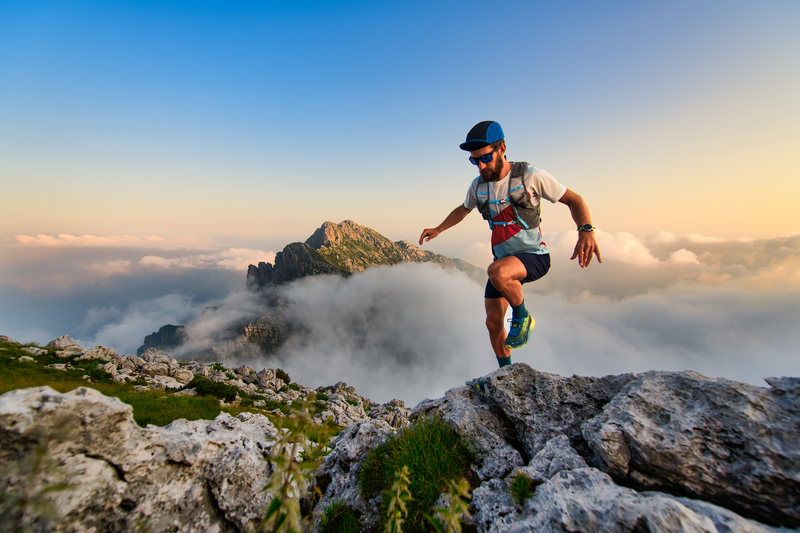
95% of researchers rate our articles as excellent or good
Learn more about the work of our research integrity team to safeguard the quality of each article we publish.
Find out more
MINI REVIEW article
Front. Environ. Sci. , 29 October 2021
Sec. Toxicology, Pollution and the Environment
Volume 9 - 2021 | https://doi.org/10.3389/fenvs.2021.757365
This article is part of the Research Topic Antibiotics and Antibiotic Resistance Genes in Waters: Pollution, Risks, and Control View all 11 articles
Antibiotics resistance genes (ARGs) are mainly caused by the extensive use and abuse of antibiotics and have become a global public health concern. Owing to the development of high-throughput sequencing, metagenomic sequencing has been widely applied to profile the composition of ARGs, investigate their distribution pattern, and track their sources in diverse environments. However, the lack of a detailed transmission mechanism of ARGs limits the management of its pollution. Hence, it’s essential to introduce how to utilize the metagenomic data to obtain an in-depth understanding of the distribution pattern and transmission of ARGs. This review provides an assessment of metagenomic data utilization in ARG studies and summarizes current bioinformatic tools and databases, including ARGs-OAP, ARG analyzer, DeepARG, CARD, and SARG, for profiling the composition of ARGs and tracking the source of ARGs. Several bioinformatic tools and databases were then benchmarked. Our results showed that although SARG is a good database, the application of two or more bioinformatic tools and databases could provide a comprehensive view of ARG profiles in diverse environmental samples. Finally, several perspectives were proposed for future studies to obtain an in-depth understanding of ARGs based on metagenomic data. Our review of the utilization of metagenomic data together with bioinformatic tools and databases in ARG studies could provide insights on exploring the profiles and transmission mechanism of ARG in different environments that mitigate the spread of ARGs and manage the ARGs pollution.
Since the discovery of penicillin, researchers have opened the modern era of the innovation, development, and application of antibiotics in human society. At present, antibiotics are used as medicine for humans and animals and widely applied in animal husbandry, agriculture, and aquaculture (Manage, 2018). However, with the intense use and abuse of antibiotics for human and agricultural purposes, antibiotics are continuously discharged into different environments, particularly those with limited sewage treatment capacity, resulting in a substantial increase of antibiotic residue in different environmental niches (Carvalho and Santos, 2016; Qiao et al., 2018). These residual antibiotics increase the risk of antibiotic resistance and produce antibiotic resistance genes (ARGs) that could be transferred to various microorganisms. This phenomenon is not new (Yang et al., 2018) and has attracted global concern, particularly its spread and transmission mechanism (Holmes et al., 2016). To date, antibiotics and their effects on different environment niches, for example, the emergence and spread of ARGs, have become an urgent and growing global public health threat in environmental science (Sanderson et al., 2016; Yang et al., 2018; Iwu et al., 2020). Hence, many researchers paid attention to ARGs to investigate their distribution and transmission.
With the successes of investigation on ARGs, researchers have identified the composition of ARGs and explored their distribution in different environment niches. For example, a total of 139, 442, and 491 ARG subtypes were identified in sediments from the Yamuna River, sediments from an urban river in Beijing (Chaobai River), and activated sludge reactors, respectively (Chen et al., 2019a; Zhao et al., 2019; Das et al., 2020). Based on these published studies, we found that many studies have focused on the composition of ARGs and their dynamics; however, only a few studies investigated the transmission of ARGs; the transmission route for ARGs is poorly characterized (Zhou et al., 2018; Chao et al., 2019; Vrancianu et al., 2020). A comparison on the occurrence and abundance of ARGs and microbiota in healthy humans and sewage treatment systems in a Chinese village identified 53 ARGs and 28 bacteria genera in all samples; this result supports the idea that bacteria could carry and transfer several ARGs to humans and the environment (Zhou et al., 2018). Furthermore, different mechanisms of horizontal gene transfer, including conjugation, transduction, and transformation, were also found to contribute to the accumulation and transmission of ARGs in bacteria (Chen et al., 2019b; Li et al., 2019; Vrancianu et al., 2020). Given the scarcity of studies exploring the transmission of ARGs, their detailed transmission mechanism remains elusive.
Recently, many sequencing techniques have been developed and applied in ARG studies. Owing to its advantages, high-throughput sequencing has been widely applied in microbiome studies to detect ARGs and is expected to solve the problem of transmission and proliferation mechanism of ARGs in different environments. With the growing number of microbiome studies focusing on ARGs, many metagenomic datasets, bioinformatic tools, and associated ARG databases have been generated for ARG analysis. By using these tools and databases, researchers have profiled ARG composition in different environments and deepened the understanding of ARGs. However, some urgent scientific questions remain unanswered, such as which bioinformatic tools and ARG databases are suitable for detecting potential ARGs? In addition, the various equations for ARG abundance calculation make it impossible to directly compare the results of different ARG studies. Besides, details on the transmission and management of ARGs remain elusive. Therefore, given that antimicrobial resistance is still a crucial and urgent threat to human’s health and the environment, a summary of the methods and prospects for ARG studies is essential. Therefore, this review first summarized the popular and latest bioinformatics methods for analyzing the metagenomic data generated by next-generation sequencing and third-generation sequencing, including bioinformatic tools, ARG databases, and MGE databases. And then several bioinformatic tools and databases were benchmarked to evaluate their benefits and drawbacks. Finally, several critical comments and perspectives were proposed for future ARG studies to obtain an in-depth understanding of ARGs based on metagenomic data.
In past decades, researchers have proved that microbiota plays an important role in maintaining human health (Marchesi et al., 2016; Valdes et al., 2018) and participating in biogeochemical circulation (Carnevali et al., 2021). Owing to its advantages, high-throughput sequencing has been widely applied in ARG studies. With the successful investigation on microbial communities in diverse environments, massive metagenomic datasets have been produced to investigate the taxonomical and functional compositions of microbial communities, obtain an in-depth understanding of functional traits, such as nitrogen cycle (Jansson and Hofmockel, 2018; Miao and Liu, 2018) and ARGs (Stalder et al., 2019; Xiang et al., 2020), and explore the driving factors for the dynamic changes of functional traits (Pan et al., 2020). For example, based on metagenomic datasets, ARG profiles in different environments have been investigated and explored, such as activated sludge under high selective pressure with different antibiotics (Zhao et al., 2019) and seed activated sludge collected from a municipal wastewater treatment plant and five experiment groups with different antibiotics (Zhao et al., 2020) and a deep subtropical lake (Carnevali et al., 2021). These studies revealed that metagenomic sequencing creates an opportunity for capturing the majority of ARGs and their potential hosts. In addition, metagenomic analysis can reveal the transmission of ARGs and the risk of resistome (including ARGs) (Manaia, 2017; Yin et al., 2019; Qian et al., 2021a). In summary, proper utilization of metagenomic data can effectively provide an in-depth understanding of ARGs in the environment, particularly their transmission and risks.
With the increasing of metagenomic datasets from next-generation sequencing and third-generation sequencing, many bioinformatic tools have been developed to conduct analyses at different aspects. In general, the methodological approaches of the whole metagenomic dataset can be divided into two types, namely, assembly-based and read-based (non-assembly, Figure 1A) (Boolchandani et al., 2019; Harris et al., 2019). With these strategies, several bioinformatics tools, including online tools, have been developed for identifying the ARGs and detecting new ARGs (Figure 1).
FIGURE 1. Workflow and several bioinformatic tools and databases used in ARG studies for detecting potential ARGs. (A) Two different strategies used in ARG studies for profiling the composition of ARGs based on metagenomic data; (B) Information of several bioinformatic tools used for detecting potential ARGs. (C) Information of several bioinformatic databases used in ARG studies.
Specifically, on the basis of assembly-based strategy, ARGs-OAP (v1.0 and v2.0, http://smile.hku.hk/SARGs) (Yang et al., 2016; Yin et al., 2018), ARGs-OSP (http://smile.hku.hk/SARGs) (Zhang et al., 2020), PathoFact (https://git-r3lab.uni.lu/laura.denies/PathoFact/) (de Nies et al., 2021), ARG analyzer (ARGA, http://mem.rcees.ac.cn:8083/) (Wei et al., 2019), Resistance Gene Identifier (RGI, https://github.com/arpcard/rgi) (Alcock et al., 2020), ResFinder (https://cge.cbs.dtu.dk/services/ResFinder/), DeepARG (https://bench.cs.vt.edu/deeparg) (Arango-Argoty et al., 2018), and HMD-ARG (http://www.cbrc.kaust.edu.sa/HMDARG/) (Li et al., 2021a) were developed and have been widely applied to detect potential ARGs from the gene datasets predicted from metagenomic contigs (Figure 1B). Together with the ARG database, ARGs-OAP was designed as an online pipeline to fast annotate and classify ARG-like sequences from metagenomic data (Yang et al., 2016). Compared with the version 1.0 of ARGs-OAP, the latest version was updated and added with the Hidden Markov Model algorithm for the enhancement characterization and quantification of ARGs in metagenomic datasets based on the 16S rRNA gene and the average coverage of essential single-copy marker genes (Yin et al., 2018). Similarly, to solve the most challenging topics and provide a guide for diverse research in ARG studies, including the risk, evolution, and emergence of ARGs, a comprehensive profile of the distribution of ARGs on an ARGs online searching platform (ARGs-OSP) was constructed based on the distribution of potential ARGs in 55,000 bacterial genomes, 16,000 bacterial plasmid sequences, 3,000 bacterial integrin sequences and 850 metagenomes (Zhang et al., 2020). Furthermore, PathoFact was designed and developed to solve the virulence factors (VFs) and ARGs of pathogenic microorganisms; this an easy-to-use, modular, and reproducible tool can predict VFs, bacterial toxins, and ARG from metagenomic data with high accuracy (de Nies et al., 2021). Moreover, on the basis of an updated database, ARGA was developed to assess the primer of ARGs and identify and annotate ARGs from environmental metagenomes (Wei et al., 2019). It should be noted that the identification of potential ARGs usually depends on the search results. The selection strategy is to choose the best hit among the search results; however, this strategy can produce a high rate of false negatives. As a solution, DeepARG with two deep learning models (Arango-Argoty et al., 2018) and HMD-ARG (Li et al., 2021a) were constructed for ARG detection.
In contrast, only a few read-based bioinformatic tools were developed for ARG detection. For example, one deep learning model of DeepARG, namely, DeepARG-SS, was designed to analyze the short-read sequences in metagenomes (Arango-Argoty et al., 2018). Moreover, it’s well-known that Oxford Nanopore sequencing can produce ultra-long read sequencing reads; however, the identified ARGs can be analyzed at reads level. As a solution, ARGpore (Xia et al., 2017) and NanoARG (Arango-Argoty et al., 2019) were constructed (Figure 1B). Specifically, ARGpore was designed to detect ARGs and their hosts by utilizing BLAST, HMMER, and UBLAST (Xia et al., 2017). NanoARG was constructed as a web service to identify the ARGs from the long reads generated by Oxford Nanopore sequencing and provide the identification of metal resistance genes, mobile genetic elements (MGEs), and sequences with high similarity to known pathogens (Arango-Argoty et al., 2019).
In summary, diverse bioinformatic tools have been constructed and developed with different strategies. These bioinformatic tools can efficiently detect ARGs in different environments to meet the requirements of ARG analysis. With the use of ARG profiles in environmental metagenomes, downstream analyses on co-occurrence patterns among ARGs, the arrangement of ARGs and MGEs, and host identification of ARGs can be performed to enhance the understanding of ARGs in diverse environments.
The identification of potential ARGs depends on the search results against the database. Therefore, ARG databases are very important because they determine the accuracy and completeness of ARGs in environmental metagenomes. To date, several ARGs databases have been constructed for ARG detection (Figure 1C), such as Antibiotic Resistance Genes Database (ARDB, http://ardb.cbcb.umd.edu/) (Liu and Pop, 2009), the Comprehensive Antibiotic Resistance Database (CARD, https://card.mcmaster.ca/) (McArthur et al., 2013), structured ARG-database (SARG, http://smile.hku.hk/SARGs) (Yang et al., 2016; Yin et al., 2018), sequence database of antibiotic resistance genes (SDARG, http://mem.rcees.ac.cn:8083/) (Wei et al., 2019), and deepARG-DB (Arango-Argoty et al., 2018). Specifically, ARDB was constructed in 2009 and contains the resistance information for 13,293 genes and 257 antibiotics (Liu and Pop, 2009). This database was widely applied to detect potential ARGs but is now abandoned because of the lack of updates. As a solution, CARD was rigorously constructed and developed in 2013. This database integrates disparate molecular and sequence data, provides a unique organizing principle (antibiotic resistance ontology and antimicrobial resistance gene detection models), and can quickly and effectively detect putative ARGs (McArthur et al., 2013). CARD is currently a bioinformatic database and a compressive platform for identifying resistance genes, including their products and associated phenotypes (https://card.mcmaster.ca/). In 2016, SARG was constructed with a hierarchical structure (type-subtype-reference sequence) by integrating the two most commonly used ARG databases ARDB and CARD, removing their redundant sequences, and re-selecting the query sequences based on the similarity of sequences; this database can identify ARG sequences through similarity search (Yang et al., 2016) and has been widely used in ARG studies (Zhao et al., 2019; Zhao et al., 2020). The latest version of SARG (v2.0) has tripled the sequences of the first version, improved the coverage of ARG detection, and annotated the high-throughput raw reads by using a similarity search strategy in diverse environmental metagenomes (Zhao et al., 2020). Based on ARDB, an updated SDARG, including 1,260,069 protein sequences and 1,164,479 nucleotide sequences from 448 types of ARGs belonging to 18 categories of antibiotics, was constructed and used in ARGA (Wei et al., 2019). Moreover, as a companion database to DeepARG, DeepARG-DB was designed to improve the quality of the model (Arango-Argoty et al., 2018). These ARG databases provide choices for researchers to comprehensively detect ARGs in environmental metagenomes.
Additionally, previous studies have demonstrated that the transmission of ARGs is associated with MGEs and the relationship between ARGs and MGEs is a hot topic in ARG studies (Wang et al., 2020a; Liu et al., 2021; Lin et al., 2021; Wang et al., 2020b). Therefore, the associated databases for MGE identification in metagenomic datasets must be constructed to address these issues. At present, several databases, including ACLAME (Leplae et al., 2004; Leplae et al., 2010), ISfinder Database (Siguier et al., 2006), ISsaga2 (Varani et al., 2011), INTEGRALL (Moura et al., 2009), Gypsy Database (GyDB) (Llorens et al., 2010), and a MGE database (https://github.com/KatariinaParnanen/MobileGeneticElementDatabase) (Pärnänen et al., 2018), have been constructed and applied to investigate the occurrence of ARGs and MGEs.
Together, these diverse ARG and MGE databases provide a powerful resource for identifying ARGs and MGEs, exploring the distribution of ARGs, investigating the relationship between ARGs and MGEs, and obtaining an in-depth understanding of ARG transmission that benefits their management.
Several bioinformatic tools, including Abricate (https://github.com/tseemann/abricate), DeepARG, and Blastx, and ARG databases, including ARG-ANNOT, Resfinder, CARD, DeepARG-DB, and SARG (v2.0), were benchmarked to determine which bioinformatic tools and ARG databases are suitable to detect potential ARGs in diverse environmental samples. In particular, three metagenomic datasets were collected for each of the five kinds of environmental niches chosen for benchmarking, namely, the water of lake (SRR14578319, SRR14578320, and SRR14578321), the sediment of lake (SRR14887730, SRR14887731, and SRR14887732), activated sludge (SRR14610228, SRR14610229, and SRR14610230), ocean (SRR14865845, SRR14865846, and SRR14865847), and human feces (SRR15032192, SRR15032193, and SRR15032194). Finally, 15 metagenomic datasets were downloaded from NCBI. The quality of these metagenomic datasets was controlled with Trimmomatic (Bolger et al., 2014) using the following parameters: TruSeq3-PE.fa:2:30:10, LEADING:3, TRAILING:3, SLIDINGWINDOW:5:20, and MINLEN:25, to obtain high-quality reads. High-quality reads were assembled by MEGAHIT (Li et al., 2016) with the following parameters: -meta-large and a k-mer list of 27, 37, 47, 57, 67, 77, 87, 97, 107, 117, and 127, to obtain the metagenomic contigs (contigs of length >500 bp were kept), and then the gene sequences and protein sequences were predicted with Prodigal (Hyatt et al., 2010). The nucleic acid sequences obtained from different environmental samples were searched against the ARG-ANNOT, Resfinder, and CARD databases by using Abriate and the potential ARGs were selected with similarity ≥80% and coverage ≥70%. Whereas, the potential ARGs identified with DeepARG (v2.0) were selected with default settings (similarity ≥80%), and the ARG candidates identified with Blastx against SARG databases (v2.0, protein sequences) were selected with similarity ≥80% and coverage ≥70%.
Comparison of ARG profiles in various environmental niches identified with different bioinformatic tools and databases revealed inconsistency in the kinds and total number of ARGs (Figure 2). For the sediment of lake and activated sludge samples, the number of ARG types identified with ARG-ANNOT, CARD, and Resfinder was higher than that with DeepARG-DB but fewer than that with SARG (v2.0, Figure 2A). Similarly, the total number of ARGs identified with SARG (v2.0) was the highest among all databases (Figure 2B). Further comparison of ARG profiles in sample SRR14610228 revealed the differences in the intersections of ARG profiles detected with two, three, four, and five bioinformatic tools and databases (Figure 2C). All these results suggested that although SARG (v2.0) is a good database for identifying potential ARGs, the application of two or more bioinformatic tools and databases could provide comprehensive ARG profiles in different environmental samples.
FIGURE 2. Benchmark of the bioinformatic tools and databases for ARG detection in various environments. Distribution of (A) kinds of ARGs and (B) the total number of ARGs identified in various environmental niches with different bioinformatic tools and databases. (C) Different kinds of ARGs detected with different ARG databases in sample SRR14610228.
Considering the tight linkage between ARGs in the environment, the ARG source is important to their transmission and management. Therefore, an ARG source tracking platform must be urgently developed. In the past 2 decades, many researchers have realized the importance of tracking the source of ARG and thus developed many bioinformatic tools or frameworks. Among which, a series of bioinformatic tools or frameworks were developed and proposed for tracking ARG pollution from different sources, such as SourceTracker (Knights et al., 2011) and its application in metagenomic datasets (Meta-SourceTracker) (McGhee et al., 2020), Microbial Source Tracking (MST) (Li et al., 2018), Meta-Prism (Zhu et al., 2021), and FEAST (Shenhav et al., 2019). However, only a few tools and applications were used to track the genetic location of the host of ARGs (host-tracking of ARGs), such as PlasFlow (Krawczyk et al., 2018). Specifically, among these source-tracking tools, some including SourceTracker and MST can be used to precisely track ARG pollution from different sources. For example, based on deep-sequencing marker genes, such as 16S rRNA, SourceTracker was designed and constructed with a Bayesian classification model; this tool uses Gibbs sampling to determine the possibility and predict the source of samples (Knights et al., 2011) and has been widely applied to determine the source of ARG pollution in diverse environment samples (Hu et al., 2020; Chen et al., 2019c). Moreover, on the basis of a machine-learning classification strategy with ARG abundance profiles, MST was developed and constructed as a source-tracking platform that can precisely track ARG pollution from different sources, such as feces of humans and animals, wastewater treatment plants, and other natural environments (Li et al., 2018; Li et al., 2020), which is available at https://smile.hku.hk/SARGs/. Additionally, based on the genome signatures of sequences from 9,565 bacterial plasmid and chromosomes, PlasFlow with a deep neural network model was developed to predict the bacterial plasmid sequences or chromosomes in metagenomic contigs with high classification accuracy (Krawczyk et al., 2018) and then assist in the tracking of the genetic location and taxonomy of ARG host. To date, the accurate host-tracking of ARGs remains a challenge in ARG studies. Nevertheless, numerous studies using these bioinformatic tools have been conducted to determine the source of ARG pollution and host-tracking of ARGs and explore the distribution pattern of ARGs in diverse environment samples (Ma et al., 2017; Chen et al., 2019c; Dang et al., 2020; Raza et al., 2021; Zhou et al., 2021). Undoubtedly, tracking the source of ARG, including the source of ARG pollution and the host-tracking of ARGs, is important to obtain an in-depth understanding of ARG transmission and provide suggestions for managing ARGs in natural environments.
ARG pollution caused by the overuse of antibiotics has increased in diverse natural environments and has become a global concern about human health. At present, the metagenomic dataset produced by high-throughput techniques was popularly applied in ARG studies. Numerous investigations have been conducted to explore the distribution, transmission, source of ARG, and the key factors driving ARGs (Li et al., 2015; Zhao et al., 2020; Li et al., 2021b). Although an increasing number of studies have been conducted, the lack of in-depth understanding of ARGs limits the management and elimination of ARG pollution. Hence, we provide several critical perspectives about research methods and data analysis in ARG studies to deep mining the knowledge of ARGs.
First, the deep mining of metagenomic datasets is essential, especially in studying the transmission and host-tracking of ARGs. Current metagenomic analyses are mainly focused on the detection of ARGs, the co-occurrence network among ARGs, and the relationship between ARGs and MGEs. However, the content of the analysis is nearly ending, and the metagenomic dataset is not fully utilized. Hence, a comprehensive analysis of metagenomic data is essential to expand the understanding of ARGs. For example, investigating the arrangement of ARGs and their relationship with adjacent genes and MGEs is a potential approach to reveal the transmission of ARGs. Moreover, on the basis of metagenome binning results, the taxonomy of ARG-carrying contigs can be accurately identified, and the key challenge of annotating the taxonomical source of ARG can be solved, thereby benefiting the host-tracking of ARGs.
Second, a formula or standard for calculating the ARGs abundance should be unified. Recent calculation methods of ARGs abundance are diverse, such as the transcripts per kilobase of exon model per million mapped reads (TPM) (Jing and Yan, 2020), reads per kilobase of exon per million mapped reads (RPKM) (Sekizuka et al., 2020), one read in one million reads (parts per million, ppm) (Zhang et al., 2015), (number) copy of ARG per copy of 16S rRNA gene (Li et al., 2015), and abundance (coverage, ×/Gb) (Zhao et al., 2020). This condition limits the intuitive comparison of the profiles and risks of ARGs in various environments. Designing and appointing a unified formula for calculating ARG abundance are necessary to estimate ARG pollution and its risks in different environments.
Third, the application of third-generation sequencing in ARG studies can expand the understanding of ARGs, especially their genetic location and hosts. Third-generation sequencing techniques, such as Pacific Biosciences (PacBio) and Nanopore sequencing techniques, should be applied to profile ARG composition in diverse environments, explore the occurrence pattern of ARGs, and track their source. These techniques can generate long reads and obtain large genomes that can span most repetitive sequences and benefit the taxonomical identification of ARGs (Ye et al., 2016; Qian et al., 2021b). For example, the profile, genetic location, and hosts of ARGs, particularly the potential ARG-carrying pathogens, were investigated and explored throughout the wastewater treatment process by using the combination of Nanopore and Illumina sequencing; this work established a baseline analysis framework to explore ARGs in environmental niche and expanded the knowledge of resistome in wastewater treatment plants (Che et al., 2019). Several shortcomings, such as the cost of sequencing and the extract method of high-quality DNA, limit the use of third-generation sequencing in current ARG studies.
Finally, the findings should be verified in a wet laboratory. Current ARG studies mainly collected samples from natural environments, and the results or conclusions are untested and un-verified in a wet laboratory. Hence, proper experimental works should be designed and conducted to simulate the natural environment in the laboratory and verify the pattern of ARGs under these conditions. The results will have substantial implications for estimating ARG pollution and managing the related risks.
This review summarized current bioinformatic approaches and databases for identifying potential ARGs in metagenomic data. In particular, several bioinformatic tools and databases were benchmarked to estimate their advantages in detecting ARGs in different environmental niches. Several suggestions were also proposed to expand the analysis content of ARG studies. Together, by accumulating and updating current bioinformatic tools for analyzing metagenomic datasets and ARG and MGE databases, source-tracking tools for ARGs, and providing perspectives for future ARG studies, this comprehensive review provides a holistic assessment of the application of metagenomic data in ARG studies. The findings provide insights into the transmission of ARGs and pave the way for establishing priority in managing ARG pollution.
MH and ZW designed the study. ZP, YM, MH, and ZW wrote the initial draft of the manuscript. All authors read, modified, and approved the final manuscript.
This work was partially supported by Grants for Scientific Research of BSKY (No: XJ201916) from Anhui Medical University, Young Foundation of Anhui Medical University (2020xkj015), Cultivation Fund of School of Life Sciences from Anhui Medical University, the Key Project of Hubei Province Natural Science Foundation (2020CFA110), and the Youth Innovation Promotion Association, Chinese of Academy of Sciences (2018369).
The authors declare that the research was conducted in the absence of any commercial or financial relationships that could be construed as a potential conflict of interest.
All claims expressed in this article are solely those of the authors and do not necessarily represent those of their affiliated organizations, or those of the publisher, the editors and the reviewers. Any product that may be evaluated in this article, or claim that may be made by its manufacturer, is not guaranteed or endorsed by the publisher.
Alcock, B. P., Raphenya, A. R., Lau, T. T. Y., Tsang, K. K., Bouchard, M., Edalatmand, A., et al. (2020). CARD 2020: Antibiotic Resistome Surveillance with the Comprehensive Antibiotic Resistance Database. Nucleic Acids Res. 48, D517–D525. doi:10.1093/nar/gkz935
Arango-Argoty, G. A., Dai, D., Pruden, A., Vikesland, P., Heath, L. S., and Zhang, L. (2019). NanoARG: a Web Service for Detecting and Contextualizing Antimicrobial Resistance Genes from Nanopore-Derived Metagenomes. Microbiome 7, 88. doi:10.1186/s40168-019-0703-9
Arango-Argoty, G., Garner, E., Pruden, A., Heath, L. S., Vikesland, P., and Zhang, L. (2018). DeepARG: a Deep Learning Approach for Predicting Antibiotic Resistance Genes from Metagenomic Data. Microbiome 6, 23. doi:10.1186/s40168-018-0401-z
Bolger, A. M., Lohse, M., and Usadel, B. (2014). Trimmomatic: a Flexible Trimmer for Illumina Sequence Data. Bioinformatics 30, 2114–2120. doi:10.1093/bioinformatics/btu170
Boolchandani, M., D'Souza, A. W., and Dantas, G. (2019). Sequencing-based Methods and Resources to Study Antimicrobial Resistance. Nat. Rev. Genet. 20, 356–370. doi:10.1038/s41576-019-0108-4
Carnevali, P. B. M., Lavy, A., Thomas, A. D., Crits-Christoph, A., Diamond, S., Méheust, R., et al. (2021). Meanders as a Scaling Motif for Understanding of Floodplain Soil Microbiome and Biogeochemical Potential at the Watershed Scale. Microbiome 9, 1–23. doi:10.1186/s40168-020-00957-z
Carvalho, I. T., and Santos, L. (2016). Antibiotics in the Aquatic Environments: a Review of the European Scenario. Environ. Int. 94, 736–757. doi:10.1016/j.envint.2016.06.025
Chao, H., Kong, L., Zhang, H., Sun, M., Ye, M., Huang, D., et al. (2019). Metaphire Guillelmi Gut as Hospitable Micro-environment for the Potential Transmission of Antibiotic Resistance Genes. Sci. Total Environ. 669, 353–361. doi:10.1016/j.scitotenv.2019.03.017
Che, Y., Xia, Y., Liu, L., Li, A.-D., Yang, Y., and Zhang, T. (2019). Mobile Antibiotic Resistome in Wastewater Treatment Plants Revealed by Nanopore Metagenomic Sequencing. Microbiome 7, 44. doi:10.1186/s40168-019-0663-0
Chen, H., Bai, X., Jing, L., Chen, R., and Teng, Y. (2019). Characterization of Antibiotic Resistance Genes in the Sediments of an Urban River Revealed by Comparative Metagenomics Analysis. Sci. Total Environ. 653, 1513–1521. doi:10.1016/j.scitotenv.2018.11.052
Chen, H., Bai, X., Li, Y., Jing, L., Chen, R., and Teng, Y. (2019). Source Identification of Antibiotic Resistance Genes in a Peri-Urban River Using Novel crAssphage Marker Genes and Metagenomic Signatures. Water Res. 167, 115098. doi:10.1016/j.watres.2019.115098
Chen, Y.-r., Guo, X.-p., Feng, J.-n., Lu, D.-p., Niu, Z.-s., Tou, F.-y., et al. (2019). Impact of ZnO Nanoparticles on the Antibiotic Resistance Genes (ARGs) in Estuarine Water: ARG Variations and Their Association with the Microbial Community. Environ. Sci. Nano 6, 2405–2419. doi:10.1039/c9en00338j
Dang, C., Xia, Y., Zheng, M., Liu, T., Liu, W., Chen, Q., et al. (2020). Metagenomic Insights into the Profile of Antibiotic Resistomes in a Large Drinking Water Reservoir. Environ. Int. 136, 105449. doi:10.1016/j.envint.2019.105449
Das, B. K., Behera, B. K., Chakraborty, H. J., Paria, P., Gangopadhyay, A., Rout, A. K., et al. (2020). Metagenomic Study Focusing on Antibiotic Resistance Genes from the Sediments of River Yamuna. Gene 758, 144951. doi:10.1016/j.gene.2020.144951
de Nies, L., Lopes, S., Busi, S. B., Galata, V., Heintz-Buschart, A., Laczny, C. C., et al. (2021). PathoFact: a Pipeline for the Prediction of Virulence Factors and Antimicrobial Resistance Genes in Metagenomic Data. Microbiome 9, 49. doi:10.1186/s40168-020-00993-9
Harris, Z. N., Dhungel, E., Mosior, M., and Ahn, T.-H. (2019). Massive Metagenomic Data Analysis Using Abundance-Based Machine Learning. Biol. Direct 14, 12. doi:10.1186/s13062-019-0242-0
Holmes, A. H., Moore, L. S. P., Sundsfjord, A., Steinbakk, M., Regmi, S., Karkey, A., et al. (2016). Understanding the Mechanisms and Drivers of Antimicrobial Resistance. The Lancet 387, 176–187. doi:10.1016/s0140-6736(15)00473-0
Hu, A., Wang, H., Li, J., Mulla, S. I., Qiu, Q., Tang, L., et al. (2020). Homogeneous Selection Drives Antibiotic Resistome in Two Adjacent Sub-watersheds, China. J. Hazard. Mater. 398, 122820. doi:10.1016/j.jhazmat.2020.122820
Hyatt, D., Chen, G.-L., Locascio, P. F., Land, M. L., Larimer, F. W., and Hauser, L. J. (2010). Prodigal: Prokaryotic Gene Recognition and Translation Initiation Site Identification. BMC Bioinformatics 11, 119. doi:10.1186/1471-2105-11-119
Iwu, C. D., Korsten, L., and Okoh, A. I. (2020). The Incidence of Antibiotic Resistance within and beyond the Agricultural Ecosystem: A Concern for Public Health. MicrobiologyOpen 9, e1035. doi:10.1002/mbo3.1035
Jansson, J. K., and Hofmockel, K. S. (2018). The Soil Microbiome - from Metagenomics to Metaphenomics. Curr. Opin. Microbiol. 43, 162–168. doi:10.1016/j.mib.2018.01.013
Jing, R., and Yan, Y. (2020). Metagenomic Analysis Reveals Antibiotic Resistance Genes in the Bovine Rumen. Microb. Pathogenesis 149, 104350. doi:10.1016/j.micpath.2020.104350
Knights, D., Kuczynski, J., Charlson, E. S., Zaneveld, J., Mozer, M. C., Collman, R. G., et al. (2011). Bayesian Community-wide Culture-independent Microbial Source Tracking. Nat. Methods 8, 761–763. doi:10.1038/nmeth.1650
Krawczyk, P. S., Lipinski, L., and Dziembowski, A. (2018). PlasFlow: Predicting Plasmid Sequences in Metagenomic Data Using Genome Signatures. Nucleic Acids Res. 46, e35. doi:10.1093/nar/gkx1321
Leplae, R., Hebrant, A., Wodak, S. J., and Toussaint, A. (2004). ACLAME: a CLAssification of Mobile Genetic Elements. Nucleic Acids Res. 32, D45–D49. doi:10.1093/nar/gkh084
Leplae, R., Lima-Mendez, G., and Toussaint, A. (2010). ACLAME: a CLAssification of Mobile Genetic Elements, Update 2010. Nucleic Acids Res. 38, D57–D61. doi:10.1093/nar/gkp938
Li, B., Qiu, Y., Song, Y., Lin, H., and Yin, H. (2019). Dissecting Horizontal and Vertical Gene Transfer of Antibiotic Resistance Plasmid in Bacterial Community Using Microfluidics. Environ. Int. 131, 105007. doi:10.1016/j.envint.2019.105007
Li, B., Yang, Y., Ma, L., Ju, F., Guo, F., Tiedje, J. M., et al. (2015). Metagenomic and Network Analysis Reveal Wide Distribution and Co-occurrence of Environmental Antibiotic Resistance Genes. Isme J. 9, 2490–2502. doi:10.1038/ismej.2015.59
Li, D., Luo, R., Liu, C.-M., Leung, C.-M., Ting, H.-F., Sadakane, K., et al. (2016). MEGAHIT v1.0: A Fast and Scalable Metagenome Assembler Driven by Advanced Methodologies and Community Practices. Methods 102, 3–11. doi:10.1016/j.ymeth.2016.02.020
Li, L.-G., Huang, Q., Yin, X., and Zhang, T. (2020). Source Tracking of Antibiotic Resistance Genes in the Environment - Challenges, Progress, and Prospects. Water Res. 185, 116127. doi:10.1016/j.watres.2020.116127
Li, L. G., Yin, X., and Zhang, T. (2018). Tracking Antibiotic Resistance Gene Pollution from Different Sources Using Machine-Learning Classification. Microbiome 6, 93–12. doi:10.1186/s40168-018-0480-x
Li, X., Wu, Z., Dang, C., Zhang, M., Zhao, B., Cheng, Z., et al. (2021). A Metagenomic-Based Method to Study Hospital Air Dust Resistome. Chem. Eng. J. 406, 126854. doi:10.1016/j.cej.2020.126854
Li, Y., Xu, Z., Han, W., Cao, H., Umarov, R., Yan, A., et al. (2021). HMD-ARG: Hierarchical Multi-Task Deep Learning for Annotating Antibiotic Resistance Genes. Microbiome 9, 40. doi:10.1186/s40168-021-01002-3
Lin, Z.-J., Zhou, Z.-C., Zhu, L., Meng, L.-X., Shuai, X.-Y., Sun, Y.-J., et al. (2021). Behavior of Antibiotic Resistance Genes in a Wastewater Treatment Plant with Different Upgrading Processes. Sci. Total Environ. 771, 144814. doi:10.1016/j.scitotenv.2020.144814
Liu, B., and Pop, M. (2009). ARDB--Antibiotic Resistance Genes Database. Nucleic Acids Res. 37, D443–D447. doi:10.1093/nar/gkn656
Liu, W., Ling, N., Guo, J., Ruan, Y., Wang, M., Shen, Q., et al. (2021). Dynamics of the Antibiotic Resistome in Agricultural Soils Amended with Different Sources of Animal Manures over Three Consecutive Years. J. Hazard. Mater. 401, 123399. doi:10.1016/j.jhazmat.2020.123399
Llorens, C., Futami, R., Covelli, L., Domínguez-Escribá, L., Viu, J. M., Tamarit, D., et al. (2010). The Gypsy Database (GyDB) of mobile Genetic Elements: Release 2.0. Nucleic Acids Res. 39, D70–D74. doi:10.1093/nar/gkq1061
Ma, L., Li, B., Jiang, X.-T., Wang, Y.-L., Xia, Y., Li, A.-D., et al. (2017). Catalogue of Antibiotic Resistome and Host-Tracking in Drinking Water Deciphered by a Large Scale Survey. Microbiome 5, 154. doi:10.1186/s40168-017-0369-0
Manage, P. M. (2018). Heavy Use of Antibiotics in Aquaculture: Emerging Human and Animal Health Problems - A Review. Sri Lanka J. Aquat. 23 (1), 13–27. doi:10.4038/sljas.v23i1.7543
Manaia, C. M. (2017). Assessing the Risk of Antibiotic Resistance Transmission from the Environment to Humans: Non-direct Proportionality between Abundance and Risk. Trends Microbiol. 25, 173–181. doi:10.1016/j.tim.2016.11.014
Marchesi, J. R., Adams, D. H., Fava, F., Hermes, G. D. A., Hirschfield, G. M., Hold, G., et al. (2016). The Gut Microbiota and Host Health: a New Clinical Frontier. Gut 65, 330–339. doi:10.1136/gutjnl-2015-309990
McArthur, A. G., Waglechner, N., Nizam, F., Yan, A., Azad, M. A., Baylay, A. J., et al. (2013). The Comprehensive Antibiotic Resistance Database. Antimicrob. Agents Chemother. 57, 3348–3357. doi:10.1128/aac.00419-13
McGhee, J. J., Rawson, N., Bailey, B. A., Fernandez-Guerra, A., Sisk-Hackworth, L., and Kelley, S. T. (2020). Meta-SourceTracker: Application of Bayesian Source Tracking to Shotgun Metagenomics. PeerJ 8, e8783. doi:10.7717/peerj.8783
Miao, L., and Liu, Z. (2018). Microbiome Analysis and -omics Studies of Microbial Denitrification Processes in Wastewater Treatment: Recent Advances. Sci. China Life Sci. 61, 753–761. doi:10.1007/s11427-017-9228-2
Moura, A., Soares, M., Pereira, C., Leitão, N., Henriques, I., and Correia, A. (2009). INTEGRALL: a Database and Search Engine for Integrons, Integrases and Gene Cassettes. Bioinformatics 25, 1096–1098. doi:10.1093/bioinformatics/btp105
Pan, X., Lin, L., Zhang, W., Dong, L., and Yang, Y. (2020). Metagenome Sequencing to Unveil the Resistome in a Deep Subtropical lake on the Yunnan-Guizhou Plateau, China. Environ. Pollut. 263, 114470. doi:10.1016/j.envpol.2020.114470
Pärnänen, K., Karkman, A., Hultman, J., Lyra, C., Bengtsson-Palme, J., Larsson, D. G. J., et al. (2018). Maternal Gut and Breast Milk Microbiota Affect Infant Gut Antibiotic Resistome and mobile Genetic Elements. Nat. Commun. 9, 3891–3911. doi:10.1038/s41467-018-06393-w
Qian, X., Gunturu, S., Guo, J., Chai, B., Cole, J. R., Gu, J., et al. (2021). Metagenomic Analysis Reveals the Shared and Distinct Features of the Soil Resistome across Tundra, Temperate Prairie, and Tropical Ecosystems. Microbiome 9, 108–113. doi:10.1186/s40168-021-01047-4
Qian, X., Gunturu, S., Sun, W., Cole, J. R., Norby, B., Gu, J., et al. (2021). Long-read Sequencing Revealed Cooccurrence, Host Range, and Potential Mobility of Antibiotic Resistome in Cow Feces. Proc. Natl. Acad. Sci. USA 118, e2024464118. doi:10.1073/pnas.2024464118
Qiao, M., Ying, G.-G., Singer, A. C., and Zhu, Y.-G. (2018). Review of Antibiotic Resistance in China and its Environment. Environ. Int. 110, 160–172. doi:10.1016/j.envint.2017.10.016
Raza, S., Jo, H., Kim, J., Shin, H., Hur, H.-G., and Unno, T. (2021). Metagenomic Exploration of Antibiotic Resistome in Treated Wastewater Effluents and Their Receiving Water. Sci. Total Environ. 765, 142755. doi:10.1016/j.scitotenv.2020.142755
Sanderson, H., Fricker, C., Brown, R. S., Majury, A., and Liss, S. N. (2016). Antibiotic Resistance Genes as an Emerging Environmental Contaminant. Environ. Rev. 24, 205–218. doi:10.1139/er-2015-0069
Sekizuka, T., Itokawa, K., Tanaka, R., Hashino, M., Yatsu, K., and Kuroda, M. (2020). Characterization of Urban Wastewater Treatment Plant Effluent from Tokyo Using Metagenomics and β-lactam-resistant Enterobacteriaceae Isolates. Res. Square.
Shenhav, L., Thompson, M., Joseph, T. A., Briscoe, L., Furman, O., Bogumil, D., et al. (2019). FEAST: Fast Expectation-Maximization for Microbial Source Tracking. Nat. Methods 16, 627–632. doi:10.1038/s41592-019-0431-x
Siguier, P., Perochon, J., Lestrade, L., Mahillon, J., and Chandler, M. (2006). ISfinder: the Reference centre for Bacterial Insertion Sequences. Nucleic Acids Res. 34, D32–D36. doi:10.1093/nar/gkj014
Stalder, T., Press, M. O., Sullivan, S., Liachko, I., and Top, E. M. (2019). Linking the Resistome and Plasmidome to the Microbiome. Isme J. 13, 2437–2446. doi:10.1038/s41396-019-0446-4
Valdes, A. M., Walter, J., Segal, E., and Spector, T. D. (2018). Role of the Gut Microbiota in Nutrition and Health. Bmj 361, k2179. doi:10.1136/bmj.k2179
Varani, A. M., Siguier, P., Gourbeyre, E., Charneau, V., and Chandler, M. (2011). ISsaga Is an Ensemble of Web-Based Methods for High Throughput Identification and Semi-automatic Annotation of Insertion Sequences in Prokaryotic Genomes. Genome Biol. 12, R30. doi:10.1186/gb-2011-12-3-r30
Vrancianu, C. O., Popa, L. I., Bleotu, C., and Chifiriuc, M. C. (2020). Targeting Plasmids to Limit Acquisition and Transmission of Antimicrobial Resistance. Front. Microbiol. 11, 761. doi:10.3389/fmicb.2020.00761
Wang, Y.-F., Qiao, M., Zhu, D., and Zhu, Y.-G. (2020). Antibiotic Resistance in the Collembolan Gut Microbiome Accelerated by the Nonantibiotic Drug Carbamazepine. Environ. Sci. Technol. 54, 10754–10762. doi:10.1021/acs.est.0c03075
Wang, Z., Han, M., Li, E., Liu, X., Wei, H., Yang, C., et al. (2020). Distribution of Antibiotic Resistance Genes in an Agriculturally Disturbed lake in China: Their Links with Microbial Communities, Antibiotics, and Water Quality. J. Hazard. Mater. 393, 122426. doi:10.1016/j.jhazmat.2020.122426
Wei, Z., Wu, Y., Feng, K., Yang, M., Zhang, Y., Tu, Q., et al. (2019). ARGA, a Pipeline for Primer Evaluation on Antibiotic Resistance Genes. Environ. Int. 128, 137–145. doi:10.1016/j.envint.2019.04.030
Xia, Y., Li, A.-D., Deng, Y., Jiang, X.-T., Li, L.-G., and Zhang, T. (2017). MinION Nanopore Sequencing Enables Correlation between Resistome Phenotype and Genotype of Coliform Bacteria in Municipal Sewage. Front. Microbiol. 8, 2105. doi:10.3389/fmicb.2017.02105
Xiang, Q., Zhu, D., Giles, M., Neilson, R., Yang, X.-R., Qiao, M., et al. (2020). Agricultural Activities Affect the Pattern of the Resistome within the Phyllosphere Microbiome in Peri-Urban Environments. J. Hazard. Mater. 382, 121068. doi:10.1016/j.jhazmat.2019.121068
Yang, Y., Jiang, X., Chai, B., Ma, L., Li, B., Zhang, A., et al. (2016). ARGs-OAP: Online Analysis Pipeline for Antibiotic Resistance Genes Detection from Metagenomic Data Using an Integrated Structured ARG-Database. Bioinformatics 32, 2346–2351. doi:10.1093/bioinformatics/btw136
Yang, Y., Song, W., Lin, H., Wang, W., Du, L., and Xing, W. (2018). Antibiotics and Antibiotic Resistance Genes in Global Lakes: a Review and Meta-Analysis. Environ. Int. 116, 60–73. doi:10.1016/j.envint.2018.04.011
Ye, C., Hill, C. M., Wu, S., Ruan, J., and Ma, Z. S. (2016). DBG2OLC: Efficient Assembly of Large Genomes Using Long Erroneous Reads of the Third Generation Sequencing Technologies. Sci. Rep. 6, 31900–31909. doi:10.1038/srep31900
Yin, X., Deng, Y., Ma, L., Wang, Y., Chan, L. Y. L., and Zhang, T. (2019). Exploration of the Antibiotic Resistome in a Wastewater Treatment Plant by a Nine-Year Longitudinal Metagenomic Study. Environ. Int. 133, 105270. doi:10.1016/j.envint.2019.105270
Yin, X., Jiang, X.-T., Chai, B., Li, L., Yang, Y., Cole, J. R., et al. (2018). ARGs-OAP v2.0 with an Expanded SARG Database and Hidden Markov Models for Enhancement Characterization and Quantification of Antibiotic Resistance Genes in Environmental Metagenomes. Bioinformatics 34, 2263–2270. doi:10.1093/bioinformatics/bty053
Zhang, A. N., Hou, C. J., Negi, M., Li, L. G., and Zhang, T. (2020). Online Searching Platform for the Antibiotic Resistome in Bacterial Tree of Life and Global Habitats. FEMS Microbiol. Ecol. 96 (7), fiaa107. doi:10.1093/femsec/fiaa107
Zhang, T., Yang, Y., and Pruden, A. (2015). Effect of Temperature on Removal of Antibiotic Resistance Genes by Anaerobic Digestion of Activated Sludge Revealed by Metagenomic Approach. Appl. Microbiol. Biotechnol. 99, 7771–7779. doi:10.1007/s00253-015-6688-9
Zhao, R., Feng, J., Liu, J., Fu, W., Li, X., and Li, B. (2019). Deciphering of Microbial Community and Antibiotic Resistance Genes in Activated Sludge Reactors under High Selective Pressure of Different Antibiotics. Water Res. 151, 388–402. doi:10.1016/j.watres.2018.12.034
Zhao, R., Yu, K., Zhang, J., Zhang, G., Huang, J., Ma, L., et al. (2020). Deciphering the Mobility and Bacterial Hosts of Antibiotic Resistance Genes under Antibiotic Selection Pressure by Metagenomic Assembly and Binning Approaches. Water Res. 186, 116318. doi:10.1016/j.watres.2020.116318
Zhou, Z.-C., Feng, W.-Q., Han, Y., Zheng, J., Chen, T., Wei, Y.-Y., et al. (2018). Prevalence and Transmission of Antibiotic Resistance and Microbiota between Humans and Water Environments. Environ. Int. 121, 1155–1161. doi:10.1016/j.envint.2018.10.032
Zhou, Z., Xu, L., Zhu, L., Liu, Y., Shuai, X., Lin, Z., et al. (2021). Metagenomic Analysis of Microbiota and Antibiotic Resistome in Household Activated Carbon Drinking Water Purifiers. Environ. Int. 148, 106394. doi:10.1016/j.envint.2021.106394
Keywords: antibiotics resistance genes, metagenomic data, bioinformatic tools, databases, source tracking
Citation: Peng Z, Mao Y, Zhang N, Zhang L, Wang Z and Han M (2021) Utilizing Metagenomic Data and Bioinformatic Tools for Elucidating Antibiotic Resistance Genes in Environment. Front. Environ. Sci. 9:757365. doi: 10.3389/fenvs.2021.757365
Received: 12 August 2021; Accepted: 18 October 2021;
Published: 29 October 2021.
Edited by:
Oladele Ogunseitan, University of California, Irvine, United StatesReviewed by:
Shuying Li, Zhejiang University, ChinaCopyright © 2021 Peng, Mao, Zhang, Zhang, Wang and Han. This is an open-access article distributed under the terms of the Creative Commons Attribution License (CC BY). The use, distribution or reproduction in other forums is permitted, provided the original author(s) and the copyright owner(s) are credited and that the original publication in this journal is cited, in accordance with accepted academic practice. No use, distribution or reproduction is permitted which does not comply with these terms.
*Correspondence: Maozhen Han, aGFubXpAYWhtdS5lZHUuY24=; Zhi Wang, endhbmdAYXBtLmFjLmNu
†These authors have contributed equally to this work
Disclaimer: All claims expressed in this article are solely those of the authors and do not necessarily represent those of their affiliated organizations, or those of the publisher, the editors and the reviewers. Any product that may be evaluated in this article or claim that may be made by its manufacturer is not guaranteed or endorsed by the publisher.
Research integrity at Frontiers
Learn more about the work of our research integrity team to safeguard the quality of each article we publish.