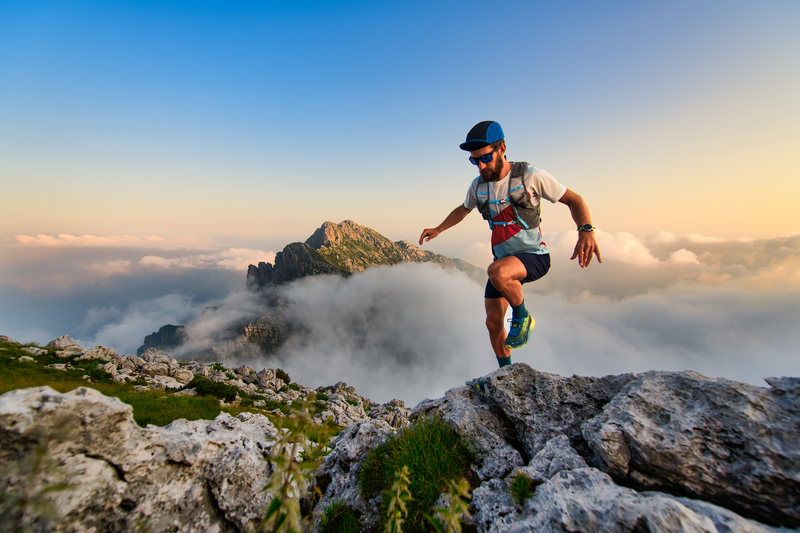
94% of researchers rate our articles as excellent or good
Learn more about the work of our research integrity team to safeguard the quality of each article we publish.
Find out more
ORIGINAL RESEARCH article
Front. Environ. Sci. , 21 December 2021
Sec. Environmental Economics and Management
Volume 9 - 2021 | https://doi.org/10.3389/fenvs.2021.796083
This article is part of the Research Topic Export Product Quality, Renewable Energy, and Sustainable Production View all 48 articles
Although a number of studies have been conducted on the environmental Kuznets curve (EKC) and the pollution halo hypothesis (PHH), few researchers have assessed the scope in the light of the BRICS— Brazil, Russia, India, China, and South Africa—nations. Therefore, the current research assesses the income-induced EKC as well as the role of technological innovation and renewable energy consumption utilizing a dataset stretching from 1990 to 2018. The present research utilized the novel method of moments quantile regression (MMQR) developed by Machado and Silva (2019) to assess these interrelationships. The empirical outcomes from the MMQR affirmed an inverted U-shaped interrelationship between CO2 emissions and economic growth across all quantiles (first to ninth) for the BRICS nations, thus confirming the presence of the EKC hypothesis. Furthermore, we affirmed the PHH, thus confirming the negative interrelationship between globalization and ecological footprint across all quantiles (first to ninth). Moreover, it was found that renewable energy use plays a vital role in curbing the emissions of CO2 across all quantiles (first to ninth), while no evidence of significant connection was established between technological innovation and ecological footprint across all quantiles. In addition, the Granger causality outcomes revealed a feedback causality between income and ecological footprint, while a unidirectional causality was established from globalization and renewable energy use to ecological footprint.
The world is now dealing with various climatic concerns and deteriorating ecological conditions. This is due to the increasing ecological challenges that are arising as a result of changing lifestyles and economic practices (Essien et al., 2019; Vaseashta et al., 2020). According to BP data, global total CO2 emissions from fossil energy reached 33,890.8 million tonnes in 2018, up from 11,190 million tonnes in 1965. In 2018, the average annual CO2 growth rate increased by 2.0%, the highest rate in the previous 7 years (British Petroleum, 2021). Climate change presents a danger to people’s lives and livelihoods. Pollution and extreme weather events, which are frequently observed, cause substantial threats to human beings (Farooq et al., 2019; Vaseashta et al., 2020; Adebayo and Kirikkaleli, 2021; Akhayere and Kavaz, 2021; Fareed et al., 2021). In this regard, it is important to enhance public knowledge about environmental issues; otherwise, the risks and threats connected with climate change, which are expected to increase in the coming years, will have devastating implications for human safety and health.
Technological innovation (TI) plays a critical role in curbing emissions, while also helping in energy conservation. In addition, TI plays a critical role not only in the efficient use of conventional energy sources but also in the utilization of renewables (Shahzad et al., 2020; Wang et al., 2021). Moreover, TI can help in the expansion of renewable energy consumption (REC). TI also improves REC ability, therefore increasing the total supply of REC to fulfill future energy demand. Because of the continually increasing need for energy, it is a well-recognized fact that REC will be the most important source of energy in the future, as well as an ecologically friendly one. The importance of REC in addressing the challenge of energy security while also reducing GHG emissions is well understood. It offers an extra, non-exhausting source of energy that needs to be encouraged for sustainable growth (Alola et al., 2021; Pata, 2021c; Fatima et al., 2021; Kirikkaleli and Adebayo, 2021). Recent increases in REC output appear to be the result of government initiatives, as well as a significant drop in the cost of renewable energy technology as the demand for energy increases. We discovered a wealth of literature on the influence of REC on ecological degradation in the BRICS—Brazil, Russia, India, China, and South Africa—countries. To mitigate the CO2 emissions in these countries, the majority of prior research has recommended that the percentage of REC in the overall energy mix should be increased (Alola, 2019; Banday and Aneja, 2019; Khattak et al., 2020; Pata, 2021a; Awosusi et al., 2021; Doğan et al., 2021; Usman and Makhdum, 2021).
The role of TI in curbing CO2 emissions is well documented in the current literature, although it has not been given significant consideration. There are few studies on the effect of TI on ecological deterioration. For instance, the studies of Khan et al. (2020) and Rjoub et al. (2021) found that TI aids in curbing the emissions of CO2. On the other hand, some studies have found a positive interconnection between TI and deterioration of the environment, such as Ghazouani et al. (2021), Soylu et al. (2021), Su et al. (2021), Udemba et al. (2021), and Paramati et al. (2022) for Chile and Adebayo and Kirikkaleli (2021) for Japan, who established a positive interrelationship between TI and environmental deterioration. In addition, studies such as those by Garrone and Grilli (2010) and Santra (2017) have reported insignificant interrelationships between ecological deterioration and TI.
Globalization refers to the creation of new markets and the interconnectedness of the global economy through trade, finance, and FDI (Adebayo and Acheampong, 2021). Globalization facilitates trade by lowering tariffs and taxes to boost exports and assisting industries in exporting or importing the necessary inputs for product promotion and development (Shahbaz et al., 2018a). Over the years, a significant number of studies have been conducted on the effect of globalization on the environment; nonetheless, the available research presents contradictory conclusions on this topic. For instance Shahbaz et al. (2018a) found that globalization causes an increase in the demand of energy in 12 nations, whereas globalization has a negative impact on the consumption of energy in the United Kingdom and the United States. Likewise, the research found that for the vast majority of nations studied, there was no substantial relationship between globalization and energy use. Globalization, on the other hand, is producing a decrease in energy consumption in the Swedish economy, according to Rjoub et al. (2021). In separate research for Turkey, Kirikkaleli et al. (2021) examined the globalization–environmental deterioration connection and discovered a positive link between globalization and ecological footprint from 1990 to 2006. Following the ongoing debate, the present research assesses the effect of globalization, technological innovation, renewable energy use, and economic growth on the ecological footprint in the BRICS economies utilizing a dataset from 1990 to 2018.
Based on the following factors, the current analysis focuses solely on the BRICS nations: 1) unlike several other developing nations, the BRICS have experienced a rapid transition from ecological surplus to ecological deficit, primarily due to the nations’ extraordinary development over the previous decade. The BRICS nations generate 21% of the world’s gross domestic product (GDP), and the overall populace accounts for 41% of the global population, with US$4 trillion in foreign currency reserves (Pata, 2021c). More importantly, the “BRICS” GDP rose from US$2,187 to US$16,266 billion from 2005 to 2016. As a result of its rapid economic progress, the countries now consume more than 40% of world energy, making them an important contributor to worldwide CO2 emissions (Juan Lin et al., 2021). As a result, robust policies are necessary to comprehend the ecological repercussions of this economic progress in order to fix the BRICS countries’ present trajectory. 2) Notwithstanding the region’s renewable energy (RE) prospects, the BRICS nations largely rely on fossil fuels to satisfy their energy consumption, thus posing severe ecological concerns. In addition, the countries’ biocapacity has dwindled as a result of their pursuit of economic progress. The BRICS nations are currently at a decision point in terms of new environmental measures, rendering the region an interesting case study for researchers seeking to understand the interrelationship between globalization, technological innovation, renewable energy use, income, and ecological footprint. Furthermore, the findings of this research will aid policymakers in developing robust policies to combat the deterioration of the environment.
This research adds to the current knowledge in three ways: based on the aforementioned debates and concerns. Firstly, as far as we know, this is the first attempt to use the innovative “method of moments quantile regression” (MMQR) for the BRICS nations to investigate the interrelationship between globalization, technological innovation, income, renewable energy consumption, and ecological footprint. Secondly, this research adds to the minimal number of studies that have used MMQR (Machado and Santos Silva, 2019). By combining this technique with fixed effects, it will become easier to obtain a practical understanding of the interrelationship’s heterogeneity. This technique also allows for heterogeneous income–emissions linkages at different conditional quantile distributions, which are not captured by traditional mean regressions. Subsequently, a thorough examination of the environmental Kuznets curve (EKC) hypothesis in the BRICS nations will be conducted. Thirdly, in the context of the EKC hypothesis for the BRICS countries, this research utilizes technological innovation to model globalization–income–environment connection. The evaluation of the EKC hypothesis at various quantiles is conducted for myriad of reasons: 1) to compare and contrast conditional mean evaluations; because of the stronger conditional quantiles, these analyses are prone to misinterpretation of the effects of outliers arising from endogenous factors (Ike et al., 2020; Shahzad et al., 2021); 2) the quantile regression is particularly convincing in the context of panel regression because it has an extra logical use; and 3) because of the exogenous factors’ distributional effects on the endogenous factors at different quantile ranges. On the other hand, the conditional mean estimate is incapable of capturing the entire distributional influence of income on emissions. It is therefore easier to characterize the diverse effects of groups of heterogeneous cross-sections in this manner.
The next section presents a synopsis of related studies. Data, Model Specification, and Methodology presents data and the method, which is followed by the Findings and Discussion, and then finally the Conclusion and Policy Path presented.
This section reveals studies conducted on the impact of technological innovation, economic growth, renewable energy usage, and globalization on ecological footprint. Over the years, several studies have been conducted regarding these interconnections; however, their findings are mixed based on the methodologies applied, timeframe, and country/countries of investigation. For example, the research of Danish et al. (2019) on the emissions–growth interconnection utilizing the autoregressive distributed lag (ARDL) approach from 1971 to 2014 in China shows that increase in ecological footprint (ECF) is caused by a 1% upsurge in GDP. Moreover, the study of Uddin et al. (2016) on the drivers of ECF in 22 countries utilizing a dataset between 1961 and 2011 and the ARDL technique disclosed that an upsurge in GDP caused an upsurge in ECF. The research also affirmed the EKC hypothesis. Likewise, utilizing dataset from 1990 to 2017, Ulucak and Bilgili (2018) scrutinized the effect of income of ECF in high-, middle-, and low-income nations from 1990 to 2016 using the CUP-BC models, and their findings showed that a 1% rise in income contributes to an upsurge in ECF. Their outcome also confirmed the EKC hypothesis. Furthermore, the study of Udemba (2020) reported a positive ECF–GDP association in Nigeria utilizing ARD and a dataset from 1971 to 2017. Likewise, the research of Destek and Sinha (2020) affirmed a positive interrelationship between ECF and growth in 24 selected nations from 1980 to 2014. The investigators used panel ARDL, and their outcomes unveiled a U-shaped interrelationship between ECF and income. Moreover the study of Magazzino et al. (2020) on the emissions–growth interconnection in South Africa unveiled that economic growth triggers environmental degradation. Moreover, the studies of Mele et al. (2021), Ulucak and Yucel (2021), Adebayo and Rjoub (2021) Magazzino et al. (2021), and Magazzino et al. (2020) also reported a positive association between economic growth and environmental degradation. Recently, scholars have started to look into the interrelationship between TI and environmental degradation utilizing various proxies of environmental degradation such as CO2 emissions, load factor, ecological footprint, and biocapacity. However, mixed findings have surfaced from these investigations. For instance, utilizing data from 1984 to 2016, Ahmad et al. (2020) scrutinized the TI influence on ECF in China. Their empirical outcome disclosed that TI plays an important role in mitigating ECF in China. Likewise, the study of Adebayo and Kirikkaleli (2021) in Japan utilizing wavelet tools between 1990 Q1 and 2015 Q4 shows that, in all frequencies, there is an in-phase (positive) coherence between TI and environmental degradation in Japan. Moreover, the research of Kirikkaleli and Adebayo (2020) using the global economy and dataset from 1990 to 2018 reported a negative coherence between TI and emissions, which demonstrates that an upsurge in TI curbs emissions. Similarly, the study of Khan et al. (2020) on drivers of ecological degradation for G-7 economies using the CS-ARDL and AMG reported that TI plays a major role in abating emissions in the G7 nations.
On the connection between ecological footprint and renewable energy utilization, significant studies have been conducted by prior scholars. For instance, the study of Pata (2021c) on the effect of REC on ECF in the United States between 1980 and 2016 using ARDL disclosed that the ECF–REC interrelationship is negative, which demonstrates that REC aids in curbing ECF. Similarly, the research of Nathaniel et al. (2021) on the ECF–REC interconnection in BRICS economies utilizing datasets from panel techniques disclosed that, in order to abate emissions in BRICS nations, REC is an essential variable. Likewise, Usman and Makhdum (2021, p. 2) assessed the ECF–REC interaction in the BRICS-T economies from 1990 to 2018 using CS-ARDL and Dumitrescu and Hurlin (DH) causality approaches, and their outcomes disclosed that REC aids in enhancing the sustainability of the environment. Similarly, the study of Xue et al. (2021) reported negative interrelationships between ecological footprint and REC in South Asian nations from 1990 to 2018 utilizing panel techniques. Moreover, the studies of Yuping et al. (2021) and Rjoub et al. (2021) for Argentina and Sweden, respectively, confirmed the negative emissions–REC interrelationship.
Regarding the interrelationship between ecological footprint and globalization, mixed findings have surfaced in the literature. For instance, the study of Yuping et al. (2021) on the emissions–globalization association in Argentina utilizing data from 1980 to 2018 disclosed that an upsurge in globalization aids in curbing emission levels in Argentina, which validates the pollution–halo–hypothesis. Likewise, the study of He et al. (2021) in Mexico from 1990 to 2018 assesses the emissions–globalization connection utilizing dual gap and ARDL. The empirical outcome from the research disclosed that globalization improves the quality of the environment. Furthermore, utilizing quantile regression, the study of Rjoub et al. (2021) in Sweden uncovered a negative emissions–globalization interrelationship. On the contrary, the study of Kirikkaleli et al. (2021) for Turkey using a dataset from 1980 to 2016 and fully modified ordinary least square (FMOLS) and dynamic ordinary least square (DOLS) approaches affirmed a positive emissions–globalization connection. Similarly, the research of Adebayo and Kirikkaleli (2021) in Japan on the emission–globalization nexus showed that globalization mitigates the quality of the environment.
The present study distinguishes itself by providing a robust analysis of the effect of economic growth, technological innovation, renewable energy consumption, and globalization on ecological footprint for the case of BRICS nations within the framework of advanced econometric methodologies including the method of moment quantile regression, fixed effects ordinary least squares (FE-OLS), DOLS, and FMOLS. Scrutinizing the combination of these methodologies aids to tap into the innovation of the methods, thereby informing robust estimates that support proactive policy directions.
The current empirical research assesses the effect of technological innovation and renewable energy use on the ecological footprint in BRICS nations as well as the role of globalization and income. The research utilized panel data for BRICS stretching from 1990 to 2018. The information of data utilized and their source are presented in Table 1. Furthermore, the trend analysis flow is depicted in Figure 1. Table 2 presents the variables’ descriptive statistics. TI mean (89,816.73) is the highest, which ranges from 3,140.000 to 1,542,002. This is followed by GDP (5,919.155), which ranges from 575.5016 to 11,993.48, globalization (GLO) (41.96485), which ranges from 14.74376 to 58.69128, REC (26.28307), which ranges from 3.180,500 to 58.65286, and ECF (2.984,167), which ranges from 0.761,262 to 6.836,081. The skewness revealed that GDP and GLO are negatively skewed while ECF, TI, and REC are positively skewed. The JB value revealed that all the variables with the exemption of GDP do not conform to normality. This outcome supports the use of non-linear technique such as MMQR.
This research assesses the interrelationship between ECF and its drivers, such as economic expansion (GDP), TI, GLO, and REC in the case of BRICS nations. In line with the studies of Kirikkaleli et al. (2021) and Shan et al. (2021), the model specifications are illustrated as follows:
where ECF, GDP, REC, TI, GLO, t, and
For comparative purposes, we employ DOLS, FE-OLS, and FMOLS. We used the MMQR approach, which enables us to examine the various effects of independent variables across multiple quantiles. Driscoll and Kraay standard errors, which are resilient to general types of cross-sectional dependency and autocorrelation up to a given lag, are added to the FE-OLS method. Heterogeneity problems with discrepancies in means between cross-sections and differences in cross-sectional modification to the cointegrating equilibriums of the conditional distribution of CO2 emissions, beneficial for a more rigorous evaluation of the empirical interrelationship, are the pivotal significant concerns in estimating dynamic cointegrated panels, as emphasized by Pedroni (2004). Pedroni’s FMOLS model incorporates individual intercepts and permits for varied serial correlation characteristics of error processes among individual panel members; therefore, it addresses these concerns appropriately. Kao and Chiang (2001) expanded the DOLS estimator to panel data settings, and premised on Monte Carlo simulations, the DOLS estimate was shown to be impartial when contrasted to both the OLS and the FMOLS estimators in finite samples. Endogeneity is also controlled via the DOLS estimator, which augments lead and lagged differences to decrease endogenous feedback.
Because prior estimate approaches had drawbacks, a panel quantile regression methodology was used to investigate the distributional and heterogeneous effect across quantiles (Ike et al., 2020) The fundamental work by Koenker and Bassett (1978) established the panel quantile regression technique. Unlike frequent least-squares regressions, which produce estimates of the conditional mean of the endogenous variable pertaining to certain values of the independent factors, quantile regressions are used to estimate the conditional median or a variety of different quantiles of the response variables subject to certain values of the exogenous variables. Quantile regressions are more resistant to outliers in the estimation process. Aside from that, it is the most useful when the conditional means of two variables have a weak or non-existent connection (Binder and Coad, 2011).
Nevertheless, we used the (Machado and Santos Silva, 2019) MMQR with fixed effects in this investigation. While a quantile regression is resilient against outliers, it does not account for unobserved variability across individuals in a panel. The MMQR technique allows for the identification of conditional heterogeneous covariance effects of CO2 emission drivers by enabling individual effects to influence the whole distribution rather than just moving means. When the panel data model is integrated with individual effects and the model has endogenous explanatory variables, the MMQR estimation approach is especially useful. The MMQR method is also straightforward since it produces non-crossing regression quantile estimates. For a framework of the location-scale variation, the conditional quantiles QY (τ|X) are estimated as follows:
The probability P {
For any fixed Xit is distributed independently and identically, and it is time independent (t). Uit is distributed independently and similarly across individuals (i) and across time (t) and is orthogonal to Xit and normalized to fulfil the moment criteria in Machado and Silva (2019), which do not entail stringent exogeneity, among many other things. The following is implied by Eq. (5).
In Equation 4, vectors of independent variables are denoted by
where
This section of the paper presents the research findings based on the methodologies applied in the Data, Model Specification, and Methodology. As an initial test, we assess the cross-sectional dependence (CSD) and slope heterogeneity (SH) before scrutinizing drivers of ecological footprint in BRICS economies. In the model, the null hypothesis is rejected based on the values of delta tilde
The CSD findings are shown in Table 4, and the results show that the null hypothesis of CSD is refuted at a level of significance of 1%. This indicates that there is a strong CSD between the variables under consideration. Taking into consideration SH and CSD in panel estimates assists policymakers in legitimizing the diverse ecological externalities associated with the indicators and, as a consequence, assists them in formulating well-structured policies.
In this empirical analysis, we utilized second-generation unit root tests, which take into account both heterogeneity and cross-sectional dependence. CADF and CIPS are reliable when there is cross-sectional dependence and heterogeneity issues. The outcomes of the CIPS and CADF (Table 5) unveiled that all the variables are I (1). This implies that all the variables (ECF, GDP, TI, GLO, and REC) are stationary at first difference. This knowledge helps in choosing suitable techniques for assessing the drivers of ecological footprint in BRICS economies.
The present research proceeds by assessing the long-run interrelationship between ECF and GDP, GLO, REC, and TI utilizing the Westerlund (2007) cointegration. The outcomes of the Westerlund test (Table 6) unveiled that in the long run, there is an association between ECF and GDP, GLO, REC, and TI. Therefore, we reject the null hypothesis of “no cointegration” in the model.
TABLE 6. Westerlund (2007) outcomes.
We proceed by assessing the effects of GDP, TI, GLO, and REC on ECF using long-run estimators (FMOLS, DOLS, and FE-OLS), the results of which are presented in Table 7. We observe that the effect of GDP on ecological footprint is significant and positive. This demonstrates that keeping other indicators constant, a 1% upsurge in GDP in the BRICS causes ecological footprint by 2.243%
Moreover, we found a negative interrelationship between REC and ECF. This illustrates that keeping other factors constant, a 1% upsurge in REC leads to a decrease of 0.2854%
Furthermore, the research shows a negative and insignificant interconnection between TI and ECF. This implies that TI does not play a vital role in curbing the degradation of the environment. This outcome shows that the TI level in the BRICS economies is not sufficient to improve the quality of the environment. The research of Adebayo and Kirikkaleli (2021) on the emissions–innovation interrelationship in Japan between 1990 Q1 and 2015 Q4 as well as the research of Udemba et al. (2021) in Chile from 1990 to 2018 reported the same result.
Lastly, we observed a negative and significant ECF–GLO interrelationship in the BRICS nations. This observation indicates that keeping other indicators constant, a 1% upsurge in globalization leads to a fall in ecological footprint by 0.3467%
The present research takes a step further by assessing the influence of income, globalization, technological innovation, and renewable energy on ecological footprint at different quantiles. We employed MMQR to assess the drivers of ecological footprint at various quantiles of ecological footprint. The MMQR is presented in Table 8. We observed that in all quantiles (first to ninth), the effect of GDP on ecological footprint is positive; although the positive effect is more pronounced in the higher quantiles of ecological footprint. This demonstrates that in all quantiles, an upsurge in GDP caused an increase in ecological footprint. Furthermore, the present research validates the EKC hypothesis across all quantiles (first to ninth). In the lower and higher quantiles, the negative effect of economic growth squared is evident, although the influence is more pronounced in the higher quantiles of ecological footprint distribution. The studies of Khan et al. (2020), Alhassan et al. (2020), and Ike et al. (2020a) validated this outcome.
Moreover, across all quantiles (first to ninth), we observed a negative influence of REC on ecological footprint, which demonstrates that REC mitigates ecological footprint across all quantiles (first to ninth). Renewable energy technology uses pure and cleaner sources of energy that are sustainable and can meet existing and future demands, which is the theoretical foundation for the negative ecological footprint–renewable energy use interrelationship in all quantiles (first to ninth). This outcome substantiates the finding of Pata (2021c). Moreover, a negative ECF–GLO interrelationship was seen in all quantiles (first to ninth). The negative interrelationship between globalization and environmental impact is due to the fact that globalization via trade also enables technical advancement and increased economic activity. The studies of Koengkan et al. (2020) for Latin America and Rjoub et al. (2021) for Sweden validated the negative environmental deterioration and globalization interrelationship. Lastly, we observed no significant interconnection between technological innovation and ecological footprint in the BRICS economies across all quantiles (first to ninth), and the result was affirmed by the studies of Ahmad et al. (2020) and Udemba et al. (2021).
Figure 2 shows a comparison of the estimated coefficients for all specifications employed, including MMQR, DOLS, FMOLS, and FE-OLS. The MMQR coefficient method is heterogeneous and produces a dynamic image across all quantiles, whereas the coefficients of FMOLS, DOLS, and FE-OLS are stable. Figure 2 depicts that the coefficients of globalization grow from the lower to upper quantiles, indicating that more globalization is beneficial to the environment. Furthermore, the MMQR technique shows that the coefficients of economic growth are increasing upward, indicating that the increase in economic growth is wreaking havoc on the environment. In contrast, the coefficients of renewable energy consumption have significantly reduced the negative impacts of economic activities on society and the environment by giving logical answers that assist policymakers in making decisions that can lead to ecologically friendly progress. As a result, when analyzing all panel estimators to provide an inclusive demonstration of connection across variables, it is clear that MMQR is an excellent and efficient approach.
FIGURE 2. Graphical representation of coefficient estimates for all variables across all quantiles, obtained from all four estimator.
The current paper explores the causality between ecological footprints and its drivers utilizing the Dumitrescu and Hurlin, (2012) causality test. The causality test unveiled feedback causal interconnection between GDP and ECF, which illustrates that GDP can forecast ECF and ECF can forecast GDP as well (Table 9). Furthermore, at significance level of 5%, the null hypothesis of “no causality” from GLO to ECF is rejected, which illustrates that GDP is a major driver of ECF in BRICS nations. Moreover, we noticed feedback causality between GDPSQ and ECF, which makes the study reject the null hypothesis of no causality. Therefore, GDPSQ and ECF can significantly predict each other. Lastly, the null hypothesis of no causality from REC to ECF is rejected at 1% level of significance. These outcomes are important for BRICS economies’ policymakers when formulating policies pertaining to environmental degradation.
The present research assesses the influence of renewable energy consumption and technological innovation on ecological footprint as well as the role of economic growth and globalization in the BRICS nations utilizing a panel dataset spanning from 1990 to 2018. The study utilized second-generation panel techniques such as CSD, SH, Westerlund cointegration, FMOLS, DOLS, FE-OLS, MMQR, and DH causality tests. The outcomes from the SH and CSD tests supported the use of second-generation techniques due to the issues of homogeneity and cross-sectional dependence. The outcomes of the Westerlund cointegration affirmed the long-run interrelationship between ecological footprint and the exogenous variables. The MMQR outcomes showed that across all quantiles (first to ninth), renewable energy use and globalization curb ecological footprint, while economic growth increases ecological footprint. The study also validated the EKC hypothesis across all quantiles (first to ninth). We applied the FE-OLS, DOLS, and FMOLS as a robustness check for the MMQR, and their outcomes showed that renewable energy use and globalization decrease ecological footprint, while economic growth increases ecological footprint. Furthermore, the DH causality results indicated that globalization and renewable energy use established unidirectional causality to ecological footprint, while Granger causality outcomes revealed that feedback causality was observed between economic growth and ecological footprint.
The results show that economic expansion has a negative impact on the environment. One of the goals of the BRICS economies is to sustain economic growth, and reducing their ecological footprint might have a negative impact on their economic progress. The deployment of green technologies and the integration of renewable energy should be the measures implemented by these nations to balance the momentum. Facilitating the transition to a green economy and modernizing industry could be utilized as ways of addressing ecological concerns while simultaneously boosting economic expansion. In order to secure economic progress, policymakers in the BRICS nations should enact stronger energy-conservation measures. Renewable energy and globalization have an important role in improving the local economy and connecting it with the international economy, as well as assisting in the creation of a healthier environment. As a result, measures aimed at enhancing various dimensions of globalization should be encouraged. There is a particular need for energy-efficient technology to spread across nations.
This is feasible when a nation takes an aggressive role in international climate change negotiations and other energy-linked global organizations. Globalization can be classified into three types: political, social, and economic. It could improve the quality of the environment through governmental action or it could have a negative influence due to pollution; each kind of globalization has a direct effect on the quality of the environment in which humans coexist. The understanding of best practices and dissemination of information about best business practices aid in the development of sustainable energy sources in economic operations, thereby promoting sustainable development.
Regarding the possibility for replacement between the various types of energy usage (NREC and REC), climate change policies should place a greater emphasis on boosting REC. They should utilize a market-based system to stimulate the use of renewable energy sources in various sectors while discouraging the usage of commonly utilized fossil fuel energy sources. As a result, policies such as tax credits for renewable energy generation, installation of renewable energy systems, and markets for renewable energy finance will go a long way toward encouraging investment in renewable energy technology. These will bring about a change in the energy mix toward greener energy, as well as the generation of sustainable energy. The significant use of renewable energy paves the way for sustainable economic progress and a transition to a low-carbon economy. According to our empirical findings, the usage of renewable energy reduces the ecological footprint in these nations. As a result, encouraging the use of renewable energy for higher economic expansion and lower ecological footprint is beneficial.
Moreover Shahbaz et al. (2018a) argued that policymakers should create a multilateral system at the global level to assure energy efficiency and encourage renewable energy utilization. Effective incentive mechanisms for market accessibility and renewable energy development should be established and applied globally. Renewable energy market development between public and private sector stakeholders in terms of advancement of technologies, project financing, and ongoing initiatives will once again contribute significantly to the considerable development of the renewable energy sector.
The study’s scope was restricted to the BRICS countries, and only a few variables were taken into account when assessing the influence of technological innovation and renewable energy use on CO2. Institutional quality, financial development, economic complexity, and human capital could be included in the model in future research. In addition, assessments at the state and local levels will be beneficial for more specific policy ramifications.
The original contributions presented in the study are included in the article/Supplementary Material; further inquiries can be directed to the corresponding author.
LD made significant contributions through administration and funding acquisition. MR made significant contributions in the methodology, results and discussion, and supervision. TA conducted the formal analysis. ÖG made significant contributions in the introduction. HI made significant contributions in the literature review and methodology. MI made significant contributions in the discussion and conclusion. RH made significant contributions in the policy recommendations. All authors contributed to the article and approved the submitted version.
The authors declare that the research was conducted in the absence of any commercial or financial relationships that could be construed as a potential conflict of interest.
All claims expressed in this article are solely those of the authors and do not necessarily represent those of their affiliated organizations, or those of the publisher, the editors, and the reviewers. Any product that may be evaluated in this article, or claim that may be made by its manufacturer, is not guaranteed or endorsed by the publisher.
Adebayo, T. S., and Acheampong, A. O. (2021). Modelling the globalization-CO2 Emission Nexus in Australia: Evidence from Quantile-On-Quantile Approach. Environ. Sci. Pollut. Res. doi:10.1007/s11356-021-16368-y
Adebayo, T. S., Akinsola, G. D., Kirikkaleli, D., Bekun, F. V., Umarbeyli, S., and Osemeahon, O. S. (2021). Economic Performance of Indonesia amidst CO2 Emissions and Agriculture: A Time Series Analysis. Environ. Sci. Pollut. Res. 28, 47942–47956. doi:10.1007/s11356-021-13992-6
Adebayo, T. S., Awosusi, A. A., Kirikkaleli, D., Akinsola, G. D., and Mwamba, M. N. (2021). Can CO2 Emissions and Energy Consumption Determine the Economic Performance of South Korea? A Time Series Analysis. Environ. Sci. Pollut. Res. 28 (29), 38969–38984. doi:10.1007/s11356-021-13498-1
Adebayo, T. S., and Kirikkaleli, D. (2021). Impact of Renewable Energy Consumption, Globalization, and Technological Innovation on Environmental Degradation in Japan: Application of Wavelet Tools. Environ. Dev. Sustain. 23, 16057–16082. doi:10.1007/s10668-021-01322-2
Adebayo, T. S., and Rjoub, H. (2021). A New Perspective into the Impact of Renewable and Nonrenewable Energy Consumption on Environmental Degradation in Argentina: a Time-Frequency Analysis. Environ. Sci. Pollut. Res. doi:10.1007/s11356-021-16897-6
Adebayo, T. S., Rjoub, H., Akinsola, G. D., and Oladipupo, S. D. (2021). The Asymmetric Effects of Renewable Energy Consumption and Trade Openness on Carbon Emissions in Sweden: New Evidence from Quantile-On-Quantile Regression Approach. Environ. Sci. Pollut. Res., 1–12. doi:10.1007/s11356-021-15706-4
Adebayo, T. S., Udemba, E. N., Ahmed, Z., and Kirikkaleli, D. (2021). Determinants of Consumption-Based Carbon Emissions in Chile: An Application of Non-linear ARDL. Environ. Sci. Pollut. Res. 28, 43908–43922. doi:10.1007/s11356-021-13830-9
Ahmad, M., Jiang, P., Majeed, A., Umar, M., Khan, Z., and Muhammad, S. (2020). The Dynamic Impact of Natural Resources, Technological Innovations and Economic Growth on Ecological Footprint: An Advanced Panel Data Estimation. Resour. Pol. 69, 101817. doi:10.1016/j.resourpol.2020.101817
Akhayere, E., Essien, E. A., and Kavaz, D. (2019). Effective and Reusable Nano-Silica Synthesized from Barley and Wheat Grass for the Removal of Nickel from Agricultural Wastewater. Environ. Sci. Pollut. Res. 26 (25), 25802–25813. doi:10.1007/s11356-019-05759-x
Akhayere, E., and Kavaz, D. (2021). Nano-silica and Nano-Zeolite Synthesized from Barley Grass Straw for Effective Removal of Gasoline from Aqueous Solution: A Comparative Study. Chem. Eng. Commun. 208 (10), 1419–1435. doi:10.1080/00986445.2020.1786373
Akhayere, E., Vaseashta, A., and Kavaz, D. (2020). Novel Magnetic Nano Silica Synthesis Using Barley Husk Waste for Removing Petroleum from Polluted Water for Environmental Sustainability. Sustainability 12 (24), 10646. doi:10.3390/su122410646
Alhassan, A., Usman, O., Ike, G. N., and Sarkodie, S. A. (2020). Impact Assessment of Trade on Environmental Performance: Accounting for the Role of Government Integrity and Economic Development in 79 Countries. Heliyon 6 (9), e05046. doi:10.1016/j.heliyon.2020.e05046
Alola, A. A., Adebayo, T. S., and Onifade, S. T. (2021). Examining the Dynamics of Ecological Footprint in China with Spectral Granger Causality and Quantile-On-Quantile Approaches. Int. J. Sust. Dev. World Ecol. 0 (0), 1–14. doi:10.1080/13504509.2021.1990158
Alola, A. A. (2019). The Trilemma of Trade, Monetary and Immigration Policies in the United States: Accounting for Environmental Sustainability. Sci. Total Environ. 658, 260–267. doi:10.1016/j.scitotenv.2018.12.212
Banday, U. J., and Aneja, R. (2020). Renewable and Non-renewable Energy Consumption, Economic Growth and Carbon Emission in BRICS. Ijesm 14 (1), 248–260. doi:10.1108/IJESM-02-2019-0007
Bandyopadhyay, A., and Rej, S. (2021). Can Nuclear Energy Fuel an Environmentally Sustainable Economic Growth? Revisiting the EKC Hypothesis for India. Environ. Sci. Pollut. Res. doi:10.1007/s11356-021-15220-7
Bekun, F. V., Emir, F., and Sarkodie, S. A. (2019). Another Look at the Relationship between Energy Consumption, Carbon Dioxide Emissions, and Economic Growth in South Africa. Sci. Total Environ. 655, 759–765. doi:10.1016/j.scitotenv.2018.11.271
Binder, M., and Coad, A. (2011). From Average Joe's Happiness to Miserable Jane and Cheerful John: Using Quantile Regressions to Analyze the Full Subjective Well-Being Distribution. J. Econ. Behav. Organ. 79 (3), 275–290. doi:10.1016/j.jebo.2011.02.005
British Petroleum. (2021). British Petroleum. Available at: Https://www.bp.com/content/dam/bp/business-sites/en/global/corporate/pdfs/energy-economics/statistical-review/bp-stats-review-2019-china-insights.pdf (accessed on 30 May 2021).
Danish, M. S., Hassan, S. T., Baloch, M. A., Mahmood, N., and Zhang, J. (2019). Linking Economic Growth and Ecological Footprint through Human Capital and Biocapacity. Sust. Cities Soc. 47, 101516. doi:10.1016/j.scs.2019.101516
Destek, M. A., and Sinha, A. (2020). Renewable, Non-renewable Energy Consumption, Economic Growth, Trade Openness and Ecological Footprint: Evidence from Organisation for Economic Co-operation and Development Countries. J. Clean. Prod. 242, 118537. doi:10.1016/j.jclepro.2019.118537
Doğan, B., Driha, O. M., Balsalobre Lorente, D., and Shahzad, U. (2021). The Mitigating Effects of Economic Complexity and Renewable Energy on Carbon Emissions in Developed Countries. Sust. Dev. 29 (1), 1–12. doi:10.1002/sd.2125
Dumitrescu, E.-I., and Hurlin, C. (2012). Testing for Granger Non-causality in Heterogeneous Panels. Econ. Model. 29 (4), 1450–1460. doi:10.1016/j.econmod.2012.02.014
Fareed, Z., Salem, S., Pata, U. K., and Shahzad, F. (2021). Role of export Diversification and Renewable Energy on the Load Capacity Factor in Indonesia. Front. Environ. Sci. doi:10.3389/fenvs.2021.770152
Farooq, M. U., Shahzad, U., Sarwar, S., and ZaiJun, L. (2019). The Impact of Carbon Emission and forest Activities on Health Outcomes: Empirical Evidence from China. Environ. Sci. Pollut. Res. 26 (13), 12894–12906. doi:10.1007/s11356-019-04779-x
Fatima, T., Shahzad, U., and Cui, L. (2021). Renewable and Nonrenewable Energy Consumption, Trade and CO2 Emissions in High Emitter Countries: Does the Income Level Matter. J. Environ. Plann. Manag. 64 (7), 1227–1251. doi:10.1080/09640568.2020.1816532
Gao, J., Xu, W., and Zhang, L. (2021). Tourism, Economic Growth, and Tourism-Induced EKC Hypothesis: Evidence from the Mediterranean Region. Empir Econ. 60 (3), 1507–1529. doi:10.1007/s00181-019-01787-1
Garrone, P., and Grilli, L. (2010). Is There a Relationship between Public Expenditures in Energy R&D and Carbon Emissions Per GDP? an Empirical Investigation. Energy Policy 38 (10), 5600–5613. doi:10.1016/j.enpol.2010.04.057
Ghazouani, A., Jebli, M. B., and Shahzad, U. (2021). Impacts of Environmental Taxes and Technologies on Greenhouse Gas Emissions: Contextual Evidence from Leading Emitter European Countries. Environ. Sci. Pollut. Res. 28 (18), 22758–22767. doi:10.1007/s11356-020-11911-9
He, X., Adebayo, T. S., Kirikkaleli, D., and Umar, M. (2021). Consumption-based Carbon Emissions in Mexico: An Analysis Using the Dual Adjustment Approach. Sustainable Prod. Consumption 27, 947–957. doi:10.1016/j.spc.2021.02.020
Ike, G. N., Usman, O., Alola, A. A., and Sarkodie, S. A. (2020). Environmental Quality Effects of Income, Energy Prices and Trade: The Role of Renewable Energy Consumption in G-7 Countries. Sci. Total Environ. 721, 137813. doi:10.1016/j.scitotenv.2020.137813
Ike, G. N., Usman, O., and Sarkodie, S. A. (2020a). Testing the Role of Oil Production in the Environmental Kuznets Curve of Oil Producing Countries: New Insights from Method of Moments Quantile Regression. Sci. Total Environ. 711, 135208. doi:10.1016/j.scitotenv.2019.135208
Khan, Z., Ali, M., Jinyu, L., Shahbaz, M., and Siqun, Y. (2020). Consumption-based Carbon Emissions and Trade Nexus: Evidence from Nine Oil Exporting Countries. Energ. Econ. 89, 104806. doi:10.1016/j.eneco.2020.104806
Khattak, S. I., Ahmad, M., Khan, Z. U., and Khan, A. (2020). Exploring the Impact of Innovation, Renewable Energy Consumption, and Income on CO2 Emissions: New Evidence from the BRICS Economies. Environ. Sci. Pollut. Res. 27 (12), 13866–13881. doi:10.1007/s11356-020-07876-4
Kirikkaleli, D., and Adebayo, T. S. (2021). Do public-private Partnerships in Energy and Renewable Energy Consumption Matter for Consumption-Based Carbon Dioxide Emissions in India. Environ. Sci. Pollut. Res. 28 (23), 30139–30152. doi:10.1007/s11356-021-12692-5
Kirikkaleli, D., and Adebayo, T. S. (2020). Do renewable Energy Consumption and Financial Development Matter for Environmental Sustainability? New Global Evidence. Sust. Dev. 29 (4), 583–594. doi:10.1002/sd.2159
Kirikkaleli, D., Adebayo, T. S., Khan, Z., and Ali, S. (2021). Does Globalization Matter for Ecological Footprint in Turkey? Evidence from Dual Adjustment Approach. Environ. Sci. Pollut. Res. 28 (11), 14009–14017. doi:10.1007/s11356-020-11654-7
Koengkan, M., Fuinhas, J. A., and Santiago, R. (2020). Asymmetric Impacts of Globalisation on CO2 Emissions of Countries in Latin America and the Caribbean. Environ. Syst. Decis. 40 (1), 135–147. doi:10.1007/s10669-019-09752-0
Koenker, R., and Bassett, G. (1978). Regression Quantiles. Econometrica 46 (1), 33–50. doi:10.2307/1913643
lin, J., Shen, Y., Li, X., and Hasnaoui, A. (2021). BRICS Carbon Neutrality Target: Measuring the Impact of Electricity Production from Renewable Energy Sources and Globalization. J. Environ. Manage. 298, 113460. doi:10.1016/j.jenvman.2021.113460
Machado, J. A. F., and Santos Silva, J. M. C. (2019). Quantiles via Moments. J. Econom. 213 (1), 145–173. doi:10.1016/j.jeconom.2019.04.009
Magazzino, C., Bekun, F. V., Etokakpan, M. U., and Uzuner, G. (2020). Modeling the Dynamic Nexus Among Coal Consumption, Pollutant Emissions and Real Income: Empirical Evidence from South Africa. Environ. Sci. Pollut. Res. 27 (8), 8772–8782. doi:10.1007/s11356-019-07345-7
Magazzino, C., Mele, M., and Schneider, N. (2021). A Machine Learning Approach on the Relationship Among Solar and Wind Energy Production, Coal Consumption, GDP, and CO2 Emissions. Renew. Energ. 167, 99–115. doi:10.1016/j.renene.2020.11.050
Mele, M., Gurrieri, A. R., Morelli, G., and Magazzino, C. (2021). Nature and Climate Change Effects on Economic Growth: An LSTM experiment on Renewable Energy Resources. Environ. Sci. Pollut. Res. 28 (30), 41127–41134. doi:10.1007/s11356-021-13337-3
Nathaniel, S. P., Yalçiner, K., and Bekun, F. V. (2021). Assessing the Environmental Sustainability Corridor: Linking Natural Resources, Renewable Energy, Human Capital, and Ecological Footprint in BRICS. Resour. Pol. 70, 101924. doi:10.1016/j.resourpol.2020.101924
Paramati, S. R., Shahzad, U., and Doğan, B. (2022). The Role of Environmental Technology for Energy Demand and Energy Efficiency: Evidence from OECD Countries. Renew. Sust. Energ. Rev. 153, 111735. doi:10.1016/j.rser.2021.111735
Pata, U. K. (2021c). Linking Renewable Energy, Globalization, Agriculture, CO2 Emissions and Ecological Footprint in BRIC Countries: A Sustainability Perspective. Renew. Energ. 173, 197–208. doi:10.1016/j.renene.2021.03.125
Pata, U. K. (2021a). Renewable and Non-renewable Energy Consumption, Economic Complexity, CO2 Emissions, and Ecological Footprint in the USA: Testing the EKC Hypothesis with a Structural Break. Environ. Sci. Pollut. Res. 28 (1), 846–861. doi:10.1007/s11356-020-10446-3
Pedroni, P. (2004). PANEL COINTEGRATION: ASYMPTOTIC AND FINITE SAMPLE PROPERTIES OF POOLED TIME SERIES TESTS WITH AN APPLICATION TO THE PPP HYPOTHESIS. Econ. Theor. 20 (3), 597–625. doi:10.1017/S0266466604203073
Santra, S. (2017). The Effect of Technological Innovation on Production-Based Energy and CO2 Emission Productivity: Evidence from BRICS Countries. Afr. J. Sci. Technol. Innovation Dev. 9 (5), 503–512. doi:10.1080/20421338.2017.1308069
Shahbaz, M., Shahzad, S. J. H., Mahalik, M. K., and Hammoudeh, S. (2018a). Does Globalisation Worsen Environmental Quality in Developed Economies. Environ. Model. Assess. 23 (2), 141–156. doi:10.1007/s10666-017-9574-2
Shahzad, U., Fareed, Z., Shahzad, F., and Shahzad, K. (2021). Investigating the Nexus between Economic Complexity, Energy Consumption and Ecological Footprint for the United States: New Insights from Quantile Methods. J. Clean. Prod. 279, 123806. doi:10.1016/j.jclepro.2020.123806
Shahzad, U., Ferraz, D., Doğan, B., and Aparecida do Nascimento Rebelatto, D. (2020). Export Product Diversification and CO2 Emissions: Contextual Evidences from Developing and Developed Economies. J. Clean. Prod. 276, 124146. doi:10.1016/j.jclepro.2020.124146
Shahzad, U., Ferraz, D., Nguyen, H.-H., and Cui, L. (2022). Investigating the Spill Overs and Connectedness between Financial Globalization, High-Tech Industries and Environmental Footprints: Fresh Evidence in Context of China. Technol. Forecast. Soc. Change 174, 121205. doi:10.1016/j.techfore.2021.121205
Shan, S., Ahmad, M., Tan, Z., Adebayo, T. S., Man Li, R. Y., and Kirikkaleli, D. (2021). The Role of Energy Prices and Non-linear Fiscal Decentralization in Limiting Carbon Emissions: Tracking Environmental Sustainability. Energy 234, 121243. doi:10.1016/j.energy.2021.121243
Solarin, S. A., Al-Mulali, U., Musah, I., and Ozturk, I. (2017). Investigating the Pollution haven Hypothesis in Ghana: An Empirical Investigation. Energy 124, 706–719. doi:10.1016/j.energy.2017.02.089
Soylu, Ö. B., Adebayo, T. S., and Kirikkaleli, D. (2021). The Imperativeness of Environmental Quality in China amidst Renewable Energy Consumption and Trade Openness. Sustainability 13 (9), 5054. doi:10.3390/su13095054
Su, Z.-W., Umar, M., Kirikkaleli, D., and Adebayo, T. S. (2021). Role of Political Risk to Achieve Carbon Neutrality: Evidence from Brazil. J. Environ. Manage. 298, 113463. doi:10.1016/j.jenvman.2021.113463
Uddin, G. A., Alam, K., and Gow, J. (2016). Does Ecological Footprint Impede Economic Growth? an Empirical Analysis Based on the Environmental Kuznets Curve Hypothesis. Aust. Econ. Pap. 55 (3), 301–316. doi:10.1111/1467-8454.12061
Udemba, E. N. (2020). A Sustainable Study of Economic Growth and Development amidst Ecological Footprint: New Insight from Nigerian Perspective. Sci. Total Environ. 732, 139270. doi:10.1016/j.scitotenv.2020.139270
Ulucak, R., and Bilgili, F. (2018). A Reinvestigation of EKC Model by Ecological Footprint Measurement for High, Middle and Low Income Countries. J. Clean. Prod. 188, 144–157. doi:10.1016/j.jclepro.2018.03.191
Ulucak, Z. S., and Yucel, A. G. (2021). Can Renewable Energy Be Used as an Effective Tool in the Decarbonization of the Mediterranean Region: Fresh Evidence under Cross-Sectional Dependence. Environ. Sci. Pollut. Res. 28 (37), 52082–52092. doi:10.1007/s11356-021-14350-2
Usman, M., and Makhdum, M. S. A. (2021). What Abates Ecological Footprint in BRICS-T Region? Exploring the Influence of Renewable Energy, Non-renewable Energy, Agriculture, forest Area and Financial Development. Renew. Energ. 179, 12–28. doi:10.1016/j.renene.2021.07.014
Wang, Z., Ben Jebli, M., Madaleno, M., Doğan, B., and Shahzad, U. (2021). Does export Product Quality and Renewable Energy Induce Carbon Dioxide Emissions: Evidence from Leading Complex and Renewable Energy Economies. Renew. Energ. 171, 360–370. doi:10.1016/j.renene.2021.02.066
Westerlund, J. (2007). Testing for Error Correction in Panel Data. Oxford Bull. Econ. Stats 69 (6), 709–748. doi:10.1111/j.1468-0084.2007.00477.x
Xue, L., Haseeb, M., Mahmood, H., Alkhateeb, T. T. Y., and Murshed, M. (2021). Renewable Energy Use and Ecological Footprints Mitigation: Evidence from Selected South Asian Economies. Sustainability 13 (4), 1613. doi:10.3390/su13041613
Keywords: ecological footprint, renewable energy consumption, technological innovation, method of moment quantile regression, carbon neutrality
Citation: Dingru L, Ramzan M, Irfan M, Gülmez Ö, Isik H, Adebayo TS and Husam R (2021) The Role of Renewable Energy Consumption Towards Carbon Neutrality in BRICS Nations: Does Globalization Matter?. Front. Environ. Sci. 9:796083. doi: 10.3389/fenvs.2021.796083
Received: 15 October 2021; Accepted: 09 November 2021;
Published: 21 December 2021.
Edited by:
Umer Shahzad, Anhui University of Finance and Economics, ChinaReviewed by:
Cosimo Magazzino, Roma Tre University, ItalyCopyright © 2021 Dingru, Ramzan, Irfan, Gülmez, Isik, Adebayo and Husam. This is an open-access article distributed under the terms of the Creative Commons Attribution License (CC BY). The use, distribution or reproduction in other forums is permitted, provided the original author(s) and the copyright owner(s) are credited and that the original publication in this journal is cited, in accordance with accepted academic practice. No use, distribution or reproduction is permitted which does not comply with these terms.
*Correspondence: Muhammad Ramzan, cmFtemFubWVoYXI3QGdtYWlsLmNvbQ==
†ORCID: Muhammad Ramzan, orcid.org/0000-0001-7803-7960; Tomiwa Sunday Adebayo, orcid.org/0000-0003-0094-1778
Disclaimer: All claims expressed in this article are solely those of the authors and do not necessarily represent those of their affiliated organizations, or those of the publisher, the editors and the reviewers. Any product that may be evaluated in this article or claim that may be made by its manufacturer is not guaranteed or endorsed by the publisher.
Research integrity at Frontiers
Learn more about the work of our research integrity team to safeguard the quality of each article we publish.