- 1Institute of Environment and Sustainable Development in Agriculture, Chinese Academy of Agricultural Sciences, Beijing, China
- 2College of Forestry, Northwest A&F University, Xianyang, China
- 3State Key Laboratory of Soil Erosion and Dryland Farming on the Loess Plateau, Institute of Soil and Water Conservation, Northwest A&F University, Xianyang, China
- 4College of Environment Science and Engineering, Hebei University of Science and Technology, Hebei, China
In this study, the influence of ciprofloxacin, chlorotetracycline, lincomycin, and sulfamethoxazole on the composition of the bacterial community structure was studied during aerobic composting with swine manure. Firmicutes (26.67%) and Chloroflexi (23.33%) were the most widely distributed phyla. Under all antibiotic treatments, the relative abundances of Bacillaceae, Streptosporangiaceae, Limnochordaceae, and Peptostreptococcaceae increased during the composting process. Moreover, norank_SBR1031, Planococcaceae, Thermomonosporaceae, Peptostreptococcaceae, Erysipelotrichaceae, Limnochordaceae, and Clostridiaceae_1 were the families showing the most significant differences across all treatments (p < 0.05). Principal co-ordinates analysis indicated that the family composition in the ciprofloxacin treatment significantly differed from the other treatments. The presence of ciprofloxacin increased both the abundance and diversity of the bacterial community (the Chao index changed from 588.44 to 680.17, and the Shannon index changed from 3.41 to 4.06) in the end of composting. Crocinitomicaceae dominated (relative abundance of 79.10%) among the unique families in the ciprofloxacin treatment. Network analysis indicated that ciprofloxacin altered the synergistic or competitive relationships between different families (norank_SBR1031 and Microscillaceae), leading to different bacterial community composition compared with other treatments. Further, a structural equation model showed that the C:N ratio was significantly negatively correlated with the bacterial community (λ = −0.869, p < 0.01), whereas pH showed a direct, significant positive relationship with the bacterial community (λ = 0.701, p < 0.01), especially in ciprofloxacin treatment. Overall, ciprofloxacin significantly influenced the physical and chemical properties of composting, altered the bacterial community structure. These findings have important implications for a better understanding of the effects of antibiotic types on bacterial community structure and the involved mechanisms during swine manure composting.
Introduction
Antibiotics play a key role globally in the management of infectious diseases in humans, their companion animals, livestock, and the aquaculture industry. Antibiotics are being produced, consumed, and released into the environment on an unprecedented scale, and their residues can adversely affect aquatic and terrestrial ecosystems (Monteiro and Boxall, 2010; Brandt et al., 2015). Economic projections using global data have estimated that the use of antibiotics in food animal production would increase by 67% (from 63,151 ± 1,560 to 105,596 ± 3,605 tons) for the period 2010–2030 (Van Boeckel et al., 2015). Previous studies found that only a small amount of antibiotics used for animals were involved in metabolism by the body and actually utilized, with the vast majority of antibiotics incompletely absorbed and degraded. About 30–90% of administered antibiotics are eliminated from animals in the form of a bioactive drug along with their feces and urine (Alvarez et al., 2010; Zhao et al., 2010; Chen Z. et al., 2018; Ren et al., 2018). Due to their lower metabolism, many veterinary antibiotics, especially the tetracyclines, macrolides, fluoroquinolones, and sulfonamides, would persist in animal manure (Kuppusamy et al., 2018; Song et al., 2020; Cheng et al., 2021). The residual concentration of lincomycin (LIN) and ciprofloxacin (CIP) was 3,800 and 33,980 μg/kg (Zhao et al., 2010; Pan and Chu, 2017); for sulfamethoxazole (SMX) and chlorotetracycline (CTC), it was 89,000 and 139,400 μg/kg in swine manure, respectively (Chen et al., 2012; Hou et al., 2015). Composting is an environmentally and economically sound way to dispose of livestock and poultry feces (Awasthi et al., 2019; Liu et al., 2020). Many studies confirmed that those residual antibiotics present in livestock manure could be effectively removed via composting (Liu et al., 2020; Cheng et al., 2021).
Composting is the biodegradation of solids by microorganisms under aerobic conditions. The structure and function of the microbial community forms a dynamic core, which drives the decomposition and mineralization of various substances. The microorganism is the main functional factor in the process of composting (Zhong et al., 2018; Wang et al., 2020). Traditional methods of studying composting microorganisms rely on plate colony counting. For example, recently Wang L. et al. (2019) concluded that the total concentrations of culturable bacteria was 2.00 × 108 CFU/g, and the abundance of enrofloxacin, doxycycline, sulfadimidine, and tylosin-resistant bacteria was 1.33 × 106–2.67×107, 3.90 × 107–8.77×107, 1.08 × 108–1.64×108, and 4.80 × 107–1.27 × 108 CFU/g, respectively. In addition, the plate method has been implemented successfully to identify antibiotic-resistant bacteria (ARB) (Wang L. et al., 2019; Staley et al., 2020); these genera, namely Bacillus (21.05%), Enterococcus (20.00%), Sporosarcina (19.00%), Kurthia (14.28%), and Psychrobacter (14.28%) were found resistant to enrofloxacin, sulfadimidine, doxycycline, and tylosin, respectively (Wang L. et al., 2019). Generally, Enterococcus, Escherichia, Acinetobacter, Alcaligenes, Myroides, and Providencia were the most common culturable multiple ARB (Yang et al., 2017).
Moreover, techniques of modern molecular biology research are gaining more attention and traction in microbial ecology research, having now been applied to numerous studies of microbial changes during the composting process (Nakasaki et al., 2009; Knight et al., 2018). Previously, Tran et al. (2021) made use of food waste, fresh mature compost, and sawdust for composting, concluded that Lactobacillus sp. (0.4) was the most abundant by the end of the composting (i.e., on day 35). Amoxicillin’s impact on bacterial community succession was also evaluated during aerobic composting by Liu et al. (2019); they concluded that this antibiotic increased the relative abundance of Proteobacteria by 1.90–24.80%, and also inhibited the growth and reproduction of Bacteroidetes and Firmicutes, with reductions of 0–7.76% and 17.80–26.10%, respectively. Other research has shown that Chloroflexi and Proteobacteria were the major phyla found in cattle and sheep manure composts, while Firmicutes dominated the chicken and pig manure composts. Hence, the feeding habit of livestock can have predictable effects on microbial community composition during the composting of their manure (Wan et al., 2021). On day 2 of composting, the abundance of Ammoniibacillus and Thermobifida was enhanced by 1.4 and 1.8 times, respectively, in Tly25 (tylosin, 25), whereas Thermobacillus, Sinibacillus, and Symbiobacterium were enriched in Tly50, but Candidatus_Chlorothrix and Bacillus are most enriched in Tly75 (Zhang et al., 2018).
Numerous studies also provided much evidence that bacterial communities are strongly affected by residual antibiotics (Ren et al., 2018; Wang L. et al., 2019; Liu et al., 2019). Yet, surprisingly few studies have focused on the comparative effects of residual antibiotic types upon bacterial community succession during aerobic composting of manure. Our previous work indicated that ciprofloxacin (CIP) impacted the bacterial community differently than that of chlorotetracycline (CTC), lincomycin (LIN), or sulfamethoxazole (SMX) during aerobic composting with swine manure (Song et al., 2020). Accordingly, conducting a detailed analysis of the bacterial community structure, as well as elucidating the specific reasons driving the variation, is urgent. To this end, the effects of various antibiotics on alpha diversity and the composition of bacterial community structure were studied here. The bacterial competitive and synergistic correlations of different treatments were explored. We also evaluated major physical and chemical properties possibly influencing how bacteria changes at the community level. This study’s results provide a theoretical basis for how antibiotic types could modify bacterial community structure and clarify the prominent physical and chemical properties affecting it.
Materials and Methods
Design of Composting Experiment and Sample Collection
The composting materials included pig manure, coconut shell powder, and corn stalks. The specific experimental design is already described in our previous study (Song et al., 2020). Briefly, the composting experiment was carried out in foam tanks, each having dimension of 55 cm × 45 cm × 45 cm. The ratio of total carbon (TC) to total nitrogen (TN) [C:N] was maintained at 30:1, and moisture content was about 65%, both being favorable for the aerobic composting (Zhao et al., 2020). After running some preliminary calculations, the total weight of raw material was 14.28 kg (ratio of corn straw: coconut shell powder: pig manure was 4.94: 2.67: 6.67 [in kg]). Using this ratio was suitable for performing the composting in the rectangular foam box once the moisture content was appropriately adjusted in the experiment. A treatment without any antibiotic applied served as the control (T0). The other five treatments consisted of added CTC (50 mg/kg), SMX (50 mg/kg), LIN (50 mg/kg), and CIP (50 mg/kg), as well as the four antibiotics added (CIP + CTC + SMX + LIN, 50 mg/kg), these respectively named T1, T2, T3, T4, and T5. All treatments were replicated for three times.
To ensure the composting temperature met the national standard (GBNY525-2012), the temperature of the pile was monitored daily. The composting material was turned over every 6 days, and the whole composting process lasted 35 days. At the end of composting, the upper, middle, and lower layers of the reactor were sampled using the five-point sampling method, and mixed evenly forming a composite sample per layer (per reactor). Then, 250 g of samples were collected. One part was used for measurement of physical and chemical properties (pH, moisture content, electrical conductivity (EC), TC, and TN). The pH and EC were measured by pH meter and conductivity Meter. The moisture content was calculated from oven-drying method. TN was measured by Kjeldahl method. TC was determined by the oil bath method. The final physical and chemical properties were described in previous studies (Song et al., 2020). The remaining samples were stored in a refrigerator at –80°C for the later determination of bacterial community structure and diversity.
Extraction of DNA and High-Throughput Sequencing of 16S rRNA Region
Total DNA was extracted from each sample using the TIANamp Stool DNA Kit (TIANGEN BIOTECH, USA), accord ing to the manufacturing instructions. DNA concentration was determined by Nano Drop one (Thermo, USA). DNA quality was checked on 1% agarose gel.
The PCR amplification of the V3-V4 variable region of 16S rRNA gene was performed using the primer pair 338F (5′-ACTCCTACGGGAGCAGCAG-3′) and 806R (5′-GGACTACHVGGGTWTCTAAT-3′) (Ren et al., 2019). PCR products from the same sample were mixed and recovered using 2% agarose gel. The extracted product was purified with the AxyPrep DNA Gel Extraction Kit (Axygen Biosciences, USA), and detected on 2% agarose gel. The recovered products were then quantified using a Quantus™ Fluorometer (Promega, USA). The NextFlex Rapid DNA-Seq Kit was used to build the sequence library: this entailed 1) a connector link; 2) the use of magnetic bead screening to remove the joint self-connecting segment; 3) enrichment of library templates by PCR amplification; and 4) PCR products’ recovery by magnetic beads, to yield the final library.
Sequencing was performed using Illumina’s Mi-Seq PE300 platform. The original sequences were qualitatively controlled using fastp software (https://github.com/OpenGene/fastp, v0.20.0) (Chen S. et al., 2018), and FLASH software was used for their splicing (http://www.cbcb.umd.edu/software/flash, version 1.2.7) (Magoc and Salzberg, 2011). Those reads smaller than 50 bp in length after quality control processing were filtered out, and any containing N bases were also removed. According to the overlap relationship between PE (paired-end) reads, pairs of reads were merged into a sequence, whose minimum overlap length was 10 bp. The allowable maximum error ratio of overlap area of a given stitching sequence was set to 0.2, and the non-conforming sequence was screened. Samples were distinguished according to their respective barcode and primers at both ends of the sequence, and the sequence direction was adjusted accordingly. The number of mismatches allowed by barcode was 0, and the maximum number of primer mismatches was 2. The UPARSE tool (http://drive5.com/uparse/, v7.1) was used to carry out the OTU (operational taxonomic unit) clustering based on 97% similarity and to eliminate chimeras (Edgar, 2013). The RDP (Ribosomal Database Project) Classifier (http://rdp.cme.msu.edu/, v2.2) was used to annotate each sequence (Wang et al., 2007).
Alpha Diversity Analysis
Alpha diversity is the term for species diversity within the habitat and conveys the degree of diversity at the different classification levels in a given sample. Mothur software (v.1.30.1 http://www.mothur.org/wiki/Schloss_SOP#Alpha_diversity) was used to perform a rarefication analysis, through which the Chao, Shannon, Simpson and Coverage indexes were obtained. The Chao index was used to estimate the number of OTUs contained in a sample, using the Chao1 algorithm, to describe the abundance of the microbial community. The higher the Chao index is, the higher the microbial community abundance is Eq. 1. The Shannon index was one of the microbial diversity indexes calculated for the samples; the higher Shannon value is, the higher microbial community diversity is Eq. 2. The Simpson index is another well-known one, also used here to derive a measure of microbial diversity for each sample; the higher the Simpson value is, the lower the microbial community diversity is Eq. 3. Finally, coverage index can be used to gauge whether the sequencing results represented the real conditions of microbial community in a given treatment Eq. 4. The corresponding formulas are as follows:
where Schao1 is estimated OTU number; Sobs is the number of OTUs actually observed; n1 is the number of OTUs containing only one sequence (i.e., the “singletons”); n2 is the number of OTUs consisting of only two sequences (i.e., the “Doubletons”); ni is the number of sequences contained in the ith OTU; finally, N is equal to the number of sequences.
where ni is the number of OTUs containing only one sequence, and N is the total number of sequences present in the sample.
Data Analysis
The Venn, pie, heatmap, and PcoA (principal co-ordinates analysis) results were all derived and visualized using the packages “Venn Diagram,” “Pie,” “Vegan,” and “PCoA” for R v3.5.2 (https://www.r-project.org/), respectively. One-way ANOVA (analysis of variance) was implemented using in SPSS v23.0 Statistics (IBM, Chicago, IL, USA). Tests for significant differences between species groups were made using the free online platform of Majorbio Cloud Platform (www.majorbio.com). The bacterial network was built, based on a Spearman’s correlation analysis (p < 0.05), using Networkx software (http://networkx.github.io/). A structural equation model (SEM) was developed using Smart PLS-3.3.3 (https://www.smartpls.com/).
Results and Discussion
Alpha Diversity of Bacterial Community
In this study, alpha diversity of bacterial communities was analyzed at the 97% OTU similarity threshold level. Coverage index values of all treatments were >0.995, indicating that the gene sequences had a high probability of being detected in the composting samples; this also confirmed that the sequencing results could reflect the real bacterial community in the composting process (Tran et al., 2021; Tian et al., 2013). Chao index of the six treatments ranged from 588.44 to 680.17, ranked as T4 > T1 > T3 > T2 > T5 > T0, indicating that each antibiotic treatment had increased the abundance of the bacterial community by the end of composting (Figure 1A). The Shannon index was also the highest in T4 (4.06), whose Simpson index was the lowest (0.07), strongly indicating that bacterial community diversity in T4 treatment surpassed that in the other treatments. In a recent study, the Chao and Shannon indexes increased in response to CIP (10 mg/kg) and other antibiotic treatments after 42 days (end) of composting, with CIP’s influence on bacterial abundance and diversity significantly greater than that of oxytetracycline and sulfadiazine (Liu et al., 2020). Other studies showed that adding antibiotics to composting significantly increased its Shannon index, likely because antibiotics induced the replication of significant portion of the dominant ARB already present, these being found positively correlated with the antibiotic concentration used (Gao et al., 2012; Liu et al., 2012). Sulfadiazine and CTC were completely within 3 and 21 days, respectively, while 1.17 mg/kg of CIP was extracted after 56 days of composting (Selvam et al., 2012). In addition, ciprofloxacin belongs to quinolone antibiotics. The mutations generated by the bacteria against quinolone antibiotics are generally located on the target enzyme binding sites in topoisomerase IV and DNA gyrase. Resistance to quinolone antibiotics can be obtained by acquisition of a resistant plasmid from other sources in the environment through horizontal transfer, resulting to the rapid spread of resistance (Pham et al., 2019). Such findings coupled those of our study suggested that CIP was more capable of favoring the production of ARB than other antibiotics.
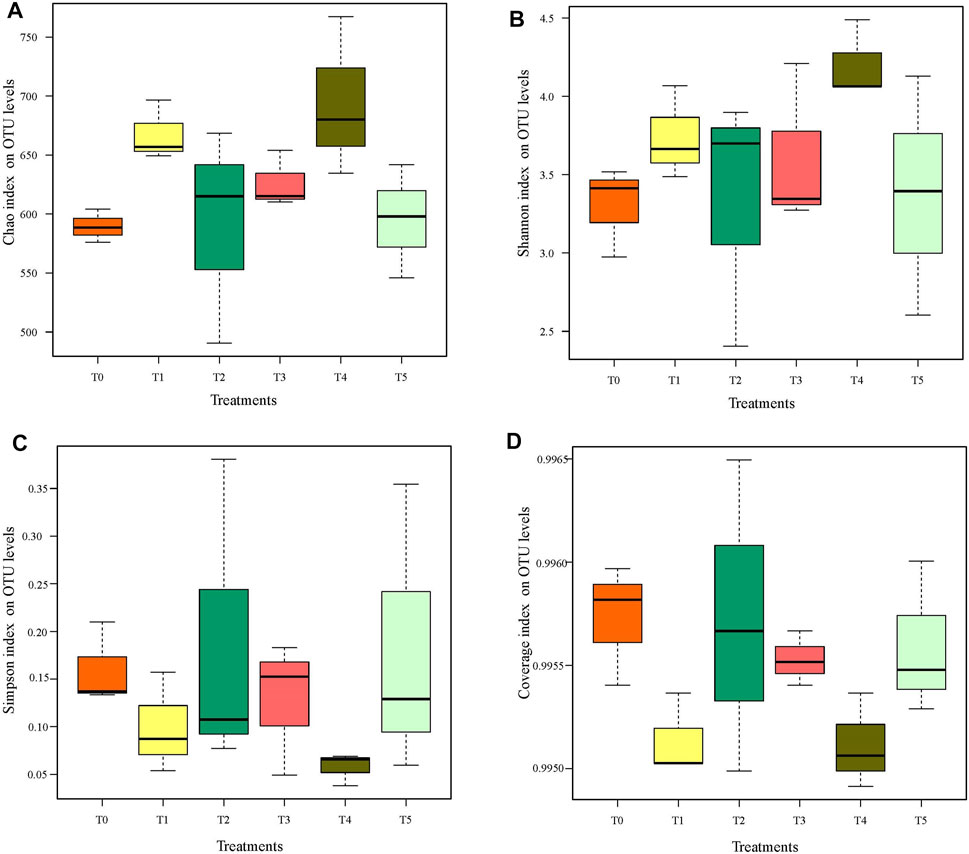
FIGURE 1. Boxplots showing the influence of antibiotic types (T0 to T5) on the alpha diversity of the bacterial community in swine manure composting: (A) the Chao index, (B) the Shannon index, (C) the Simpson index, and (D) the coverage index. T0: the control, T1: CTC added, T2: SMX added, T3: LIN added, T4: CIP added, T5: four antibiotics (CIP + CTC + SMX + LIN) added, the same below. Differences among Bacterial Communities at the OTU Level and Changes in Relative Abundance of their Members at the Family Level.
While the Chao index was lowest in T0, its Shannon index (3.41) was higher than that of either under T3 (3.35) and T5 (3.39) (Figure 1B). This revealed that LIN and the multiple-antibiotic treatments could reduce the bacterial community diversity. LIN was the main reason for the lower Shannon index of T5. In addition, CTC, SMX, and CIP were able to increase not only the abundance of bacterial community but also its diversity. These results also implicate a stronger ability of these three antibiotics (CTC, SMX, and CIP) than LIN to induce dominant ARB. When compared with T1 or T3, the Chao index was lower under T2 but the Shannon index was higher, indicating a greater influence of SMX upon bacterial community diversity than from CTC or LIN (Figure 1).
The Venn diagram can be used to derive the number of common and unique OTUs in multiple groups or samples, to intuitively show the extent of similarity and overlap of the samples’ OTU composition (Figure 2A). The OTUs ranged from 658 to 746 in all six treatments, but peaked in T4, which was consistent with the results for the Shannon and Simpson indexes (Figure 1). The number of common OTUs was 441 in six treatments. Unique OTUs were most numerous in T4, with 23, followed by T3 (with 8) and T2 (6), and with 5 OTUs in other treatments (Figure 2A). CIP obviously influenced bacterial community structure, which not only increased the number of OTUs in the community, but also favored more unique OTUs in the composting process.
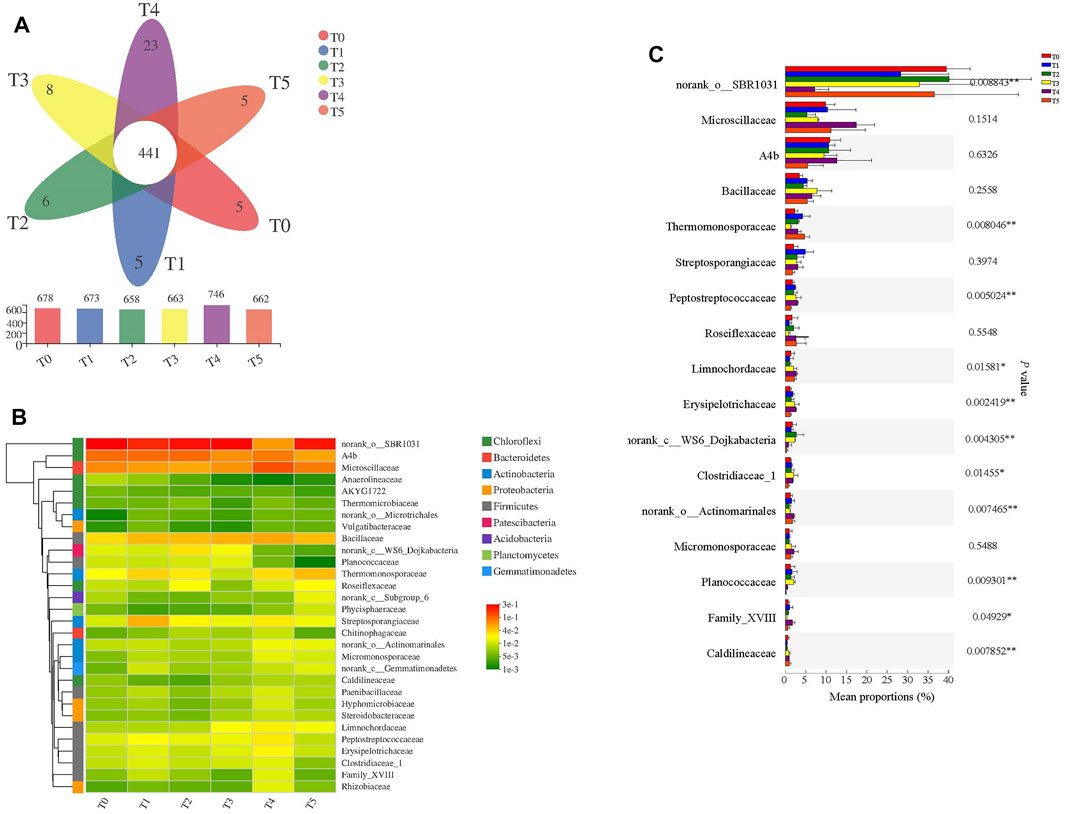
FIGURE 2. Effects of antibiotic type on bacterial community structure in six different treatments in swine manure composting. In (A) the Venn diagram at the phylum level; in (B) the heatmap of species composition at the family level, and in (C) the significance analysis of differences at the family level among the treatments. Comparison of Bacterial Composition at the Family Level among the Treatments.
To further investigate the bacterial community composition at the family level, the relative abundance of the first 30 families was analyzed in detail (Figure 2B). These top 30 families were mainly distributed in nine phyla: Firmicutes (26.67%), Chloroflexi (23.33%), Actinobacteria (16.67%), Proteobacteria (13.33%), Bacteroidetes (6.67%), Acidobacteria (3.33%), Patescibacteria (3.33%), Planctomycetes (3.33%), and Gemmatimonadetes (3.33%). Hence, members of Firmicutes and Chloroflexi were thus the most common by the end of composting. Firmicutes often underwent relatively high enrichment during composting due to its tolerance of high temperatures (Liu et al., 2018; Liu et al., 2020). Chloroflexi is a facultative anaerobe (Song et al., 2020) and aerobic composting yields localized anaerobic microenvironments (Franke-Whittle et al., 2014). Hence, it was natural that Chloroflexi had an advantage in composting.
The relative abundances of norank_SBR1031, A4B, Microscillaceae, Bacillaceae, and Thermomonosporaceae was higher that of other families in all treatments (Figure 2B). That of norank_SBR1031 peaked in T0, at 39.66% (Figure 2B), but reduced by the other antibiotic treatments (T1 through T5), its sensitivity to these tested antibiotics. The lowest abundance of norank_SBR1031 was 7.59% in T4 treatment. CIP is a bactericidal antibiotic that causes bacterial death by inhibiting DNA synthesis and replication (Gowrie and Abiraami, 2019), while CTC, SMX, and LIN are all anti-microbial agents (Zielezny et al., 2006; Bayen et al., 2014). These might be partly reasons for the lower abundance of norank_SBR1031 than other treatments. In addition, a previous study indicated that norank_SBR1031 was highly associated with the removal of antibiotics under anaerobic-denitrifying circumstances (Zheng et al., 2020). The relative abundance of A4b and Microscillaceae increased, reaching 10.75 and 17.12%, respectively, in T4, suggesting they have stronger resistance to CIP than the other antibiotics.
Furthermore, the relative abundances of Erysipelotrichaceae, Limnochordaceae, Clostridiaceae_1, Micromonosporaceae, Paenibacillaceae, Hyphomicrobiaceae, and Rhizobiaceae were all the highest in T4. The Bacillaceae often harbor a variety of antibiotic resistance genes, endowing them with resistant to various antibiotics, and their presence has been linked to antibiotic degradation, organic degradation, and nitrification (Liu et al., 2018; Qiu et al., 2021). In this study, compared with control (T0), the relative abundances of Bacillaceae, Streptosporangiaceae, Limnochordaceae, and Peptostreptococcaceae increased in the five antibiotic treatments, indicating that these bacteria are highly resistant to the various antibiotics. One-way ANOVA was used to test for significant differences in families’ composition among the different treatments (Figure 2C). Norank_SBR1031, Planococcaceae, Thermomonosporaceae, Peptostreptococcaceae, norank_Actinomarinales, norank_WS6_Dojkabacteria, and Erysipelotrichaceae all showed significant differences (p < 0.01). Limnochordaceae and Clostridiaceae_1 were also found to be significantly different among the treatments (p < 0.05). These results support the perspective that antibiotic types influence the composition of bacterial community structure (Liu et al., 2020).
Based on previous results, PcoA was used to study the similarity or difference of all microbial communities (Wang et al., 2018; Chang et al., 2021). Points with the same color represent different samples within the same treatment, and the distance between samples correspond to the size of differences between microbial communities under the six different treatments. Together, PC1 and PC2 explained 68.51% of the bacterial community differences, with PC1 accounting for 54.61% and PC2 for 13.9% of the variance in the data (Figure 3). The sample points of T3, T0, and T1 clearly intersected together, suggesting the family composition of T3 and T1 was the most similar to the control (T0) (Figure 3A). In another study, the addition of four concentrations of SMX (0, 25, 50, and 100 mg/kg) impacted bacterial community composition and diversity: there was a significant difference between the treatment of 25 mg/kg SMX vis-à-vis the other treatments (Sardar et al., 2021). Applying a greater antibiotic concentration can thus affect microbial community structure. Over time, the preferential growth of ARB generally occurs, leading to change in the sensitivity of the whole microbial community to antibiotics (Cycon et al., 2019). Using the compochip microarray, changes to microbial community structure in the mixed composting process of biogas residue were studied by Franke-Whittle et al. (2014), who found that the bacterial community composition was affected by the original composting material as well as the composting stage. In other research, changes in microbial community were influenced more by antibiotic concentration, composting raw materials, and composting stage than by antibiotic types during the composting (Zhang et al., 2018). In our study, the concentration of each antibiotic type was fixed at 50 mg/kg, which might explain, in part, the lack of significant differences in bacterial community composition among the four treatments by the end of swine manure composting.
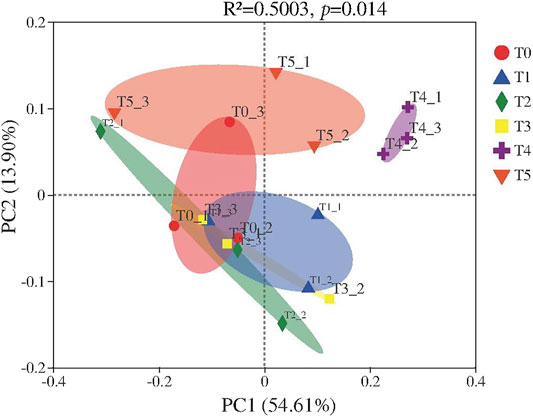
FIGURE 3. Analysis of bacterial community composition in six different treatments in swine manure composting.
The sample points of T5 intersected with a sample point of T0, but did not intersect with those of any other treatments, indicating that the effect of multiple antibiotics on family community composition was higher than that of CTC, SMX, and LIN, but still lower than that of CIP. These results indicated that CIP plays a major role in governing bacterial community composition and diversity under a multiple-antibiotic treatment (i.e., T5). Previously, the close distance showed that a mixture of three antibiotics (oxytetracycline, CIP, sulfamerazine) could reduce the selective pressure on CIP, perhaps by limiting the proliferation of certain bacteria (such as Lysinibacillus and Brachybacterium) that are resistant to CIP (Liu et al., 2020). The family composition in T4 treatment was clearly separated from the other five treatments, indicating that its families were significantly different. The common and unique families were then analyzed to study the differences between T4 and other treatments (Figure 4). Common families were the high relative abundance of bacterial community composition (Figures 2B, 4A). Eight unique families were found in the compost of T4: Crocinitomicaceae (79.10%), Saccharimonadaceae (5.97%), norank_Elusimicrobia (4.48%), Caulobacteraceae (1.49%), Methylophilaceae (2.99%), unclassified_Corynebacteriales (2.99%), Dermabacteraceae (1.49%), and norank_Actinobacteria (1.49%) (Figure 4B). Among them, Crocinitomicaceae had the highest relative abundance, by far. Yet there are few published reports about Crocinitomicaceae, what was only known was that it was a new family belonging to the class Flavobacteriia, the phylum Bacteroidetes (Munoz et al., 2016). However, it is undeniable that these unique families may partly explain the difference in bacterial community structure in T4 versus the other treatments.
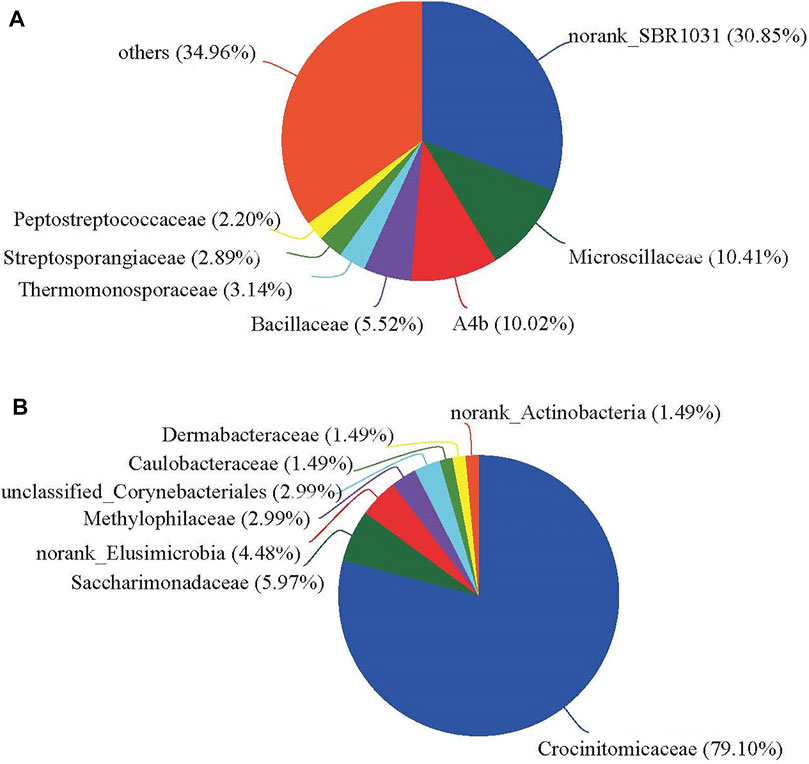
FIGURE 4. Pie charts conveying the unique and common families in the bacterial community in swine manure composting: (A) main families common to all six antibiotic treatments (T0 to T5), and (B) those families uniquely found in response to the ciprofloxacin addition treatment (i.e., T4).
Single Factor Network Analysis
In light of the above results, networks were constructed according to the difference in bacterial community composition between T4 and the other five treatments. This approach of network analysis is often utilized to explore the co-occurrence relationships of taxa members between bacterial communities (Zhang et al., 2018). The top-30 abundant families were selected for the correlation analysis of a single factor. Steroidobacteraceae, norank_SBR1031, Peptostreptococcaceae, Clostridiaceae_1, Bacillaceae, Erysipelotrichaceae, and Euzebyaceae were revealed to be relatively important bacterial families in Figure 5A (Supplementary Table S1). Birii41, norank_Alphaproteobacteria, Paenibacillaceae, Microscillaceae, Steroidobacteraceae, Blastocatellaceae, Family_XVIII, Desulfarculaceae, norank_Gemmatimonadetes, Peptostreptococcaceae, norank_subgroup_6, Micromonosporaceae, and Phycisphaeraceae were evidently the important families in Figure 5B (Supplementary Table S2).
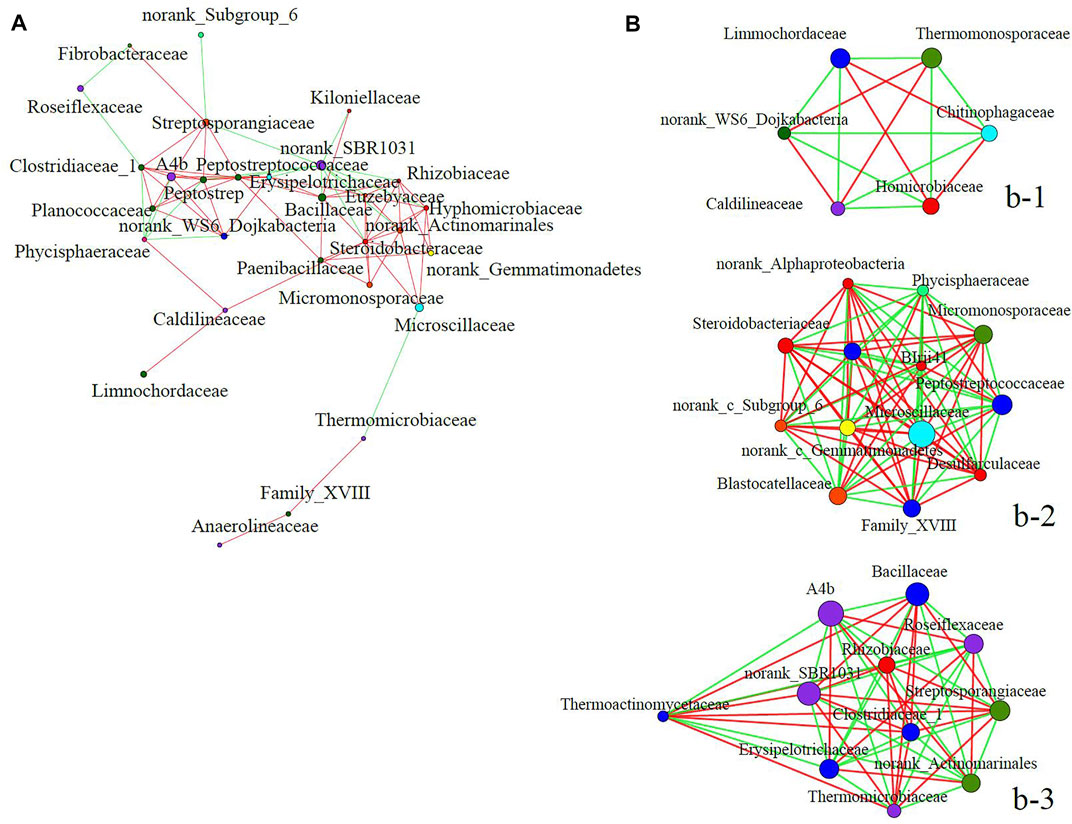
FIGURE 5. Single factor correlation networks of bacterial community structure at the family level. (A) Network analysis excluding the ciprofloxacin treatment; (B) network analysis of the ciprofloxacin treatment. Spearman correlations were used to analyze the relationships between species, rs ≥ 0.5, p value <0.05. The size of a node indicates the abundance of a species; the red lines show significant positive correlations and the green lines show the significant negative correlations.
Norank_SBR1031 was significantly negatively correlated with 11 families, including Kiloniellaceae, Streptosporangiaceae, Rhizobiaceae, Bacillaceae, and Chitinophagaceae (p < 0.05). This result indicated that norank_SBR1031 was antagonistic or competitive with these families, which could explain why its relative abundance exceeded theirs (Figure 2B). By contrast, norank_SBR1031 was significantly positively correlated with Bacillaceae, Rhizobiaceae, Streptosporangiaceae, Clostridiaceae_1, Thermomicrobiaceae, and Thermoactinomycetaceae in the community network of T4 (p < 0.05), pointing to the synergistic effects between them (Figure 5B). The relative abundance of norank_SBR1031 and Bacillaceae were 7.59 and 6.60%, respectively, in T4 (Figure 2B). Sobratee et al. (2009) also uncovered a competitive relationship among bacteria in environment. Therefore, these results could partially explain the relative abundance of different families in the total bacterial community after the composting of swine manure. Further, we found that Rhizobiaceae, Bacillaceae, and Streptosporangiaceae had significant negative correlations with norank_SBR1031 in Figure 5A, but positive correlations in Figure 5B. This suggests CIP could shift the direction of correlations between different families; perhaps another reason for the abundance and diversity of bacterial communities in T4 being starkly different from those in the other treatments.
The relative abundance of Microscillaceae was higher in T4 treatment than the other treatments (Figure 2B). Microscillaceae was significantly positively correlated with many bacterial families including Micromonosporaceae, Desulfarculaceae, Family_XVIII, and Paenibacillaceae in Figure 5A. However, Microscillaceae was negatively correlated with Thermomicrobiaceae and positively correlated with Steroidobacteraceae, norank_Actinomarinales, and Hyphomicrobiaceae in Figure 5B. In addition, these three families were all negatively correlated with norank_SBR1031, which suggests that Microscillaceae and norank_SBR1031 also engaged in an antagonistic or competitive relationship. Accordingly, the relative abundance of Microscillaceae (17.12%) increased while that of norank_SBR1031 decreased in T4 treatment. The network correlation analysis demonstrated that CIP might alter the competitive or cooperative relationships between different bacteria at the family level, resulting in bacterial community abundance and diversity that differs significantly from those of other treatments.
Main Factors Affecting the Change of Bacterial Community Structure
Previous studies indicated that changes in microbial community structure arisen in response to the physical and chemical properties of composting (Duan et al., 2019; Gurmessa et al., 2021; Wan et al., 2021). For example, redundancy analysis uncovered strong correlations between bacterial community abundance and manure’s physical and chemical properties and composition, with a positive correlation found between EC and C:N, and a negative correlation between total solids and the soluble components of heavy metals (Gurmessa et al., 2021). Another recent study revealed that the composition of both fungal and bacterial communities were significantly influenced by total phosphorus and NH4--N contents, indicating that composting’s metataxonomy is involved in phosphorus and nitrogen metabolism (Wan et al., 2021). One purpose of our study was to explore the specific physical and chemical properties of swine manure composting that might affect bacterial community composition.
Using SmartPLS software to build an SEM, a partial least-squares path model was used to assess the direct and indirect influences between the observed structures. The physical and chemical properties were taken from our previous study (Song et al., 2020). The influence of temperature, pH, EC, moisture content, and C:N upon changes to bacterial community structure was analyzed. We found that the bacterial community of the composted material was significantly affected by pH and C:N. Specifically, pH had a direct and significant positive influence on bacterial community (λ = 0.701, p < 0.01). The pH reflects the particular acid–base environment of microorganisms in the composting process, and a high or low pH could impact the growth of microorganisms and the decomposition of organic matter (Liu et al., 2019). On the one hand, microorganisms would cause organic acids to accumulate during the composting process (Lopez-Gonzalez et al., 2015); on the other hand, variation of pH values would lead to the changes in calcium and magnesium content during the composting, thus indirectly affecting the microbial community structure (Lucas et al., 2011). In our study, the pH was mostly similar across the different antibiotic treatments by the end of composting. At its early stage, it was CIP that maximally influenced the pH among the treatments (song et al., 2020). The bacterial community structure clearly became modified in the high-temperature stage of composting, perhaps because of the composting temperature’s influence on the growth and reproduction of bacterial populations, leaving the bacterial community structure highly stable in the ripening stage (Liu et al., 2020). The variation of pH is one of the main reasons for the changes in the bacterial community structure at the early stage of swine manure composting.
In addition, we found that bacterial community structure was significantly negatively affected by the C:N ratio (λ = −0.869, p < 0.01) (Figure 6), but pH was significantly positively correlated with C:N (λ = 0.592, p < 0.01). The variation in C:N was mainly due to the rapid degradation of organic matter and the decomposition of refractory substances, whose degradation was mainly driven by microorganisms’ activity in the composting process; therefore, the C:N modulated microbial community structure (Arab et al., 2017). Although temperature did not significantly affect the changed microbial community structure in this study (Figure 6), it could still lead to the changes in microbial community structure by affecting the biological activity of microorganisms (Wang J. et al., 2019). The addition of different antibiotics altered the physical and chemical properties of composting in its early stage, for which the effect of CIP was higher than other antibiotics (Song et al., 2020), leading to changes in bacterial community structure in the composting. To sum up, pH and C:N were respectively main physical and chemical factors affecting the bacterial community of swine manure composting. The change in bacterial community that occurred during the high-temperature period of composting in T4 led to its pronounced difference from other treatments by the end of composting.
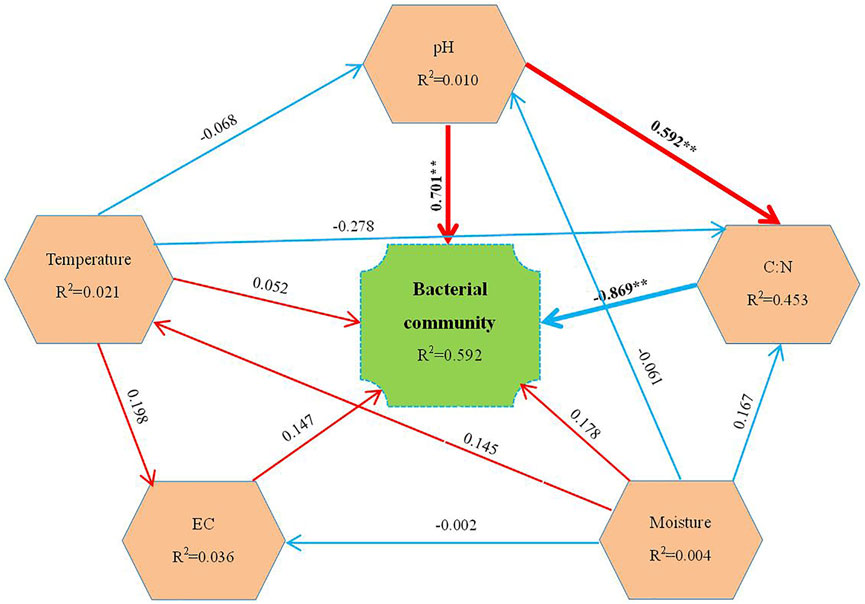
FIGURE 6. The derived structural equation model (SEM) linking the environmental factors to the total bacterial community structure. The path coefficients are given adjacent to the arrows. Blue and red lines respectively indicate the negative and positive pathways. The significance levels are indicated by *(p < 0.05), **(p < 0.01).
Conclusion
The Chao index increased under the antibiotic treatments, for which the ranking was T4 > T1 > T3 > T2 > T5 > T0 (range: 588.44–680.17). Ciprofloxacin had the most pronounced effect on bacterial diversity among all treatments, under which the Shannon index increased from 3.41 to 4.06 by the end of composting. At this time, Firmicutes (26.67%) and Chloroflexi (23.33%) were the most distributed phyla; norank_SBR1031, A4b, Microscillaceae, Bacillaceae, and Thermomonosporaceae were relatively abundant at the family level (among the top 30 families). The relative abundance of A4b and Microscillaceae increased to 10.75%, and 17.12%, respectively in T4 treatment. Bacillaceae, Streptosporangiaceae, Limnochordaceae, and Peptostreptococcaceae were all resistant to a variety of antibiotics. The PcoA results showed that the family composition of T4 was different from the other treatments: it had eight unique families, of which Crocinitomicaceae was dominant (79.10%). The network analysis revealed that ciprofloxacin altered the synergistic or competitive relationships between bacteria, and then caused the differences in bacterial community abundance and composition. Further, the structural equation modeling showed that pH had a direct and significant positive influence on the bacterial community, whereas it was significantly negatively affected by C:N ratio, especially under the ciprofloxacin treatment. Overall, ciprofloxacin significantly influenced the physical and chemical properties of composting in the early stage of composting, leading to significantly different bacterial community structure compared with other antibiotics.
Data Availability Statement
The data presented in the study are deposited in the Genbank database (https://www.ncbi.nlm.nih.gov/WebSub/), accession number PRJNA768315.
Author Contributions
Conceptualization, analysis, and original draft writing, TS; funding acquisition, and supervision, CZ; data visualization, BL and KJ; sample collection, XW and MS; correction, SX; funding acquisition, YH; methodology, original draft review, and funding acquisition, HL. All authors have read and agreed to the published version of the manuscript.
Funding
This research was funded by the National Water Pollution Control and Treatment Science and Technology Major Project in China (2017ZX07401002), the Natural Science Foundation of Beijing, China (6192029), The Young Elite Scientist Sponsorship Program by CAST (YESS) (2018QNRC001). National Key Research and Development Program of Hebei Province (20327507D).
Conflict of Interest
The authors declare that the research was conducted in the absence of any commercial or financial relationships that could be construed as a potential conflict of interest.
Publisher’s Note
All claims expressed in this article are solely those of the authors and do not necessarily represent those of their affiliated organizations, or those of the publisher, the editors and the reviewers. Any product that may be evaluated in this article, or claim that may be made by its manufacturer, is not guaranteed or endorsed by the publisher.
Acknowledgments
We thank Yu Liang and Wenjun Su for their help during experiment.
Supplementary Material
The Supplementary Material for this article can be found online at: https://www.frontiersin.org/articles/10.3389/fenvs.2021.786592/full#supplementary-material
References
Abiraami, V. S., and Gowrie, S. U. (2019). Sprouts as Functional Food- an Approach towards the Identification of Natural Antibiotic Resistance Breakers. J. Drug Deliv. Ther. 9, 23–35. doi:10.22270/jddt.v9i1-s.2240
Álvarez, J. A., Otero, L., Lema, J. M., and Omil, F. (2010). The Effect and Fate of Antibiotics during the Anaerobic Digestion of Pig Manure. Bioresour. Technology 101, 8581–8586. doi:10.1016/j.biortech.2010.06.075
Arab, G., Razaviarani, V., Sheng, Z., Liu, Y., and McCartney, D. (2017). Benefits to Decomposition Rates when Using Digestate as Compost Co-feedstock: Part II - Focus on Microbial Community Dynamics. Waste Management 68, 85–95. doi:10.1016/j.wasman.2017.07.014
Bayen, S., Yi, X., Segovia, E., Zhou, Z., and Kelly, B. C. (2014). Analysis of Selected Antibiotics in Surface Freshwater and Seawater Using Direct Injection in Liquid Chromatography Electrospray Ionization Tandem Mass Spectrometry. J. Chromatogr. A 1338, 38–43. doi:10.1016/j.chroma.2014.02.034
Brandt, K. K., Amézquita, A., Backhaus, T., Boxall, A., Coors, A., Heberer, T., et al. (2015). Ecotoxicological Assessment of Antibiotics: A Call for Improved Consideration of Microorganisms. Environ. Int. 85, 189–205. doi:10.1016/j.envint.2015.09.013
Chang, R., Li, Y., Li, N., Wu, X., and Chen, Q. (2021). Effect of Microbial Transformation Induced by Metallic Compound Additives and Temperature Variations during Composting on Suppression of Soil-Borne Pathogens. J. Environ. Manage. 279, 111816. doi:10.1016/j.jenvman.2020.111816
Chen, S., Zhou, Y., Chen, Y., and Gu, J. (2018b). Fastp: an Ultra-fast All-In-One FASTQ Preprocessor. Bioinformatics 34, i884–i890. doi:10.1093/bioinformatics/bty560
Chen, Y., Zhang, H., Luo, Y., and Song, J. (2012). Occurrence and Assessment of Veterinary Antibiotics in Swine Manures: A Case Study in East China. Chin. Sci. Bull. 57, 606–614. doi:10.1007/s11434-011-4830-3
Chen, Z., Wang, Y., and Wen, Q. (2018a). Effects of Chlortetracycline on the Fate of Multi-Antibiotic Resistance Genes and the Microbial Community during Swine Manure Composting. Environ. Pollut. 237, 977–987. doi:10.1016/j.envpol.2017.11.009
Cheng, D., Liu, Y., Shehata, E., Feng, Y., Lin, H., Xue, J., et al. (2021). In-feed Antibiotic Use Changed the Behaviors of Oxytetracycline, Sulfamerazine, and Ciprofloxacin and Related Antibiotic Resistance Genes during Swine Manure Composting. J. Hazard. Mater. 402, 123710. doi:10.1016/j.jhazmat.2020.123710
Cycoń, M., Mrozik, A., and Piotrowska-Seget, Z. (2019). Antibiotics in the Soil Environment-Degradation and Their Impact on Microbial Activity and Diversity. Front. Microbiol. 10, 338. doi:10.3389/fmicb.2019.00338
Duan, Y., Awasthi, S. K., Chen, H., Liu, T., Zhang, Z., Zhang, L., et al. (2019). Evaluating the Impact of Bamboo Biochar on the Fungal Community Succession during Chicken Manure Composting. Bioresour. Technology 272, 308–314. doi:10.1016/j.biortech.2018.10.045
Edgar, R. C. (2013). UPARSE: Highly Accurate OTU Sequences from Microbial Amplicon Reads. Nat. Methods 10, 996–998. doi:10.1038/nmeth.2604
Franke-Whittle, I. H., Confalonieri, A., Insam, H., Schlegelmilch, M., and Körner, I. (2014). Changes in the Microbial Communities during Co-composting of Digestates. Waste Management 34, 632–641. doi:10.1016/j.wasman.2013.12.009
Gao, P., Mao, D., Luo, Y., Wang, L., Xu, B., and Xu, L. (2012). Occurrence of Sulfonamide and Tetracycline-Resistant Bacteria and Resistance Genes in Aquaculture Environment. Water Res. 46, 2355–2364. doi:10.1016/j.watres.2012.02.004
Gurmessa, B., Ashworth, A. J., Yang, Y., Savin, M., Moore, P. A., Ricke, S. C., et al. (2021). Variations in Bacterial Community Structure and Antimicrobial Resistance Gene Abundance in Cattle Manure and Poultry Litter. Environ. Res. 197, 111011. doi:10.1016/j.envres.2021.111011
Hou, J., Wan, W., Mao, D., Wang, C., Mu, Q., Qin, S., et al. (2015). Occurrence and Distribution of Sulfonamides, Tetracyclines, Quinolones, Macrolides, and Nitrofurans in Livestock Manure and Amended Soils of Northern China. Environ. Sci. Pollut. Res. 22, 4545–4554. doi:10.1007/s11356-014-3632-y
Knight, R., Vrbanac, A., Taylor, B. C., Aksenov, A., Callewaert, C., Debelius, J., et al. (2018). Best Practices for Analysing Microbiomes. Nat. Rev. Microbiol. 16, 410–422. doi:10.1038/s41579-018-0029-9
Kumar Awasthi, M., Chen, H., Duan, Y., Liu, T., Kumar Awasthi, S., Wang, Q., et al. (2019). An Assessment of the Persistence of Pathogenic Bacteria Removal in Chicken Manure Compost Employing clay as Additive via Meta-Genomic Analysis. J. Hazard. Mater. 366, 184–191. doi:10.1016/j.jhazmat.2018.11.108
Kuppusamy, S., Kakarla, D., Venkateswarlu, K., Megharaj, M., Yoon, Y.-E., and Lee, Y. B. (2018). Veterinary Antibiotics (VAs) Contamination as a Global Agro-Ecological Issue: A Critical View. Agric. Ecosyst. Environ. 257, 47–59. doi:10.1016/j.agee.2018.01.026
Liu, F., Wu, J., Ying, G.-G., Luo, Z., and Feng, H. (2012). Changes in Functional Diversity of Soil Microbial Community with Addition of Antibiotics Sulfamethoxazole and Chlortetracycline. Appl. Microbiol. Biotechnol. 95, 1615–1623. doi:10.1007/s00253-011-3831-0
Liu, N., Hou, T., Yin, H., Han, L., and Huang, G. (2019). Effects of Amoxicillin on Nitrogen Transformation and Bacterial Community Succession during Aerobic Composting. J. Hazard. Mater. 362, 258–265. doi:10.1016/j.jhazmat.2018.09.028
Liu, Y., Cheng, D., Xue, J., Weaver, L., Wakelin, S. A., Feng, Y., et al. (2020). Changes in Microbial Community Structure during Pig Manure Composting and its Relationship to the Fate of Antibiotics and Antibiotic Resistance Genes. J. Hazard. Mater. 389, 122082. doi:10.1016/j.jhazmat.2020.122082
Liu, Y., Feng, Y., Cheng, D., Xue, J., Wakelin, S., and Li, Z. (2018). Dynamics of Bacterial Composition and the Fate of Antibiotic Resistance Genes and mobile Genetic Elements during the Co-composting with Gentamicin Fermentation Residue and Lovastatin Fermentation Residue. Bioresour. Technology 261, 249–256. doi:10.1016/j.biortech.2018.04.008
López-González, J. A., Suárez-Estrella, F., Vargas-García, M. C., López, M. J., Jurado, M. M., and Moreno, J. (2015). Dynamics of Bacterial Microbiota during Lignocellulosic Waste Composting: Studies upon its Structure, Functionality and Biodiversity. Bioresour. Technology 175, 406–416. doi:10.1016/j.biortech.2014.10.123
Lucas, R. W., Klaminder, J., Futter, M. N., Bishop, K. H., Egnell, G., Laudon, H., et al. (2011). A Meta-Analysis of the Effects of Nitrogen Additions on Base Cations: Implications for Plants, Soils, and Streams. For. Ecol. Management 262, 95–104. doi:10.1016/j.foreco.2011.03.018
Magoc, T., and Salzberg, S. L. (2011). FLASH: Fast Length Adjustment of Short Reads to Improve Genome Assemblies. Bioinformatics 27, 2957–2963. doi:10.1093/bioinformatics/btr507
Monteiro, S. C., and Boxall, A. B. A. (2010). Occurrence and Fate of Human Pharmaceuticals in the Environment. Rev. Environ. Contam. Toxicol. 202, 53–154. doi:10.1007/978-1-4419-1157-5_2
Munoz, R., Rosselló-Móra, R., and Amann, R. (2016). Revised Phylogeny of Bacteroidetes and Proposal of Sixteen New Taxa and Two New Combinations Including Rhodothermaeota Phyl. Nov. Syst. Appl. Microbiol. 39, 281–296. doi:10.1016/j.syapm.2016.04.004
Nakasaki, K., Tran, L. T. H., Idemoto, Y., Abe, M., and Rollon, A. P. (2009). Comparison of Organic Matter Degradation and Microbial Community during Thermophilic Composting of Two Different Types of Anaerobic Sludge. Bioresour. Technology 100, 676–682. doi:10.1016/j.biortech.2008.07.046
Pan, M., and Chu, L. M. (2017). Fate of Antibiotics in Soil and Their Uptake by Edible Crops. Sci. Total Environ. 599-600, 500–512. doi:10.1016/j.scitotenv.2017.04.214
Pham, T. D. M., Ziora, Z. M., and Blaskovich, M. A. T. (2019). Quinolone Antibiotics. Med. Chem. Commun. 10, 1719–1739. doi:10.1039/c9md00120d
Qiu, X., Zhou, G., Wang, H., and Wu, X. (2021). The Behavior of Antibiotic-Resistance Genes and Their Relationships with the Bacterial Community and Heavy Metals during Sewage Sludge Composting. Ecotoxicology Environ. Saf. 216, 112190. doi:10.1016/j.ecoenv.2021.112190
Ren, S., Guo, X., Lu, A., Guo, X., Wang, Y., Sun, G., et al. (2018). Effects of Co-composting of Lincomycin Mycelia Dregs with Furfural Slag on Lincomycin Degradation, Maturity and Microbial Communities. Bioresour. Technology 265, 155–162. doi:10.1016/j.biortech.2018.05.087
Ren, S., Lu, A., Guo, X., Zhang, Q., Wang, Y., Guo, X., et al. (2019). Effects of Co-composting of Lincomycin Mycelia Dregs with Furfural Slag on Lincomycin Degradation, Degradation Products, Antibiotic Resistance Genes and Bacterial Community. Bioresour. Technology 272, 83–91. doi:10.1016/j.biortech.2018.10.014
Sardar, M. F., Zhu, C., Geng, B., Huang, Y., Abbasi, B., Zhang, Z., et al. (2021). Enhanced Control of Sulfonamide Resistance Genes and Host Bacteria during Thermophilic Aerobic Composting of Cow Manure. Environ. Pollut. 275, 116587. doi:10.1016/j.envpol.2021.116587
Selvam, A., Xu, D., Zhao, Z., and Wong, J. W. C. (2012). Fate of Tetracycline, Sulfonamide and Fluoroquinolone Resistance Genes and the Changes in Bacterial Diversity during Composting of Swine Manure. Bioresour. Technology 126, 383–390. doi:10.1016/j.biortech.2012.03.045
Sobratee, N., Mohee, R., and Driver, M.-F. B. (2009). Variation of Broth Composition by Addition of Broiler Litter Composting Substrate Extracts: Influence on Faecal Bacterial Growth. J. Appl. Microbiol. 107, 1287–1297. doi:10.1111/j.1365-2672.2009.04318.x
Song, T., Zhu, C., Xue, S., Li, B., Ye, J., Geng, B., et al. (2020). Comparative Effects of Different Antibiotics on Antibiotic Resistance during Swine Manure Composting. Bioresour. Technology 315, 123820. doi:10.1016/j.biortech.2020.123820
Staley, Z. R., Schmidt, A. M., Woodbury, B., Eskridge, K. M., Durso, L., and Li, X. (2020). Corn Stalk Residue May Add Antibiotic‐resistant Bacteria to Manure Composting Piles. J. Environ. Qual. 49, 745–753. doi:10.1002/jeq2.20017
Tian, W., Sun, Q., Xu, D., Zhang, Z., Chen, D., Li, C., et al. (2013). Succession of Bacterial Communities during Composting Process as Detected by 16S rRNA Clone Libraries Analysis. Int. Biodeterioration Biodegradation 78, 58–66. doi:10.1016/j.ibiod.2012.12.008
Tran, H. T., Lin, C., Bui, X. T., Itayama, T., Dang, B. T., Cheruiyot, N. K., et al. (2021). Bacterial Community Progression during Food Waste Composting Containing High Dioctyl Terephthalate (DOTP) Concentration. Chemosphere 265, 129064. doi:10.1016/j.chemosphere.2020.129064
Van Boeckel, T. P., Brower, C., Gilbert, M., Grenfell, B. T., Levin, S. A., Robinson, T. P., et al. (2015). Global Trends in Antimicrobial Use in Food Animals. Proc. Natl. Acad. Sci. USA. 112, 5649–5654. doi:10.1073/pnas.1503141112
Wan, J., Wang, X., Yang, T., Wei, Z., Banerjee, S., Friman, V.-P., et al. (2021). Livestock Manure Type Affects Microbial Community Composition and Assembly during Composting. Front. Microbiol. 12, 621126. doi:10.3389/fmicb.2021.621126
Wang, J., Liu, Z., Xia, J., and Chen, Y. (2019b). Effect of Microbial Inoculation on Physicochemical Properties and Bacterial Community Structure of Citrus Peel Composting. Bioresour. Technology 291, 121843. doi:10.1016/j.biortech.2019.121843
Wang, K., Mao, H., and Li, X. (2018). Functional Characteristics and Influence Factors of Microbial Community in Sewage Sludge Composting with Inorganic Bulking Agent. Bioresour. Technology 249, 527–535. doi:10.1016/j.biortech.2017.10.034
Wang, L., Wang, J., Wang, J., Zhu, L., Yang, L., and Yang, R. (2019a). Distribution Characteristics of Antibiotic Resistant Bacteria and Genes in Fresh and Composted Manures of Livestock Farms. Sci. Total Environ. 695, 133781. doi:10.1016/j.scitotenv.2019.133781
Wang, Q., Garrity, G. M., Tiedje, J. M., and Cole, J. R. (2007). Naïve Bayesian Classifier for Rapid Assignment of rRNA Sequences into the New Bacterial Taxonomy. Appl. Environ. Microbiol. 73, 5261–5267. doi:10.1128/AEM.00062-07
Wang, Y., Liu, L., Yang, J., Duan, Y., Luo, Y., Taherzadeh, M. J., et al. (2020). The Diversity of Microbial Community and Function Varied in Response to Different Agricultural Residues Composting. Sci. Total Environ. 715, 136983. doi:10.1016/j.scitotenv.2020.136983
Yang, Q., Tian, T., Niu, T., and Wang, P. (2017). Molecular Characterization of Antibiotic Resistance in Cultivable Multidrug-Resistant Bacteria from Livestock Manure. Environ. Pollut. 229, 188–198. doi:10.1016/j.envpol.2017.05.073
Zhang, R., Gu, J., Wang, X., Li, Y., Zhang, K., Yin, Y., et al. (2018). Contributions of the Microbial Community and Environmental Variables to Antibiotic Resistance Genes during Co-composting with Swine Manure and Cotton Stalks. J. Hazard. Mater. 358, 82–91. doi:10.1016/j.jhazmat.2018.06.052
Zhao, L., Dong, Y. H., and Wang, H. (2010). Residues of Veterinary Antibiotics in Manures from Feedlot Livestock in Eight Provinces of China. Sci. Total Environ. 408, 1069–1075. doi:10.1016/j.scitotenv.2009.11.014
Zhao, S., Schmidt, S., Qin, W., Li, J., Li, G., and Zhang, W. (2020). Towards the Circular Nitrogen Economy - A Global Meta-Analysis of Composting Technologies Reveals Much Potential for Mitigating Nitrogen Losses. Sci. Total Environ. 704, 135401. doi:10.1016/j.scitotenv.2019.135401
Zheng, J., Wang, S., Zhou, A., Zhao, B., Dong, J., Zhao, X., et al. (2020). Efficient Elimination of Sulfadiazine in an Anaerobic Denitrifying Circumstance: Biodegradation Characteristics, Biotoxicity Removal and Microbial Community Analysis. Chemosphere 252, 126472. doi:10.1016/j.chemosphere.2020.126472
Zhong, Y., Yan, W., Wang, R., Wang, W., and Shangguan, Z. (2018). Decreased Occurrence of Carbon Cycle Functions in Microbial Communities along with Long-Term Secondary Succession. Soil Biol. Biochem. 123, 207–217. doi:10.1016/j.soilbio.2018.05.017
Keywords: swine manure, ciprofloxacin, bacterial community, antibiotic types, aerobic composting, structural equation model
Citation: Song T, Zhu C, Li B, Jiang K, Wang X, Sardar MF, Xue S, Huang Y and Li H (2021) Ciprofloxacin Causes the Greatest Bacterial Community Variation in Swine Manure Composting. Front. Environ. Sci. 9:786592. doi: 10.3389/fenvs.2021.786592
Received: 30 September 2021; Accepted: 22 November 2021;
Published: 20 December 2021.
Edited by:
Xiuping Zhu, Louisiana State University, United StatesReviewed by:
Si Li, China Agricultural University, ChinaHanlin Zhang, Shanghai Academy of Agricultural Sciences, China
Copyright © 2021 Song, Zhu, Li, Jiang, Wang, Sardar, Xue, Huang and Li. This is an open-access article distributed under the terms of the Creative Commons Attribution License (CC BY). The use, distribution or reproduction in other forums is permitted, provided the original author(s) and the copyright owner(s) are credited and that the original publication in this journal is cited, in accordance with accepted academic practice. No use, distribution or reproduction is permitted which does not comply with these terms.
*Correspondence: Hongna Li, lihongna828@163.com