- 1Southern California Coastal Water Research Project, Costa Mesa, CA, United States
- 2County of Orange–OC Public Works, Orange, CA, United States
- 3Center for Watershed Sciences, University of California, Davis, Davis, CA, United States
A key challenge in managing flow alteration is determining the severity and pattern of alteration associated with the degradation of biological communities. Understanding these patterns helps managers prioritize locations for restoration and flow management actions. However, the choices made about how to use these flow-ecology relationships can have profound implications on management decisions (e.g., which biological endpoints, which thresholds, which seasonal flow components to use). We describe a process for using flow-ecology relationships to prioritize management actions that 1) Represents the most relevant components of the annual hydrograph, 2) Demonstrates an appropriate level of sensitivity in order to discriminate locations to inform decision making, 3) Aims to protect multiple biological assemblages, 4) Reduces misclassification of priority areas (i.e., error of omission). Our approach is based on the functional flows approach which uses multiple flow metrics that describe the frequency, timing, magnitude, duration, and rate of change of seasonal process-based components of the annual hydrograph. Using this approach, we performed a flow-ecology analysis of regional bioassessment data, through which we determined where flow alteration impacts biology and prioritized reaches for changes in flow management to protect aquatic resources in a highly urbanized region of southern California, where managing scarce water resources leads to difficult decisions about tradeoffs that require technical information. We identified three important functional flow metrics for each of two bioassessment indices, one based on benthic macroinvertebrates, and another based on benthic algae. Based on thresholds that describe levels of alteration as well as thresholds describing the probability of achieving a healthy biological condition, we compared nine biological threshold combinations for each index. We found instances of flow alteration that impact biological condition highly variable (0–100% of subbasins) between combinations and we present a method for finding the most appropriate combination for prioritizing locations for flow management. We apply the final thresholds to the study region and propose 16 subbasins of high priority for implementing flow management and restoration. Importantly, we show that focusing on a single biological group would result in biologically altered locations being effectively ignored.
1 Introduction
Flow alteration is a pervasive and global issue, the extent of which has critical consequences for shaping biological communities and regulating ecological processes (Poff et al., 1997; Bunn and Arthington 2002; Poff and Zimmerman 2010; Tonkin et al., 2018). While many documented cases of flow alteration arise from large dams and hydropower plants (Poff et al., 2007; Lehner et al., 2011; Couto and Olden 2018), flow alteration is also a product of abstraction, urban run-off and channel modification, creating a depleted or augmented flow magnitude, homogenization of seasonal fluctuations and altered timing and duration of flow events (White and Greer 2006; Zimmerman et al., 2018). The latter source of alteration is typical of semi-arid, highly urbanized regions such as Southern California (Hawley and Bledsoe 2011) and causes reduction or detriment to suitable habitat for native and endangered species. The influence of flow alteration on various ecological responses is well documented (Poff et al., 1997; Konrad et al., 2008; Poff and Zimmerman 2010; Carlisle et al., 2011; Carlisle et al., 2017; Yarnell et al., 2020) and has been evaluated in flow management assessments of stream condition and used to define flow targets and recommendations (Cartwright et al., 2017; Stein et al., 2017; Mazor et al., 2018; Maloney et al., 2021). A key challenge in managing flow alteration is determining the severity and pattern of alteration that is associated with the degradation of biological communities that warrants a management response. An additional challenge in highly altered urban areas, is understanding which seasonal-specific components of the annual hydrograph are necessary to address the impact on biological response (Yarnell et al., 2015). Understanding these patterns helps managers prioritize locations for restoration and flow management actions.
Much progress has been made in identifying important components of the annual hydrograph and relating those to biological alteration. The functional flows approach (FFA) outlined in (Yarnell et al., 2020; Yarnell et al., 2015) is a quantifiable method that determines the range and characteristics of flow in the system. FFA consists of 24 distinct functional flow metrics that describe the frequency, timing, magnitude, duration, and rate of change of seasonal process-based components of the annual hydrograph. Five key components of the natural flow regime have been identified for California (Yarnell et al., 2020, Table 1), where each component relates to one or more ecological, geomorphic or biogeochemical processes that support ecosystem function (Yarnell et al., 2015). Limited empirical studies are available that link the functional flow metrics and stream biota (but see Peek et al., 2022). However, through a literature based conceptual study, specific functional flow components and associated metrics have been selected to represent the ecological processes and importance to stream communities in California (Yarnell et al., 2020).
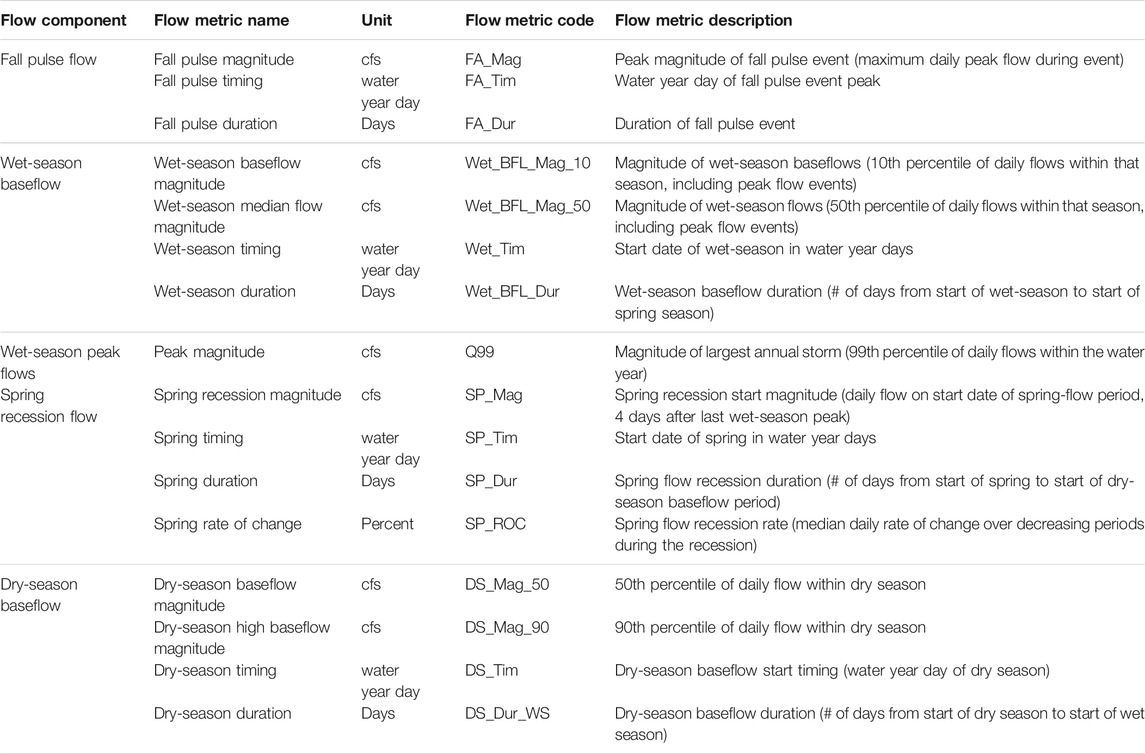
TABLE 1. Initial Functional Flow Metrics (n = 16) used in this study, modified from Yarnell et al. (2020). With their associated flow components (n = 5), codes, descriptions, and units.
Bioassessment tools based on primary producers (e.g., algae) and consumers (e.g., benthic macroinvertebrates) have been developed as indicators of stream health for aiding management decisions. The California Stream Condition Index (CSCI) and the Algal Stream Condition Index (ASCI) are predictive biological indices developed specifically for California streams (Mazor et al., 2016; Theroux et al., 2020) comprised of multiple measures of taxonomic composition and completeness, compared to reference-based benchmarks that are calculated for individual sites based on watershed characteristics. Both indices are measures of biological alteration that compare observed taxa and component metrics to values expected under reference conditions based on site-specific landscape-scale environmental variables. Both indices are intended to aid stream management and decision making and both have been integrated into unified assessments of stream health (Beck et al., 2019b). Moreover, CSCI is currently being used to evaluate regulatory compliance in regions of California (Loflen and Fetscher, 2020). These tools can be used to identify areas where hydrologic alteration is affecting biological condition. The CSCI index has been previously applied to innovatively derive flow targets through flow-ecology relationships (Stein et al., 2017; Mazor et al., 2018) to aid management decisions. In this previous application, flow alteration was measured on various temporal scales (i.e., daily, monthly, annually) but provided limited direct assessments of flow alteration on a seasonal scale (Mazor et al., 2018).
Given its pervasiveness, it is necessary to prioritize flow management in areas where biology is most impacted by flow alteration. Flow-ecology relationships can be used to inform decisions about where to prioritize flow management actions. However, this requires a series of choices be made, such as which biological endpoints to focus on, which thresholds to use to relate the change in flow (Delta H) to biological condition and which seasonal components of the hydrograph to prioritize. These choices may have substantial implications on the resulting management actions. For example, a vital first step in quantifying flow-ecology relationships is establishing connections that are functionally and biologically meaningful (Davies et al., 2014). That is, to link specific functional flow components and associated metrics to represent the ecological processes and importance to stream communities (Yarnell et al., 2020). Threshold-based approaches are frequently applied in flow-ecology analysis to first, determine a value where biological condition is considered close to reference expectations, and second, determine a limit of flow alteration associated with achieving biological reference condition. Common applications apply a single index threshold, e.g., a CSCI score ≥ 0.79 (10th percentile) that indicates biological composition similar to reference expectations (Mazor et al., 2016; Stein et al., 2017; Mazor et al., 2018; Beck et al., 2019a), and a probability threshold defined as the Delta H where the likelihood of achieving a healthy CSCI score is half the likelihood at an unaltered site (Mazor et al., 2018). However, to aid flow management prioritization decisions, the thresholds applied need to ensure there is adequate discriminatory power among locations within the study area. To achieve discriminatory power the assessment tool should demonstrate an adequate level of sensitivity. For example, a flow-ecology assessment that applies sensitive thresholds (a high index threshold together with a high probability threshold) increases the chance that most sites within the study area would be deemed biologically altered, making it challenging to identify the most impacted sites and prioritize accordingly.
In addition, focusing only on one particular group of stream biota may compromise other aspects of the stream ecosystem (Tonkin et al., 2021). Certain flow components, e.g., summer baseflow, maintain in-stream habitat for benthic macroinvertebrates (Supplementary Table S1) where augmented flows could increase habitat availability. In contrast, the same flows could impact the composition of algal communities by introducing scouring events, or lengthen the duration of scour (Schneider and Petrin, 2017). Consequently, areas considered biologically altered for algal communities could be overlooked if flow alteration impacts are only estimated for benthic macroinvertebrates.
In this study, our objectives were to demonstrate a process for using flow-ecology relationships to prioritize management actions and explore the implications of various choices (i.e., seasonal flow components, index thresholds, probability levels associated with achieving reference condition biology) on the outcomes. The process observes the following criteria:
1. Aims to protect multiple biological assemblages
2. Reduces misclassification of priority areas (i.e., error of omission).
3. Representative of the most relevant components of the annual hydrograph
4. Demonstrative of an appropriate level of sensitivity to discriminate locations to inform decision making
We demonstrate this process in the San Juan Hydrologic Unit in Southern California, where the County of Orange on behalf of the municipalities within the SJHU applied this approach to inform decisions on restoration and management prioritization in compliance with requirements under a local stormwater permit, National Pollutant Discharge Elimination System (NPDES). However, the approach can have broader applications to inform watershed management decisions.
2 Materials and Methods
2.1 Study Area
Our study area is the San Juan Hydrologic Unit (SJHU, Figure 1) located in Southern California, United States that covers an area of 496 mi2 throughout Riverside (∼18%), Orange (∼52%) and San Diego (∼30%) Counties. SJHU comprises several major watersheds that drain directly to the Pacific Ocean, including San Juan Creek, Aliso Creek, Laguna Canyon Creek, Salt Creek, and Segunda Deshecha Creek. Most of the upper tributaries of the major watersheds are undeveloped, while the lower coastal portions of the watersheds are highly urbanized (Figure 2). The major land uses in the region include developed pervious land, single- and multi-family residential homes, transportation, and open space with low vegetation. The region is characterized by a Mediterranean climate with wet winters and dry summers. This region, also known as The South Orange County Watershed Management Area (South OC WMA) has a designated Water Quality Improvement Plan (WQIP) instigated by the San Diego Regional Water Quality Control Board. Altered hydrology and channel erosion from increasing urbanization in the area has been identified as a high priority water quality condition. The identification and elimination of nuisance dry weather flows and restoration of 23,000 linear feet of degraded stream habitat are key implementation strategies to achieve the goals of the WQIP.1
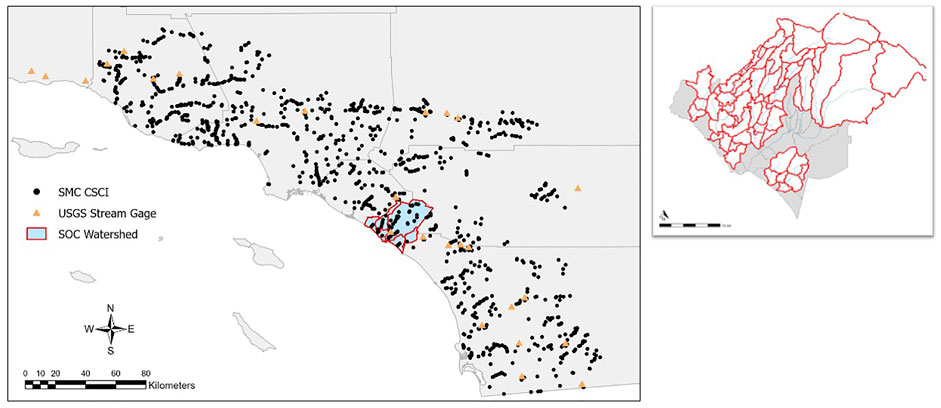
FIGURE 1. Bioassessment sites from Southern California with blue study area highlighted (left). Map of study area divided into individual subbasins (right). Red borders are subbasins assessed under the flow-ecology analysis (n = 60). Grey sub basins were not evaluated (n = 13).
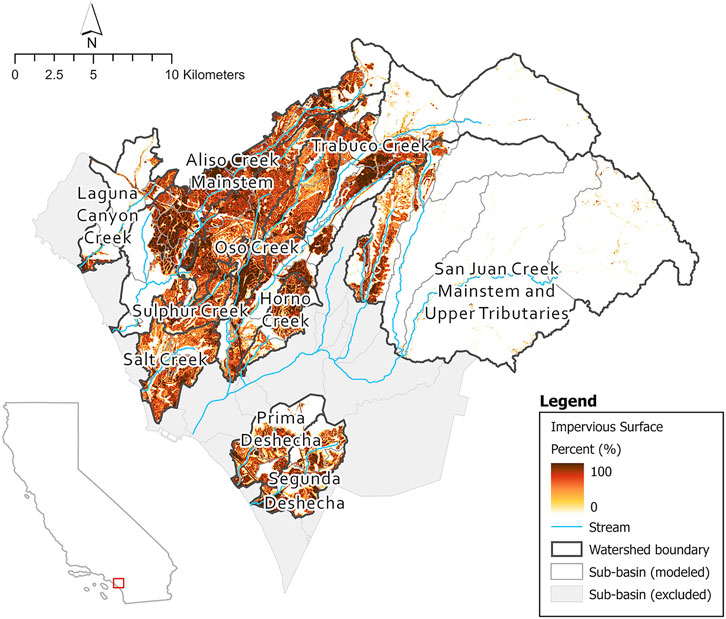
FIGURE 2. Percent impervious surfaces of all subbasins in SJHI and location in California state. NLCD 2019 urban impervious raster.4
For modeling purposes, SJHU was divided into 73 sub basins and stream segments, including areas that are heavily urbanized and areas that remain mostly natural. However, as some subbasins in the SJHU have existing flow management (n = 13), they were not included in the analysis. The subbasins were delineated following Taniguchi-Quan (this issue).
2.2 Overview of Approach
The process we provide observes the following criteria:
5. Aims to protect multiple biological assemblages
6. Reduces misclassification of priority areas (i.e., error of omission).
7. Representative of the most relevant components of the annual hydrograph
8. Demonstrative of an appropriate level of sensitivity to discriminate locations to inform decision making
Acknowledging these criteria, we explored the implications of various choices outlined above through the following process: Bioassessment data collected from wadeable streams across southern California were modeled with Functional Flow Metrics (FFM) calculated from regional flow models developed for southern California (Sengupta et al., 2018) to create regional flow-ecology curves. Ecologically meaningful relationships were determined through a FFM filtering process that included boosted regression tree analysis and generalized linear models. Three thresholds relating to the index score, i.e. that defines a reference or altered biological condition, and three thresholds of probability, i.e. defining the point in the curve at which the Delta H limit is determined, were combined to create nine threshold combinations for each index (e.g., 0.79 index threshold and 0.5 probability threshold represents one combination, Table 2). All threshold combinations were compared for each chosen FFM. To estimate where altered flow impacts biological condition (hereafter referred to as biological alteration) in the study area, FFM calculated from a hydrological model were used to predict the probability of achieving a healthy bioassessment index score for each subbasin. These scores were used to define biological alteration for each threshold combination using specific criteria. The threshold combinations were compared and tested for appropriate discriminatory power for use in prioritization decision making. The final combinations were used to prioritize subbasins for management actions for both bioassessment indices separately as well as a synthesized combination of both indices. Our recommendations are based on the outcome of this approach.
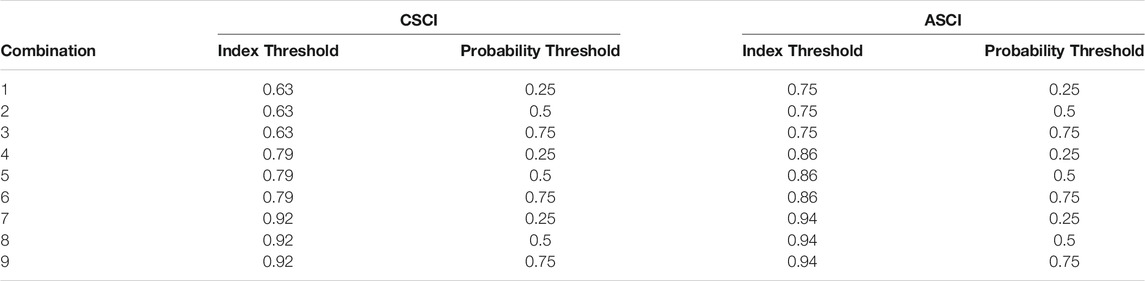
TABLE 2. Threshold combinations (n = 9) for CSCI and ASCI. Each combination consists of one index threshold and one probability threshold.
2.2.1 Biological Data
We gathered data from 480 unique bioassessment sites sampled under a variety of long term, statewide ambient monitoring programs in the southern California region that were selected probabilistically and visited between 2001 and 2018. Samples containing benthic macroinvertebrate data were available from 420 sites, and samples containing algae data were available from 324 sites. For sites with multiple sampling events, we selected the most recent sampling. Each site consisted of a 150-m assessment reach that was divided into 11 equidistant transects. At each transect, benthic macroinvertebrates were collected using a technique-frame kick net, and algae were collected using sampling tools appropriate for the substrate at each location (i.e., a rubber delimiter for small, hard substrates; a plastic delimiter for soft substrates, and a syringe scrubber for large hard substrates) following the standardized protocol outlined in Ode et al. (2016); thus, both assemblages were sampled from microhabitats (e.g., riffles, pools, fallen wood) in proportion to their relative abundance within the reach. Taxonomic analyses were performed according to Woodard et al. (2012) for benthic macroinvertebrates and Stancheva et al. (2015) for algae.
We calculated biological index scores for all samples and generated benthic macroinvertebrate CSCI scores following (Mazor et al., 2016) and algal ASCI scores following (Theroux et al., 2020). Both the CSCI and the ASCI are predictive indices that incorporate site-specific landscape scale environmental variables (e.g., watershed area, geology, and climate) into predictions of reference expectations. The CSCI index is comprised of two components: a multi-metric index and a ratio of observed/expected (O/E) taxa. The ASCI is a multi-metric index and is calculated using a hybrid combination of diatoms and soft-bodied algae. As primary producers, diatoms and soft-bodied algae are sensitive bioindicators that are responsive to multiple stressors, including temperature, nutrients, and flow (Stevenson et al., 2010; Tornés and Sabater, 2010; Stancheva and Sheath, 2016), and we opted to apply the hybrid index in this study due to its sensitivity and incorporation of multiple assemblages (Theroux et al., 2020). Index scores were calculated for each site for the year in which the sampling took place, this resulted in one score per site through the time series.
2.2.2 Hydrological Data
Though the large majority of bioassessment sites were ungauged, we leveraged the readily available, modeled flow timeseries from an ensemble of regional HEC-HMS rainfall–runoff models developed for southern California (Sengupta et al., 2018). In brief, simple mechanistic ensemble models were calibrated on 26 gauges (Figure 1) using high quality hourly streamflow and precipitation data. The ungauged reaches were assigned to the most similar gauged reaches through a random forest model using both natural and anthropogenic catchment characteristics. At each bioassessment site, daily flow timeseries were modeled under current and reference conditions, where reference conditions were defined as no developed land and zero imperviousness and used the same rainfall timeseries as the current condition. FFM (also see Section 2.3) were calculated annually from the reference and current timeseries for each site using the Functional Flows Calculator API client package in R (version 0.9.7.2, https://github.com/ceff-tech/ffc_api_client). The package uses hydrologic feature detection algorithms, which includes iterative Gaussian smoothing, feature detection, and a data windowing methodology to detect the timing of seasonal flow transitions, developed by Patterson et al. (2020) from the Python functional flows calculator.2 The functional flows calculator has difficulty detecting the timing of seasonal flow transitions (i.e., transition from dry-season to wet-season or wet-season to spring recession) if the annual hydrograph lacks seasonality. In such cases, the timing, duration, and magnitude metrics cannot be estimated for the water year. If timing values were not quantified with the calculator, we used the median timing value calculated across the period of record, to calculate the seasonal magnitude metrics for dry-season and wet-season baseflow and spring rate of change. If there were less than 5 timing metric values calculated across the period of record, we used the median timing value for the given water year across all sites. Delta H (the difference in flow metric from current to reference) was estimated as a measure of flow alteration (Sengupta et al., 2018). The total number of years with Delta H estimations varied by site, ranging from 1 to 23 years and an average of 15 years per site. Fall pulse flow may not be observed every water year, and therefore, may have limited number of Delta H values calculated at a given site. Note that the peak magnitude metrics (i.e., 2-year, 5-year, and 10-year flood magnitude, timing, frequency, and duration) that were identified in the suite of functional flow metrics for California (Yarnell et al., 2020) were not utilized in this study because 242 sites had modeled flow timeseries with less than 20 years, primarily due to gaps in the rainfall data. Instead, we used the 99th percentile of daily flow each year, referred to herein as the magnitude of the largest annual storm, as our peak magnitude flow metric which was found to have strong importance to CSCI (Mazor et al., 2018). Therefore, we used a total of 16 FFM in our analysis.
2.3 Identifying the Most Relevant Flow Components
2.3.1 Determining Relative Importance of Flow Metrics
To determine the importance of each FFM in predicting the values of bioassessment indices, Boosted Regression Trees (BRTs) were developed for both CSCI and ASCI scores, using the Delta H of all FFMs as predictors. BRTs are a nonparametric, part regression (decision trees) and part machine learning (boosting), application commonly used in ecological analysis (Elith et al., 2008; Elith and Leathwick 2015) and have been successful in similar applications for variable selection (Irving et al., 2020) and analysis (Peek et al., this issue). BRTs were chosen over other modeling approaches to deduce the relative importance of variables as they are able to fit complex nonlinear relationships between the predictors (i.e., FFMs) and the response (i.e., index scores) (Elith et al., 2008). BRTs are also robust to correlation, outliers and handle metrics of varying scales without the need to standardize (Friedman 2001; Elith et al., 2008). The percentage explained variance for the BRTs was calculated using the formula: null deviance–residual deviance/null deviance. We applied a 10-fold cross-validation procedure through the gbm. step function in R package dismo (Elith et al., 2008; Hijmans et al., 2020) with method = “Gaussian”. The following BRT criteria were applied: CSCI; learning rate = 0.005, bag fraction = 0.8 and tree complexity = 5, ASCI; learning rate = 0.003, bag fraction = 0.8 and tree complexity = 5.
2.3.2 Flow-Ecology Relationships
Flow-ecology analysis was performed on both bioassessment indices and individual FFMs separately. This analysis relates Delta H to biologically relevant flow alteration, i.e., location of sites where altered flow likely impacts the biology. All analysis was carried out in R statistical programming version 4.0.2 (R Core Team, 2020). Generalized Linear Models (GLMs) were applied on each response variable separately, ASCI and CSCI, with a single predictor variable (FFM), repeated for each FFM (n = 16). The GLMs were applied with binomial error distribution (1,0) with logit link function. Delta H is a value either higher or lower than reference condition, therefore separate GLMs were performed on positive and negative Delta H gradients for each FFM to create a full curve. The GLMs were compared by using the relative measure of model fit Akaike Information Criterion (AIC) where a lower value denotes a better fitting model. The probability of achieving a healthy index score was extracted from each GLM and scaled between 0 and 1. Delta H limits were determined by estimating the negative and positive value associated with each probability threshold, that is, the value on the X axis (Delta H) at the point in which Y (probability of achieving a healthy score) intercepts the flow-ecology curve.
The CSCI and ASCI scores contained one value per site for each index throughout the time series. The scores were converted to binary format using the index thresholds outlined in Section 2.4. The index data were combined with the FFM data to produce a value of each, per site. FFM data is an annual time series, therefore the median Delta H value across the modeled period of record was calculated to have one value per site as per the index data. Metrics for sites that contained only one delta H value were removed from analysis. Here, median Delta H was applied as a proxy for flow alteration.
2.3.3 Identifying the Most Relevant Seasonal Flow Metrics
To identify the most relevant FFM for analysis, we prioritized metrics based on relevancy and amenability to management actions using the following process: 1) removed FFM that showed less than 5% relative importance from the BRT analysis, 2) removed highly correlated variables (Spearman, >0.7) and retained the metric with the lowest AIC value from the GLMs (based on the 10th percentile score value) per pairwise comparison, 3) ensured data density was sufficient for analysis (i.e., flow-ecology relationships were not driven by only very few data points), 4) ensured metrics chosen could be influenced through flow management actions.
2.4 Identifying Appropriate Bioassessment Index Thresholds and Levels of Probability
Each index had three identified thresholds of alteration that correspond to 1) Likely altered (ASCI: 0.75, CSCI: 0.63), 2) possibly altered (ASCI: 0.86, CSCI: 0.79), and 3) likely intact (ASCI: 0.94, CSCI: 0.92), which correspond to the first, 10th and 30th percentile value of the index based on the distribution of reference scores (Mazor et al., 2016; Theroux et al., 2020). The index threshold is the score in which to transform the continuous index score to binary (1: reference condition, 0: altered condition) as the response input for the GLMs. Following the FFM filtering process, GLMs were performed, as above, on the chosen FFM and bioassessment indices formatted with each index threshold. Here, the AIC values from each model are reported, but not used for comparison. The probability threshold is the point in the curve where we determine the Delta H limits and ranges from 0 to 1. For simplicity and to include a full range of values, we tested the probability thresholds of 0.25, 0.50 and 0.75. This resulted in 9 threshold combinations for each FFM (Table 2).
Flow-ecology analysis (as described in Section 2.3.2) was conducted for each index threshold and Delta H limits were extracted for each bioassessment index, chosen FFM and threshold combination.
Delta FFM in the SJHI study area was calculated through a continuous Loading Simulation Program in C++ (LSPC) following Taniguchi-Quan et al. (this issue), which resulted in one Delta H value per subbasin (n = 60) per year (1994–2018).
2.5 Determining Biological Alteration
Biological alteration for each threshold combination was annually classified using the following criteria:
• Biologically Altered: if change in FFM falls outside of Delta FFM limits
• Biologically Unaltered: if change in FFM falls within Delta FFM limits
The percentage of subbasins biologically altered for each threshold combination for both bioassessment index and single FFM were plotted over time. Any threshold combinations that had percentage altered subbasins between 25 and 75% were kept for further analysis. In cases where more than three combinations were determined, the limits were reduced to 40–60%. This process was repeated for each FFM and the combination that appeared consistently in all FFM for each bioassessment index was chosen for the final analysis.
Biologically altered years based on the final threshold combination for each index were summarized as a percentage of the modelled period (1994–2018), which were used to synthesize alteration across all chosen FFM within each subbasin. The subbasin was classified as “likely altered” if at least two of the three chosen FFM were altered for >50 percent of years. This resulted in an alteration map for both CSCI and ASCI.
To determine priority subbasins, we synthesized biological alteration across bioassessment indices the following criteria was applied:
• High priority: Both indices indicate biologically altered flow
• Medium priority: One index indicates biologically altered flow
• Low priority: Neither index indicates biologically altered flow
Biological alteration was mapped by subbasin for each index separately and prioritization was mapped using the synthesized combination of indices. All maps were created in R statistical programming version 4.0.2 (R Core Team, 2020), using packages “sf” (Pebesma, 2018) and ggmap (Kahle and Wickham, 2013) with spatial projection of NAD83, California zone 6 (ft US).
3 Results
3.1 Identifying the Most Important Flow Components
The FFM filtering process resulted in three ecologically meaningful flow-ecology relationships for each bioassessment index.
3.1.1 Determining Relative Importance of Flow Metrics
The BRTs demonstrated a consistent ability to discriminate FFM relationships to index scores; CSCI (explained variance: 0.37), ASCI (explained variance: 0.3). The first step of the FFM filtering process (BRT relative importance, Figure 3; Supplementary Table S2) showed that Q99 had the highest relative importance for both CSCI and ASCI (CSCI; 51.5%, ASCI; 55.2%). In models applied on CSCI, FFMs describing timing and duration showed the highest relative importance (i.e., Wet_BFL_Dur; 12%, SP_Tim; 5.5%). In models applied on ASCI, FFM describing magnitude and duration had the highest relative importance (SP_Mag; 9.4%, DS_Dur_WS; 5.6%, SP_Dur; 5.3%). FFM describing Fall Pulse flows showed relatively low importance for both bioassessment indices so were removed from further analysis.
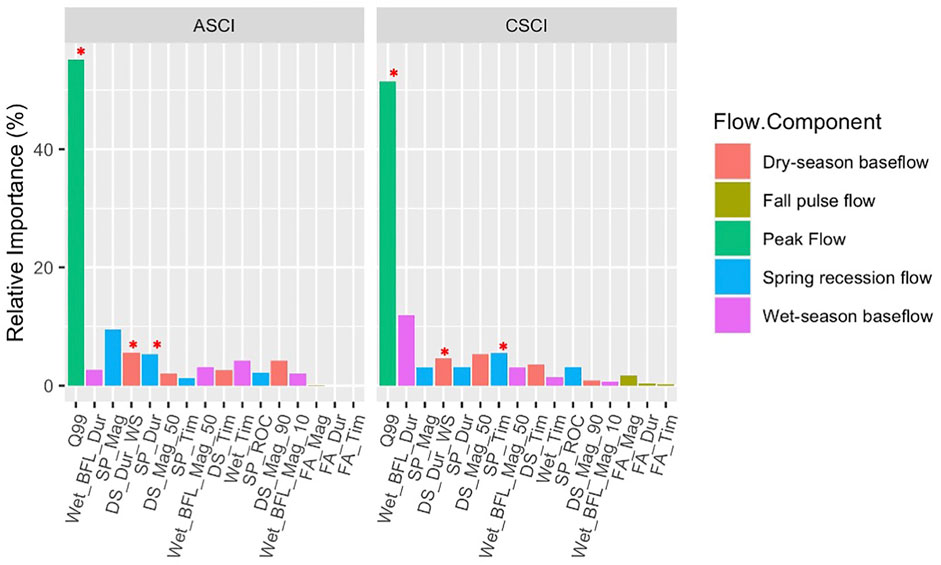
FIGURE 3. Relative importance of FFM for each bioassessment index extracted from BRTs, color coded by flow component. Red stars indicate the chosen metrics.
3.1.2 Flow-Ecology Relationships
The AIC of the flow-ecology relationships between bioassessment indices and FFM was wide ranging for both CSCI (AIC: range = 13.5–396.3, mean = 215.3 ± 20.9) and ASCI (AIC: range 15.4–324, mean = 175 ± 17.1). All flow-ecology figures available in Supplementary Material.
3.1.3 Identifying the Most Relevant Metrics
GLM figures, coefficients (AIC) and data density (n) for all FFMs and indices are available in Supplementary Table S3 and Supplementary Figures S2A,B. The FFM for CSCI with high relative importance were Q99, Wet_BFL_Dur, SP_Tim, DS_Mag_50 and DS_Dur_WS. Q99 and Wet_BFL_Dur were highly correlated (Spearman: 0.79), however, as Q99 showed such high relative importance it was kept in the analysis (Figure 3). From the remaining highly important metrics, SP_Tim and DS_Dur_WS were kept for further analysis. Although not correlated and the relative importance of DS_Mag_50 was slightly higher than DS_Dur_WS, DS_Mag_50 showed a positive relationship with increasing alteration for positive Delta (i.e., alteration), therefore was deemed unrealistic and removed from analysis (Supplementary Figure S2). The final FFM for CSCI had sufficient data density for both negative and positive Delta H GLMs.
The FFM for ASCI with high relative importance were Q99, SP_Mag, SP_Dur, and DS_Dur_WS. There was a high correlation between Q99 and SP_Mag (Spearman; 0.73). similar to CSCI, as Q99 showed such high relative importance it was kept in the analysis (Figure 3) and. SP_Mag was removed. The remaining two FFM; SP_Dur, and DS_Dur_WS were not correlated, therefore remained in the analysis. The final FFM for ASCI had appropriate data density for both negative and positive Delta H GLMs. It is important to note that the negative delta Q99 was driven by one or two outliers in both CSCI and ASCI flow-ecology curves (Figures 4, 5), however as no biologically altered subbasins in the SJHU showed depleted Q99 (see Section 3.3) and this metric showed the highest relative importance overall, Q99 was retained for further analysis.
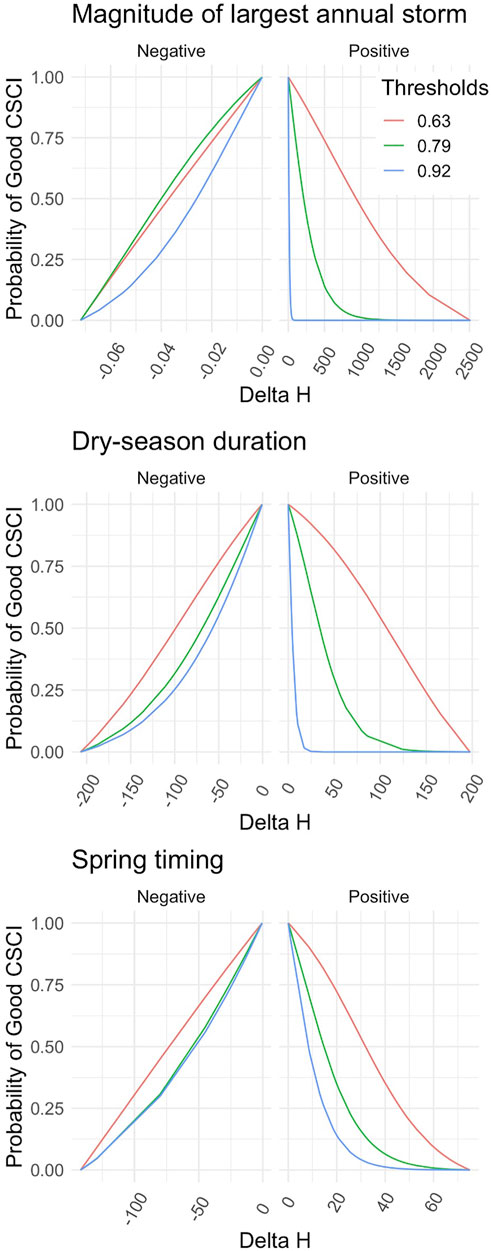
FIGURE 4. Flow-Ecology relationship between probability of achieving a healthy CSCI score and all chosen FFM. Each threshold refers to the first, 10th and 30th CSCI score percentile, i.e., likely altered possibly altered and likely intact.
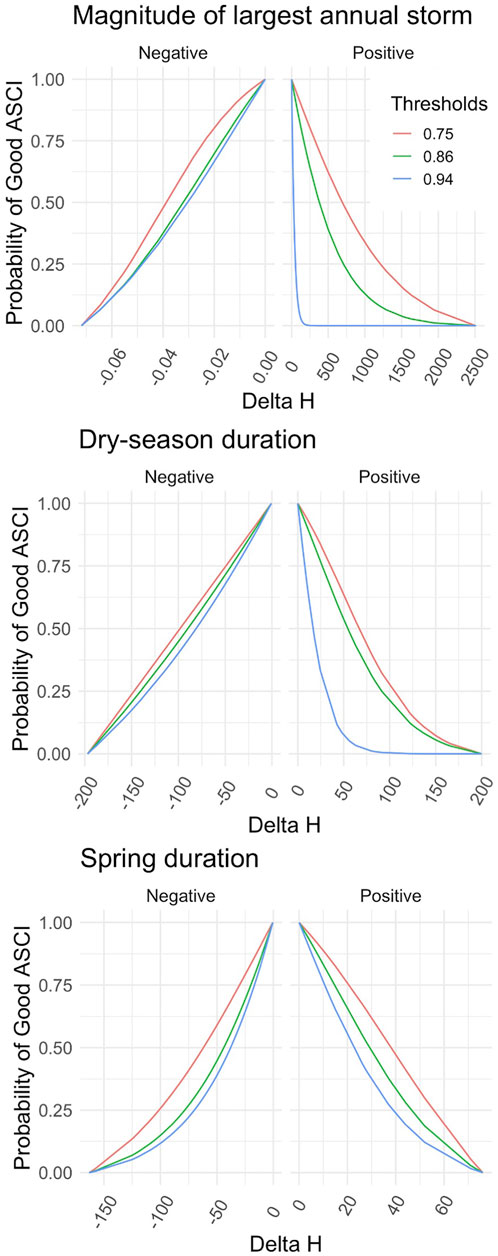
FIGURE 5. Flow-Ecology relationship between probability of achieving a healthy ASCI score and all chosen FFM. Each threshold refers to the first, 10th and 30th score percentile, i.e., likely altered, possibly altered and likely intact.
3.2 Identifying Appropriate Bioassessment Index Thresholds and Levels of Probability
Applying the different combinations of index thresholds on the GLMs and probability thresholds produced highly varied Delta H limits (Supplementary Table S4; Figures 4, 5). For example, the most conservative combination for CSCI and DS_Dur_WS (index: 0.92, probability: 0.75, Delta H limits: −26.17 to 1.95 days) and the most liberal combination (index: 0.63, probability: 0.25, Delta H limits: −146.5 to 148.4 days) showed a percentage difference of 165.8% (266 days). This difference comprises 71.7% of the entire range of Delta H values (372 days) in the study area across all subbasins and years.
The delta H limits extracted from the flow-ecology analysis for all threshold combinations applied to the 60 subbasins of the study area produced biological alteration that ranged widely (Figure 6; Supplementary Table S6). The most lenient threshold combination (CSCI = 0.63, ASCI = 0.75, Probability = 0.25) in each panel, generally shows the lowest level of alteration of all combinations. The most stringent combinations (CSCI = 0.92, ASCI = 0.94, Probability = 0.75) show a much higher level of alteration for all FFM. The yellow color throughout the subbasins for Q99 (Figure 6 top panel) indicates that both indices show low alteration throughout the study area for most threshold combinations. The remaining FFM for both CSCI and ASCI show higher and more varied levels of alteration throughout the study area and for the threshold combinations indicated by the varied blue (high alteration), green (moderate alteration) and yellow (low alteration) subbasins. The percentage of biological alteration over subbasins and years ranged between 0 and 100 for both CSCI and ASCI across all chosen metrics and subbasins. Threshold combinations applied on CSCI models initially deduced several combination options for DS_Dur_WS and SP_Tim (Figure 7). However, only one combination within the % biological alteration limits for Q99 (index: 0.92, Probability: 0.25), which corresponded with combination determined for DS_Dur_WS and SP_Tim. Threshold combinations applied on ASCI models deduced several combination options with one combination consistently determined for each FFM (index: 0.94, Probability: 0.50). Delta H limits for the final threshold combinations, together with overall biological alteration are outlined in Table 3.
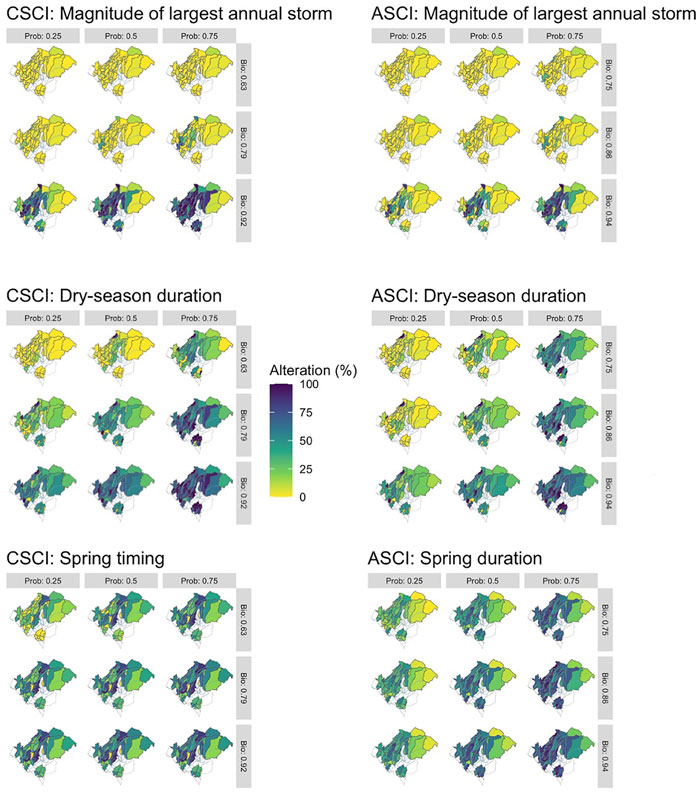
FIGURE 6. Threshold combinations comparison for CSCI, ASCI and all chosen FFM. Horizontal values are the probability thresholds and vertical are the index thresholds. Percentage alteration is percentage of years deemed as altered following the alteration definition.
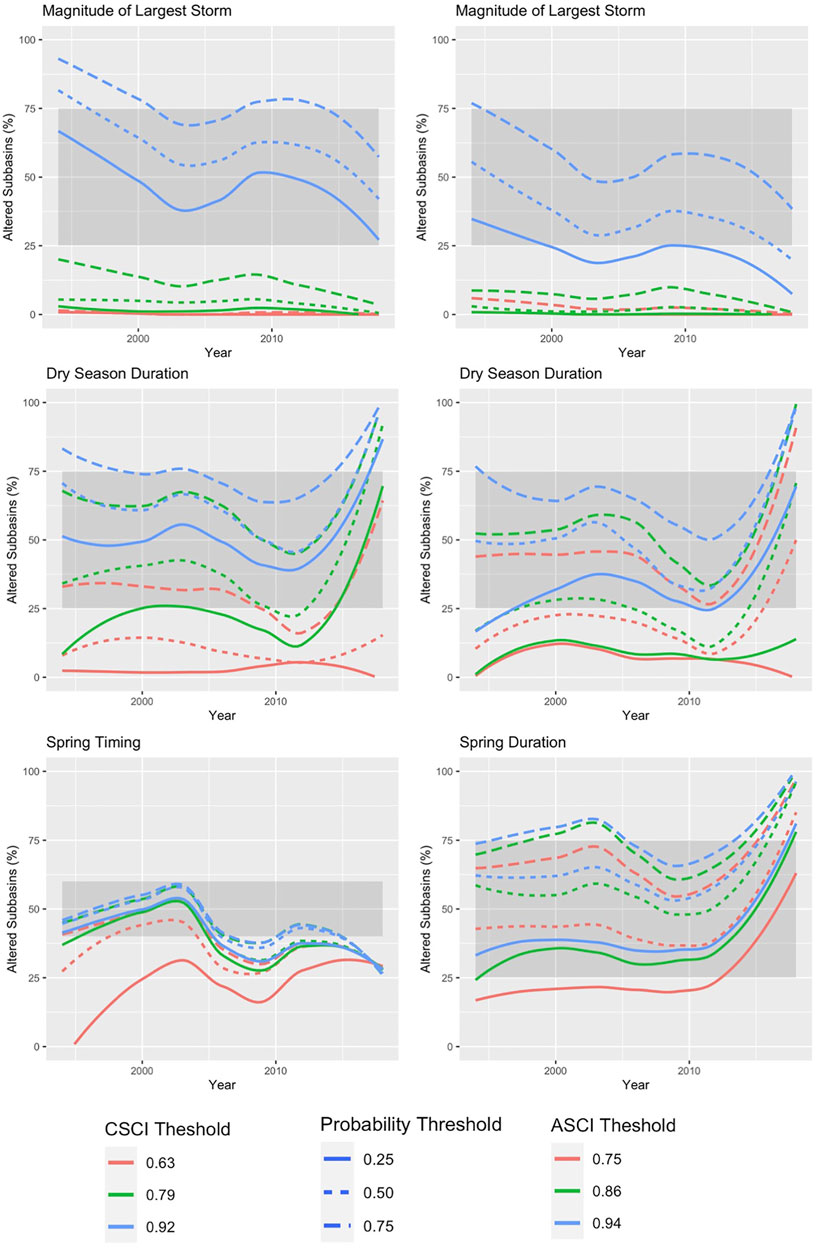
FIGURE 7. Percentage of subbasins classified as altered each year for the modelled period of record (1994–2018) under each threshold combination for CSCI (left panel) and ASCI (right panel). The grey box indicates the range of altered subbasins (%) needed to provide discriminatory power. The threshold combination lines must be within the grey box throughout the time series to remain in the analysis.

TABLE 3. Final threshold combinations for each chosen metric, mean alteration over all sites and years and delta H limits for each FFM. Percentage of years altered per subbasin available in Supplementary Table S5.
3.3 Determining Biological Alteration
Biological alteration for each index (Figure 8) showed fewer subbasins likely to be altered (n = 20) for CSCI than ASCI (n = 29). The synthesized prioritization map (Figure 8) shows varied levels of biological alteration throughout the study area (High priority; n subbasins = 16, Medium priority; n subbasins = 17, Low priority; n subbasins = 27). The subbasins determined as medium priority consisted of 4 subbasins deemed likely altered for CSCI and 13 subbasins deemed likely altered for ASCI. In most cases of alteration, the FFM show higher values than the delta H limits for each bioassessment indices, i.e., Q99 (n = 16), SP_Tim (n = 16) and DS_Dur_WS (n = 15) in subbasins determined as high priority. In contrast, SP_Dur showed lower values than the delta H limits for the majority of high priority subbasins (n = 13).
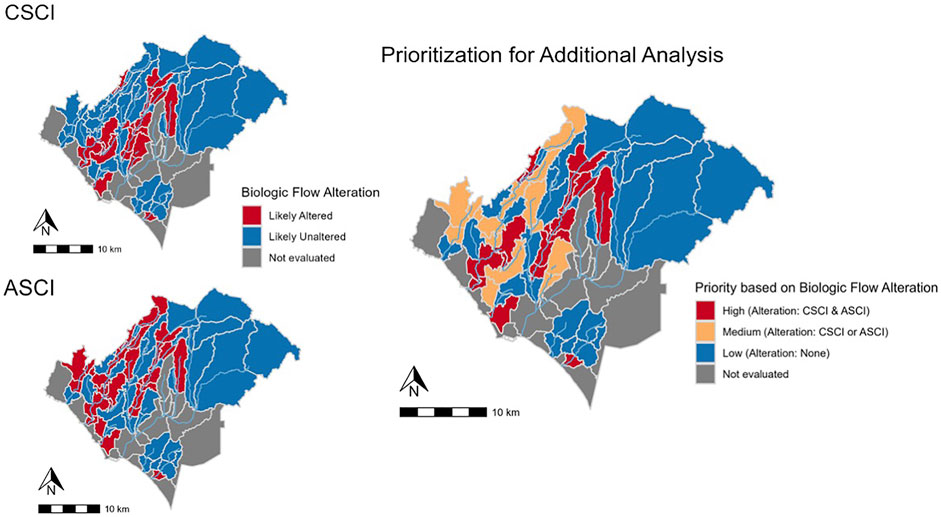
FIGURE 8. Locations of biological alteration for CSCI and ASCI individually (left panel) and final prioritization using synthesized alteration from both bioassessment indices (right panel).
4 Discussion
Through the analysis, we have shown that applying different thresholds for different bioassessment indices can vastly impact the outcome of biological alteration assessments. These differences could be instrumental in determining priority locations for flow management or restoration. In addition, by focusing on most relevant seasonal flow metrics we are able to make recommendations for flow management on specific aspects of the hydrograph that impact biological communities.
4.1 Prioritization
Our prioritization analysis identified locations of basins that are high, medium, and low priority for management actions. Importantly, 17 subbasins were determined as medium priority. These subbasins outline the locations where biological alteration was determined as altered for one, but not both, bioassessment indices. By using only one index, these locations would have been mis-classified as high or low priority. This miscalculation would have resulted in several subbasins not being designated for management actions, effectively being ignored, as well as several subbasins requiring management that could compromise other aspects of the stream ecosystem (Tonkin et al., 2021). This result adheres to two items of our process criteria 1) Aiming to protect multiple biological assemblages and 2) Reduces misclassification of priority areas (i.e., error of omission).
In this study, ASCI and CSCI were impacted mostly through augmented flow metrics where the values were higher than the associated upper Delta H limits. That is, three flow metrics, magnitude of largest storm (96%), dry season duration (86–89%) and spring timing (100%) were frequently too high or too long, respectively, to support healthy algae and macroinvertebrate communities. These exceedances could be a result of increased flows in the region due to urban run-off, including irrigation overspray which can augment baseflows and flashier storm events from an increase in impervious surfaces also impacting peak flows (Hawley and Bledsoe 2011). Spring recession duration varies in direction of alteration, that is, some subbasins have flows that are too long, while others are too short. It is challenging to discuss the source of depletion without further modeling or investigation, however the high variability in the direction of the spring recession duration (overall 31 subbasins showed a duration that was too short) could be caused by the rain dominated, highly impervious system promoting flashy flow that reduces the prominence of the spring recession compared to natural conditions or those observed in snow dominated systems (Yarnell et al., 2010).
The locations classed as high priority are generally characterized by a high level of imperviousness from urbanization (Figures 2, 8). However, several subbasins, i.e., in Trabuco Creek consist of lower imperviousness than the surrounding subbasins yet are still classified as high priority. These subbasins may be impacted by development in the adjacent subbasins from which urban run-off flows into the creek through multiple outflows in the Trabuco Creek area.3 The seven high priority subbasins are hydrologically connected, therefore impacts downstream may be the result of accumulative effect of augmented flows upstream, which is potentially also the situation in Lower Aliso Creek. The high priority subbasins, located in urbanized areas, are the places that need the most attention from flow managers. There are substantial socioeconomic considerations associated with restoration and flow management within urban streams. To reduce augmented flows from urban run-off, management will need to focus on specific source-control techniques in conjunction with community commitment (Fletcher et al., 2013). Under these circumstances it is vital to maintain support of the local community, however balancing perspectives from cultural, political, and ecological viewpoints can create critical challenges (Wohl et al., 2015).
The locations classed as low priority are generally characterized by low imperviousness (Figures 2, 8) and hence, are less impacted by urbanization and exhibit more natural conditions, resulting in less degradation. Exceptions to this rule, are the subbasins located in Oso Creek, including the confluence with Trabuco Creek. Interestingly, nine subbasins were identified as low priority in highly impervious areas (Figures 2, 8). Three clusters of two subbasins are hydrologically connected and comprise much of Oso Creek and tributaries of San Juan creek as well as the smaller watershed of Salt Creek. The remaining four subbasins are not hydrologically connected but contain sections of Aliso Creek. These subbasins may show some form of resilience to flow alteration dependent on several biological and physical factors likely acting on different scales (see Mazor et al., 2018), such as water abstraction reducing the volume of urban run-off. Further investigation of potential influencing factors will be necessary to fully understand the resilience to flow alteration in these subbasins.
4.2 Identifying Appropriate Bioassessment Index Thresholds and Levels of Probability
Our comparison of index and probability combination thresholds adheres to our process criteria item: demonstrating an appropriate level of sensitivity to discriminate locations to inform decision making. Committing to specific thresholds is not strictly a scientific distinction, however, it is a decision that could impact flow management outcomes.
In most applications of CSCI and ASCI, the 10th percentile value (possibly altered, CSCI: 0.79, ASCI: 0.86) is applied (Stein et al., 2017; Mazor et al., 2018; Beck et al., 2019a; Beck et al., 2019b). We show that for determining prioritization the most useful threshold was the 30th percentile for both indices (likely intact, CSCI: 0.92, ASCI: 0.94) due to both sensitivity and discriminatory power.
CSCI relationships to FFM were variable across different combinations of thresholds and probabilities (e.g., Figure 7, Dry Season Duration). This variation may stem from their diverse life histories and hence direct responses to variations in streamflow (Konrad et al., 2008; Rehn 2009). ASCI relationships to FFM were also variable (Figure 7 right panel), although, with the chosen threshold combination we see a higher amount of biological alteration overall for ASCI (Figure 8) than CSCI. The combination able to discriminate algal response effectively was the second most stringent combinations in the analysis, which suggests that extreme flow alteration is needed to drastically impact algal communities. Previous studies indicate that flow influences algal communities (Kirkwood et al., 2009; Miller et al., 2009; Schneider and Petrin 2017; Cheng et al., 2019). However confounding factors (i.e., water quality) mediated by altered flow regimes (Allan 2004; Nilsson and Renöfält 2008; Lange et al., 2016), are likely also driving algal condition in these subbasins. Further investigation would be needed understand the impact of flow-mediated water quality on algal communities to ensure a successful response to flow management and restoration (Suren et al., 2003).
4.3 Identifying the Most Important Flow Components
Through our BRT and GLM analysis we deduced the most important flow components for our study, thus abiding by our criteria item to represent the most relevant components of the annual hydrograph. By applying the functional flows approach we can deduce a more mechanistic understanding of the relationship between flow and the bioassessment indices. The flow metric describing the magnitude of the largest storm (Q99) was the most important metric for both CSCI and ASCI. This is not surprising, as peak flows support many physical (e.g., maintenance and rejuvenation of habitat), biogeochemical (e.g., increase nutrient cycling and exchange) and biological (e.g., limit nonnative species through natural disturbance) functions (Supplementary Table S1). This relationship agrees with several studies that have determined peak flow metrics as important influences on macroinvertebrates (Buchanan et al., 2013; Stein et al., 2017; Mazor et al., 2018; Yarnell et al., 2020; Bower et al., 2022) and algae (Lake 2000; Tsai et al., 2013; Townsend et al., 2017).
It is challenging to compare the relationship between the remaining FFM and bioassessment indices due to limitations in the flow-ecology literature. Specifically, few studies focus flow-ecology analysis directly on primary producers (Poff and Zimmerman 2010; Yarnell et al., 2020) and efforts to model season-specific metrics such as spring recession have only recently been undertaken (Yarnell et al., 2016). Nonetheless, recent studies provide insights into the FFM and index relationships identified in this study.
The selected FFM for evaluating biological condition using CSCI and ASCI scores describe duration of dry season (Dry_Dur_WS) as well as the timing and duration of spring recession flow (SP_Tim & SP_Dur), respectively. Steel et al. (2018) similarly found spring recession to have a high influence on macroinvertebrate community diversity possibly through increasing habitat heterogeneity which in turn increases species diversity and decreases water temperature, promoting algal productivity and macroinvertebrate diversity (Supplementary Table S1). Additionally, spring recession timing as well as seasonal predictability was found to be important for biological communities statewide across a range of stream types (Peek et al., this issue). In our study, the timing of the spring recession was consistently later in all subbasins, with the duration of the spring recession being both too long and too short. Spring duration is dependent on the timing of both the spring recession and the dry season, therefore a late spring recession, coupled with an early dry season may drive the short duration, with a late dry season driving the longer spring duration. This lack of seasonal predictability may be influencing biological alteration in our study area as many species are adapted to specific flow regimes and/or rely on timing cues for reproduction or developmental aspects of their life cycle (Poff and Ward 1989; Kennen et al., 2010; de la Fuente et al., 2018).
Dry season flows are known to maintain habitat availability for a broad range of aquatic species as well as support algal growth and primary productivity by, e.g., maintaining water temperature and dissolved oxygen (Supplementary Table S1). The duration of these low flows in our study area are consistently longer, which could explain the importance of this flow metric in augmented subbasins. The deviations from reference condition can alter hydraulic condition favoring certain types of algae, or creating unsuitable conditions for algal growth (Townsend and Padovan 2009) as well as favoring macroinvertebrate species less sensitive to changes in water quality (Leigh 2013). For example, low flows tend to favor filamentous algal mats due to increases in water temperature, nutrient concentration and reductions in velocity, and high flows can favor scour-tolerant diatoms (McIntire 1966; Dewson et al., 2007; Tang et al., 2013), which will ultimately impact algal community assemblages.
Fall pulse flows were of minimal importance in our study for both CSCI and ASCI, however Peek et al. (this issue) found a prominent influence statewide and has been found elsewhere to be important for benthic macroinvertebrates (Kennen et al., 2010; Yarnell et al., 2020) as functionally, fall pulse flows reactivate the system (Supplementary Table S1) after summer low flows. Our metrics describing fall pulse flow however, included multiple missing values (i.e., NA), which may be due to the hardcoded fall time window that the functional flow calculator uses (Patterson et al., 2020). Fall pulse flows, which represent the first flushing flows of the water year, may occur outside of the defined time window for fall flows, i.e., 1st October to 15th December (Patterson et al., 2020). This temporal mismatch may lead the calculator to discard genuine fall pulse flows that fall outside the specific time window.
4.4 Further Applications
We focused our study on prioritizing areas for restoration and flow management, however our process can be used for other applications such as deriving flow targets, assessing current flow alteration effects on biological condition and changes under different management and climate change scenarios (e.g., Buchanan et al., 2013; Cartwright et al., 2017; Stein et al., 2017; Kakouei et al., 2018; Mazor et al., 2018; Maloney et al., 2021). Although the data used in this study was specific to southern California, the data types are common to many other regions. The two primary sources of data needed to run the analysis are hydrologic model outputs and a bioassessment index. The CSCI and ASCI are specific to California, but Indices of Biotic Integrity (IBIs) are common. The approach described can be applied in any area where these two common data sources can be generated or compiled. The process used here is a great complement to established environmental flows frameworks such as the Ecological Limits of Hydrologic Alteration (Poff et al., 2010) where flow-ecology relationships are developed to assess the response of stream ecology, e.g., fish, vegetation and invertebrates, to the alteration of flow. The recently developed California Environmental Flow Framework (CEFF) is a generalized management approach for “determining ecosystem water needs that can be used to inform the development of environmental flow recommendations statewide” (Stein et al., 2021). The framework outlines guidance for identifying and developing ecological flow needs through flow-ecology analysis to ultimately provide environmental flow recommendations to aid management decisions. By providing a process to evaluate prioritization through flow-ecology analysis, our study has provided an important foundation for the application of CEFF. The next step in CEFF is to develop ecological flow needs that consider altered physical habitat including the evaluation of non-flow related influences (Stein et al., 2021). To implement flow management actions in the high priority areas, further investigation is needed the fully understand the source of the alteration. The direction of alteration in our study differed throughout the study area; therefore, implementation of management actions would need to be tailored to individual subbasins accordingly. For example, in cases where, e.g., magnitude of largest storm are augmented, flow could be managed through outflow diversions and/or retention areas with slow-release of storm flow. In areas where flows are depleted, management related to groundwater pumping or channel morphology could be implemented.
Application of CEFF involves function-based flow metrics, that account for ecological functions that occur across the entire active floodplain at a seasonal scale. Targets therefore would be considered based on the five functional flow components that relate to seasons, however implementation programs to meet these targets could be at daily, monthly or seasonal scales depending on the variability of the system and how finely the discharges can be managed. Taniguchi-Quan et al. (this issue), investigated the high priority subbasins identified from our study and illustrated the use of CEFF to develop ecological flow needs supportive of focal species of management concern.
4.5 Limitations
Our study focused on macroinvertebrate and algae response to altered flow. However, flow is not the only variable affecting the biological condition of streams. Local scale conditions such as water temperature, dissolved oxygen availability, nutrient concentration, substrate, and habitat complexity, also play a key role in the structure of biological communities. Many of these factors are strongly linked to flow, therefore it is possible that flow management and restoration efforts may be able to address multiple issues related to biological degradation. However, for flow management and restoration to be fully successful, it is important to consider the effects of other influencing factors at various spatial scales (King et al., 2016; Verdonschot et al., 2016). Such a task is complicated by interactive effects that modify the impact of confounding factors (Folt et al., 1999), such as habitat complexity dampening the strength of temperature effects (Scrine et al., 2017) or a combined effect of flow velocity and increased nutrient concentrations being smaller than the individual impact (Bækkelie et al., 2017).
5 Summary and Recommendations
Through this study, we have demonstrated the consequences of the various choices made during the development of flow-ecology analysis to aid management decisions. Relying on specific biological and probability thresholds can vastly change the level of biological alteration, as well as the importance of applying flow-ecology analysis on more than one biological group. Specific thresholds will vary in different regions however, we recommend applying our process to test for sensitivity and ensure discriminatory power in the study area. By applying our metric filtering process using the functional flow approach we ensured only the most relevant flow metrics were used, which help determine what component of the flow regime is mostly affecting stream biota. These considerations impact the prioritization of locations for flow management as well as which components of the flow regime to focus management actions.
Data Availability Statement
The datasets presented in this study can be found in online repositories. The names of the repository/repositories and accession number(s) can be found below: https://github.com/ksirving/SOC_Irving_et_al_data.
Author Contributions
KI led the preparation of this manuscript and data analysis. KT-Q and AH contributed to flow metric evaluation. RP contributed to coding and analysis. ES, KT-Q, KI, RM, ST contributed to analysis interpretation. ES, KT-Q, KI, RM, ST, AA, CR, GS contributed to the conceptualization of the project design. All authors contributed to writing this manuscript.
Funding
Funding for this work was provided by Orange County Public Works under Agreement #MA-080-18010919 and by the California Wildlife Conservation Board’s Stream Flow Enhancement Program (Proposition 1) under Grant Agreement #WC-1849AB.
Conflict of Interest
The authors declare that the research was conducted in the absence of any commercial or financial relationships that could be construed as a potential conflict of interest.
Publisher’s Note
All claims expressed in this article are solely those of the authors and do not necessarily represent those of their affiliated organizations, or those of the publisher, the editors and the reviewers. Any product that may be evaluated in this article, or claim that may be made by its manufacturer, is not guaranteed or endorsed by the publisher.
Acknowledgments
We would like to thank the members of the South OC Flow-Ecology Special Study Stakeholder and Technical Advisory Groups for valuable feedback on the overall project scope and technical approach and members of the California Environmental Flows Framework technical team for providing input and review of this manuscript. In addition, we would like to thank Aaron Poresky, Rich Wildman, and Jamie Feldman at Geosyntec Consultants for providing the flow data for SJHU. Liesl Tiefenthaler and Kristine Gesulga for helping with data and mapping.
Supplementary Material
The Supplementary Material for this article can be found online at: https://www.frontiersin.org/articles/10.3389/fenvs.2021.787462/full#supplementary-material
Abbreviations
ASCI, Algal Stream Condition Index; BRT, Boosted Regression Trees; CSCI, California Stream Condition Index; Delta H, Difference in FFM from reference to current conditions; FFA, Functional Flows Approach; FFM, Functional Flow Metric(s); GLM, Generalized Linear Model; HEC-HMS, Hydrologic Engineering Center Hydrologic Modeling System; LSPC, Loading Simulation Program in C++; SJHU, San Juan Hydrologic Unit; South OC WMA, South Orange County Watershed Management Area ; WQIP, Water Quality Improvement Plan.
Footnotes
2https://github.com/NoellePatterson/ffc-readme
3https://data-ocpw.opendata.arcgis.com/datasets/OCPW::outfall-locations-and-observations-combined/explore
4https://www.mrlc.gov/data/type/urban-imperviousness
References
Allan, J. D. (2004). Landscapes and Riverscapes: The Influence of Land Use on Stream Ecosystems. Annu. Rev. Ecol. Evol. Syst. 35, 257–284. doi:10.1146/annurev.ecolsys.35.120202.110122
Bækkelie, K. A. E., Schneider, S. C., Hagman, C. H. C., and Petrin, Z. (2017). Effects of Flow Events and Nutrient Addition on Stream Periphyton and Macroinvertebrates: An Experimental Study Using Flumes. Knowl. Manag. Aquat. Ecosyst. 418, 47. doi:10.1051/kmae/2017041
Beck, M. W., Mazor, R. D., Johnson, S., Wisenbaker, K., Westfall, J., Ode, P. R., et al. (2019a). Prioritizing Management Goals for Stream Biological Integrity within the Developed Landscape Context. Freshw. Sci. 38 (4), 883–898. doi:10.1086/705996
Beck, M. W., Mazor, R. D., Theroux, S., and Schiff, K. C. (2019b). The Stream Quality Index: A Multi-Indicator Tool for Enhancing Environmental Management. Environ. Sustainability Indicators 1-2 (August), 100004. doi:10.1016/j.indic.2019.100004
Bower, L. M., Peoples, B. K., Eddy, M. C., and Scott, M. C. (2022). Quantifying Flow-Ecology Relationships across Flow Regime Class and Ecoregions in South Carolina. Sci. Total Environ. 802, 149721. doi:10.1016/j.scitotenv.2021.149721
Buchanan, C., Moltz, H. L. N., Haywood, H. C., Palmer, J. B., and Griggs, A. N. (2013). A Test of the Ecological Limits of Hydrologic Alteration (ELOHA) Method for Determining Environmental Flows in the Potomac River Basin, U.S.A. Freshw. Biol. 58 (12), 2632–2647. doi:10.1111/fwb.12240
Bunn, S. E., and Arthington, A. H. (2002). Basic Principles and Ecological Consequences of Altered Flow Regimes for Aquatic Biodiversity. Environ. Manage. 30 (4), 492–507. doi:10.1007/s00267-002-2737-0
Carlisle, D. M., Grantham, T. E., Eng, K., and Wolock, D. M. (2017). Biological Relevance of Streamflow Metrics: Regional and National Perspectives. Freshw. Sci. 36 (4), 927–940. doi:10.1086/694913
Carlisle, D. M., Wolock, D. M., and Meador, M. R. (2011). Alteration of Streamflow Magnitudes and Potential Ecological Consequences: A Multiregional Assessment. Front. Ecol. Environ. 9 (5), 264–270. doi:10.1890/100053
Cartwright, J., Caldwell, C., Nebiker, S., and Knight, R. (2017). Putting Flow-Ecology Relationships into Practice: A Decision-Support System to Assess Fish Community Response to Water-Management Scenarios. Water 9 (3), 196. doi:10.3390/w9030196
Cheng, B., Xia, R., Zhang, Y., Yang, Z., Hu, S., Guo, F., et al. (2019). Characterization and Causes Analysis for Algae Blooms in Large River System. Sustain. Cities Soc. 51 (8), 101707. doi:10.1016/j.scs.2019.101707
Couto, T. B., and Olden, J. D. (2018). Global Proliferation of Small Hydropower Plants - Science and Policy. Front. Ecol. Environ. 16 (2), 91–100. doi:10.1002/fee.1746
Davies, P. M., Naiman, R. J., Warfe, D. M., Pettit, N. E., Arthington, A. H., and Bunn, S. E. (2014). Flow-Ecology Relationships: Closing the Loop on Effective Environmental Flows. Mar. Freshw. Res. 65 (2), 133–141. doi:10.1071/MF13110
de la Fuente, M., Bonada, N., Bêche, L., Dahm, C. N., Mendez, P. K., Tockner, K., et al. (2018). Evolutionary Responses of Aquatic Macroinvertebrates to Two Contrasting Flow Regimes. Hydrobiologia 808 (1), 353–370. doi:10.1007/s10750-017-3437-3
Dewson, Z. S., James, A. B. W., and Death, R. G. (2007). A Review of the Consequences of Decreased Flow for Instream Habitat and Macroinvertebrates. J. North Am. Benthological Soc. 26 (3), 401–415. doi:10.1899/06-110.1
Elith, J., Leathwick, J. R., and Hastie, T. (2008). A Working Guide to Boosted Regression Trees. J. Anim. Ecol. 77 (4), 802–813. doi:10.1111/j.1365-2656.2008.01390.x
Fletcher, T. D., Andrieu, H., and Hamel, P. (2013). Understanding, Management and Modelling of Urban Hydrology and its Consequences for Receiving Waters: A State of the Art. Adv. Water Resour. 51, 261–279. doi:10.1016/j.advwatres.2012.09.001
Folt, C. L., Chen, C. Y., Moore, M. V., and Burnaford, J. (1999). Synergism and Antagonism Among Multiple Stressors. Limnol. Oceanogr. 44 (3part2), 864–877. doi:10.4319/lo.1999.44.3_part_2.0864
Friedman, J. H. (2001). Greedy Function Approximation: A Gradient Boosting Machine. Ann. Statist. 29 (5), 1189–1232. doi:10.1214/aos/1013203451
Hawley, R. J., and Bledsoe, B. P. (2011). How Do Flow Peaks and Durations Change in Suburbanizing Semi-arid Watersheds? A Southern California Case Study. J. Hydrol. 405 (1–2), 69–82. doi:10.1016/j.jhydrol.2011.05.011
Hijmans, R. J., Phillips, S., Leathwick, J., and Elith, J. (2020). Dismo: Species Distribution Modeling. R Package Version 1.3-3. Available at: https://cran.r-project.org/package=dismo.
Irving, K., Jähnig, S. C., and Kuemmerlen, M. (2020). Identifying and Applying an Optimum Set of Environmental Variables in Species Distribution Models. Inland Waters 10 (1), 11–28. doi:10.1080/20442041.2019.1653111
Kahle, D., and Wickham, H. (2013). ggmap: Spatial Visualization with ggplot2. The R Journal 5 (1), 144–161. doi:10.1002/ece3.3907
Kakouei, K., Kiesel, J., Domisch, S., Irving, K. S., Jähnig, S. C., and Kail, J. (2018). Projected Effects of Climate-Change-Induced Flow Alterations on Stream Macroinvertebrate Abundances. Ecol. Evol. 8 (6), 3393–3409. doi:10.1002/ece3.3907
Kennen, J. G., Riva-Murray, K., and Beaulieu, K. M. (2009). Determining Hydrologic Factors that Influence Stream Macroinvertebrate Assemblages in the Northeastern US. Ecohydrol. 3 (1), a–n. doi:10.1002/eco.99
King, A. J., Gwinn, D. C., Tonkin, Z., Mahoney, J., Raymond, S., and Beesley, L. (2016). Using Abiotic Drivers of Fish Spawning to Inform Environmental Flow Management. J. Appl. Ecol. 53 (1), 34–43. doi:10.1111/1365-2664.12542
Kirkwood, A. E., Jackson, L. J., and McCauley, E. (2009). Are Dams Hotspots forDidymosphenia Geminatablooms. Freshw. Biol. 54 (9), 1856–1863. doi:10.1111/j.1365-2427.2009.02231.x
Konrad, C. P., Brasher, A. M. D., and May, J. T. (2008). Assessing Streamflow Characteristics as Limiting Factors on Benthic Invertebrate Assemblages in Streams across the Western United States. Freshw. Biol. 53 (10), 1983–1998. doi:10.1111/j.1365-2427.2008.02024.x
Lake, P. S. (2000). Disturbance, Patchiness, and Diversity in Streams. J. North Am. Benthological Soc. 19 (4), 573–592. doi:10.2307/1468118
Lange, K., Townsend, C. R., and Matthaei, C. D. (2016). A Trait‐based Framework for Stream Algal Communities. Ecol. Evol. 6 (1), 23–36. doi:10.1002/ece3.1822
Lehner, B., Liermann, C. R., Revenga, C., Vörösmarty, C., Fekete, B., Crouzet, P., et al. (2011). High‐resolution Mapping of the World's Reservoirs and Dams for Sustainable River‐flow Management. Front. Ecol. Environ. 9 (9), 494–502. doi:10.1890/100125
Leigh, C. (2013). Dry-Season Changes in Macroinvertebrate Assemblages of Highly Seasonal Rivers: Responses to Low Flow, No Flow and Antecedent Hydrology. Hydrobiologia 703 (1), 95–112. doi:10.1007/s10750-012-1347-y
Loflen, C., and Fetscher, A. E. (2020). San Diego Regional Water Quality Control Board Biological Objectives for the San Diego Region. San Diego, CA: California Environmental Protection Agency.
Maloney, K. O., Carlisle, D. M., Buchanan, C., Rapp, J. L., Austin, S. H., Cashman, M. J., et al. (2021). Linking Altered Flow Regimes to Biological Condition: An Example Using Benthic Macroinvertebrates in Small Streams of the Chesapeake Bay Watershed. Environ. Manage. 67 (6), 1171–1185. doi:10.1007/s00267-021-01450-5
Mazor, R. D., May, J. T., Sengupta, A., McCune, K. S., Bledsoe, B. P., and Stein, E. D. (2018). Tools for Managing Hydrologic Alteration on a Regional Scale: Setting Targets to Protect Stream Health. Freshw. Biol. 63 (8), 786–803. doi:10.1111/fwb.13062
Mazor, R. D., Rehn, A. C., Ode, P. R., Engeln, M., Schiff, K. C., Stein, E. D., et al. (2016). Bioassessment in Complex Environments: Designing an Index for Consistent Meaning in Different Settings. Freshw. Sci. 35 (1), 249–271. doi:10.1086/684130
McIntire, C. D. (1966). Some Effects of Current Velocity on Periphyton Communities in Laboratory Streams. Hydrobiologia 27 (3), 559–570. doi:10.1007/BF00042713
Miller, M. P., McKnight, D. M., Cullis, J. D., Greene, A., Vietti, K., and Liptzin, D. (2009). Factors Controlling Streambed Coverage of Didymosphenia Geminata in Two Regulated Streams in the Colorado Front Range. Hydrobiologia 630 (1), 207–218. doi:10.1007/s10750-009-9793-x
Nilsson, C., and Renöfält, B. M. (2008). Linking Flow Regime and Water Quality in Rivers: A Challenge to Adaptive Catchment Management. Ecol. Soc. 13 (2), 18. http://www.ecologyandsociety. doi:10.5751/es-02588-130218
Ode, P. R., Fetscher, A. E., and Busse, L. B. (2016). Standard Operating Procedures (SOP) for the Collection of Field Data for Bioassessments of California Wadeable Streams: Benthic Macroinvertebrates, Algae, and Physical Habita. Technical Report 835. State Water Resources Control Board Surface Water Ambient Monitoring Program: Sacramento, CA.
Patterson, N. K., Lane, B. A., Sandoval-Solis, S., Pasternack, G. B., Yarnell, S. M., and Qiu, Y. (2020). A Hydrologic Feature Detection Algorithm to Quantify Seasonal Components of Flow Regimes. J. Hydrol. 585 (August 2019), 124787. doi:10.1016/j.jhydrol.2020.124787
Pebesma, E. (2018). Simple Features for R: Standardized Support for Spatial Vector Data. The R Journal 10 (1), 439–446. doi:10.32614/RJ-2018-009
Peek, R., Irving, K., Yarnell, S. M., Lusardi, R., Stein, E. D., and Mazor, R. (2022). Identifying Functional Flow Linkages Between Stream Alteration and Biological Stream Condition Indices Across California. Front. environ. sci.. doi:10.3389/fenvs.2021.790667
Poff, N. L., Allan, J. D., Bain, M. B., Karr, J. R., Prestegaard, K. L., Richter, B. D., et al. (1997). The Natural Flow Regime. BioScience 47 (11), 769–784. doi:10.2307/1313099
Poff, N. L., Olden, J. D., Merritt, D. M., and Pepin, D. M. (2007). Homogenization of Regional River Dynamics by Dams and Global Biodiversity Implications. Proc. Natl. Acad. Sci. 104 (14), 5732–5737. doi:10.1073/pnas.0609812104
Poff, N. L., Richter, B. D., Arthington, A. H., Bunn, S. E., Naiman, R. J., Kendy, E., et al. (2010). The Ecological Limits of Hydrologic Alteration (ELOHA): A New Framework for Developing Regional Environmental Flow Standards. Freshw. Biol. 55 (1), 147–170. doi:10.1111/j.1365-2427.2009.02204.x
Poff, N. L., and Ward, J. V. (1989). Implications of Streamflow Variability and Predictability for Lotic Community Structure: A Regional Analysis of Streamflow Patterns. Can. J. Fish. Aquat. Sci. 46 (10), 1805–1818. doi:10.1139/f89-228
Poff, N. L., and Zimmerman, J. K. H. (2010). Ecological Responses to Altered Flow Regimes: A Literature Review to Inform the Science and Management of Environmental Flows. Freshw. Biol. 55 (1), 194–205. doi:10.1111/j.1365-2427.2009.02272.x
R Core Team (2020). R: A Language and Environment for Statistical Computing. Vienna, Austria: R Foundation for Statistical Computing. Available at: https://www.R-project.org/.
Rehn, A. C. (2009). Benthic Macroinvertebrates as Indicators of Biological Condition below Hydropower Dams on West Slope Sierra Nevada Streams, California, USA. River Res. Applic. 25 (2), 208–228. doi:10.1002/rra.1121
Schneider, S. C., and Petrin, Z. (2017). Effects of Flow Regime on Benthic Algae and Macroinvertebrates - A Comparison between Regulated and Unregulated Rivers. Sci. Total Environ. 579, 1059–1072. doi:10.1016/j.scitotenv.2016.11.060
Scrine, J., Jochum, M., Ólafsson, J. S., and O'Gorman, E. J. (2017). Interactive Effects of Temperature and Habitat Complexity on Freshwater Communities. Ecol. Evol. 7 (22), 9333–9346. doi:10.1002/ece3.3412
Sengupta, A., Adams, S. K., Bledsoe, B. P., Stein, E. D., McCune, K. S., and Mazor, R. D. (2018). Tools for Managing Hydrologic Alteration on a Regional Scale: Estimating Changes in Flow Characteristics at Ungauged Sites. Freshw. Biol. 63 (8), 769–785. doi:10.1111/fwb.13074
Stancheva, R., Busse, L., Kociolek, J. P., and Sheath, R. (2015). Standard Operating Procedures for Laboratory Processing and Identification of Stream Algae in California. Report No. SWAMP-SOP-2015-0003.
Stancheva, R., and Sheath, R. G. (2016). Benthic Soft-Bodied Algae as Bioindicators of Stream Water Quality. Knowl. Manag. Aquat. 417 (15). doi:10.1051/kmae/2016002
Steel, A. E., Peek, R. A., Lusardi, R. A., and Yarnell, S. M. (2018). Associating Metrics of Hydrologic Variability with Benthic Macroinvertebrate Communities in Regulated and Unregulated Snowmelt-Dominated Rivers. Freshw. Biol. 63 (8), 844–858. doi:10.1111/fwb.12994
Stein, E. D., Sengupta, A., Mazor, R. D., McCune, K., Bledsoe, B. P., and Adams, S. (2017). Application of Regional Flow-Ecology Relationships to Inform Watershed Management Decisions: Application of the ELOHA Framework in the San Diego River Watershed, California, USA. Ecohydrology 10 (7), e1869–16. doi:10.1002/eco.1869
Stein, E. D., Zimmerman, J., Yarnell, S. M., Stanford, B., Lane, B., Taniguchi-Quan, K. T., et al. (2021). The California Environmental Flows Framework: Meeting the Challenges of Developing a Large-Scale Environmental Flows Program. Front. environ. sci. 9. doi:10.3389/fenvs.2021.769943
Stevenson, R. J., Pan, Y., and van Dam, H. (2010). “Assessing Environmental Conditions in Rivers and Streams with Diatoms,” in The Diatoms: Applications for the Environmental and Earth Sciences. Editors E. F. Stoermer, and J. P. Smol. (Cambridge: Cambridge University Press), 57–85. doi:10.1017/CBO9780511763175.005
Suren, A. M., Biggs, B. J. F., Kilroy, C., and Bergey, L. (2003). Benthic Community Dynamics during Summer Low‐flows in two rivers of Contrasting Enrichment 1. Periphyton. New Zealand J. Mar. Freshw. Res. 37 (1), 53–70. doi:10.1080/00288330.2003.9517146
Tang, T., Niu, S. Q., and Dudgeon, D. (2013). Responses of Epibenthic Algal Assemblages to Water Abstraction in Hong Kong Streams. Hydrobiologia 703 (1), 225–237. doi:10.1007/s10750-012-1362-z
Theroux, S., Mazor, R. D., Beck, M. W., Ode, P. R., Stein, E. D., and Sutula, M. (2020). Predictive Biological Indices for Algae Populations in Diverse Stream Environments. Ecol. Indicators 119 (January), 106421. doi:10.1016/j.ecolind.2020.106421
Tonkin, J. D., Merritt, D. M., Olden, J. D., Reynolds, L. V., and Lytle, D. A. (2018). Flow Regime Alteration Degrades Ecological Networks in Riparian Ecosystems. Nat. Ecol. Evol. 2 (1), 86–93. doi:10.1038/s41559-017-0379-0
Tonkin, J. D., Olden, J. D., Merritt, D. M., Reynolds, L. V., Rogosch, J. S., and Lytle, D. A. (2021). Designing Flow Regimes to Support Entire River Ecosystems. Front. Ecol. Environ. 19 (6), 326–333. doi:10.1002/fee.2348
Tornés, E., and Sabater, S. (2010). Variable Discharge Alters Habitat Suitability for Benthic Algae and Cyanobacteria in a Forested Mediterranean Stream. Mar. Freshwater Res. 61, 441–450. doi:10.1071/MF09095
Townsend, S. A., and Padovan, A. V. (2009). A Model to Predict the Response of the Benthic macroalgaSpirogyrato Reduced Base Flow in the Tropical Australia. River Res. Applic. 25 (9), 1193–1203. doi:10.1002/rra.1230
Townsend, S., Schult, J., Douglas, M., and Lautenschlager, A. (2017). Recovery of Benthic Primary Producers from Flood Disturbance and its Implications for an Altered Flow Regime in a Tropical Savannah River (Australia). Aquat. Bot. 136, 9–20. doi:10.1016/j.aquabot.2016.08.004
Tsai, J.-W., Chuang, Y.-L., Wu, Z.-Y., Kuo, M.-H., and Lin, H.-J. (2014). The Effects of Storm-Induced Events on the Seasonal Dynamics of Epilithic Algal Biomass in Subtropical Mountain Streams. Mar. Freshw. Res. 65 (1), 25–38. doi:10.1071/MF13058
Verdonschot, R. C. M., Kail, J., McKie, B. G., and Verdonschot, P. F. M. (2016). The Role of Benthic Microhabitats in Determining the Effects of Hydromorphological River Restoration on Macroinvertebrates. Hydrobiologia 769 (1), 55–66. doi:10.1007/s10750-015-2575-8
White, M. D., and Greer, K. A. (2006). The Effects of Watershed Urbanization on the Stream Hydrology and Riparian Vegetation of Los Peñasquitos Creek, California. Landscape Urban Plann. 74 (2), 125–138. doi:10.1016/j.landurbplan.2004.11.015
Wohl, E., Lane, S. N., and Wilcox, A. C. (2015). The Science and Practice of River Restoration. Water Resour. Res. 51 (8), 5974–5997. doi:10.1002/2014WR016874
Woodard, M., Slusark, J., and Ode, P. (2012). Standard Operating Procedures For Laboratory Processing And Identification Of Benthic Macroinvertebrates In California. Report No: Bioassessment SOP 0003.
Yarnell, S. M., Petts, G. E., Schmidt, J. C., Whipple, A. A., Beller, E. E., Dahm, C. N., et al. (2015). Functional Flows in Modified Riverscapes: Hydrographs, Habitats and Opportunities. BioScience 65 (10), 963–972. doi:10.1093/biosci/biv102
Yarnell, S. M., Stein, E. D., Webb, J. A., Grantham, T., Lusardi, R. A., Zimmerman, J., et al. (2020). A Functional Flows Approach to Selecting Ecologically Relevant Flow Metrics for Environmental Flow Applications. River Res. Applic 36 (2), 318–324. doi:10.1002/rra.3575
Yarnell, S. M., Viers, J. H., and Mount, J. F. (2010). Ecology and Management of the Spring Snowmelt Recession. BioScience 60 (2), 114–127. doi:10.1525/bio.2010.60.2.6
Yarnell, S., Peek, R., Epke, G., and Lind, A. (2016). Management of the Spring Snowmelt Recession in Regulated Systems. J. Am. Water Resour. Assoc. 52 (3), 723–736. doi:10.1111/1752-1688.12424
Keywords: flow-ecology, prioritization, benthic macroinvertebrates, algae, flow alteration, functional flows, urban streams, flow management
Citation: Irving K, Taniguchi-Quan KT, Aprahamian A, Rivers C, Sharp G, Mazor RD, Theroux S, Holt A, Peek R and Stein ED (2022) Application of Flow-Ecology Analysis to Inform Prioritization for Stream Restoration and Management Actions. Front. Environ. Sci. 9:787462. doi: 10.3389/fenvs.2021.787462
Received: 30 September 2021; Accepted: 21 December 2021;
Published: 08 February 2022.
Edited by:
Teresa Ferreira, University of Lisbon, PortugalReviewed by:
Alban Kuriqi, Universidade de Lisboa, PortugalJohn Tyler Fox, University of Arkansas, United States
Copyright © 2022 Irving, Taniguchi-Quan, Aprahamian, Rivers, Sharp, Mazor, Theroux, Holt, Peek and Stein. This is an open-access article distributed under the terms of the Creative Commons Attribution License (CC BY). The use, distribution or reproduction in other forums is permitted, provided the original author(s) and the copyright owner(s) are credited and that the original publication in this journal is cited, in accordance with accepted academic practice. No use, distribution or reproduction is permitted which does not comply with these terms.
*Correspondence: Katie Irving, katiei@sccwrp.org