- 1College of Management Engineering and Business, Hebei University of Engineering, Handan, China
- 2Hebei Key Laboratory of Intelligent Water Conservancy, Hebei University of Engineering, Handan, China
At the beginning of 2020, COVID-19 broke out. Because the virus is extremely contagious and the mortality rate after infection is extremely high, China and many countries in the world have imposed lockdowns. Air pollutants during the epidemic period have attracted the attention of many scholars. This research is to use predictive models to describe changes in extreme air pollutants. China is the first country in the world to enter the lockdown state. This study uses data from 2015-2020 to compare and predict the concentration of extreme pollutants before and after the lockdown. The results show that the lockdown of the epidemic will reduce the annual average concentration of PM2.5, and the annual average concentration of O3 will increase first and then decrease. Through analysis, it is concluded that there is a synergistic decrease trend between PM2.5 and O3. With the various blockade measures for epidemic prevention and control, the reduction of extreme air pollutant concentrations is sustainable. The assessment of China’s air quality in conjunction with the COVID-19 can provide scientific guidance for the Chinese government and other relevant departments to formulate policies.
Introduction
At the end of 2019, Wuhan (China) reported a new coronavirus disease (COVID-19) for the first time. The virus was found all over the world in a very short time. At the end of January 2020, the World Health Organization (WHO) declared a state of public health emergency, and the COVID-19 widespread concern in the international community (WHO 2020). In the first place, China has put all efforts across the country to support Wuhan, the hardest-hit area of the epidemic. At the same time, work and production were suspended across the country for home isolation. With the continuous efforts of the Chinese people, the COVID-19 has been gradually suppressed.
Under the lockdown of the COVID-19, people’s activities are restricted. Thereby reducing the concentration of environmental pollutants to improve China’s air quality (Shakoor et al., 2020). And extreme air pollution has gradually become an important indicator of air quality. The development trend of extreme air pollution affects China’s future air quality. Accurate pollution prediction plays an important role in air pollution control.
The Guiding Opinions on Doing a Good Job in the Normalization of the Prevention and Control of the New Coronary Pneumonia Epidemic pointed out that the COVID-19 will exist in China for a certain period of time. Many scholars have discussed the impact of environmental quality on the COVID-19. But this paper will discuss how extreme air pollution will develop in China under the normalization of the epidemic. That is, what impact does the COVID-19 have on air quality (Cai et al., 2021). However, due to the short duration of the n COVID-19, there are fewer data available for application. This paper will use the gray model to predict and analyze China’s air quality. This article will provide researchers with a new perspective to analyze China’s air quality in combination with gray forecasting models. The assessment of China’s air quality in conjunction with COVID-19 can provide scientific guidance for the Chinese government and other relevant departments to formulate policies.
This paper is divided into five sections. The introduction is given in Introduction. The literature review is provided in Literature Review. The methods are introduced in Methods. The results and discussion are presented in Results and Discussion. The conclusions are drawn in Conclusion.
Literature Review
The new crown virus was discovered at the end of 2019, and since then there have been endless discussions about the new crown. Because of severe air pollution in winter, humans are at higher risk of contracting the new crown (Naqvi et al., 2021). Therefore, more scholars have focused their research on the impact of air and climate on COVID-19 (Linares et al., 2021), NO2, PM2.5, PM10, O3 are directly proportional to the incidence and mortality of COVID-19 (Montse and Domingo José, 2022). Weather has become an important factor in determining the disease (Khurram et al., 2002). Temperature plays an important role in slowing down the spread of COVID-19 (Najaf Iqbal et al., 2020). The performance of the new crown varies in different environments (Shahzad et al., 2021). However, the harsh environment will always increase the incidence and mortality of new coronary pneumonia (Fareed et al., 2020). In order to control the development of the epidemic, the lockdown period has been continuously extended, and the new crown epidemic has therefore been partially controlled. During the COVID-19 lockdown, air quality has been significantly improved (Pandey et al., 2021; Raffaele et al., 2021). The reduction in personal travel and economic activities provides researchers with an opportunity to objectively evaluate air pollutants (Adam et al., 2021). The main pollutants of air quality can be determined by the changes in the concentration of pollutants before and after the epidemic is blocked (Xu et al., 2021). Thierno et al. (2021) used the atmospheric simulation data set method to analyze the changes in air pollution emissions during the epidemic. Found that the concentration of air pollutants dropped significantly during the blockade (Maji et al., 2021). According to environmental data released by NASA and ESA, it is found that pollution in many places has been reduced to 30% (Muhammad et al., 2020). For the quantitative analysis of air pollutants. Many scholars used a variety of methods to measure the air quality during the blockade, such as satellite data (Fatemeh and Alihsan, 2021), double-difference models (Yumin et al., 2021), and machine learning algorithms (Wei et al., 2021). However, since the outbreak of the new crown epidemic, there are fewer air pollutant data available to study, resulting in inaccurate prediction results of some models. The gray fractional cumulative GM(1,1) model (FGM(1,1) model) is extremely effective in short-term prediction (Wu et al., 2013). Therefore, this paper will use the FGM(1,1) model to predict the extremely polluted air quality in China to analyze the impact of the lockdown of the COVID-19 epidemic on air quality.
Methods
Overview of Extreme Pollution in Seven Provinces and Three Cities
The concentration of air pollutants reflects the overall air quality at a specific time and place. In recent years, China has taken a variety of measures to deal with air pollution, and air quality has improved significantly. However, at this stage, China is still facing the pressure of extremely polluted air quality. Especially in summer, ozone has become the primary factor leading to excessive air quality in some cities, especially in key areas such as the Beijing-Tianjin-Hebei and surrounding areas, the Yangtze River Delta, the Fen-Wei Plain, and the junction of Jiangsu, Anhui, Shandong, and Henan. The scope of air pollution prevention and control strengthened supervision and designated assistance areas expanded from key areas such as Beijing-Tianjin-Hebei to non-key areas such as Jiangsu, Anhui, Shandong, Henan, etc. The target is summer ozone pollution. The increasing trend of ozone pollution is very obvious, and in some cities ozone even exceeds PM2.5 as the primary pollutant. This shows that the control of extremely polluted air quality is imminent.
The Beijing-Tianjin-Hebei region is at the heart of China, with one of the largest economies in the country but also the most serious air quality problems. The industrial structure of Shanghai in the Yangtze River Delta is relatively balanced and the industrial industry is relatively developed, but the air quality is relatively poor. Shanxi Province and Shaanxi Province, the key areas of the Fen-Wei Plain, are rich in resources, and the continuous demand for resource extraction activities has deteriorated air quality. Structural problems are prominent in the junction area of Jiangsu, Anhui, Shandong and Henan. The development of steel and petrochemical industry leads to large basic emissions of air pollutants in this area, and it is relatively difficult to control them. The detailed geographical location is shown in Figure 1. However, air quality management is not achieved overnight. It requires long-term planning and collaborative governance Forecasting the annual average air index concentration from 2021 to 2025 can provide technical support for future air quality management of local governments.
Data and Methods
The annual average concentration of inhalable particulate matter (PM2.5) and the annual average 90% quantile concentration of 8-hour ozone (8-h O3) are selected as predictive indicators. The indicators data are from the Air Quality Assessment Report VIII (CSSPKU, 2021), Table 1; Table 2. The current air quality data depends on a new standard (GB 3095-2012) published in 2012. The PM2.5 values were mandatorily included in this new standard for the first time. Only a few sets of annual data are available. Due to the limited amount of data, the effect of other forecasting methods is not obvious. Therefore, the grey forecasting theory can deal with the forecasting problem of limited samples. The high-precision FGM(1,1) model is used to predict the annual average concentrations of PM2.5 and 8-h O3 from 2021 to 2025 in seven provinces and three cities.
Given a non-negative time series
Step 1: By using
where
The original time series has been represented by superscription
Step 2: For the
Where
Because the least squares estimate minimizes the sum of the squared residuals, the parameters are obtained by using the least squares. The unknown parameters
where
Step 3: Inputting
Step 4:
Where
Step 5: The mean absolute percentage error (MAPE) is used for evaluating the models, which is calculated as:
Where
Calculation Process
Take Beijing as an example. The data from 2015 to 2020 are taken as the sample set. The traditional GM(1,1) model and the FGM(1,1) model are established respectively. The FGM(1,1) modelling process is as follows.
The average annual concentration of PM2.5 is
The optimal fractional order found by the particle swarm algorithm (PSO) is 0.39, The 0.39-order accumulation sequence is
The unknown parameters
where
Then, the time response function is
Can get
The Predictive Sequence is
Model Validation
The fitting results of annual average concentration of PM2.5 in Beijing are shown in Table 3. The MAPE of the FGM(1,1) model is significantly lower than that of the traditional GM(1,1) model. The result of the FGM(1,1) model with the best score is obtained through PSO on MaltlabR 2018b. In this order, the MAPE is the smallest and the fitting accuracy is higher. In addition, the Wilcoxon signed rank test is widely used to determine the importance of the superiority of a predictive model (Xiangmei Meng et al., 2021). According to the above literature, the prediction accuracy of FGM(1,1) model and traditional GM(1,1) model is consistent with the fitting results of Beijing PM2.5 concentration. Wilcoxon signed rank test and 0.05 significance level 1 test show that the FGM(1,1) model is significantly better than the traditional GM(1,1) model. Therefore, it is effective to use FGM(1,1) model to predict Beijing PM2.5 concentration.
Results and Discussion
Extremely Polluted Air Quality Forecast
The forecast results of the annual average concentration of PM2.5 in Beijing are listed in Table 4.
Similarly, the 8-hour ozone (8-h O3) annual average 90% quantile concentration is also limited. Grey prediction theory is also applicable. In order to obtain higher-precision prediction values, the FGM(1,1) model is used to predict these indicators. Therefore, the annual average concentrations of inhalable particulate matter (PM2.5) and 8-h O3 in Beijing, Tianjin, and Shanghai from 2021 to 2025 are predicted by the FGM(1,1) model, respectively.
Figure 2 shows the changing trends of air indicators in Beijing, Tianjin, and Shanghai. Obviously, the annual average concentrations of PM2.5 and 8-h O3 in the three cities are decreasing and the ranges are different. Among these indicators, the annual average PM2.5 concentration in 2020–2025 will exceed 50
Using similar methods, predict the annual average concentrations of PM2.5 and 8-h O3 in Shanxi, Hebei, Henan, Shandong, Jiangsu, Shaanxi and Anhui provinces respectively.
The change trends of air indicators in Shanxi, Hebei, Henan and Shandong provinces are shown in Figure 3. It can be seen from the figure that the downward trend of annual average PM2.5 concentration is basically the same in the four provinces, and the trend of annual average concentration of 8-h O3 varies. The annual average concentration of PM2.5 declines rapidly and steadily from 2020 to 2025, which is better than the performance of the above three cities. In 2025, the annual average concentration of PM2.5 in the three provinces except Shandong is less than 50
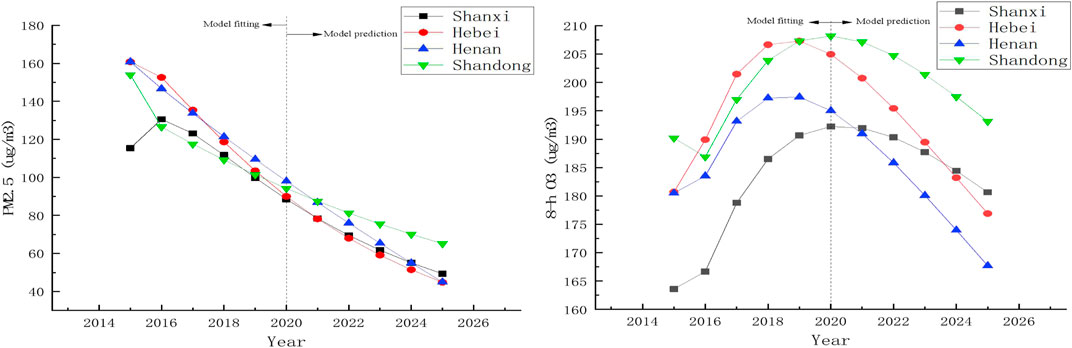
FIGURE 3. Trends of annual average concentrations of PM2.55 and 8-h O3 in the four northern provinces.
The change trends of air indicators in Jiangsu, Shanxi and Anhui provinces are shown in Figure 4. From the figure, it can be seen that the annual average concentration of PM2.5 and 8-h O3 in Anhui Province has dropped significantly. The annual average concentration of PM2.5 and 8-h O3 in Jiangsu Province declined relatively slowly. The raw data of air quality indicators in Jiangsu, Shanxi and Anhui provinces are better than the other three cities and four provinces. By 2025, only Anhui province’s annual average concentration of PM2.5 will fall below 50
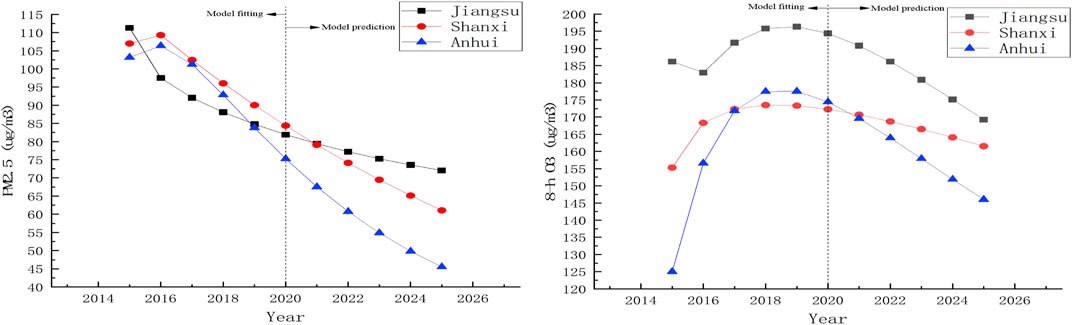
FIGURE 4. Trends of annual average concentrations of PM2.5 and 8-h O3 in the three southern provinces.
Discussion of Analysis
Based on the above prediction results, it can be known that the lockdown of the COVID-19 will have a continuous impact on China’s extreme air pollution. That is, the annual average concentration of PM2.5 in China will continue to decrease under lockdown, which is the same as the results of Shakoor et al. (2020) and (Yumin et al., 2021) research. However, the annual average concentration of O3 will increase first and then decrease, which is the same as the results of the study by (Wei et al., 2021).
The reason for the decrease in the concentration of extreme air pollutants is inseparable from the national policy control, such as coal-to-gas, loose coal management, ultra-low emissions from coal combustion, and energy-saving transformation (Lifu et al., 2018). Another important aspect is that after the outbreak of the COVID-19, China has actively adopted strict and comprehensive prevention and control measures. The whole society suspends work and production, and all employees are isolated at home (Hsiang et al., 2020). The simultaneous suspension of industry and construction has led to a sharp reduction in inhalable particulate matter. At the same time, home isolation restricts residents’ traffic and travel, which sharply reduces the pollutants emitted by fuel vehicles. The lockdown of the epidemic has accelerated the reduction in the annual average concentration of PM2.5. However, the annual average concentration of PM2.5 in some cities did not decrease significantly. It is necessary to consider the shortage of epidemic prevention materials during the epidemic. Medical material production enterprises resumed work ahead of schedule and started production at full capacity. (http://finance.people.com.cn/n1/2020/0131/c1004-31565804.html)No inspection of medical rescue vehicles (http://www.baiduhl.com/z/2019/10/17/8 0405.html). However, after the reduction of PM2.5, there is no barrier of haze, and sunlight can penetrate the air more easily. Although ultraviolet light is not directly related to killing viruses (Farooq et al., 2021), it provides more energy for surface ozone. So while the PM2.5 concentration is reduced, ozone pollution is further aggravated (Li et al., 2019). This also indirectly proves the research conclusions of Shahzad et al. (2021). That is, there is a significant interaction between PM2.5 in air quality and ozone and the transmission of new coronary pneumonia. Ozone is the main factor that increases the spread of the COVID-19 (Sarwar et al., 2021), and the harsh environment will increase the death rate of the new crown virus (Fareed et al., 2020). Most ozone is produced by secondary conversion of anthropogenic NOX (nitrogen oxides) and VOCs (volatile organic compounds) under high-temperature light conditions. NASA researchers found that NO2 first dropped sharply in Wuhan in a short period of time. At the same time, the NO2 concentration in four metropolitan areas has declined (Cai et al., 2021). NO2 mainly comes from toxic gases emitted by motor vehicles, power plants and industrial facilities. During the period of the epidemic blockade, the emissions of these toxic gases will be reduced, which will reduce the concentration of ozone. This is also in line with the above-mentioned prediction results.
Conclusion
This may be the first paper using grey theory to explore the impact of COVID-19 on extreme air pollution. The data from 2015 to 2020 are used for comparative analysis. This study uses the grey fractional model to predict extreme air pollution in ten regions of China in the next 4 years. The results show that COVID-19 blockade will reduce the concentration of extreme air pollutants in China.
Research on extreme air pollutants during the lockdown period can reduce the impact of human factors on them. Thus, it can be considered directly from the source of extreme air pollution. Simply banning the discharge of certain pollutants will have limited governance effects, and sometimes adverse effects will occur. In response to China’s extreme air pollution. The main strategy is to further advance the adjustment of the industrial structure, further optimize the energy structure, further optimize the transportation structure, strengthen the reduction of nitrogen oxides and VOCs, promote the modernization of the governance system and capacity, and realize the coordinated control of ozone pollution and PM2.5. (http://www.zhangye.gov.cn/zyszfxxgk/zfwj_5652/zzf_5653/ 202009/t20200918_486525.html).
Finally, in an era when extreme air pollutants are proliferating, the normalized development of the COVID-19 can moderately improve extreme air pollution. However, the fundamental way to comprehensively improve extreme air pollution is to reduce fossil fuel consumption, switch to more environmentally friendly energy sources, develop green transportation networks, and avoid biomass burning. Specifically, the industry can be rationally planned, the energy structure can be changed, clean energy can be promoted, and clean production processes can be used. Continue to implement the vehicle number restriction policy, and strengthen the environmental supervision and management of relevant government departments. During the COVID-19, some unnecessary organizations and units have disintegrated or are on the verge of disintegration. Government departments should issue policies to prohibit unnecessary organizations that occupy public resources. And for a long period of time in the future, China will be required to remain in a semi-closed state to protect the health of the people and the quality of the environment. With the normalization of COVID-19, many pollutants have changed trends compared to the past. In future research, we will guide us to conduct a more comprehensive analysis of air quality.
Data Availability Statement
The original contributions presented in the study are included in the article/Supplementary Material, further inquiries can be directed to the corresponding author.
Author Contributions
XP: Data curation, Investigation, Methodology, Project administration, Formal analysis, Resources, Validation, Visualization, Editing. MW: Writing-original draft. YZ: Conceptualization, Funding acquisition, Supervision.
Funding
The relevant researches are supported by the National Natural Science Foundation of China (U20A20316), the Excellent Young Scientist Foundation of Hebei Education Department (SLRC2019001), the Natural Science Foundation of Hebei Province (E2020402074), the Handan Social Science Federation Project (2021058, 2021077), the Soft Science Research Project of Hebei Provincial Science and Technology Program (215576114D), and the Humanities and Social Science Research Project of Higher Education in Hebei Province (SQ201027).
Conflict of Interest
The authors declare that the research was conducted in the absence of any commercial or financial relationships that could be construed as a potential conflict of interest.
Publisher’s Note
All claims expressed in this article are solely those of the authors and do not necessarily represent those of their affiliated organizations, or those of the publisher, the editors and the reviewers. Any product that may be evaluated in this article, or claim that may be made by its manufacturer, is not guaranteed or endorsed by the publisher.
References
Adam, M. G., Tran, P. T. M., and Balasubramanian, R. (2021). Air Quality Changes in Cities during the COVID-19 Lockdown: A Critical Review. Atmos. Res. 264, 105823. doi:10.1016/j.atmosres.2021.105823
Cai, Wan-Jin., Wang, Hong-Wei., Cui-Lin, Wu., Lu, Kai-Fa., Zhong-Ren, Peng., and He, Hong-Di. (2021). Characterizing the Interruption-Recovery Patterns of Urban Air Pollution under the COVID-19 Lockdown in China. Shanghai, China: Building and Environment, 205.
Fareed, Z., Iqbal, N., Shahzad, F., Shah, S. G. M., Zulfiqar, B., Shahzad, K., et al. (2020). Co-variance Nexus between COVID-19 Mortality, Humidity, and Air Quality index in Wuhan, China: New Insights from Partial and Multiple Wavelet Coherence. Air Qual. Atmos. Health 13, 673–682. doi:10.1007/s11869-020-00847-1
Farooq, A., Kumar, U., Uddin, J. B. G., Rashid, M. H. U., Gilani, M. M., Farooq, T. H., et al. (2021). Climatological and Social Fallacies about COVID-19 Pandemic. Environ. Sustainability 2021, 1–6. doi:10.1007/s42398-021-00175-9
Fatemeh, Ghasempour., Aliihsan, Sekertekin., and Senol Hakan, Kutoglu. (2021). Google Earth Engine Based Spatio-Temporal Analysis of Air Pollutants before and during the First Wave COVID-19 Outbreak over Turkey via Remote Sensing. J. Clean. Prod. 319, 128599. doi:10.1016/j.jclepro.2021.128599
Hsiang, S., Allen, D., Annan-Phan, S., Bell, K., Bolliger, I., Chong, T., et al. (2020). The Effect of Large-Scale Anti-contagion Policies on the COVID-19 Pandemic. Nature 584 (7820), 262–267. doi:10.1038/s41586-020-2404-8
Iqbal, N., Fareed, Z., Shahzad, F., He, X., Shahzad, U., and Lina, M. (2020). The Nexus between COVID-19, Temperature and Exchange Rate in Wuhan City: New Findings from Partial and Multiple Wavelet Coherence. Sci. Total Environ. 729, 138916. doi:10.1016/j.scitotenv.2020.138916
Khurram, Shahzad., Umer, Shahzad., Iqbal, Najaf., Farrukh, Shahzad., and Zeeshan, Fareed. (2020). Effects of Climatological Parameters on the Outbreak Spread of COVID-19 in Highly Affected Regions of Spain. Environ. Sci. Pollut. Res. Int. 27 (31), 39657–39666. doi:10.1007/s11356-020-10551-3
Li, K., Jacob, D. J., Liao, H., Shen, L., Zhang, Q., and Bates, K. H. (2019). Anthropogenic Drivers of 2013-2017 Trends in Summer Surface Ozone in China. Proc. Natl. Acad. Sci. USA 116 (2), 422–427. doi:10.1073/pnas.1812168116
Lifu, Munila. Abdumtai., Halik, Maize., Aishan, Tayirjan., Keyim, Merdang., Nasrdin, Nasman., and Ainwar, Aria. (2018). Study on the Characteristics of Main Air Pollutant Concentration Changes in Urumqi City. J. Ecol. Environ. 27 (03), 533–541.
Linares, C., Belda, F., López-Bueno, J. A., Luna, M. Y., Sánchez-Martínez, G., Hervella, B., et al. (2021). Short-term Associations of Air Pollution and Meteorological Variables on the Incidence and Severity of COVID-19 in Madrid (Spain): a Time Series Study. Environ. Sci. Eur. 33 (1), 107. doi:10.1186/s12302-021-00548-1
Maji, K. J., Namdeo, A., Bell, M., Goodman, P., Nagendra, S. M. S., Barnes, J. H., et al. (2021). Unprecedented Reduction in Air Pollution and Corresponding Short-Term Premature Mortality Associated with COVID-19 Lockdown in Delhi, India. J. Air Waste Manag. Assoc. 71 (9), 1085–1101. doi:10.1080/10962247.2021.1905104
Montse, Marquès., and Domingo José, L. (2022). Positive Association between Outdoor Air Pollution and the Incidence and Severity of COVID-19. A Review of the Recent Scientific Evidences. Environ. Res. 203, 111930. doi:10.1016/j.envres.2021.111930
Muhammad, S., Long, X., and Salman, M. (2020). COVID-19 Pandemic and Environmental Pollution: A Blessing in Disguise. Sci. Total Environ. 728, 138820. doi:10.1016/j.scitotenv.2020.138820
Naqvi, H. R., Mutreja, G., Hashim, M., Singh, A., Nawazuzzoha, M., Naqvi, D. F., et al. (2021). Global Assessment of Tropospheric and Ground Air Pollutants and its Correlation with COVID-19. Atmos. Pollut. Res. 12 (9), 101172. doi:10.1016/j.apr.2021.101172
Pandey, M., George, M. P., Gupta, R. K., Gusain, D., and Dwivedi, A. (2021). Impact of COVID-19 Induced Lockdown and Unlock Down Phases on the Ambient Air Quality of Delhi, Capital City of India. Urban Clim. 39, 100945. doi:10.1016/j.uclim.2021.100945
Raffaele, Cucciniello., Letizia, Raia., and Ermanno, Vasca. (2021). Air Quality Evaluation during COVID-19 in Avellino (South Italy): A Significant Case Study to Drive an Urgent Policy Decision Making. Environ. Res. 203, 111803. doi:10.1016/j.envres.2021.111803
Sarwar, S., Shahzad, K., Fareed, Z., and Shahzad, U. (2021). A Study on the Effects of Meteorological and Climatic Factors on the COVID-19 Spread in Canada during 2020. J. Environ. Health Sci. Engineer2021, 1–9. doi:10.1007/s40201-021-00707-9
Shahzad, K., Farooq, T. H., Zhong Hu, B. L., and Shahzad, U. (2021). Does Environmental Quality and Weather Induce COVID-19: Case Study of Istanbul, Turkey. Environ. Forensics2021, 1–12. doi:10.1080/15275922.2021.1940380
Shakoor, A., Chen, X., Farooq, T. H., Shahzad, U., Ashraf, F., Rehman, A., et al. (2020). Fluctuations in Environmental Pollutants and Air Quality during the Lockdown in the USA and China: Two Sides of COVID-19 Pandemic. Air Qual. Atmos. Health 13, 1335–1342. doi:10.1007/s11869-020-00888-6
Thierno, Doumbia., Claire, Granier., Nellie, Elguindi., Idir, Bouarar., Guy, Brasseur., Benjamin, Gaubert., et al. (2021). Changes in Global Air Pollutant Emissions during the COVID-19 Pandemic: a Dataset for Atmospheric Modeling. Earth Syst. Sci. Data 13 (8), 4191–4206. doi:10.5194/essd-13-4191-2021
Wei, Y., Xu, Q. X., Zhao, J. S., and Zhang, R. Q. (2021). Simulation Analysis of the Impact of COVID-19 Pandemic Control on Air Quality in Henan Province Based on Machine Learning Algorithm. Huan Jing Ke Xue 42 (9), 4126–4139. doi:10.13227/j.hjkx.202012288
Wu, L., Liu, S., Yao, L., Yan, S., and Liu, D. (2013). Grey System Model with the Fractional Order Accumulation. Commun. Nonlinear Sci. Numer. Simulation 18 (7), 1775–1785. doi:10.1016/j.cnsns.2012.11.017
Xu, Chao., Wu, Shuipi., Liu, Yijing., and Zhong, Xuefen. (2021). The Response of Air Quality in Xiamen Bay to the Control of the New crown Epidemic. Environ. Sci. 2021, 1–11. doi:10.13227/j.hjkx.202103009
Keywords: Extremely polluted air quality, COVID-19 impact, Seven provinces and Three cities, FGM(1, 1) model, Predict
Citation: Pan X, Zhao Y and Wang M (2021) Impact of COVID-19 on Extremely Polluted Air Quality and Trend Forecast in Seven Provinces and Three Cities of China. Front. Environ. Sci. 9:770900. doi: 10.3389/fenvs.2021.770900
Received: 06 September 2021; Accepted: 22 September 2021;
Published: 06 October 2021.
Edited by:
Yiliao Song, University of Technology Sydney, AustraliaReviewed by:
Feng Liu, University of Technology Sydney, AustraliaUmer Shahzad, Anhui University of Finance and Economics, China
Copyright © 2021 Pan, Zhao and Wang. This is an open-access article distributed under the terms of the Creative Commons Attribution License (CC BY). The use, distribution or reproduction in other forums is permitted, provided the original author(s) and the copyright owner(s) are credited and that the original publication in this journal is cited, in accordance with accepted academic practice. No use, distribution or reproduction is permitted which does not comply with these terms.
*Correspondence: Yonggang Zhao, MTIwNzUyNTVAcXEuY29t