: Energy and Biomass Engineering (Vol. VI). St. Joseph, MI: American Society of Agricultural Engineers, 330.
Koga, N., Tsuruta, H., Tsuji, H., and Nakano, H. (2003). Fuel Consumption-Derived CO2 Emissions under Conventional and Reduced Tillage Cropping Systems in Northern Japan. Agric. Ecosyst. Environ. 99, 213–219. doi:10.1016/S0167-8809(03)00132-4
Kumar, C. R., and Majid, M. A. (2020). Renewable Energy for Sustainable Development in India: Current Status, Future Prospects, Challenges, Employment, and Investment Opportunities. Energ Sustain. Soc. 10, 1–36. doi:10.1186/S13705-019-0232-1
Lal, R. (2004). Carbon Emission from Farm Operations. Environ. Int. 30, 981–990. doi:10.1016/j.envint.2004.03.005
Liu, T. (2021). Study on the Influence of Commercial Credit on Technological Innovation of Enterprises [M]. Beijing: China Social Sciences Press.
Malana, N. M., and Malano, H. M. (2006). Benchmarking Productive Efficiency of Selected Wheat Areas in Pakistan and India Using Data Envelopment Analysis. Irrig. Drain. 55, 383–394. doi:10.1002/ird.264
Mir, K. A., Park, C., Purohit, P., and Kim, S. (2021). Comparative Analysis of Greenhouse Gas Emission Inventory for Pakistan: Part II Agriculture, Forestry and Other Land Use and Waste. Adv. Clim. Change Res. 12, 132–144. doi:10.1016/J.ACCRE.2021.01.003
Mohammadi, A., Rafiee, S., Jafari, A., Keyhani, A., Mousavi-Avval, S. H., and Nonhebel, S. (2014). Energy Use Efficiency and Greenhouse Gas Emissions of Farming Systems in north Iran. Renew. Sust. Energ. Rev. 30, 724–733. doi:10.1016/j.rser.2013.11.012
Mohammadi, A., Rafiee, S., Mohtasebi, S. S., and Rafiee, H. (2010). Energy Inputs - Yield Relationship and Cost Analysis of Kiwifruit Production in Iran. Renew. Energ. 35, 1071–1075. doi:10.1016/j.renene.2009.09.004
Mondani, F., Aleagha, S., Khoramivafa, M., and Ghobadi, R. (2017). Evaluation of Greenhouse Gases Emission Based on Energy Consumption in Wheat Agroecosystems. Energ. Rep. 3, 37–45. doi:10.1016/J.EGYR.2017.01.002
Mousavi-Avval, S. H., Rafiee, S., Jafari, A., and Mohammadi, A. (2011a). Energy Flow Modeling and Sensitivity Analysis of Inputs for Canola Production in Iran. J. Clean. Prod. 19, 1464–1470. doi:10.1016/J.JCLEPRO.2011.04.013
Mousavi-Avval, S. H., Rafiee, S., Jafari, A., and Mohammadi, A. (2011b). Improving Energy Use Efficiency of Canola Production Using Data Envelopment Analysis (DEA) Approach. Energy 36, 2765–2772. doi:10.1016/j.energy.2011.02.016
Mousavi-Avval, S. H., Rafiee, S., Jafari, A., and Mohammadi, A. (2011c). Optimization of Energy Consumption for Soybean Production Using Data Envelopment Analysis (DEA) Approach. Appl. Energ. 88, 3765–3772. doi:10.1016/j.apenergy.2011.04.021
Muazu, A., Yahya, A., Ishak, W. I. W., and Khairunniza-Bejo, S. (2015). Energy Audit for Sustainable Wetland Paddy Cultivation in Malaysia. Energy 87, 182–191. doi:10.1016/j.energy.2015.04.066
Nabavi-Pelesaraei, A., Hosseinzadeh-Bandbafha, H., Qasemi-Kordkheili, P., Kouchaki-Penchah, H., and Riahi-Dorcheh, F. (2016). Applying Optimization Techniques to Improve of Energy Efficiency and GHG (Greenhouse Gas) Emissions of Wheat Production. Energy 103, 672–678. doi:10.1016/j.energy.2016.03.003
Nadeem, A. H., Nazim, M., Hashim, M., and Javed, M. K. (2014). Factors Which Affect the Sustainable Production of Cotton in Pakistan: A Detailed Case Study from Bahawalpur District. Lect. Notes Electr. Eng. 241, 745–753. doi:10.1007/978-3-642-40078-0_64
Nassani, A. A., Aldakhil, A. M., and Zaman, K. (2021). Ecological Footprints Jeopardy for mineral Resource Extraction: Efficient Use of Energy, Financial Development and Insurance Services to Conserve Natural Resources. Resour. Pol. 74, 102271. doi:10.1016/J.RESOURPOL.2021.102271
Niu, B., Peng, S., Li, C., Liang, Q., Li, X., and Wang, Z. (2020). Nexus of Embodied Land Use and Greenhouse Gas Emissions in Global Agricultural Trade: A Quasi-Input–Output Analysis. J. Clean. Prod. 267, 122067. doi:10.1016/J.JCLEPRO.2020.122067
Odhiambo, M. R. O., Abbas, A., Wang, X., and Elahi, E. (2020). Thermo-environmental Assessment of a Heated Venlo-type Greenhouse in the Yangtze River delta Region. Sustainability 12, 10412. doi:10.3390/su122410412
Owusu, P. A., and Asumadu-Sarkodie, S. (2016). A Review of Renewable Energy Sources, Sustainability Issues and Climate Change Mitigation. Cogent Eng. 3 (1), 1167990. doi:10.1080/23311916.2016.1167990
Pellegrini, P., and Fernández, R. J. (2018). Crop Intensification, Land Use, and On-Farm Energy-Use Efficiency during the Worldwide Spread of the green Revolution. Proc. Natl. Acad. Sci. USA 115, 2335–2340. doi:10.1073/pnas.1717072115
Peng, B., Chen, S., Elahi, E., and Wan, A. (2021). Can Corporate Environmental Responsibility Improve Environmental Performance? an Inter-temporal Analysis of Chinese Chemical Companies. Environ. Sci. Pollut. Res., 1–12. doi:10.1007/s11356-020-11636-9
Peng, B., Huang, Q., Elahi, E., and Wei, G. (2019a). Ecological Environment Vulnerability and Driving Force of Yangtze River Urban Agglomeration. Sustainability 11, 6623. doi:10.3390/su11236623
Peng, B., Wang, Y., Elahi, E., and Wei, G. (2019b). Behavioral Game and Simulation Analysis of Extended Producer Responsibility System's Implementation under Environmental Regulations. Environ. Sci. Pollut. Res. 26, 17644–17654. doi:10.1007/s11356-019-05215-w
Pishgar Komleh, S. H., Keyhani, A., Rafiee, S., and Sefeedpary, P. (2011). Energy Use and Economic Analysis of Corn Silage Production under Three Cultivated Area Levels in Tehran Province of Iran. Energy 36, 3335–3341. doi:10.1016/j.energy.2011.03.029
Pishgar-Komleh, S. H., Sefeedpari, P., and Ghahderijani, M. (2012). Exploring Energy Consumption and CO2 Emission of Cotton Production in Iran. J. Renew. Sust. Energ. 4, 033115. doi:10.1063/1.4727906
Rafiee, S., Mousavi Avval, S. H., and Mohammadi, A. (2010). Modeling and Sensitivity Analysis of Energy Inputs for Apple Production in Iran. Energy 35, 3301–3306. doi:10.1016/J.ENERGY.2010.04.015
Rehman, A., Chandio, A. A., Hussain, I., and Jingdong, L. (2019a). Fertilizer Consumption, Water Availability and Credit Distribution: Major Factors Affecting Agricultural Productivity in Pakistan. J. Saudi Soc. Agric. Sci. 18, 269–274. doi:10.1016/j.jssas.2017.08.002
Rehman, A., Jingdong, L., Chandio, A. A., Hussain, I., Wagan, S. A., and Memon, Q. U. A. (2019b). Economic Perspectives of Cotton Crop in Pakistan: A Time Series Analysis (1970-2015) (Part 1). J. Saudi Soc. Agric. Sci. 18, 49–54. doi:10.1016/j.jssas.2016.12.005
Rehman, A., Jingdong, L., Shahzad, B., Chandio, A. A., Hussain, I., Nabi, G., et al. (2015). Economic Perspectives of Major Field Crops of Pakistan: An Empirical Study. Pac. Sci. Rev. B: Humanities Soc. Sci. 1, 145–158. doi:10.1016/j.psrb.2016.09.002
Rezvani Moghaddam, P., Feizi, H., and Mondani, F. (2011). Evaluation of Tomato Production Systems in Terms of Energy Use Efficiency and Economical Analysis in Iran. Not. Sci. Biol. 3, 58–65. doi:10.15835/NSB346279
Rokicki, T., Perkowska, A., Klepacki, B., Bórawski, P., Bełdycka-Bórawska, A., and Michalski, K. (2021). Changes in Energy Consumption in Agriculture in the EU Countries. Energies 14, 1570. doi:10.3390/EN14061570
Saiki, T., Karaki, I., and Roy, K. (1999). CIGR Handbook of Agricultural Engineering, Volume V Energy and Biomass Engineering, Chapter 3 Biomass Engineering, Part 3.1 Biomass Liquid Fuels, Part 3.1.1 Ethanol and Methanol. CIGR Handbook Agric. Eng. Volume V Energ. Biomass Eng. 5, 139. doi:10.13031/2013.36417
Sarkar, M., and Seo, Y. W. (2021). Renewable Energy Supply Chain Management with Flexibility and Automation in a Production System. J. Clean. Prod. 324, 129149. doi:10.1016/J.JCLEPRO.2021.129149
Sepehri, A., Mishra, U., and Sarkar, B. (2021). A Sustainable Production-Inventory Model with Imperfect Quality under Preservation Technology and Quality Improvement Investment. J. Clean. Prod. 310, 127332. doi:10.1016/J.JCLEPRO.2021.127332
Shah, S. M., Liu, G., Yang, Q., Casazza, M., Agostinho, F., and Giannetti, B. F. (2021). Sustainability Assessment of Agriculture Production Systems in Pakistan: A Provincial-Scale Energy-Based Evaluation. Ecol. Model. 455, 109654. doi:10.1016/j.ecolmodel.2021.109654
Shen, D., Xia, M., Zhang, Q., Elahi, E., Zhou, Y., and Zhang, H. (2019). The Impact of Public Appeals on the Performance of Environmental Governance in China: A Perspective of Provincial Panel Data. J. Clean. Prod. 231, 290–296. doi:10.1016/j.jclepro.2019.05.089
Sheng, X., Peng, B., Elahi, E., and Wei, G. (2019). Regional Convergence of Energy-Environmental Efficiency: from the Perspective of Environmental Constraints. Environ. Sci. Pollut. Res. 26, 25467–25475. doi:10.1007/s11356-019-05749-z
Shittu, W., Adedoyin, F. F., Shah, M. I., and Musibau, H. O. (2021). An Investigation of the Nexus between Natural Resources, Environmental Performance, Energy Security and Environmental Degradation: Evidence from Asia. Resour. Pol. 73, 102227. doi:10.1016/J.RESOURPOL.2021.102227
Singh, G., Singh, S., and Singh, J. (2004). Optimization of Energy Inputs for Wheat Crop in Punjab. Energ. Convers. Manage. 45, 453–465. doi:10.1016/S0196-8904(03)00155-9
Singh, P., Singh, G., and Sodhi, G. P. S. (2019). Energy Auditing and Optimization Approach for Improving Energy Efficiency of rice Cultivation in South-Western Punjab, India. Energy 174, 269–279. doi:10.1016/j.energy.2019.02.169
Soni, P., and Soe, M. N. (2016). Energy Balance and Energy Economic Analyses of rice Production Systems in Ayeyarwaddy Region of Myanmar. Energy Efficiency 9, 223–237. doi:10.1007/S12053-015-9359-X
Steinbuks, J., and Hertel, T. W. (2014). Confronting the Food-Energy-Environment Trilemma: Global Land Use in the Long Run. Environ. Resource Econ. 63, 545–570. doi:10.1007/S10640-014-9848-Y
Tayyab, M., Jemai, J., Lim, H., and Sarkar, B. (2020). A Sustainable Development Framework for a Cleaner Multi-Item Multi-Stage Textile Production System with a Process Improvement Initiative. J. Clean. Prod. 246, 119055. doi:10.1016/J.JCLEPRO.2019.119055
Tsatsarelis, C. A. (1991). Energy Requirements for Cotton Production in central Greece. J. Agric. Eng. Res. 50, 239–246. doi:10.1016/S0021-8634(05)80017-4
Tu, Y., Peng, B., Wei, G., Elahi, E., and Yu, T. (2019). Regional Environmental Regulation Efficiency: Spatiotemporal Characteristics and Influencing Factors. Environ. Sci. Pollut. Res. 26, 37152–37161. doi:10.1007/s11356-019-06837-w
Ullah, A., Pinglu, C., Ullah, S., Zaman, M., and Hashmi, S. H. (2020). The Nexus between Capital Structure, Firm-specific Factors, Macroeconomic Factors and Financial Performance in the Textile Sector of Pakistan. Heliyon 6, e04741. doi:10.1016/j.heliyon.2020.e04741
Unakıtan, G., and Aydın, B. (2018). A Comparison of Energy Use Efficiency and Economic Analysis of Wheat and sunflower Production in Turkey: A Case Study in Thrace Region. Energy 149, 279–285. doi:10.1016/j.energy.2018.02.033
Walters, J. P., Archer, D. W., Sassenrath, G. F., Hendrickson, J. R., Hanson, J. D., Halloran, J. M., et al. (2016). Exploring Agricultural Production Systems and Their Fundamental Components with System Dynamics Modelling. Ecol. Model. 333, 51–65. doi:10.1016/j.ecolmodel.2016.04.015
Wei, W., Mushtaq, Z., Ikram, A., Faisal, M., Wan-Li, Z., and Ahmad, M. I. (2020). Estimating the Economic Viability of Cotton Growers in Punjab Province, Pakistan. SAGE Open 10, 215824402092931. doi:10.1177/2158244020929310
Yilmaz, I., Akcaoz, H., and Ozkan, B. (2005). An Analysis of Energy Use and Input Costs for Cotton Production in Turkey. Renew. Energ. 30, 145–155. doi:10.1016/j.renene.2004.06.001
Zhao, X., Peng, B., Elahi, E., Zheng, C., and Wan, A. (2020). Optimization of Chinese Coal-Fired Power Plants for Cleaner Production Using Bayesian Network. J. Clean. Prod. 273, 122837. doi:10.1016/j.jclepro.2020.122837
Zhao, Y., Peng, B., Elahi, E., and Wan, A. (2021). Does the Extended Producer Responsibility System Promote the green Technological Innovation of Enterprises? an Empirical Study Based on the Difference-In-Differences Model. J. Clean. Prod. 319, 128631. doi:10.1016/j.jclepro.2021.128631
Zhong, Z., Peng, B., and Elahi, E. (2021). Spatial and Temporal Pattern Evolution and Influencing Factors of Energy-Environmental Efficiency: A Case Study of Yangtze River Urban Agglomeration in China. Energ. Environ. 32, 242–261. doi:10.1177/0958305x20923114
Zhong, Z., Peng, B., Xu, L., Andrews, A., and Elahi, E. (2020). Analysis of Regional Energy Economic Efficiency and its Influencing Factors: A Case Study of Yangtze River Urban Agglomeration. Sustainable Energ. Tech. Assessments 41, 100784. doi:10.1016/j.seta.2020.100784
Keywords: energy input–output, GHG emission, crop management, cotton production, DEA
Citation: Abbas A, Zhao C, Waseem M, Ahmed khan K and Ahmad R (2022) Analysis of Energy Input–Output of Farms and Assessment of Greenhouse Gas Emissions: A Case Study of Cotton Growers. Front. Environ. Sci. 9:826838. doi: 10.3389/fenvs.2021.826838
Received: 01 December 2021; Accepted: 20 December 2021;
Published: 03 February 2022.
Edited by:
Ehsan Elahi, Shandong University of Technology, ChinaReviewed by:
Xinru Han, Institute of Agricultural Economics and Development (CAAS), ChinaCopyright © 2022 Abbas, Zhao, Waseem, Ahmed khan and Ahmad. This is an open-access article distributed under the terms of the Creative Commons Attribution License (CC BY). The use, distribution or reproduction in other forums is permitted, provided the original author(s) and the copyright owner(s) are credited and that the original publication in this journal is cited, in accordance with accepted academic practice. No use, distribution or reproduction is permitted which does not comply with these terms.
*Correspondence: Chengyi Zhao, emhhb2N5QG51aXN0LmVkdS5jbg==
Disclaimer: All claims expressed in this article are solely those of the authors and do not necessarily represent those of their affiliated organizations, or those of the publisher, the editors and the reviewers. Any product that may be evaluated in this article or claim that may be made by its manufacturer is not guaranteed or endorsed by the publisher.
Research integrity at Frontiers
Learn more about the work of our research integrity team to safeguard the quality of each article we publish.