- School of Public Policy and Management, University of Chinese Academy of Sciences, Beijing, China
The carbon emission trading scheme (ETS) is an essential policy tool for accomplishing Chinese carbon targets. Based on the Chinese provincial panel data from 2003 to 2019, an empirical study is conducted to measure the effects of carbon emission reduction and spatial spillover effect by adopting the difference-in-differences (DID) model and spatial difference-in-differences (SDID) model. The research findings show that: 1) The ETS effectively reduced the total carbon emissions as well as emissions from coal consumption; 2) such effects come mainly from the reduction of coal consumption and the optimization of energy structure, rather than from technological innovation and optimization of industrial structure in the pilot regions; and 3) the ETS pilot regions have a positive spatial spillover effect on non-pilot regions, indicating the acceleration effect for carbon emission reduction. Geographic proximity makes the spillover effect decrease due to carbon leakage.
1 Introduction
Net zero is a necessary step to mitigate global warming and has become an international consensus. In confronting climate change, the Paris Agreement stipulated objectives to limit the rise of global average temperature within 1.5 and 2°C (UNFCCC, 2015). The Intergovernmental Panel on Climate Change then addressed this act by setting the goal “to achieve global carbon neutrality around 2050, leading to an eventual 1.5°C global warming target by 2100” (IPCC, 2018). However, the published national reduction contributions under the Paris Agreement continue to face challenges in meeting the 1.5°C temperature control target (Duan et al., 2021). Most countries have pledged carbon neutrality targets through legal provisions and policy declarations. As one of the largest energy consumption countries, China signed the Paris Climate Accord and pledged to “peak emission by 2030 and reaching carbon neutral by 2060.” However, China’s total carbon emissions reached 929 million tons in 2019, approximately one-third of total global emissions, and its coal consumption accounted for nearly 60% of the country’s total energy consumption (Wang and Yang, 2021). “Going carbon neutral” in China requires implementation of a combination of policies across the next 4 decades. At the early stages, the focus should be set on achieving optimal energy structures, deepening industrial structural reform, and constructing a green industrial chain (Huang and Zhai, 2021). In mid-to-late stages, attention should be shifted to fossil energy decommissioning and development of technologies related to Carbon Capture and Storage (CCS) (Xu et al., 2021) and Bioenergy Combined with Carbon Capture and Storage (BECCS) (Huang et al., 2020).
The Carbon Emission Trading Scheme (ETS) provides price signals for carbon emission reduction, creates an essential path towards carbon neutrality, and mitigates global warming (Wang et al., 2020). In October 2011, China carried out measures to promote the ETS, authorizing seven provinces and cities to launch pilot projects in carbon emissions trading. In 2021, the long-awaited national carbon emissions trading market was officially launched in China, establishing the world’s largest greenhouse gas (GHG) emissions trading market.
Past research focused on the ETS in China through two streams: ex ante analysis and ex post analysis. Ex ante analysis develops Computable General Equilibrium (CGE) models (Lin and Jia, 2018), the Integrated Assessment Model (IAM) (Zhao et al., 2020), or Agent- Based Model (ABM) (Tang et al., 2017) to study the influence of the ETS parameters on emission reduction effect and economic development. Topics discussed involved initial allocation, coverages, reasonable carbon price range, effects on carbon emissions, economic costs, and the interactions between the ETS and other complementing policies (Li et al., 2017; Wu and Li, 2020; Weng et al., 2021). The results of ex ante analysis are potentially affected by multi-parameter settings and model assumptions.
The ex post analysis is focused on assessing operational effectiveness and the impact of such mechanisms based on historical archival data. Most studies concluded that the ETS is an effective policy tool in carbon emissions reduction at provincial, municipal, and corporate levels (Gao et al., 2020; Shen et al., 2020; Zhang W. et al., 2020; Zhang Y. et al., 2020), The emission reduction effect increases annually with the implementation of the policy (Zhang and Zhang, 2019). Some researchers suggest that such action also reduces other pollutants such as PM2.5 and SO2, promoting China’s low-carbon innovation and economic development and finally bringing in more environmental dividends (Feng et al., 2021b; Liu et al., 2021). Wang et al. (2020) adopted the difference-in-differences (DID) model to demonstrate a positive correlation between the ETS and low-carbon economic transformation; however, researchers also argued that the ETS may improve the efficiency of emission reduction, but the actual effect remains relatively weak (Zhu et al., 2020). Due to the immaturity of the regulations and market systems for the ETS pilots (Qi et al., 2021), the ETS market lacks vitality. Such efficiency of emission reduction has slowed (Zhu et al., 2020). Studies on the impact mechanism of the European Union Emissions Trading System (EU ETS) have argued that the EU ETS reduces carbon emissions mainly through technological innovation (Jaraitė and Di Maria, 2012; Calel and Dechezleprêtre, 2016). In a similar scenario, certain Chinese scholars have argued that China’s ETS reduces carbon emissions by reducing energy consumption and optimizing energy structure, while the emission reduction effect of technological innovation is not significant (Xuan et al., 2020; Liu et al., 2021).
The emission reduction effects of the pilot regions provide a direct signal and information for the implementation of China’s ETS, therefore, this study analyzes the effect of the ETS through six pilot regions. Unlike European countries, coal consumption took a dominating role in the current Chinese energy consumption structure (Wang and Yang, 2021). The ETS will be the main driver in supporting China’s carbon neutrality goal. Few studies have analyzed the impact of the ETS on carbon emissions caused by coal consumption, and no empirical evidence has shown the effect and extent of the ETS in coal removal process.
A growing number of studies have focused on the spatial spillover effect and the carbon leakage caused by the ETS. These ex ante analyses were adopted by CGE models or IAM (Antimiani et al., 2016; Yu et al., 2021). Researchers argued that the EU ETS leads to carbon leakage, shifting carbon emissions from regions with strong environmental constraints to those with weak environmental constraints (Paroussos et al., 2015; Böhringer et al., 2017). Similarly, some scholars have demonstrated that carbon leakage is inevitable in China’s ETS using CGE model (Tan et al., 2018; Wang et al., 2018), but the simulation results of the ex ante analysis depend heavily on the model setup and the assumptions of the model (Yu et al., 2021). Such methods often underestimate or ignore the efforts of non-pilot regions in carbon emissions reduction.
Empirical analysis on the ex-post spatial spillover effect and carbon leakage are inadequate. As policy implementation increases, more attention has shifted to this area of study. Naegele and Zaklan (2019) find no evidence supporting that the EU ETS leads to carbon leakage based on evidence from European manufacturing development data. Some scholars used the DID model based on the historical data of the ETS pilot regions to test its positive spatial spillover effect. In particular, Liu et al. (2021) adopted the DID model to investigate whether cities adjacent to pilot cities also benefit from the implementation of the ETS and if there is a positive spillover effect of the ETS observed in reducing air pollution. Significantly, Zhou et al. (2020) shows that the ETS can lead to reverse carbon leakage. Pilot regions with larger industries have more industrial transfers in contrast to non-pilot regions, leading to a shift of carbon emissions from non-pilot to pilot regions. However, these empirical findings are far from sufficient to provide ex post data. Local pilots and national carbon markets will co-exist in China with heterogenetic intensity of environmental legislation across regions. Analyzing the existence of a spatial spillover effect of the ETS and the direction of spillover is vital.
As observed, the DID model are widely used to study the emission reduction effect and spatial spillover effect of the ETS, without considering spatial interactions among units. The DID model violates the SUTVA hypothesis of independent and identical distribution, which says one unit should not be affected by the treatment of the other (Cox, 1958; Rosenbaum, 2010), This issue will lead to bias or potential error. Chagas et al. (2016) determined that by performing a treatment group and control group between spatial decomposition, the spatial difference-in-differences (SDID) model was shown to be effective in addressing this challenge, and it is gradually being adopted in the corresponding analyses (Feng et al., 2021a; Yu and Zhang, 2021).
This paper applies both the DID model and SDID model to respond to whether the ETS is effective in the reduction of total carbon emissions and these from carbon emissions. What is the impact of the mechanism? How much is the spatial spillover effect? The main contributions of this research lie in the following three aspects: 1) we analyzed the impact of the ETS on total carbon emissions and those caused by coal consumption, focusing on the effects of the ETS on coal removal; 2) we adopted the DID model to evaluate the emission reduction effect and the impact mechanism of the ETS; 3) we adopted the SDID model to analyze the spatial spillover effect of the policy and its stimulating effect on carbon leakage. The results are more robust and expand the spatial perspective for the study of policy impact effects. The rest of the paper is organized as following: Section 2 explains the model setup and the selection of variables; Sections 3, 4 show the empirical processes and results; and Section 5 concludes the paper and offers corresponding policy recommendations.
2 Model Design and Data Description
2.1 Difference-in-Differences Model
The data sample is divided into the treatment and control groups. The treatment group (Policy = 1) is made up of the two pilot provinces and four municipalities directly under the central government that implemented the ETS, and the control group (Policy = 0) is made up of the non-pilot provinces and autonomous regions. Official approval of the ETS implementation was granted in 2011, and the actual year of implementation was 2013. Therefore, Post-2013 (2013 included) is the policy implementation period (Post = 1) and pre-2013 is the non-policy period (Post = 0). Therefore, DID estimation is specified as following:
where
2.2 Spatial Difference-in-Differences Model
To study spatial spillover effect of ETS, the SDID estimation was carried out using a spatial lag model (SLX) (Chagas et al., 2016). The spatial spillover effect of the ETS can be correctly estimated for both pilot and non-pilot regions. This produces the following SDID specifications:
where
The spatial distance weight matrix
2.3 Variables Description
Restricted by data availability of the balance sheets and missing data at the provincial level, this paper focuses on carbon emissions from coal consumption. The sample included data from 29 provinces and municipalities from 2003 to 2019, excluding Hong Kong, Macao, Taiwan, Fujian Province, and Tibet Autonomous Region due to the special research setting. The ETS in Fujian Province was established in 2017, which is different from other pilot regions and the pilot operation period is relatively short, so Fujian Province is excluded from the sample in this paper. Original data were obtained from China Urban Statistical Yearbook and China Energy Statistical Yearbook from 2004 to 2020.
1) Dependent Variable: Total carbon emissions and those from coal consumption. Based on the energy balance sheets of 29 provinces, the total carbon emissions and those from coal combustion are aggregated by multiplying the amount of energy consumed by the average low level of heat generation, the CO2 oxidation factor, and the oxidation rate based on varied energy sources. This research excludes the double-counting of consumption during energy conversion. Finally, the two dependent variables are normalized by logarithms and denoted as
2) Treatment Variable (did): an interaction term of the pilot policy dummy variable and the year dummy variable: pilot regions receive valued of 1 during the post-2013 period. Non-pilot regions and those pre-2013 pilot regions receive the value of 0.
3) Control Variables: Based on previous literature, the following variables have been included: Industrialization level (IL): share of secondary sector in GDP of each province; economic development level (lnPGDP): logarithm of the real GDP of each province (based on 2003) as a share of the total population at the end of the year; Population density (lnPOP): logarithm of the total population of each province at the end of the year as a proportion of the geographical region of the province; international direct investment (FDI): share of foreign direct investment in each province in the GDP of the year; service industry development level (SL): share of tertiary industry in the GDP of each province; degree of openness to the world (IET): share of the total trade of import and export of each province to the GDP of the year.
4) Mediators: Mediators adopted to study the mechanism of the impact of ETS are shown as follows: industrial structure (IL): share of value added of secondary industry in GDP; technological innovation (RD): share of R&D expenditures in GDP; energy structure (ES); share of coal consumption in total energy consumption; coal consumption (lnCOAL): logarithm of coal consumption in each province. Table 1 shows the descriptive statistics.
3 Empirical Results
3.1 Baseline Regression
The estimated results of ETS abatement effects are shown in Table 2; columns 1–2 show the results of the baseline regression of the ETS on total carbon emissions. Column 2 adds the control variables to the baseline model. The results show that the coefficients of did in columns 1–2 are negative and statistically significant at the 1% level, suggesting that the ETS has significantly reduced the total carbon emissions. The policy effect is significant. Columns 3–4 show the results of the baseline regression of the ETS on carbon emissions from coal combustion. Column 4 adds control variables to Column 3. The results show that the coefficients of did in columns 3–4 are negative and statistically significant at the 5 and 1% levels, respectively. The absolute value of the regression coefficient of did in column 4) is larger than the absolute value of the regression coefficient of did in column (2). Results suggest that under the ETS, reducing carbon emissions from coal combustion has a larger effect than that of total carbon emissions. Possible explanations might be that most of the pilot firms of the ETS are located in high energy-consuming industries. Coal dominates their energy consumption structure, and these firms prioritize the reduction of carbon emissions by reducing coal consumption. To support this conclusion, we analyzes the effects of ETS on carbon emissions from petroleum and natural gas consumption separately, and the results are shown in Supplementary Table S1. The results show that the coefficients of did is not significant, indicating that the implementation of ETS does not affect the use of these two energy sources, but has a significant effect on reducing coal consumption; our results provide evidence for the beneficial side of the ETS in the process of coal removal in the pilot regions.
3.2 Parallel Trend Test
DID model is valid only if the parallel trend hypothesis is satisfied. The hypothesis, when satisfied, indicates that the carbon emission trends in the pilot and non-pilot regions are homogeneous. They do not differ significantly before the ETS is implemented, while the emission reduction impact of the ETS only occurs after the policy is implemented. Therefore, this paper establishes a regression model to test the parallel trend hypothesis, and the model structure is shown in :
We set 2013 as the base year.
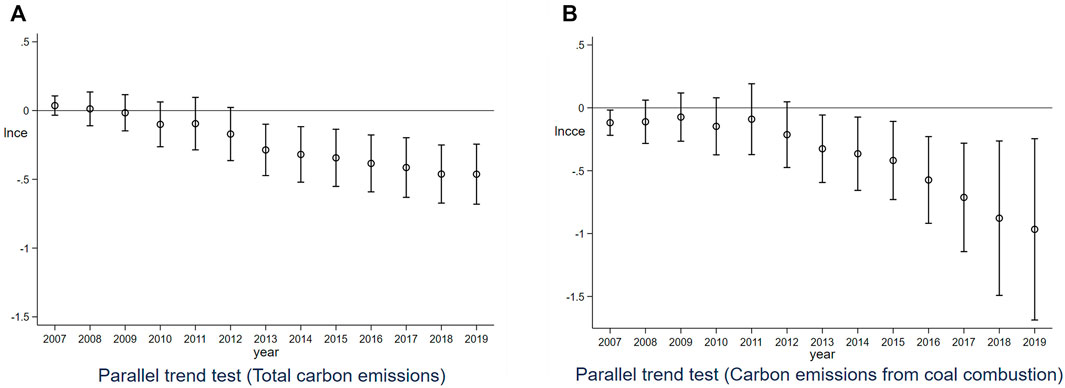
FIGURE 1. Parallel trend test. (A) Parallel trend test (Total carbon emissions). (B) Parallel trend test (Carbon emissions from coal combustion).
3.3 Placebo Test
Other unobserved regional variables affecting carbon emissions are possible in this study due to data availability issue, and this may result in estimation bias. Therefore, a placebo test needs to be applied to verify whether the omitted and unobserved variables affect the baseline results. The placebo test is adopted to test the robustness of the baseline regression through the random selection of several dummy experimental groups in full samples to perform a regression consistent with the baseline regression (Chetty et al., 2009). Specifically, all samples were sampled 1,000 times in our study. Six regions were randomly selected as the dummy experimental group for each sampling. The rest of the samples were regressed as the control group according to Eq. 1 to obtain the regression coefficients and p-values.
Figure 2 reports the distribution of regression coefficients, where the x-axis represents the regression coefficients from the 1,000 randomly assigned experimental groups of did, and the curve is the kernel density distribution of the regression coefficients. The blue dots are the p-values corresponding to the regression coefficients. The red dashed lines present the true regression coefficients with p-values for columns (2) and (4) of Table 2, respectively. It is observed that the distribution of the coefficients, estimated based on the random sample, is around 0 with p-values greater than 0.1. The coefficients estimated from the baseline regression are almost independent according to the coefficient distribution. This is in line with Placebo Test’s expectation. Thus, the significant abatement impact of the ETS is unlikely to be influenced by unobserved factors, and previous estimation analysis are robust.
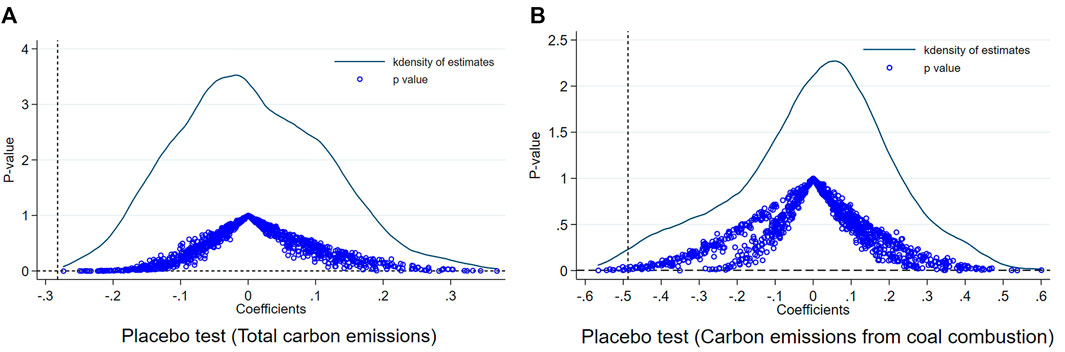
FIGURE 2. Placebo test. (A) Placebo test (Total carbon emissions). (B) Placebo test (Carbon emissions from coal combustion).
3.4 Instrumental Variable Approach
When studying the effect of ETS on CO2 emissions using DID analysis, the presumed assumption is that the selection process of the ETS pilot regions is random and maintains invariant when changes occur in other potential factors. However, pilot regions are not selected randomly but rather chosen by policy. To prevent the influence of other unobserved potential factors, this paper draws on Tsoutsoura (2011) approach in adopting the instrumental variable approach to address the endogeneity issue of experimental group selection.
Specifically, this paper follows Hering and Poncet (2014) in selecting the ventilation coefficient as an instrumental variable to explain whether a pilot regions has the policy treatment. First, for regions with smaller ventilation coefficients, larger pollutant concentrations are monitored and will incentivize local government to adopt more aggressive and effective environmental regulation policies. This region then gains a better chance to be selected as an ETS pilot. The regression results of the ventilation coefficient and the region selected as a ETS pilot are negatively correlated. Thus, the selection of ventilation coefficient as an instrumental variable satisfies the correlation hypothesis. In addition, because the ventilation coefficient is determined by the meteorological and geographical conditions of each region, it satisfies the exogeneity assumption. In this study, we match the boundary layer height and wind speed information at 10 m height from the ERA dataset of the European Centre for Medium-Range Weather Forecasts to the latitude and longitude of the 29 provinces and cities in the sample. The multiplication of wind speed and boundary layer height for each cell is the circulation coefficient. We then normalized through logarithm of circulation coefficients for 29 sample regions from 2003 to 2019 is selected.
The results of instrumental variable are shown in Table 3. Column (1) is listed as the regression results of the first stage: the regression coefficient of the interaction term iv*post is significantly negative at the 5% level, and the F-statistic is greater than the critical value of 10, indicating that the instrumental variable satisfies the correlation condition and there is no weak instrumental variable. In the second-stage regression, the regression coefficient of did is still significantly negative, which is consistent with the baseline regression, indicating that after eliminating the endogeneity problem in pilot selection, the ETS can still significantly reduce total carbon emissions and carbon emissions from coal combustion. The previous findings remain robust.
4 Further Analysis
4.1 Analysis of Impact Mechanism
Previous analysis in our study shows that the ETS has had a significant abatement effect on the local pilot. Next, this paper uses a two-stage mediating effect model for validation (Baron and Kenny, 1986) to initially analyze the mechanism of the abatement effect generated by the ETS. The mediating effect model is established as follows:
where M denotes the mediators including industrial structure (IL), technological innovation (RD), energy structure (ES), and coal consumption (lnCOAL). Other model settings are consistent with Eq. 1, and the coefficients of the treatment variable did in Eq. 4 and the coefficients of the mediators
The regression coefficients of
In summary, the reduction effect of ETS comes mainly from the absolute emission reduction from coal combustion and its share in the energy structure, rather than from the technological innovation and optimization of the industrial structure in the pilot regions.
4.2 Analysis of Spatial Spillover Effect
Based on Eq. 2, the SDID model under the spatial distance weight matrix
The regression coefficients of did are still significantly negative upon consideration of the spatial effect. The absolute values of the regression coefficients of
ETS has two possible pathways in affecting the non-pilot regions’ reduction activities. On one hand, the establishment of the ETS pilot regions is a prelude of the national carbon market, and the non-pilot regions may have realized China’s determination to reduce emissions through the implementation of the ETS; thus, the ETS pilot regions could act as role models to stimulate the non-pilot regions’ reduction activities. On the other hand, pilot regions could crowd out high emission production activities to non-pilot regions, thus promoting the increase of carbon emissions in these regions. In Table 5, the regression coefficient of
To investigate the spatial spillover effect of the ETS on different range regions, the SDID model adopts the local spatial distance weight matrixes. The results are shown in Table 6.
The regression coefficients of did remain significantly negative. As shown in column (1), the regression coefficient of
5 Discussion
Based on the panel data of Chinese ETS pilot regions and non-pilot regions from 2003 to 2019, this paper examines the emission reduction effect of the ETS using the DID model. Further analysis on the impact mechanism and spatial spillover effect of the policy is also conducted. The findings are as follows: 1) the ETS can effectively reduce the total carbon emissions and emissions from coal consumption. The relative effect of carbon emissions from coal consumption appears to be better, suggesting an acceleration effect of the ETS in coal removal. 2) China is at the initial stage of moving towards carbon neutrality, and the reduction effect of the ETS comes mainly from the absolute reduction of coal consumption and its share in the energy structure. 3) The ETS has a positive spatial spillover effect, which drives other non-pilot regions to commit to carbon reduction activities through role-modeling effect. The ETS may incentivize pilot regions to crowd out production activities to neighboring non-pilot regions, increased the carbon emissions and thus hindering the carbon reduction process in neighboring regions.
Based on these conclusions, the following recommendations are made. First, China’s goal for 2060 carbon neutrality will require the full use of the ETS to reduce emissions and coal removal. With more stringent emission reduction targets, the carbon trading mechanism is to be designed for coverage of a wide range of sources, such as involving sectors and units with a high level of electricity, petroleum and gas consumption. Second, the government should continue to improve the design of the national carbon market. Promote the synergistic development of the local carbon market with the national carbon market to ensure that the ETS can effectively achieve emission reduction effect and positive spatial spillover effect. Then, regional governments should strengthen their interaction and the national carbon market should balance the intensity of the policies of each region to prevent the transfer of high-carbon emitting industries to neighboring regions and thus the occurrence of carbon leakage. Finally, to give the full play to the effect of the carbon market, governments should focus on a high-quality development model and reduce consumption of coal and other energy. Although the reduction emission effect of the ETS does not come from technological innovation and optimization of industrial structure, in order to achieve the long-term goal of carbon neutrality, the government still needs to stimulate the innovation drive of enterprises, encourage the development of low-carbon green technology, improve energy utilization efficiency, and promote industrial structure optimization. Through the above measures, with the support of carbon trading mechanism, the emission reduction effect will be significantly improved.
Restricted to the availability of the data and methods, there are three main limitations in this paper. 1) This paper focuses on the impact of the ETS on emissions from coal consumption, but due to the limitation of coal consumption data, it cannot be studied at the city level. 2) The ETS has synergy effects with various policies, such as the air control policy and the vigorous development of clean energy policy. It is also worthwhile to consider the clean effect of the carbon emission reduction policy upon the removal of the effects of these competing policies. 3) This paper focuses on the carbon emission reduction effect of the ETS and the impact factors but does not further measure the cost of emission reduction policies. Comparative analysis among emission reduction effects and the cost of different carbon markets and the cost of carbon emission reduction of different policies are important research directions for future carbon emission reduction policy analysis.
Data Availability Statement
Publicly available datasets were analyzed in this study. This data can be found here: www.stats.gov.cn/tjsj/.
Author Contributions
ZY: conceptualized the study, analyzed the data and led drafting the manuscript. YY: contributed to the drafting of the manuscript, editing and Supervision QZ: contributed to the drafting of the manuscript and writing-reviewing.
Funding
This study was supported by funds from National Natural Science Foundation of China (Grant Nos. 71673263, 72174190) and the Fundamental Research Funds for the Central Universities.
Conflict of Interest
The authors declare that the research was conducted in the absence of any commercial or financial relationships that could be construed as a potential conflict of interest.
Publisher’s Note
All claims expressed in this article are solely those of the authors and do not necessarily represent those of their affiliated organizations, or those of the publisher, the editors, and the reviewers. Any product that may be evaluated in this article, or claim that may be made by its manufacturer, is not guaranteed or endorsed by the publisher.
Supplementary Material
The Supplementary Material for this article can be found online at: https://www.frontiersin.org/articles/10.3389/fenvs.2021.824298/full#supplementary-material
References
Antimiani, A., Costantini, V., Kuik, O., and Paglialunga, E. (2016). Mitigation of Adverse Effects on Competitiveness and Leakage of Unilateral EU Climate Policy: An Assessment of Policy Instruments. Ecol. Econ. 128, 246–259. doi:10.1016/j.ecolecon.2016.05.003
Baron, R. M., and Kenny, D. A. (1986). The Moderator-Mediator Variable Distinction in Social Psychological Research: Conceptual, Strategic, and Statistical Considerations. J. Personal. Soc. Psychol. 51, 1173–1182. doi:10.1037/0022-3514.51.6.1173
Böhringer, C., Bye, B., Fæhn, T., and Rosendahl, K. E. (2017). Targeted Carbon Tariffs: Export Response, Leakage and Welfare. Resource Energ. Econ. 50, 51–73. doi:10.1016/j.reseneeco.2017.06.003
Calel, R., and Dechezleprêtre, A. (2016). Environmental Policy and Directed Technological Change: Evidence from the European Carbon Market. Rev. Econ. Stat. 98, 173–191. doi:10.1162/REST_a_00470
Chagas, A. L. S., Azzoni, C. R., and Almeida, A. N. (2016). A Spatial Difference-In-Differences Analysis of the Impact of Sugarcane Production on Respiratory Diseases. Reg. Sci. Urban Econ. 59, 24–36. doi:10.1016/j.regsciurbeco.2016.04.002
Chetty, R., Looney, A., and Kroft, K. (2009). Salience and Taxation: Theory and Evidence. Am. Econ. Rev. 99 (34), 1145–1177. doi:10.1257/aer.99.4.1145
Duan, H., Zhou, S., Jiang, K., Bertram, C., Harmsen, M., Kriegler, E., et al. (2021). Assessing China's Efforts to Pursue the 1.5°C Warming Limit. Science 372, 378–385. doi:10.1126/science.aba8767
Feng, Y., Wang, X., and Hu, S. (2021a). Accountability Audit of Natural Resource, Air Pollution Reduction and Political Promotion in China: Empirical Evidence from a Quasi-Natural experiment. J. Clean. Prod. 287, 125002. doi:10.1016/j.jclepro.2020.125002
Feng, Y., Wang, X., Liang, Z., Hu, S., Xie, Y., and Wu, G. (2021b). Effects of Emission Trading System on green Total Factor Productivity in China: Empirical Evidence from a Quasi-Natural experiment. J. Clean. Prod. 294, 126262. doi:10.1016/j.jclepro.2021.126262
Gao, Y., Li, M., Xue, J., and Liu, Y. (2020). Evaluation of Effectiveness of China's Carbon Emissions Trading Scheme in Carbon Mitigation. Energ. Econ. 90, 104872. doi:10.1016/j.eneco.2020.104872
Hering, L., and Poncet, S. (2014). Environmental Policy and Exports: Evidence from Chinese Cities. J. Environ. Econ. Manage. 68, 296–318. doi:10.1016/j.jeem.2014.06.005
Huang, M.-T., and Zhai, P.-M. (2021). Achieving Paris Agreement Temperature Goals Requires Carbon Neutrality by Middle century with Far-Reaching Transitions in the Whole Society. Adv. Clim. Change Res. 12, 281–286. doi:10.1016/j.accre.2021.03.004
Huang, X., Chang, S., Zheng, D., and Zhang, X. (2020). The Role of BECCS in Deep Decarbonization of China's Economy: A Computable General Equilibrium Analysis. Energ. Econ. 92, 104968. doi:10.1016/j.eneco.2020.104968
IPCC (2018). Global Warming of 1.5°C. An IPCC Special Report on the Impacts of Global Warming of 1.5°C above Pre-industrial Levels and Related Global Greenhouse Gas Emission Pathways. Available at: https://www.ipcc.ch/sr15/.
Jaraitė, J., and Di Maria, C. (2012). Efficiency, Productivity and Environmental Policy: A Case Study of Power Generation in the EU. Energ. Econ. 34, 1557–1568. doi:10.1016/j.eneco.2011.11.017
Li, J., Tan, Q., and Bai, J. (2010). Spatial Econometric Analysis of Regional Innovation Production in China - An Empirical Study Based on Static and Dynamic Spatial Panel Models. Management World 7, 43–65. doi:10.19744/j.cnki.11-1235/f.2010.07.006
Li, W., Jia, Z., and Zhang, H. (2017). The Impact of Electric Vehicles and CCS in the Context of Emission Trading Scheme in China: A CGE-Based Analysis. Energy 119, 800–816. doi:10.1016/j.energy.2016.11.059
Lin, B., and Jia, Z. (2018). Impact of Quota Decline Scheme of Emission Trading in China: A Dynamic Recursive CGE Model. Energy 149, 190–203. doi:10.1016/j.energy.2018.02.039
Liu, J.-Y., Woodward, R. T., and Zhang, Y.-J. (2021). Has Carbon Emissions Trading Reduced PM2.5 in China? Environ. Sci. Technol. 55, 6631–6643. doi:10.1021/acs.est.1c00248
Naegele, H., and Zaklan, A. (2019). Does the EUETS Cause Carbon Leakage in European Manufacturing? J. Environ. Econ. Management 93, 125–147. doi:10.1016/j.jeem.2018.11.004
Paroussos, L., Fragkos, P., Capros, P., and Fragkiadakis, K. (2015). Assessment of Carbon Leakage through the Industry Channel: The EU Perspective. Technol. Forecast. Soc. Change 90, 204–219. doi:10.1016/j.techfore.2014.02.011
Qi, S., Cheng, S., and Cui, J. (2021). Environmental and Economic Effects of China's Carbon Market Pilots: Empirical Evidence Based on a DID Model. J. Clean. Prod. 279, 123720. doi:10.1016/j.jclepro.2020.123720
Shen, J., Tang, P., and Zeng, H. (2020). Does China's Carbon Emission Trading Reduce Carbon Emissions? Evidence from Listed Firms. Energ. Sustainable Development 59, 120–129. doi:10.1016/j.esd.2020.09.007
Tan, X., Liu, Y., Cui, J., and Su, B. (2018). Assessment of Carbon Leakage by Channels: An Approach Combining CGE Model and Decomposition Analysis. Energ. Econ. 74, 535–545. doi:10.1016/j.eneco.2018.07.003
Tang, L., Wu, J., Yu, L., and Bao, Q. (2017). Carbon Allowance Auction Design of China's Emissions Trading Scheme: A Multi-Agent-Based Approach. Energy Policy 102, 30–40. doi:10.1016/j.enpol.2016.11.041
Tsoutsoura, M. (2011). The Effect of Succession Taxes on Family Firm Investment: Evidence from a Natural Experiment. SSRN J. doi:10.2139/ssrn.1971038
UNFCCC (2015). Adoption of the Paris Agreement. Report No. FCCC/CP/2015/L.9/Rev.1. Available at: http://unfccc.int/resource/docs/2015/cop21/eng/l09r01.pdf.
Wang, P.-T., Wei, Y.-M., Yang, B., Li, J.-Q., Kang, J.-N., Liu, L.-C., et al. (2020). Carbon Capture and Storage in China's Power Sector: Optimal Planning under the 2 °C Constraint. Appl. Energ. 263, 114694. doi:10.1016/j.apenergy.2020.114694
Wang, Q., and Yang, X. (2021). New Insight into Aggressive Intended Nationally Determined Contributions in China - What Lessons China Should Learn from Germany to Reduce Production-Based Carbon Emission. J. Clean. Prod. 279, 123522. doi:10.1016/j.jclepro.2020.123522
Wang, X., Teng, F., Wang, G., Zhou, S., and Cai, B. (2018). Carbon Leakage Scrutiny in ETS and Non-ETS Industrial Sectors in China. Resour. Conservation Recycling 129, 424–431. doi:10.1016/j.resconrec.2016.09.017
Weng, Y., Cai, W., and Wang, C. (2021). Evaluating the Use of BECCS and Afforestation under China's Carbon-Neutral Target for 2060. Appl. Energ. 299, 117263. doi:10.1016/j.apenergy.2021.117263
Wicki, S., and Hansen, E. G. (2019). Green Technology Innovation: Anatomy of Exploration Processes from a Learning Perspective. Bus Strat Env 28, 970–988. doi:10.1002/bse.2295
Wu, Q., and Li, C. (2020). How Quota Allocation Affects the Unified ETS of China: a Simulation with Dynamic CGE Model. Environ. Sci. Pollut. Res. 27, 1835–1851. doi:10.1007/s11356-019-06588-8
Xu, C., Yang, J., He, L., Wei, W., Yang, Y., Yin, X., et al. (2021). Carbon Capture and Storage as a Strategic reserve against China's CO2 Emissions. Environ. Development 37, 100608. doi:10.1016/j.envdev.2020.100608
Xuan, D., Ma, X., and Shang, Y. (2020). Can China's Policy of Carbon Emission Trading Promote Carbon Emission Reduction? J. Clean. Prod. 270, 122383. doi:10.1016/j.jclepro.2020.122383
Yu, B., Zhao, Q., and Wei, Y.-M. (2021). Review of Carbon Leakage under Regionally Differentiated Climate Policies. Sci. Total Environ. 782, 146765. doi:10.1016/j.scitotenv.2021.146765
Yu, Y., and Zhang, N. (2021). Low-carbon City Pilot and Carbon Emission Efficiency: Quasi-Experimental Evidence from China. Energ. Econ. 96, 105125. doi:10.1016/j.eneco.2021.105125
Zhang, W., Li, J., Li, G., and Guo, S. (2020). Emission Reduction Effect and Carbon Market Efficiency of Carbon Emissions Trading Policy in China. Energy 196, 117117. doi:10.1016/j.energy.2020.117117
Zhang, Y. J., Shi, W., and Jiang, L. (2020). Does China's Carbon Emissions Trading Policy Improve the Technology Innovation of Relevant Enterprises? Bus Strat Env 29, 872–885. doi:10.1002/bse.2404
Zhang, Y., Li, S., Luo, T., and Gao, J. (2020). The Effect of Emission Trading Policy on Carbon Emission Reduction: Evidence from an Integrated Study of Pilot Regions in China. J. Clean. Prod. 265, 121843. doi:10.1016/j.jclepro.2020.121843
Zhang, Y., and Zhang, J. (2019). Estimating the Impacts of Emissions Trading Scheme on Low-Carbon Development. J. Clean. Prod. 238, 117913. doi:10.1016/j.jclepro.2019.117913
Zhao, Z.-J., Chen, X.-T., Liu, C.-Y., Yang, F., Tan, X., Zhao, Y., et al. (2020). Global Climate Damage in 2 °C and 1.5 °C Scenarios Based on BCC_SESM Model in IAM Framework. Adv. Clim. Change Res. 11, 261–272. doi:10.1016/j.accre.2020.09.008
Zhou, B., Zhang, C., Wang, Q., and Zhou, D. (2020). Does Emission Trading lead to Carbon Leakage in China? Direction and Channel Identifications. Renew. Sustainable Energ. Rev. 132, 110090. doi:10.1016/j.rser.2020.110090
Keywords: carbon emission trading scheme, coal removal, spatial spillover effect, carbon emissions reduction effects, SDID
Citation: Yang Z, Yuan Y and Zhang Q (2022) Carbon Emission Trading Scheme, Carbon Emissions Reduction and Spatial Spillover Effects: Quasi-Experimental Evidence From China. Front. Environ. Sci. 9:824298. doi: 10.3389/fenvs.2021.824298
Received: 29 November 2021; Accepted: 13 December 2021;
Published: 04 January 2022.
Edited by:
Syed Jawad Hussain Shahzad, Montpellier Business School, FranceReviewed by:
Bin Zhang, Beijing Institute of Technology, ChinaYouguo Zhang, Institute of Quantitative and Technical Economics (CASS), China
Copyright © 2022 Yang, Yuan and Zhang. This is an open-access article distributed under the terms of the Creative Commons Attribution License (CC BY). The use, distribution or reproduction in other forums is permitted, provided the original author(s) and the copyright owner(s) are credited and that the original publication in this journal is cited, in accordance with accepted academic practice. No use, distribution or reproduction is permitted which does not comply with these terms.
*Correspondence: Yongna Yuan, anVsaWF5bnlAdWNhcy5lZHUuY24=