- 1School of Economics, Tianjin University of Commerce, Tianjin, China
- 2School of Economics, Nankai University, Tianjin, China
This study aims to shed light on the effects of environmental regulation on China’s eco-innovation driven by investor sentiment and public sentiment from 2003 to 2017. Adopting a text analysis technique and LSTM model, this study identifies the investor sentiment and public sentiment by online comments. We find that environmental regulation has a positive effect on China’s eco-innovation through public sentiment, whereas environmental regulation inhibits China’s eco-innovation through investor sentiment. Second, the regulatory tools of environmental regulation not only improve the public’s enthusiasm and awareness of environmental protection and eco-innovation in China but also bring the pressure to investor survival and continuous innovation. Third, we confirm that the environmental regulation has threshold effects on general public sentiment and investor sentiment for affecting China’s eco-innovation. Based on this result, environmental regulation easily triggers public sentiment for affecting China’s eco-innovation. Furthermore, in order to test whether environmental regulation has spatial spillover effect, by constructing the Spatial Durbin model, this study finds that the environmental regulation has spatial spillover effects on different regions’ eco-innovation in China through two kinds of sentiment. To the policy implication, this study from two kinds of sentiment to guide the environmental regulators would effectively improve the level of eco-innovation in China.
Introduction
Most parts of China are facing resource overdraft and declining environmental quality, and the tension between economic development and environmental protection is becoming increasingly acute. The theme of environmental protection has received increasing attention in China’s policymakers since 2003 (Song et al., 2020). In this condition, China’s government has implemented various environmental regulations for the environmentally friendly.
Regarding environmental regulation, we cannot get around the discussion between environmental regulation and eco-innovation (Kesidou and Wu, 2020). Eco-innovation as one field of social innovation has played a sustainable role on human development, which as core competitiveness has been more concerned by the public than before (Sun et al., 2021). The Chinese government has issued the Science and Technology Development of National Environmental Protection, which clearly states that the construction of ecological civilization in China urgently needs to rely on eco-innovation to break through the bottleneck of resources and environment. Hojnik et al. (2018) state that the eco-innovations are unique and require a good understanding of the role of public policy and firm management. Particularly, motivation-based factors (regulatory pressure, expected benefits of implementation, and competitive pressure) or input factors (financial resources, technological capabilities) act as a driver to stimulate the eco-innovation development.
Previous studies in China focused on innovation of enterprises and corporate performance under environmental regulations (Peng et al., 2018; Peng et al., 2019; Peng et al., 2020; Zhao et al., 2021) Some studies emphasized agriculture from the perspective of the environment, climate change, and adoption of innovative management techniques. The previous literature mainly debated on the “reversed transmission effects” and “cost theory” to the firm investors or managers. To the “reversed transmission effects,” the government strengthens the environmental regulation that makes the enterprises realize that eco-innovation is the only choice for them to get away from the backward and heavily polluted extensive industrial production model and promote the upgrading of industrial structure. In addition, the “cost theory” refers to environmental regulations stimulating the cost of enterprises and taking up part of R & D investment, while the profits or subsidies of eco-innovation cannot make up for the cost in time. Therefore, under the pressure of declining revenue, it is difficult for enterprises to survive, and they might even go bankrupt. For the public, with the government’s vigorous publicity of environmental protection, they tend to actively invest in environmental regulation projects to promote eco-innovation based on health factors, wealth appreciation, or improving the quality of life. From this point of view, eco-innovation can be treated as an investment that has been motivated by environmental regulations.
Sentiment refers to a psychological state produced by people engaged in some activities or affected by some factors (Lu et al., 2021), including positive psychological states, such as excited and happy; it also includes negative psychological states, such as panic and depression (He, 2022). This study divides sentiment into investor sentiment and public sentiment (Chen et al., 2020). Different from the existing studies, this study concentrates on the two kinds of sentiment as a driving factor for exploring the effects of environmental regulation on eco-innovation in China. There are large number of studies certifying the produced sentiment in different ways, such as market trends, macroeconomic policies, and market environment friendliness (Murty and Kumar, 2003). Accordingly, environmental regulations have changed the environment of private and public sectors in China, which may produce different statuses of sentiment. Within environmental regulation, the investors and public form different judgments of investment value or expected return of eco-innovation through sentiment.
What is essential about our research is the possibility that improving environmental quality for accelerating eco-innovation can be regarded as the response of enterprises to the considerable negative investor sentiment on survival challenge, while the public has positive sentiment on improving quality, which has not yet been covered by the existing literature. Specifically, the contribution of this study consists of using different dimensions of environmental regulation for driving the sentiment to examine the effects of environmental regulation on eco-innovation through sentiment in China. Our principal hypothesis is that fear or uncertainty of environmental regulation in China will be negatively associated with investor sentiment, whereas environmental regulation will positively affect the general public on eco-innovation. This hypothesis builds on the argument that while strict environmental regulation in China spreads fear and generates investor negative sentiment, households and individuals tend to increase the investing in the environmental regulation’s projects aimed at improving the eco-innovation in China. Thus, the environmental regulation is through the sentiments to dominate the eco-innovation development in China.
The main contribution of this study is to explore the balance between two contrasting sentiments of strict environmental regulation. On the one side, the strict environmental regulation in China spread fear among the managers, investors, and institutions, which triggers a fear-induced sentiment on eco-innovation. On the other hand, the environmental regulation in China via media publicity could induce a positive public sentiment on eco-innovation. According to two competing (negative and positive) effects manifest in a unique framework that alleviates the possibility of spurious correlations. This study addresses the following questions that have not been explored in the environmental regulation and eco-innovation. First, does investor fear or threat, which is provoked by the different dimensions of environmental regulation, tend to weaken the incentives for eco-innovation in China? Second, does strict environmental regulation induce a positive public sentiment on China’s eco-innovation? Third, does the strict environmental regulation have the threshold effects on China’s eco-innovation through sentiment? Fourth, does environmental regulation of one region have significant spatial spillover effects on eco-innovation of neighboring provinces through sentiment?
To address these issues, this study uses the entropy weight method to respectively construct the five dimensions of environmental regulation and eco-innovation from 30 provinces in China from 2003 to 2017 as samples. The five dimensions of environmental regulation can be divided into two categories, including regulatory tools and market-oriented tools. In addition, this study uses the text analysis technique and machine learning method to identify investor sentiment and public sentiment. Our empirical strategy draws on two commonly used regression-based and spatial regression methodologies to discuss the environmental regulation how to through sentiment effect on the development of eco-innovation in China. We identify that the environmental regulation has positive and significant effects on the eco-innovation. Moreover, environmental regulation has a positive effect on China’s eco-innovation through public sentiment. Environmental regulation has a negative effect on China’s eco-innovation through investor sentiment. Using the multi threshold model, we find that the environmental regulation has threshold effects on China’s eco-innovation through investor sentiment and public sentiment. Last, this study constructs the Spatial Durbin Model to identify the spillover effects of environmental regulation on China’s eco-innovation through investor sentiment and public sentiment.
Review of Literature and Construction of Hypotheses
The existing literature has discussed extensively that the relationship between the environmental regulation with eco-innovation is mainly centered on Porter hypothesis. For example, Ramanathan et al. (2017) state that the eco-innovation in environmental regulation should rely on the market mechanism rather than the administrative order. Wang et al. (2019) argue that the Porter hypothesis is valid to a certain extent. In detail, under a certain degree of strictness, environmental regulation has a positive effect on green productivity growth; that is, the Porter hypothesis is valid. However, the sentiment as the driven factor to analyze the environmental regulation and eco-innovation in China has not been investigated, with the exception of the study. Use a text analysis technique to investigate the relationships among environmental news, investor sentiment, and green industry stock returns in China. Interestingly, there was a significant negative relationship between media environmental attention and the stock returns of green industry companies, which indicates that the Porter hypothesis is not valid in China. Environmental news had significant impact on investor sentiment, which in turn played a mediating role in the process of affecting green industry stocks.
In this section, we review the existing literature on environmental regulation, eco-innovation, and sentiment. Then, based on the existing literature, there are seven testable hypotheses developed for exploring the research gaps.
Environmental Regulation, Sentiment, and Eco-innovation
Changes in policies and regulations will change the sentiments of investors and the public and affect their own investment decisions. Find that there exist asymmetric effects of policy or regulation on investor sentiment, in which supportive government policies lead to increase in investor sentiment at the enterprise and industry levels, while restrictive government policies lead to decrease in investor sentiment. According to the emotional motivation theory, Sun et al. (2021) argue that the regulatory policy formulated by the government makes the public mood go through three periods.
Based on the Porter hypothesis, strict environmental regulation can force the enterprise to increase investment and carry out eco-innovation. With issuing the environmental regulation, the sentiment of the public and investors will be produced to invest in the eco-innovation projects actively or passively. Specifically, Zhao and Sun (2016) state that effective environmental regulation can trigger positive public sentiment on eco-innovation. Argue that investor sentiment has heterogeneity effects on the different kinds of enterprise innovation. Wu et al. (2021) state that the economic policy uncertainty (EPU) exerts negative effects on enterprise innovation through investor sentiment. Argue that the investor sentiment has moderating role on the environmental news and green industry stocks. Based on relevant studies, the regulation has triggered different sentiments of investors and public on the innovation.
The government’s strict environmental regulation may cause “panic” among investors and inhibit eco-innovation. For the public, their investment choices may follow the direction of government regulation, resulting in positive emotions, and promote eco-innovation. Therefore, this study proposes that environmental regulation has a positive/negative effect on China’s eco-innovation through public sentiment/investor sentiment.
H1. Environmental regulation has a positive effect on China’s eco-innovation through public sentiment.
H2. Environmental regulation has a negative effect on China’s eco-innovation through investor sentiment.
At present, China’s environmental regulation mainly adopts the regulatory and market-oriented tools. To the regulatory tool, it formulates the legal standards for environmental and ecological performance, which emphasizes that the government can achieve environmental objectives by implementing legal obligations or prohibitions on market subjects; that is, imposed economic or administrative penalties on those polluters who fail to fulfill and achieve environmental requirements (Lu et al., 2021), such as the government directly imposes administrative penalties on those who violate relevant regulations. In addition, due to the arduous task of environmental regulation, the government has invested a lot of human, material, and financial resources. Regulatory tools are an important measure to deal with the “market failure” of environmental problems and an important factor driving enterprise eco-innovation activities. In addition, the market-oriented tools mainly reduce the emission of harmful substances to the environment by affecting product prices or production costs so as to achieve environmental objectives. When the cost is certain, the government uses e-market–oriented tools to carry out environmental regulation. The greater the intensity of environmental regulation, the more funds to invest in the governance of environmental pollution, thus affecting the investment in eco-innovation. Therefore, this study proposes a positive relation between China’s regulatory tools and market-oriented tools with eco-innovation.
Considering the regulatory and market-oriented tools, these different nature tools may trigger different sentiments. The regulatory tools via strict administrative methods not only attract public attention on the government’s environmental protection but also change investor judgments about pollution, resources, and technology, which affect the eco-innovation in China. Overall, this study proposed that the regulatory tools of environmental regulation have triggered positive/negative sentiments of the public/investors on China’s eco-innovation.
H3a. The intensity of environmental pollution control inhibits China’s eco-innovation through negative investor sentiment.
H3b. The intensity of environmental pollution control promotes China’s eco-innovation through positive public sentiment.
H3c. The intensity of environmental punishment inhibits China’s eco-innovation through negative investor sentiment.
H3d. The intensity of environmental punishment promotes China’s eco-innovation through positive public sentiment.
H3e. The input of the environmental protection personnel system inhibits China’s eco-innovation through negative investor sentiment.
H3f. The input of environmental protection personnel promotes China’s eco-innovation through positive public sentiment.
The market-oriented tools would provide strong incentives for enterprises to apply new environmental technologies, especially when environmental policies are more flexible and enterprises can freely choose the best way to achieve environmental objectives with lower cost and higher resource utilization efficiency (Gollop and Roberts, 1983; Wang et al., 2019; He et al., 2021). Considering the relevant literature, the market-oriented tools may produce different sentiments on the public and investors to understand the relationship between environmental regulation with eco-innovation. Therefore, this study proposes that market-oriented tools of environmental regulation promote China’s eco-innovation through public sentiment and investor sentiment.
H4a. The environmental protection tax promotes China’s eco-innovation through investor sentiment.
H4b. The environmental protection tax promotes China’s eco-innovation through public sentiment.
H4c. The environmental subsidies promote China’s eco-innovation through investor sentiment.
H4d. The environmental subsidies promote China’s eco-innovation through public sentiment.
Threshold Effects of Environmental Regulation
Considering the increasingly prominent environmental problems, the environmental regulation as a long-term policy has become an important driving force to promote the development, application, and dissemination of eco-innovation. Various types of policy tools are widely used, and their application areas are also expanding. In this process, there are no relevant studies considering how the different kinds of sentiments are triggered and changed by the long-term environmental regulations. The relevant policies changed, and specific events produced can directly affect the sentiment of the public and investors.
(Lu et al., 2021; Sun et al., 2021). Therefore, this study proposed that the environmental regulation has threshold effects on public sentiment and investor sentiment for affecting China’s eco-innovation development.
H5. Environmental regulation has threshold effects on public sentiment and investor sentiment for affecting China’s eco-innovation.
Moreover, compared with the public, the investors are more constrained. First, when facing the new environmental regulation, investors are limited in financial constraints and resource in the financial market. Second, it is difficult for investors to effectively capture the effects of new regulation on the eco-innovation investment in a timely manner. Third, most of investors in new issued regulation have to face more risk and uncertainty. Under strict environmental regulation, the risk of investors as the main participants is significantly greater than that of the general public. Based on former analysis, this study proposes that the environmental regulation easily affects China’s eco-innovation through the public sentiment, rather than investor sentiment.
H6. Compared with investor sentiment, environmental regulation easily triggers public sentiment for affecting China’s eco-innovation.
Spatial Spillover Effects of Environmental Regulation
In addition, there are great differences in the environmental-friendly nature of various regions in China. Compared with the eastern regions where the economy is in rapid development, the central and western regions are more environmental-friendly.
Existing literature states the different statuses of environmentally friendly zones affecting the development of eco-innovation. Zhao and Sun (2016) state that the environmental regulation in eastern and central regions is significant according to Porter hypothesis, whereas in the western regions, it is insignificant. Find that the increasing investment in educational resources and optimizing industrial structure have affected the heterogeneity of environmental regulation on China’s green innovation efficiency, in which eastern parts are stronger than central and western parts. Du et al. (2021) find the heterogenous effects of environmental regulation on the green transformation of different types of Chinese cities. Based on the regional differences of environmental regulation on eco-innovation through the sentiment, the related studies argue that the environmental regulation has significant strategic interaction between different regions, for example, Wu et al. (2021) state that that the strategic interaction of urban environmental regulations in China’s central and western regions is significant. Zhou et al. (2021) find that the environmental regulation has significant spatial spillover effects on urban innovation. He et al. (2021) believe that market-oriented tools have significant spatial spillover effects on the port sustainable development, rather than the regulatory tools. Lu et al. (2021) find that there are differences in the spatial spillover effects of environmental regulation, which has the most significant effects on western regions. These studies emphasize the spatial spillover effects of environmental regulation, while they do not explore the transmission mechanism of the spatial spillover effect of environmental regulation. Moreover, investor sentiment positively influences stock return volatility, which exhibits both a temporal cumulative and spatial spillover effect (Jiang and Jin, 2020; Sun et al., 2021). The relevant literatures have certified the heterogenous effects of environmental regulation on China’s eco-innovation, depending on the regional economic level, education level, and being environmentally friendly. In addition, the quality of being environmentally friendly in different regions affects the severity of environmental regulation. These differences may trigger different sentiments of environmental regulation on eco-innovation. Therefore, this study proposes that the spatial spillover effects of environmental regulation are through public sentiment and investor sentiment on China’s eco-innovation.
H7a. Compared with the central and western regions, the direct effects of environmental regulation on eco-innovation in the eastern region through the investor sentiment are more significant than the indirect effects.
H7b. Compared with the central and western regions, the direct effects of environmental regulation on eco-innovation in the eastern region through the public sentiment are more significant than the indirect effects.
H7c. Compared with the eastern and western regions, the indirect effects of environmental regulation on eco-innovation in the central region through the investor sentiment are more significant than the direct effects.
H7d. Compared with the eastern and western regions, the indirect effects of environmental regulation on eco-innovation in the central region through the public sentiment are more significant than the direct effects.
Econometric Models, Variables, and Data
Econometric Models
To examine the Hypothesis1–Hypothesis4, the equations are shown in Supplementary Appendix B. From the previous literatures, it can be seen that the relationship between environmental regulation with eco-innovation is nonlinear. According to Hansen (1999), this study constructs the panel data multi-threshold models of environmental regulation on public and investor sentiments to investigate whether the correlation between the two kinds of sentiments and level of eco-innovation changes with the threshold variable of environmental regulation. Based on this condition, Eqs 13–22 examine Hypothesis 5 and 6 as following:
where
Based on the different environmental regulations in different regions, there may exist spatial spillover effects of one region’s environmental regulation on local eco-innovation and the other region’s eco-innovation through two kinds of sentiments. For testing Hypothesis 7, the models are as following:
where
Variables and Data Collection for the Study
All regions of China are faced with resource overdraft and decline in environmental quality. Since 2003, Chinese policymakers have paid more and more attention to the theme of environmental protection (Wang et al., 2015; Song et al., 2020). Under such circumstances, the Chinese government has implemented various environmental protection regulations to protect the environment. Therefore, this study takes China as the research sample and selects the data from 2003 to 2017.
Explained Variable
The explained variable of this study is eco-innovation. It is challenging to use a single index to measure eco-innovation. Reviewing previous studies, the target of eco-innovation is to promote sustainable development through production, service, and development of new products to reduce the adverse effects on the environment or improve resource utilization efficiency. It can be understood that eco-innovation includes four factors, which are technology innovation (Li et al., 2021), environmental protection (Ball et al., 2018), resource consumption (Ding et al., 2021), and economic performance (Hojnik et al., 2018). Therefore, these four factors, in Table 1’s first column, represent the level of eco-innovation. These four factors are, respectively, extracted from related variables through principal component analysis (PCA). Considering the study by Du et al. (2021), this study uses the entropy weight method to construct one proxy variable for China’s eco-innovation. The basic idea of the entropy weight method is that the more useful information a specific index provides, the higher its weight (Yang et al., 2021). From Table 1, we find that the weights of four factors are relatively balanced to reflect the level of eco-innovation in China. The calculation process of the entropy weight method is shown in Supplementary Appendix.
Explanatory Variables
The key explanatory variables in our study are environmental regulation (ER) and sentiments.
a) Environmental Regulation (ER)
According to Du et al. (2021), this study uses the entropy weight method to synthesize five kinds of environmental tools for the level of environmental regulation in China. These five factors, in Table 2’s first column, reflect the government’s environmental governance two sides: regulatory tools and market-oriented tools, which are most related to ER. These five factors are, respectively, extracted from related variables through principal component analysis (PCA). From Table 2, we can find that the weights of these five factors of environmental regulations are relatively balanced.
b) Public sentiment and Investor sentiment
To measure the sentiments, the common methods include market indicators and direct indicators. Reviewing the relevant literature, both these methods contain defects (Fu et al., 2020). Within the online We-media development in China, sentiment can be derived from online comments with the text analysis technique and machine learning method. The existing literature argues that mechanism learning methods can be applied through the large enough training set and good feature set to achieve high classification accuracy (Cai et al., 2020). Based on this condition, this study treats the general public of investor online comments around environmental regulation news as the multi-rounds of dialogue. Hence, this study uses the online text dialogue to identify public sentiment and investor sentiment.
The sentiment recognition method of a text dialogue mainly includes the following steps:
First, dialogue D usually involves two kinds of people. In this study, we assume A and B as the interlocutors. A is the initiator of the dialogue, which refers to A issuing the related environmental regulation on the Internet, and B stands for the responders of the dialogue, which refers to the investors or general public in the Internet. B gave M rounds of comments to A’s dialogue. Second, each round of dialogue D consists of M rounds, where
where
In a short conversation, the state of sentiment is continuous and changing, while it cannot be forgotten soon. The change of sentiment is via the expression of sentiments, whereas the previous state of sentiment is still stored in the memory cells, which cannot be completely forgotten. Based on this condition, this study uses the LSTM + Attention of a single sentence to analyze the sentiments of the current sentence and identifies all the comment text data sets in the sample. In the recognition task, the calculation formula of interactive two-state sentiment cells is as follows:
If
If
where
Considering the differences between the general public and investors, this study selects different kinds of website comment texts to identify the two sentiments. Specifically, the public sentiment is identified by the comments on environmental regulation in the non-financial sector of Baidu, Sina, Weibo, and TouTiao. To investor sentiment, it is from the comments of Sina Finance, NetEase Finance, Global Finance, and China Securities Network. These websites have the largest number of daily active users in China, as shown in Table 3.
According to the text dialogue sentiment recognition method, this study treats the issued news about environmental regulation on different platforms as A, one comment from the general public or investors to the issued news of environmental regulation is regarded as a completed dialogue, which means that all comments from B, respectively, correspond to the environmental regulation news released by A. If emoticons appear in comments, this study converts them into text. Moreover, in order to avoid overlapping data, duplicated comments are eliminated. Based on this condition, this study collected 200,000 online valid comments from 1 January 2003 to 31 December 2017 and divided the regions according to the user’s registration places. Through the comment statements presented by different samples, we can accurately identify the sentiments of the general public and investors for environmental regulation. Therefore, we observe that the time series of public sentiment and investor sentiment are shown in Figure 1. As shown in Figure 2, the public sentiment and investor sentiment are stable from 2003 to 2017. Compared with the general public sentiment, investor sentiment experienced a U-shaped trend.
Control Variables
In this study, the level of urbanization, industrialization, foreign direct investment, and the industrial structure supererogation are selected as control variables.
The reasons for these control variables are as follows. Ahmad et al. (2021) state that the environmental regulation has an indirect beneficial impact on ecological innovation through the channel of urbanization. Therefore, the level of urbanization is chosen. With the development of China’s industrialization, we still need to pay attention to environmental pollution. Promoting industrial intelligence not only reduces the environmental pollution but also provides a new channel for eco-innovation (Ibrahim and Vo, 2021). Therefore, we select the level of industrialization. Moreover, foreign direct investment provides sufficient capital for eco-innovation and contributes to energy use and carbon dioxide emission. It is an important channel for the renewable energy industry to realize eco-innovation (Khan et al., 2021). Therefore, foreign direct investment is chosen. Last, ecological innovation and industrial structure supererogation play a two-way promoting effect. On the one hand, the industrial structure supererogation makes the elements flow to technology-intensive industries, which promotes the level of eco-innovation. On the other hand, with the improvement of the level of eco-innovation, labor-intensive industries and capital-intensive industries gradually improve their technical level, which can transform to technology-intensive industries and improve the level of industrial structure supererogation. So, industrial structure supererogation is chosen.
In detail, the ratio of the resident population of the region to the total population of China is used to represent the level of urbanization. The level of industrialization is measured by the ratio of the industrial added value of the region to the total GDP in China. We show the level of foreign direct investment by the amount of foreign direct investment. In addition, the ratio of the total output value of the tertiary industry to the total output value of the secondary industry is used to measure the level of advanced industrial supererogation. Relevant data are from Wind Database, CEIC, and China provinces or cities Statistical-Yearbooks. Overall, the descriptive statistics of all variables are presented in Table 4.
Results and Discussion
Environmental Regulation, Sentiments, and Eco-innovation
This study aims to shed light on the role of two kinds of sentiments on the environmental regulation with China’s eco-innovation. From Table 5, we could find that the environmental regulation has positive and significant effects on the eco-innovation, which confirms the Porter Hypothesis in China. In Eq. 1, the coefficient of the interaction term is positive and significant, which indicates that the environmental regulation has a positive effect on China’s eco-innovation through public sentiment. In Eq. 2, the coefficient of the interaction term is negative and significant, which indicates that the environmental regulation inhibits China’s eco-innovation through investor sentiment. Therefore, Hypotheses 1 and 2 are confirmed.
These results are a line with the studies by Li et al. (2020). Li et al. (2020) state that the general public is more likely to believe and accept the related policies or regulation issued by government than the investors. In addition, argue that investor negative sentiment has negative effects on the innovation. On another side, analyzes the reactions of investor sentiment to environmental regulation news and argues that environmental news makes investors change their strategies in time, which promotes the development of innovation. However, the environmental regulations are long-term policies, which should not seek the investor response immediately. After all, whether the information asymmetry or policy uncertainty, investors should consider the ratio of risk-return to make the decision. With the continuous superposition of relevant policies, environmental regulation will become more and more strict than before. Ford et al. (2014) state that strict environmental regulation has a negative impact on investors, which inhibits the eco-innovation. Overall, environmental regulation not only improves the public’s enthusiasm and awareness of environmental protection and eco-innovation but also brings the pressure of continuous innovation and progress to enterprises.
Moreover, Table 6 shows the effects of five environmental regulation tools on China’s eco-innovation through two kinds of sentiment. From Panel A and B, we could find that the all of regulatory tools of environmental regulation, which include the intensity of environmental pollution control, intensity of environmental punishment, and input of environmental protection personnel, have different effects on China’s eco-innovation via two kinds of sentiment. In detail, these regulatory tools inhibit China’s eco-innovation through the negative investor sentiment, whereas China’s eco-innovation is promoted by positive public sentiment, which is confirmed by H3a, H3b, H3c, H3d, H3e, and H3f. Moreover, the environmental protection tax has negative and significant effects on China’s eco-innovation through the investor sentiment, whereas the environmental subsidies promote China’s eco-innovation through the investor sentiment, which is confirmed by H4c. Both of the market-oriented tools of environmental regulation have insignificant effects on China’s eco-innovation through public sentiment.
Considering the different effects, the reasons are as follows. 1) As the direct regulation to enterprises, the regulatory tools easily trigger negative investor sentiment, which indicates that the investor as the main body has to consider the increasing survival costs and policy uncertainty (Rehman et al., 2021). Therefore, the regulatory tools of environmental regulation hinder China’s eco-innovation through the negative investor sentiment. 2) Different with the investors, the general public generally supports the environmental regulation, especially the regulatory tools. As non-investors, the general public recognizes that the government’s determination to environmental governance often comes from the administrative orders (Guo et al., 2021). The strict regulatory tools make the general public have expectations for environmentally sustainable development, which trigger positive public sentiment. Therefore, the regulatory tools of environmental regulation promote the development of China’s eco-innovation through the public investor sentiment.
Threshold Effects of Environmental Regulation
From Table 6, we could find that the coefficients of the square term of environmental regulation show that environmental regulation has a nonlinear impact on China’s eco-innovation. Therefore, this study uses the multi-threshold models to investigate whether the general public sentiment and investor sentiment change with the intensity of environmental regulation. In order to determine the multi-threshold model, this study sets the single threshold, two thresholds, and three thresholds as the hypothesis. According to the bootstrap method, the F-value and P-value of the threshold effect test are calculated 500 times. From Panel A and B in Table 7, the results show that environmental regulation has threshold effects on general public sentiment and investor sentiment for affecting China’s eco-innovation, which is confirmed by Hypothesis five. Ultimately, the sentiment is triggered or produced by the policy implementation intensity, in which different kinds of tools of environmental regulations have threshold effects on public sentiment and investor sentiment.
Table 8 respectively shows that different thresholds of environmental regulation are how to affect China’s eco-innovation through two kinds of sentiment. In Panel A, these regulatory tools in first or second threshold have insignificant or negative effects on China’s eco-innovation through investor sentiment, whereas all of the last thresholds of regulatory tools have positive and significant effects on China’s eco-innovation. We could observe that there exists a U-shaped trend about the regulatory tools in different thresholds affecting China’s eco-innovation through the investor sentiment. To the market-oriented tools, we could find that their effects in the first threshold are insignificant. In addition, the effects of market-oriented tools in the second threshold on China’s eco-innovation via investor sentiment are strongest positive effects in three thresholds. There exists an inverted V trend about the market-oriented tools in different thresholds affecting China’s eco-innovation through investor sentiment. In Panel B, different kinds of environmental regulation in the first threshold have strongest effects on China’s eco-innovation through public sentiment. In addition, the subsequent threshold of environmental regulation has shown a decreasing effect on China’s eco-innovation.
Compared with the results in Panel A and B, we could find that the environmental regulation is easily triggers public sentiment for affecting China’s eco-innovation, which is confirmed by Hypothesis 6. These results prove that the LSTM model identification of the two sentiments in Figure 1 is correct, which indicates that the environmental regulations issued by the government satisfy the expectations of the general public for environmental protection and eco-innovation. In addition, investors need to consider restrictions or development factors brought by environmental regulation before making investment decisions about the eco-innovation.
Spatial Spillover Effects of Environmental Regulation
In this section, we use the Spatial Durbin Model to explore Hypothesis 7. Compared with the central and western regions, Table 9 shows that the direct effect of environmental regulation in the eastern region on eco-innovation through investor sentiment and public sentiment is stronger than the indirect effect, which is confirmed by H7a and H7b. Our results are consistent with those of Li et al. (2021). Li et al. (2021) find that the performance of environmental regulation of the eastern region is better than that of other regions. The eastern region has the highest level of industrial development, the fiercest market competition, and the best level of education and quality of life of residents in China. On the one side, investors want to have the regional advantages of the eastern region in China; they would carry out eco-innovation to meet the requirements of environmental regulation, even if the investor sentiment is negative. On the other side, with higher education level and living standards, the general public tends to have a positive attitude toward being environmentally friendly and eco-innovation (Song et al., 2020). Based on this point, environmental regulation in the eastern region is easier for the public to have expectations of the government’s environmental protection measures than the central and western regions, which promotes local eco-innovation.
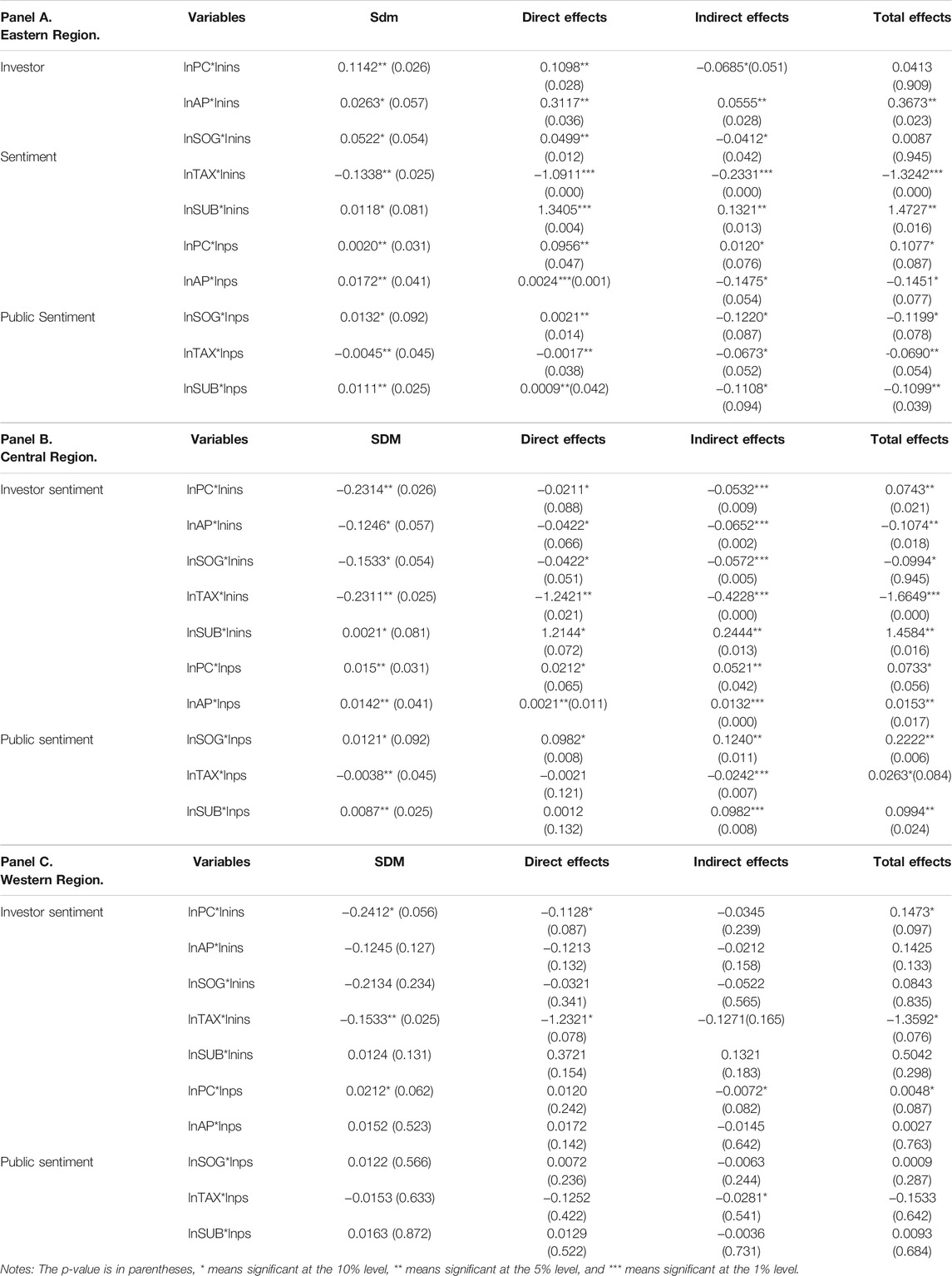
TABLE 9. Spatial spillover effects of environmental regulation on sentiment in different regions of China.
Moreover, compared with the eastern and western regions, the indirect effect of environmental regulation in central regions on eco-innovation through investor sentiment and public sentiment is more significant than the direct effect, which is confirmed by H7c and H7d are. These results have built on that the degree of environmental regulation in the central region is weaker than that of the eastern region. The effects of geographical proximity and economic proximity on the two kinds of sentiment can enhance the eco-innovation in the central region. To the geographical proximity, some enterprises in the eastern region may move to the central region with slightly relaxed environmental control, which promote the eco-innovation in the central region while reducing costs. To the economic proximity, some enterprises in the western region may develop ecological innovation in the central region with high economic development level and not strict environmental control.
Robustness Analysis
To examine the robustness of the empirical results, this study adds one-period lag of eco-innovation to construct the GMM model, as follows:
Based on Table 10, when adding the one-period lag of the explained variable, the coefficients of the key explanatory variables are consistent with the empirical results. The significance of AR (2) is expressed as accepting the original hypothesis, that is, there is no second-order autocorrelation. The significance level of the Hansen test is 0.789, 0.712, 0.499, and 0.950, which indicates that all instrumental variables are valid. Therefore, we claim that our empirical results are robust.
Conclusion
Different with previous studies, this study aims to shed light on the effects of environmental regulation on China’s eco-innovation through investor sentiment and public sentiment. This study uses the text analysis technique and machine learning method to identify investor sentiment and public sentiment, respectively, from 2003 to 2017.
The main conclusions are as follows. First, the environmental regulation has a positive effect on China’s eco-innovation through public sentiment, whereas the environmental regulation inhibits China’s eco-innovation through investor sentiment. Second, these regulatory tools inhibit China’s eco-innovation through the negative investor sentiment, whereas promoting China’s eco-innovation by positive public sentiment. Based on these results, environmental regulation not only improves the public’s enthusiasm and awareness of environmental protection and eco-innovation but also brings the pressure of continuous innovation and progress to enterprises. Third, the environmental regulation has threshold effects on general public sentiment and investor sentiment for affecting China’s eco-innovation. In detail, compared with investor sentiment, the environmental regulation easily triggers public sentiment for affecting China’s eco-innovation. Last, by constructing the SDM model, this study finds that the environmental regulation has spatial spillover effects on different regions’ eco-innovation in China through two kinds of sentiment. Among them, the direct effect of environmental regulation on ecological innovation through investor sentiment and public sentiment is stronger than the indirect effect. However, the indirect effect of environmental regulation on ecological innovation through investor sentiment and public sentiment is stronger than the direct effect. From this sight, economic level and education level in the different regions can affect the general public and investor attitudes and judgements on environmental regulation.
Following the empirical results of this study, the relevant recommendations are summarized as follows. First, to develop the eco-innovation in China, the differences between different environmental regulation tools should be deeply considered. To the regulatory tools, these easily trigger the positive public sentiment to promote China’s eco-innovation, whereas produce the negative investor sentiment to inhibit China’s eco-innovation. Instead, appropriate supporting policies or moderated tools should be adopted to trigger the positive investor sentiment. For example, the government can build a big data system to detect the green operation status and life cycle of enterprises, which can supervise the specific pollution discharge of enterprises to varying degrees through data tracking. Second, the government should pay attention to guide the public and investor sentiment to the environmental regulation. Based on the results, the public sentiment is easier to trigger, whereas the investor sentiment is difficult to trigger. Therefore, the government should play to the advantages of the public, which promulgate to increase social investment in the eco-innovation projects to wake up investor sentiment. Last, considering the spatial spillover effects of environmental regulation, the government should encourage strengthening cooperation with the western region, which promotes eco-innovation. In this article, a major limitation is that a more appropriate method is not used to deal with the nonlinear effects of environmental regulations, which may make the actual results inaccurate. Therefore, nonlinear models may be used for comparative research in the future to verify the effectiveness of the analysis results. Future research should explore these interrelationships as they may provide key directions for developing environmental regulations in China. In addition, the current review does not attempt to use nonlinear models. In the future, the nonlinear analysis of these methods may help determine the focus of environmental regulation.
Data Availability Statement
The original contributions presented in the study are included in the article/Supplementary Material, further inquiries can be directed to the corresponding authors.
Ethics Statement
Ethical review and approval was not required for the study on human participants in accordance with the local legislation and institutional requirements. Written informed consent for participation was not required for this study in accordance with the national legislation and the institutional requirements.
Author Contributions
YS: conceptualizing, writing, and drafting—original draft QB: data collection and empirical estimations HL: data and methodology ZL: review and editing.
Conflict of Interest
The authors declare that the research was conducted in the absence of any commercial or financial relationships that could be construed as a potential conflict of interest.
Publisher’s Note
All claims expressed in this article are solely those of the authors and do not necessarily represent those of their affiliated organizations, or those of the publisher, the editors, and the reviewers. Any product that may be evaluated in this article, or claim that may be made by its manufacturer, is not guaranteed or endorsed by the publisher.
Supplementary Material
The Supplementary Material for this article can be found online at: https://www.frontiersin.org/articles/10.3389/fenvs.2021.809452/full#supplementary-material
References
Ahmad, M., Jiang, P., Murshed, M., Shehzad, K., Akram, R., Cui, L., et al. (2021). Modelling the Dynamic Linkages between Eco-Innovation, Urbanization, Economic Growth and Ecological Footprints for G7 Countries: Does Financial Globalization Matter? Sustain. Cities Soc. 70, 102881. doi:10.1016/j.scs.2021.102881
Ball, C., Burt, G., De Vries, F., and MacEachern, E. (2018). How Environmental protection Agencies Can Promote Eco-Innovation: The prospect of Voluntaryreciprocal Legitimacy. Technol. Forecast. Soc. Change 129, 242. doi:10.1016/j.techfore.2017.11.004
Cai, Y., Huang, Q., Lin, Z., Xu, J., Chen, Z., and Li, Q. (2020). Recurrent Neural Network with Pooling Operation and Attention Mechanism for Sentiment Analysis: A Multi-Task Learning Approach. Knowledge-Based Syst. 203, 105856. doi:10.1016/j.knosys.2020.105856
Chen, Y., Goyal, A., Veeraraghavan, M., and Zolotoy, L. (2020). Terrorist Attacks, Investor Sentiment, and the Pricing of Initial Public Offerings. J. Corporate Finance 65, 101780. doi:10.1016/j.jcorpfin.2020.101780
Ding, Q., Khattak, S. I., and Ahmad, M. (2021). Towards Sustainable Production and Consumption: Assessing the Impact of Energy Productivity and Eco-Innovation on Consumption-Based Carbon Dioxide Emissions (CCO2) in G-7 Nations. Sustain. Prod. Consum. 27, 254. doi:10.1016/j.spc.2020.11.004
Du, K., Cheng, Y., and Yao, X. (2021). Environmental Regulation, green Technology Innovation, and Industrial Structure Upgrading: The Road to the green Transformation of Chinese Cities. Energ. Econ. 98, 105247. doi:10.1016/j.eneco.2021.105247
Ford, J. A., Steen, J., and Verreynne, M. L. (2014). How Environmental Regulations Affect Innovation in the Australian Oil and Gas Industry: Going beyond the Porter Hypothesis. J. Clean. Prod. 84, 204. doi:10.1016/j.jclepro.2013.12.062
Fu, J., Zhou, Q., Liu, Y., and Wu, X. (2020). Predicting Stock Market Crises Using Daily Stock Market Valuation and Investor Sentiment Indicators. North Am. J. Econ. Finance 51, 100905. doi:10.1016/j.najef.2019.01.002
Gollop, F. M., and Roberts, M. J. (1983). Environmental Regulations and Productivity Growth: The Case of Fossil-Fueled Electric Power Generation. J. Polit. Economy 91 (4), 654–674. doi:10.1086/261170
Guo, T., Campbell-Arvai, V., and Cardinale, B. J. (2021). Why Does the Public Support or Oppose Agricultural Nutrient Runoff Regulations? The Effects of Political Orientation, Environmental Worldview, and Policy Specific Beliefs. J. Environ. Manage. 279, 111708. doi:10.1016/j.jenvman.2020.111708
Hansen, B. E. (1999). Threshold Effects in Non-dynamic Panels: Estimation, Testing, and Inference. J. Econom. 93, 345. doi:10.1016/s0304-4076(99)00025-1
He, X., Liu, W., Hu, R., and Hu, W. (2021). Environmental Regulations on the Spatial Spillover of the Sustainable Development Capability of Chinese Clustered Ports. J. Mar. Sci. Eng. 9 (3), 301. doi:10.3390/jmse9030301
He, Z. (2022). Asymmetric Impacts of Individual Investor Sentiment on the Time-Varying Risk-Return Relation in Stock Market. Int. Rev. Econ. Finance 78, 177. doi:10.1016/j.iref.2021.11.018
Hojnik, J., Ruzzier, M., and Manolova, T. S. (2018). Internationalization and Economic Performance: The Mediating Role of Eco-Innovation. J. Clean. Prod. 171, 1312. doi:10.1016/j.jclepro.2017.10.111
Ibrahim, M., and Vo, X. V. (2021). Exploring the Relationships Among Innovation, Financial Sector Development and Environmental Pollution in Selected Industrialized Countries. J. Environ. Manage. 284, 112057. doi:10.1016/j.jenvman.2021.112057
Jiang, S., and Jin, X. (2020). Effects of Investor Sentiment on Stock Return Volatility: A Spatio-Temporal Dynamic Panel Model. Econ. Model. 97, 298. doi:10.1016/j.econmod.2020.04.002
Kesidou, E., and Wu, L. (2020). Stringency of Environmental Regulation and Eco-Innovation: Evidence from the Eleventh Five-Year Plan and green Patents. Econ. Lett. 190, 109090. doi:10.1016/j.econlet.2020.109090
Khan, A., Chenggang, Y., Hussain, J., and Kui, Z. (2021). Impact of Technological Innovation, Financial Development and Foreign Direct Investment on Renewable Energy, Non-renewable Energy and the Environment in belt & Road Initiative Countries. Renew. Energ. 171, 479. doi:10.1016/j.renene.2021.02.075
Li, S., Liu, Z., and Li, Y. (2020). Temporal and Spatial Evolution of Online Public Sentiment on Emergencies. Inf. Process. Manag. 57, 102177. doi:10.1016/j.ipm.2019.102177
Li, H., Pang, S., Cao, Y., and Cao, J. (2021). Research on the Evaluation of Comprehensive Efficiency of Technological Innovation and Eco-Environment in China. J. Clean. Prod. 283, 124603. doi:10.1016/j.jclepro.2020.124603
Lu, Z., Wang, Q., and Zhang, M. (2021). Environmental Regulation and CO2 Emissions: Based on Strategic Interaction of Environmental Governance. Ecol. Complex. 45, 100893. doi:10.1016/j.ecocom.2020.100893
Murty, M. N., and Kumar, S. (2003). Win-win Opportunities and Environmental Regulation: Testing of porter Hypothesis for Indian Manufacturing Industries. J. Environ. Manage. 67, 139–144. doi:10.1016/s0301-4797(02)00203-7
Peng, B., Tu, Y., Elahi, E., and Wei, G. (2018). Extended Producer Responsibility and Corporate Performance: Effects of Environmental Regulation and Environmental Strategy. J. Environ. Manage. 218, 181–189. doi:10.1016/j.jenvman.2018.04.068
Peng, B., Wang, Y., Elahi, E., and Wei, G. (2019). Behavioral Game and Simulation Analysis of Extended Producer Responsibility System's Implementation under Environmental Regulations. Environ. Sci. Pollut. Res. Int. 26, 17644–17654. doi:10.1007/s11356-019-05215-w
Peng, B., Zheng, C., Wei, G., and Elahi, E. (2020). The Cultivation Mechanism of green Technology Innovation in Manufacturing Industry: From the Perspective of Ecological Niche. J. Clean. Prod. 252, 119711. doi:10.1016/j.jclepro.2019.119711
Ramanathan, R., He, Q., Black, A., Ghobadian, A., and Gallear, D. (2017). Environmental Regulations, Innovation and Firm Performance: A Revisit of the Porter Hypothesis. J. Clean. Prod. 155, 79. doi:10.1016/j.jclepro.2016.08.116
Rehman, M. U., Sensoy, A., Eraslan, V., Shahzad, S. J. H., and Vo, X. V. (2021). Sensitivity of US Equity Returns to Economic Policy Uncertainty and Investor Sentiments. North Am. J. Econ. Finance 57, 101392. doi:10.1016/j.najef.2021.101392
Song, Y., Yang, T., Li, Z., Zhang, X., and Zhang, M. (2020). Research on the Direct and Indirect Effects of Environmental Regulation on Environmental Pollution: Empirical Evidence from 253 Prefecture-Level Cities in China. J. Clean. Prod. 269, 122425. doi:10.1016/j.jclepro.2020.122425
Sun, Y., Duru, O. A., Razzaq, A., and Dinca, M. S. (2021). The Asymmetric Effect Eco-Innovation and Tourism towards Carbon Neutrality Target in Turkey. J. Environ. Manage. 299, 113653. doi:10.1016/j.jenvman.2021.113653
Wang, Q., Zhao, Z., Shen, N., and Liu, T. (2015). Have Chinese Cities Achieved the Win–Win between Environmental protection and Economic Development? From the Perspective of Environmental Efficiency. Ecol. Indicators 51, 151. doi:10.1016/j.ecolind.2014.07.022
Wang, Y., Sun, X., and Xu, G. (2019). Environmental Regulation and green Productivitygrowth: Empirical Evidence on the Porter Hypothesis from OECD Industrial Sectors. Energy Policy 132. doi:10.1016/j.enpol.2019.06.016
Wu, L., Yang, M., and Wang, C. (2021). Strategic Interaction of Environmental Regulation and its Influencing Mechanism: Evidence of Spatial Effects amongChinese Cities. J. Clean. Prod. 312, 127668. doi:10.1016/j.jclepro.2021.127668
Yang, Y., Yang, X., and Tang, D. (2021). Environmental Regulations, Chinese-style Fiscal Decentralization, and Carbon Emissions: from the Perspective of Moderating Effect. Stochastic Environ. Res. Risk Assess. 35, 1–14. doi:10.1007/s00477-021-01999-x
Zhao, X., and Sun, B. (2016). The Influence of Chinese Environmental Regulation on Corporation Innovation and Competitiveness. J. Clean. Prod. 112, 1528. doi:10.1016/j.jclepro.2015.05.029
Zhao, Y., Peng, B., Elahi, E., and Wan, A. (2021). Does the Extended Producer Responsibility System Promote the green Technological Innovation of Enterprises? an Empirical Study Based on the Difference-In-Differences Model. J. Clean. Prod. 319, 128631. doi:10.1016/j.jclepro.2021.128631
Keywords: environmental regulation, eco-innovation, investor sentiment, public sentiment, sentiment
Citation: Sun Y, Li H, Bao Q and Lu Z (2022) Environmental Regulation, Sentiment, and Eco-Innovation: Evidence From China. Front. Environ. Sci. 9:809452. doi: 10.3389/fenvs.2021.809452
Received: 05 November 2021; Accepted: 17 December 2021;
Published: 09 March 2022.
Edited by:
Faik Bilgili, Erciyes University, TurkeyReviewed by:
Ehsan Elahi, Shandong University of Technology, ChinaSevda Kuşkaya, Erciyes University, Turkey
Copyright © 2022 Sun, Li, Bao and Lu. This is an open-access article distributed under the terms of the Creative Commons Attribution License (CC BY). The use, distribution or reproduction in other forums is permitted, provided the original author(s) and the copyright owner(s) are credited and that the original publication in this journal is cited, in accordance with accepted academic practice. No use, distribution or reproduction is permitted which does not comply with these terms.
*Correspondence: Yunpeng Sun, tjwade3@126.com; Haoning Li, 15822431468@163.com; Qun Bao, baoqun@nankai.edu.cn; Zhou Lu, luzhou59@gmail.com