- 1Department of Geography and Environmental Sciences, Northumbria University, Newcastle upon Tyne, United Kingdom
- 2Department of Geology and Geoenvironment, National and Kapodistrian University of Athens, Athens, Greece
- 3The Hub for Biotechnology in the Built Environment, Department of Applied Sciences, Faculty of Health and Life Sciences, Northumbria University, Newcastle upon Tyne, United Kingdom
- 4School of Computing, Engineering and Physical Sciences, University of the West of Scotland, Scotland, United Kingdom
- 5Instituto de Ciências da Terra, Polo da Universidade do Minho, Campus de Gualtar, Braga, Portugal
- 6Department of Applied Sciences, Faculty of Health and Life Sciences, Northumbria University, Newcastle upon Tyne, United Kingdom
- 7Ecology and Environment Research Centre, Department of Natural Science, Manchester Metropolitan University, Manchester, United Kingdom
- 8Exposures and Biomonitoring Division, Environmental Health Science and Research Bureau, Ottawa, ON, Canada
- 9British Geological Survey, Nottingham, United Kingdom
Our indoor microbiome consists of a wide range of microbial taxa. Whilst many of these microbes are benign, some are beneficial, some harmful, yet our knowledge of the spatial heterogeneity of bacterial assemblages in our residential environment remains limited. To investigate the existence of a common core house dust bacterial microbiome we selected household vacuum dusts, collected through a citizen science approach, from homes across two bioclimatic regions (UK, Oceanic/Maritime and Greece, Mediterranean). Following the extraction of DNA from each dust sample, we targeted the bacterial 16S rRNA gene using Illumina NextSeq sequencing. PERMANOVA analysis of the microbial communities at family level grouped samples within their distinct bioclimatic region and SIMPER analysis at genus level identified the statistically significant taxa responsible for driving diversity between these groups. A “common to all” core house dust microbiome consisted of Acinetobacter, Massalia, Rubellimicrobium, Sphingomonas and Staphylococcus; genera typically associated with human occupancy and common environmental sources. Additionally, a “unique location specific” microbiome was identified, reflective of the bioclimatic region. The Greek dusts indicated a lower average diversity than the UK house dusts, with a high abundance of Rhizobiaceae in the Greek samples. Our study highlights citizen science as a powerful approach to access the indoor residential environment, at scale, and establishes the existence of a “core” house dust microbiome independent of bioclimatic region.
Introduction
As over 90% of the average day is spent indoors, in our homes, workplaces and/or travel, indoor exposure to dust and its intrinsic biological, physical and chemical entities represents one of modern society’s greatest exposures to potentially harmful substances. A significant component of house dust is of biological origin, including microbes and their secretions, dead skin cells, dander, hair and respiratory secretions (Morawska and Salthammer, 2003). The ingress of outdoor particulate matter, including allergens, brought inside on clothes, footwear, pets or by the wind, and that originating indoors from cooking, smoking and the wear and tear of furnishings, are often major contributing sources in indoor dust, as well as a varied array of inorganic and organic chemicals from sources including cleaning products and building materials (Blanchard et al., 2020; Salthammer, 2020). This indoor dust serves as a reservoir for environmental exposure to microbial communities, many of which are benign, some are beneficial, whilst some exhibit pathogenicity. Indeed, the home environment presents an intricate microbial ecosystem inhabited by a typically diverse community of microorganisms, with 500–1,000 different species being reported in house dust (Lax et al., 2014; Barberán et al., 2015; Shan et al., 2019). While many studies describe spatial patterns of bacterial diversity in a specific geographic region or environmental habitat (such as soil, water, or sediment), our knowledge of indoor residential bacterial biodiversity, biogeography and their associated drivers are still poorly understood.
Local climate and outdoor environmental conditions play a role in the development of the indoor microbiome (Shan et al., 2019). Indoor fungal communities are known to be strongly influenced by the outdoor environment, with clear geographic patterns reported in the literature (Amend et al., 2010), but the relationship is less clear for indoor microbial communities (Meadow et al., 2014; Barberán et al., 2015). Anthropogenic factors influencing indoor microbial communities include variations in occupancy (both humans and animals), physiological differences in the occupants (e.g. age, gender), lifestyle differences (e.g. diet, living conditions), as well as activity patterns within the home environment (Leung and Lee, 2016). Some studies report Gram-positive phyla such as Firmicutes and Actinobacteria in greater abundance indoors (Täubel et al., 2009; Hanson et al., 2016; Loo et al., 2018), whilst other investigations observe the inverse trend with members of the Gram-negative phylum Proteobacteria in greater numbers (Barberán et al., 2015).
Whilst non-occupational exposure to a range of metal(loid)s and organic contaminants in house dust are a known risk factor for a range of diseases and poor health outcomes (Salthammer, 2020), we know far less about the microbial communities associated with our indoor home environments, and their interaction/impacts on human health. The majority of research has focussed on selected microbial taxa, investigating disease transmission and pathogenesis within indoor environments, with a focus on impacts on the gastrointestinal (GI) tract and atopy (Haysom and Sharp, 2003). For example, Bacteroides spp. (e.g. B. fragilis) found in indoor dusts can interfere with gut colonization by suppressing anti-microbial immune responses (Round et al., 2011; Hanson et al., 2016). However, our interactions with the micro-organisms native to the indoor environment may also be beneficial, with recent research indicating an inverse association between the diversity of these microorganisms and the prevalence of various respiratory conditions of a non-communicable nature such as wheeze, allergic rhinitis and asthma, as well as certain skin conditions like atopic eczema (Birzele et al., 2016; Lee et al., 2018).
The SARS-CoV-2 pandemic has resulted in both tiered and national lockdowns across the globe, with an increased risk management strategy emphasised by Governments’ advising regular handwashing, surface sanitation, home-schooling and directives to work from home where possible (Public Health England, 2020). These mitigation strategies have raised concerns that increased exposure to the indoor microbiome, in tandem with reduced exposure to microbial diversity in the ambient environment, could exacerbate pre-existing health conditions (Edge et al., 2011; Dannemiller et al., 2014; Juel Holst et al., 2020). Our knowledge, however, of the diversity and spatial heterogeneity of bacterial assemblages in our residential environment remains limited and we need to better characterise the diversity, similarities and differences in this microbiome between households to assess the benefits or hazard posed by our indoor house dust microbiome and to understand our exposure. Citizen science is a potentially powerful approach to both raise-awareness and to access the residential indoor environment, at scale. Our study is part of the Home Biome project (www.mapmyenvironment.com) a global study investigating a range of biological and chemical constituents in house dust samples collected by citizens themselves. In this proof-of-concept study we focused on three hypotheses. First, if the indoor residential environment provides a distinctive habitat or biome (the “home biome”) we would expect to see a common core house dust microbiome represented in the vacuum dust across all homes, regardless of bioclimatic region, building type or occupancy. Second, as global bioclimatic regions influence the fauna and flora of that region we expect to see geospatial dependency, or a region-unique core microbiome typified by bioclimatic zone. Third, citizen collected samples from regular household vacuum cleaners can provide a suitable medium for investigation of the microbial communities present in residential homes. To test these hypotheses, we focussed on the bacterial richness, composition and diversity in citizen collected vacuum dust samples from residential homes across two countries in two distinct bioclimatic regions (UK, Oceanic zone, and Greece, Mediterranean zone). Our findings have important implications for the collaboration between citizens and scientists for the development of evidence-based management strategies to modify potential benefits and hazards posed by our indoor house dust microbiome.
Methods
Experimental Design
Several methods have been employed in environmental and public health research for the acquisition of dust samples from residential dwellings. Despite the variety of approaches, the most commonly used techniques are the utilisation of dry and/or wet wipes and vacuum cleaners, typically with researcher-led sampling campaigns (e.g. Rasmussen et al., 2013). In the Home Biome study, we adopted a different approach, that of a citizen-led approach whereby participants were asked to submit either their vacuum bag, or a sub-sample. Written sampling guidance and an online video supported the citizen sampling (details in Supplementary Text S1). Whilst vacuum samples acquired as part of large-scale citizen-led campaigns have the disadvantage of being of unknown age and exact provenance, and collected using a wide array of vacuum devices, research has shown that vacuum cleaner waste is a good surrogate for human exposure to contaminants in indoor dust (Barnes et al., 2013). Indeed vacuum dust samples have been shown to compare well with measurements made by other sampling techniques, as well as providing samples that are typically reflective of an entire residential unit (Colt et al., 2008).
Twenty-eight vacuum dust samples were selected for this investigation (one vacuum sample from each home): twenty-one samples from a national campaign within the UK, representing a regional spread across the UK (including Northern Ireland), and seven samples from Greece selected to provide a different bioclimatic zone. Both positive and negative reagent controls were included to ensure sterility throughout the processing and sequencing steps, and a randomly selected sample was run in triplicate (DSUK179).
A wide range of anthropogenic factors are likely to affect the indoor microbiome and to capture some of this heterogeneity we developed an online questionnaire which citizens were asked to complete. The questionnaire was split into two sections dealing with 1) the main characteristics and activities of the household occupants, such as the number, gender and ages of occupants, pets, the areas of usage of the vacuum cleaner, whether shoes that are worn outside the house were worn indoors, smoking, hobbies, and 2) the residential environment, such as building type and age, main type of floor covering, access to outside space and the nature of local environment, etc.
Sample Processing and DNA Extraction
On receipt, the samples were logged and stored, unopened, in the dark at room temperature. Sample bags were opened and the contents sieved in a Class II microbiological safety cabinet using a one-use-only 250 μm nylon mesh filter to remove larger particulates and fibrous material; the mesh was UV sterilised for >30 min prior to use. The <250 μm particle size fraction is the fraction commonly selected for the chemical analysis of dusts, sediments and soils as this fraction is more likely to adhere to hands or food produce and be transferred by ingestion through hand-to-mouth contact compared to larger size fractions (Duggan et al., 1986). Sieved particulates were stored in 50 ml sterile falcon tubes until DNA extraction.
High throughput isolation of microbial genomic DNA from each dust sample was performed as per manufacturer’s instructions using a DNeasy 96 PowerSoil Pro Kit (384) (QIAGEN). The DNeasy 96 PowerSoil Pro Kit includes an inhibitor removal step to eliminate inhibitors commonly found in soil and environmental samples. DNA samples were stored at −20°C until sequencing.
Sequencing and Data Processing
Taxonomic investigation of bacteria was performed via paired-end sequencing of the V4 hypervariable region of the 16S rRNA gene using the 515-F (GTGCCAGCMGCCGCGGTAA) and 806-R (GGACTACHVGGGTWTCTAAT) primer pair, using a Illumina MiSeq 250 × 2 chemistry approach. Raw sequences were quality checked and trimmed using FastQC/MultiQC and BBDuk (Andrews, 2010; B B Map, 2020). Paired reads were classified using Kraken2 (Wood et al., 2019) and Bracken (Lu et al., 2017) using the SILVA v138 database (Pruesse et al., 2007). Resulting sample report files were converted to BIOM (version 1.0) format (McDonald et al., 2012) using kraken-biom and imported into R (v4.0.3) (R Development Core Team, 2020) using the R package Phyloseq (McMurdie and Holmes, 2013). All plots, unless otherwise stated, were produced using GGPlot2 (Wickham, 2009) and organised using Cowplot (Wilke, 2020), GGPubr (Kassambara, 2020) and GGRepel (Slowikowski, 2020). Due to presence in reagent negative control, the genus Escherichia-Shigella was removed from all samples prior to analysis as we cannot differentiate its presence as being in the environment sampled or as a consequence of laboratory practise and/or contaminated reagents. After sequence filtering and quality control of the 28 house dust samples, a total of 730,735 sequence reads, with a median/average of 22,519/25,198 sequence reads per sample (min 8,695, max 45,370) were produced. This corresponded to 600 taxa. The relationship between number of operational taxonomic units (OTUs) and number of sequences presented is indicative of sufficient sampling depth, increasing sequencing depth further would provide diminishing returns on newly discovered OTUs. Controls and low read count samples (only one sample, DSUK182) were removed, followed by rarefaction (without replacement) to 90% of the minimum sample read count (7,826 reads per sample) to normalise the library size across the samples. DNA sequencing data consist of discrete counts of sequence reads and the total number of which is the library size (Cameron et al., 2020). Library sizes can vary greatly between samples and thus the samples were normalised to remove bias and false inferences due to variations in library size. After rarefaction, 599 unique taxa were obtained across all samples and only one OTU was no longer present in any sample after random subsampling.
Data Analyses
Due to the inherent complexity of the samples, initial abundance profiling was undertaken using relative abundance, calculated and plotted at the phylum level. For phylum level abundance profiling, phyla were included if abundance was greater than 0.5% in at least 25% of all samples, with all other phyla collected into an “other” category using the function aggregate_rare of the “Microbiome” R package (Lahti and Shetty, 2017). Although an indicator of overall trends, phylum level analysis may not offer sufficient resolution to infer significant differences in bacterial communities. As such the phylum level relative abundance profiles were further investigated at family level, including alpha (i.e. variation of phyla in a single sample) and beta (i.e. variation of phyla between samples) diversity indices, whilst genus level was used for determinations of similarity percentage analysis (SIMPER).
When the taxa resolution was increased to family level, families were included if abundance was greater than 2% in as least 50% of the samples, otherwise they were collated as “other”. Alpha diversity of the rarefied dataset at family level was quantified by using Shannon and Inverse Simpson diversity indices, both of which relate OTU richness and evenness and the total number of observed species. Alpha diversity metrics were calculated using Phyloseq and statistical significance of observed differences was evaluated using Wilcoxon rank sum test via the GGPubr package function stat_compare_means. Bray-Curtis beta diversity was calculated using vegdist and 2-dimension non-metric multidimensional scaling (NMDS) ordination of beta-diversity distance matrix produced using the function metaMDS as part of the “Vegan” R package (Oksanen et al., 2020). Statistical significance of differences in beta-diversity between locations was tested by permutational multivariate analysis of variance (PERMANOVA) with the adonis function (Oksanen et al., 2020).
To identify both the unique and the shared genera components of the UK and Greek core microbiomes SIMPER analysis was performed at the genus level using the simper function (part of the “Vegan” R package) through the simper_pretty wrapper script (Asteinberger9, 2020). Individual genera identified through this analysis were evaluated for location differences based on their relative abundances using a Kruskal-Wallis test via the kruskal. pretty wrapper script (Asteinberger9, 2020). Both the unique and the shared genera components of the core microbiomes in the UK and the Greek samples were visualised using the plot_core function of Microbiome (Lahti and Shetty, 2017). To be defined as part of the core microbiome in a location, genera had to be present at minimum 1% abundance in at least 75% of samples, as previously employed in microbiome analyses (Fahimipour et al., 2018; Rodrigues et al., 2018).
Results and Discussion
Abundance Profiling
At the phylum level the abundance profiles in the majority of samples from both countries are dominated by Proteobacteria, Actinobacteriota, Firmicutes and Bacteriodota, often accounting for >90% of the relative composition (Supplementary Figure S1). This correlates with other recent indoor microbiome analyses (Kembel et al., 2014; Gilbert and Stephens, 2018; Nygaard and Charnock, 2018) covering educational facilities, residential spaces and other common indoor environments, with the key phyla associated with human commensals, and commonly found environmental bacteria.
The phylum-level relative abundance profiles were further investigated at family level (Supplementary Figure S2). A wide bacterial diversity is evident within each dust sample. Our study focussed on dust samples provided by participants from vacuumed indoor locations within their own homes. Thus, rather than restricting sampling to specific surfaces, in a specific room, our results reflect the core microbiome across all the vacuumed locations of a participant’s home with the wide bacterial diversity suggesting the vacuum dust sample does indeed represent a sample from across a range of environments with the home. The top 8 families identified across the whole dataset include Acetobacteraceae, Chroococcidiopsaceae, Nocardioidaceae, Moraxellaceae, Rhodobacteraceae, Sphingomonadaceae, Staphylococcaceae and Streptococcaceae. The metadata captured by our household questionnaire indicates that a range of different home environments were represented by the dust samples (48% of homes were semi-detached, 37% detached and 15% units or flats; >70% were brick built; 48% were less than 50 years old, with 11% older than 100 years; 67% of households had 3 or more people living in the property, 33% had pets (all UK) and 19% had smokers), however the limited sample size within each sub-category prevented robust analysis of the influences of these factors on the home microbiome as part of this study (Supplementary Table S1). Whilst characteristics of the dwelling, the occupants and their lifestyles, airflow and exchange of material across the indoor-outdoor interface are all likely to alter the community composition, these 8 most abundant families compare with the findings of previous studies across a range of indoor microbiomes and human-associated microbiomes (Barberán et al., 2015; Ding et al., 2020), re-enforcing the link between humans and the bacterial ecology of the indoor microbiome.
Outlier individuals include Prevotellacae (29.46% relative abundance in DSUK013) and Enterobacteriaceae (23.39% relative abundance in DSUK167), both commonly human associated groups, whilst the relative abundance data for the Greek samples appears to be skewed by the presence of Rhizobiaceae accounting for up to 50.12% relative abundance in DSGR144, with a group average of 19.20%. These outliers are specific to certain samples and have likely arisen from specific factors associated with the individual home. This warrants further investigation as we now move to scale up this study globally.
Alpha and Beta and Diversity
Family level alpha diversity distribution of samples, based upon location (UK vs. Greece), was performed and Wilcoxon rank-sum pairwise comparisons identified significant difference between the two countries (Wilcoxon, p = 0.00023 Shannon diversity indices and Wilcoxon, p = 0.00072 Inverse Simpson diversity indices; Supplementary Figure S3). Both the Shannon and Inverse Simpson diversity indices suggest well balanced UK communities with regards to abundance and diversity, whilst the Greek samples indicated a lower average diversity (Supplementary Table S2) and may suggest some degree of skewness in the dataset, likely due to a high abundance of Rhizobiaceae_uncultured in the samples.
To investigate the variation in taxonomic abundance profiles between the different samples, a 2-dimension NMDS ordination of beta-diversity distance matrix was performed using a Bray-Curtis dissimilarity index (Figure 1). NMDS ordination functions use the proximity between objects, which corresponds to their similarity, to differentiate between samples. Statistically significant differences were observed (PERMANOVA F-value: 3.5807, R-squared: 0.11709, p-value 0.001; NMDS Stress = 0.144). Figure 1 clearly differentiates the samples and clusters according to the location metadata grouping of UK vs. Greece.
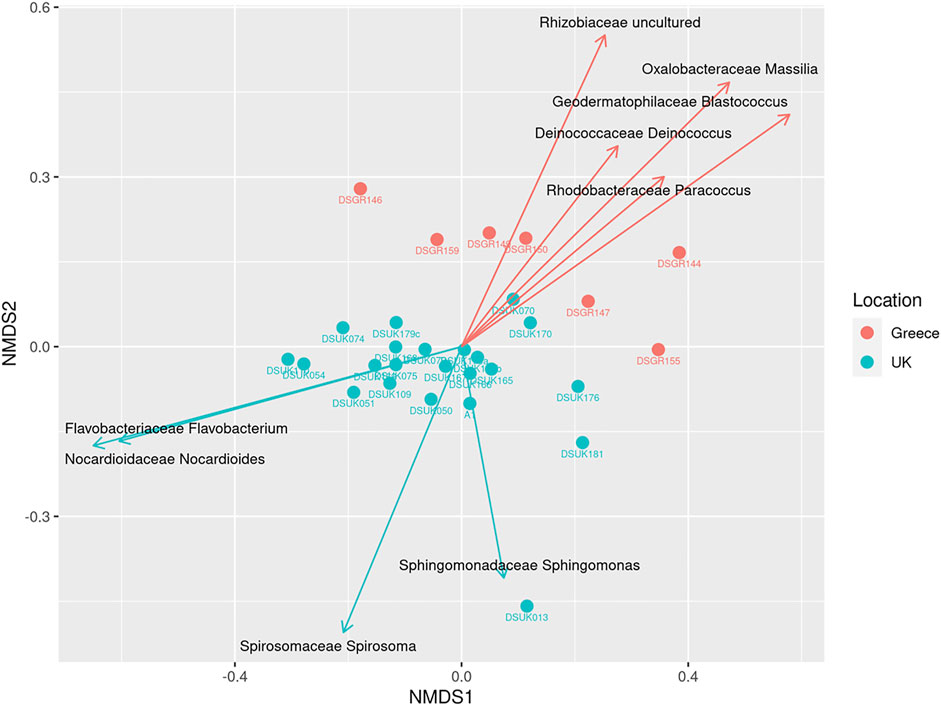
FIGURE 1. Beta Diversity NMDS bi-plot using Bray distance; vector lines show FDR-adjusted statistically significant taxa that contribute to ordination. Samples are grouped by location.
Characterization of a Common and a Region-Specific Core Indoor Microbiome
With observable trends apparent in the beta diversity identified using PERMANOVA, the effect of location was further explored at genus level using FDR-adjusted statistical methods. The contribution of individual OTUs to the genus level Bray-Curtis beta diversity was performed using SIMPER analysis to categorise most abundant OTUs using a pairwise comparison of the UK vs. Greece dataset at the genus level. SIMPER analysis cumulatively accounts 100% of the variation between the comparison of the UK vs. Greece, as identified using the Bray-Curtis measures. Table 1 lists 20 taxa responsible for >1% variation between the two countries, of which 9 taxa were identified to be statistically significant by comparison of OTU relative abundance by location metadata using Kruskal-Wallis rank sum testing with additional FDR-adjustments. To evaluate the genera contribution to ordination, the Vegan function envfit (999 permutations) was used to add species-loading vectors to the NMDS biplot (Figure 1), with vectors shown for those genera determined to be FDR-adjusted statistically significant from Table 1.
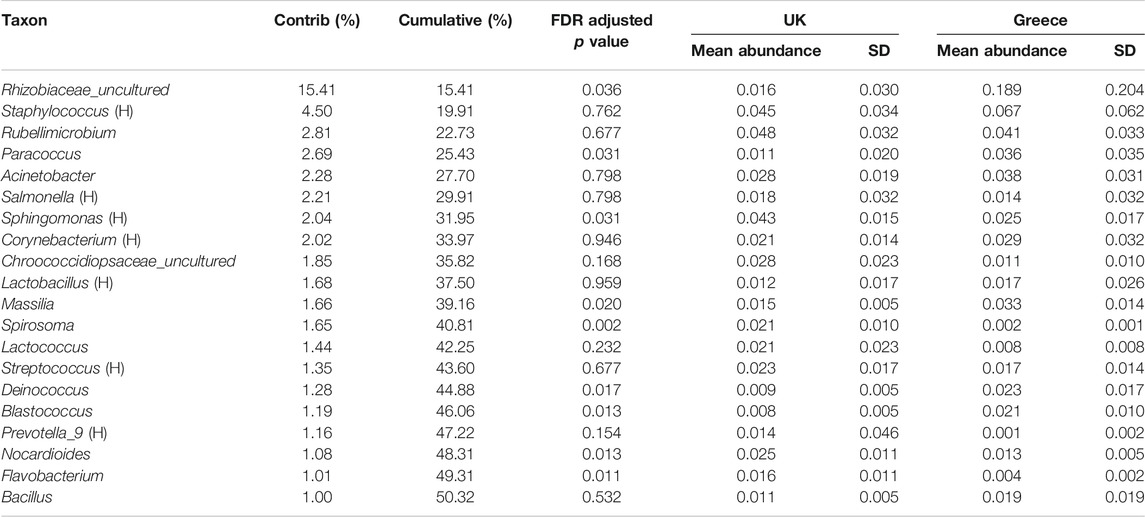
TABLE 1. SIMPER analysis of significant taxa driving diversity at genus level. Significance testing performed using Kruskal-Wallis one-way analysis of variance accounting for false discovery rates. Greyed out rows fail to meet the statistical significance cut off value of p < 0.05. H in parentheses denotes a commonly human associated Genus.
These FDR adjusted statistically significant taxa are presented in Figure 2, highlighting the relative abundance profiles between location groups (UK vs. Greece). The taxon labelled Rhizobiaceae_uncultured by the SILVA database appears to be the single most abundant bacteria responsible for diversity between samples contributing 15.41% of the dissimilarity, with other individual taxa responsible for not more than 4.50% dissimilarity. Notably, this taxon is not described in the family level relative abundance profiling described earlier (Supplementary Figure S2) but is considered during the examination of notable outliers. As abundance values are skewed from the high prevalence of this taxa in only a select number of Greek samples, in combination with low prevalence within UK samples, Rhizobiaceae_uncultured is not considered a member of the overall most abundant taxa.
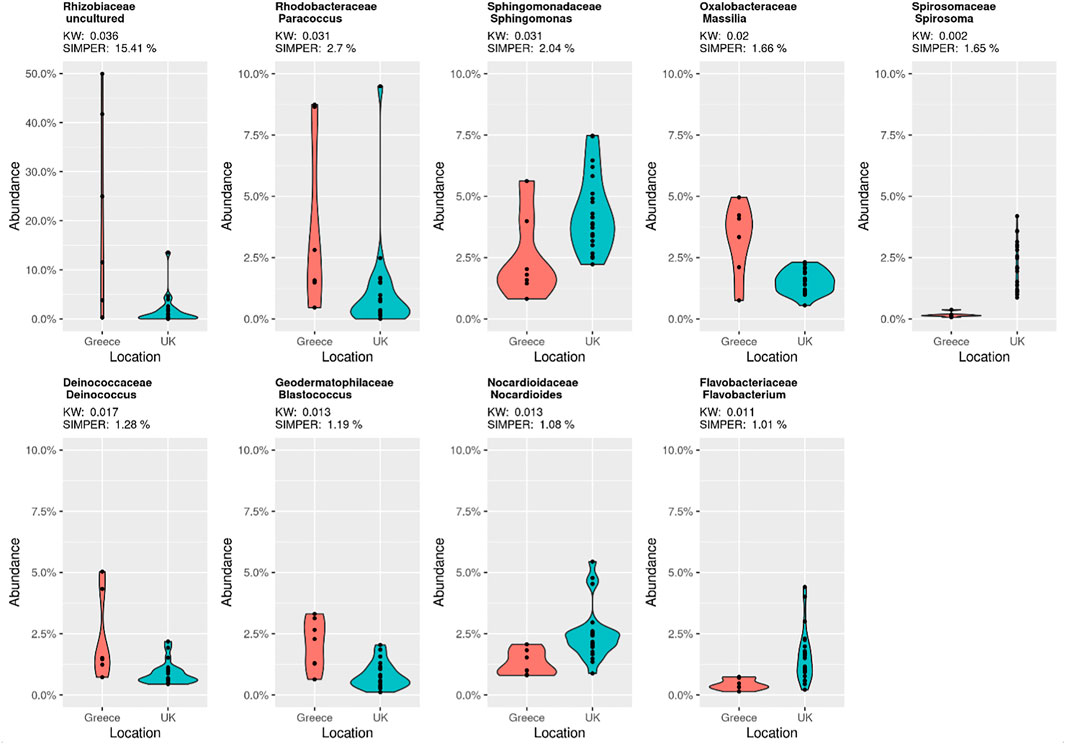
FIGURE 2. SIMPER analysis violin plots showing the statistically significant FDR adjusted p value taxa from Table 1. Contributing SIMPER and Kruskal Wallis (KW) values are displayed for each taxon, and by country.
An investigation into the core taxa was performed using a robust taxa prevalence threshold of 75% at a 1% detection (Figure 3), a threshold previously recommended (Fahimipour et al., 2018; Rodrigues et al., 2018), with the aim to identify both a set of genera common to all of the indoor dust samples (a shared common core microbiome), and also a set of genera that are unique to either all of the UK samples, or to all of the Greek samples (a unique core microbiome). To preserve the abundance values of the core microbiome between locations, the shared core microbiome was plotted independently in addition to location specific unique taxa (Figure 3).
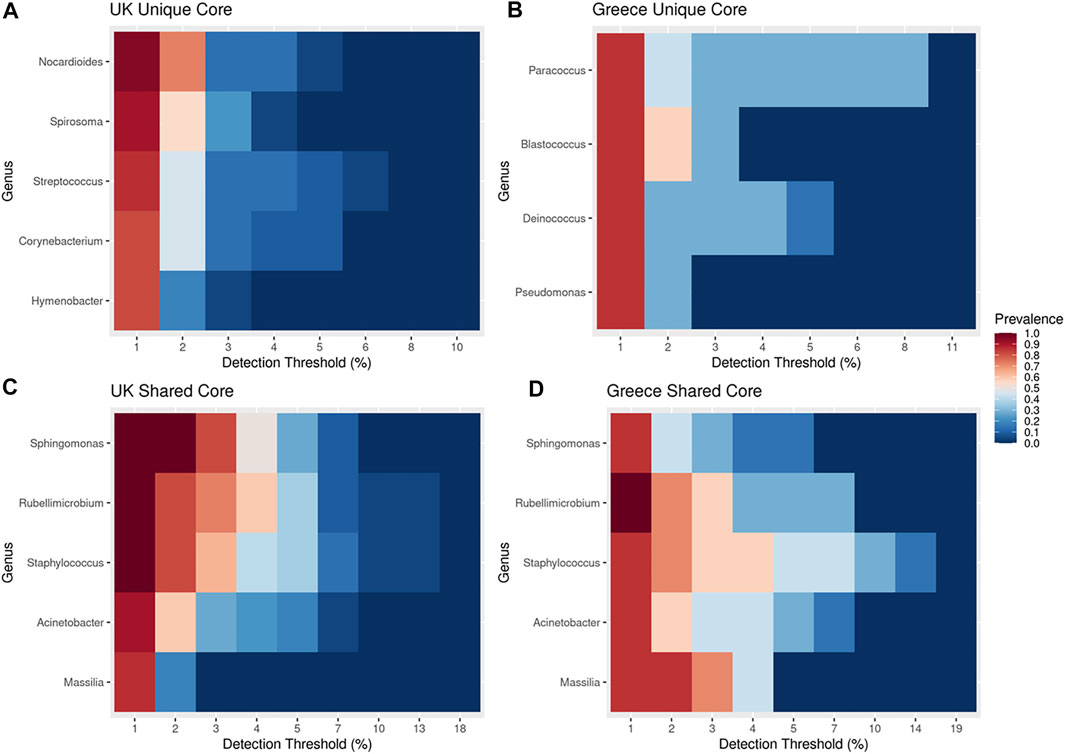
FIGURE 3. A core microbiome analysis covering unique and shared taxa for each location based upon a robust 75% prevalence threshold with at least 1% detection threshold. (A) UK unique core microbiome (B) Greece unique core microbiome, (C) UK shared core microbiome, (D) Greece shared core microbiome.
The shared core microbiome (i.e. observed throughout all of the indoor dust samples) included Massalia, Acinetobacter, Staphylococcus, Rubellimicrobium, and Sphingomonas (Figures 3C,D). Interestingly, two of these genera (Sphingomonas and Massilia) also contributed to the observed significant variation based upon geospatial location using FDR-adjusted Kruskal Wallis rank sum testing (Figure 1; pSphingomonas = 0.0312 and pMassilia = 0.0198). This indicates that while both are core genera found in house dust, Sphingomonas have a higher contribution to percentage taxonomic abundance in UK dust samples, conversely to Massilia which has a higher percentage abundance in samples from Greece (Figure 2). Sphingomonas (Family Spirosomaceae) has been found in both aqueous and terrestrial habitats, including plant root systems, clinical specimens, and other common contexts. While Massilia, a member the family Oxalobacteraceae, is typically found in a diverse range of environmental habitats and is commonly reported as an air, water, soil, and plant-associated bacterium (Chaudhary and Kim, 2017; Frediansyah et al., 2020).
The other identified members of this shared core microbiome have been frequently reported in non-residential indoor microbiomes. Acinetobacter are a strictly aerobic genera of microbes, known to reside in a wide variety of environments including soil and wastewater, cause contamination of food products, and are a known commensal bacterium residing on the skin and mucosal membranes. Previously considered saprophytic with little clinical significance, but with the emergence of antimicrobials in both agricultural and clinical settings, Acinetobacter spp. have become of increasing concern due extreme drug resistance, thereby impeding therapeutic treatments (Doughari et al., 2011). Importantly, the bacteria can also colonise the skin and respiratory tract without causing an infection, with subsequent infections occurring if the hosts primary line of defence is compromised. Staphylcoccus is a genus of Gram-positive bacteria in the family Staphylococcaceae from the order Bacillales. There are currently at least 40 species within the genus. Many are unable to cause disease, but one of the most well described species is Staphylococcus aureus, a commensal of the human microbiota that is commonly labelled as an opportunistic pathogen capable of causing a range of skin and respiratory infections. It is estimated that between 20–30% of the human population are carriers of S. aureus (Tong et al., 2015). Rubellimicrobium appears to be an under described bacterium commonly isolated from environments including soil and air (Jiang et al., 2019).
Microbes assigned as “unique core” taxa from Greece include Paracoccus, Blastococcus, Deinococcus and Pseudomonas (Figure 3B); Paracoccus, Blastococcus and Deinococcus were identified to be statistically significant contributors to the observed ordination diversity (Figure 1; FDR-adjusted Kruskal Wallis: pBlastococcus = 0.013, pDeinococcus = 0.017, pParacoccus = 0.031), indicating their contribution to the diversity. Significantly, all three taxa are associated with arid landscapes, such as occur in the Mediterranean climate of Greece, in contrast to the Oceanic/Maritime conditions of the UK (Baker et al., 1998; Lasek et al., 2018; Makarova et al., 2001; Urzì et al., 2001; Zhu et al., 2013). The UK unique core taxa (Figure 3A) included Nocardiodes, Spirosoma, Streptococcus, Corynebacterium and Hymenobacter, of which Nocardiodes and Spirosoma were identified to be statistically significant contributors to the observed ordination diversity (Figure 1; FDR-adjusted Kruskal Wallis: pSpirosoma = 0.002 pNocardioides = 0.013), again indicating their contribution to the diversity. This unique UK core consists of human commensals and environmental bacteria typically associated with soils (Yoon and Park, 2006; Jeon et al., 2013; Kim et al., 2016; Leal et al., 2016). The low prevalence of the UK unique core microbes in the samples from Greece and the low prevalence of the Greece unique core microbes in the samples from the UK further demonstrates the difference (Supplementary Figure S4). Exploration into the microbial diversity identified that all samples with sufficient read depth showed excellent richness and diversity, with a few notable exceptions of taxa dominating particular samples, including an undefined Rhizobiaceae (49.96% in sample DSGR144; 41.74% in sample DSGR146) as well as Prevotellacae (29.46% in sample DSUK013).
Study Limitations
Whilst the study is limited by its geographical extent (only two bioclimatic regions), and the number of participating households (twenty-eight), insights gained from this feasibility study are informing our scaling-up activities as a much larger study is required to determine the contribution of building type, occupancy level, building material, etc. to the overall indoor microbiome. Although we made efforts to use clear, unambiguous language to support the sampling and questionnaire survey, missing data on participant vacuum sampling date and variations in interpretation of some of the survey questions required a re-design of selected questions and procedures. For example, our attempt to capture both the “age” of the vacuum sample (i.e. time period of vacuuming represented by the sample) and the primary home construction material was inconsistently interpreted/reported (e.g. some householders reported multiple home fabric, e.g. UK166 and 167, Supplementary Table S1). Such challenges are a well-recognised aspect of citizen science, but the opportunities afforded by such collaborations are increasingly being realised and acknowledged (Phillips et al., 2019).
Conclusion
Our study showed that citizen collected samples from regular household vacuum cleaners can provide a suitable medium for investigation of the microbial communities present in residential homes. Diverse household samples, regardless of bioclimatic region, building type or occupancy, were shown to share a common core microbiome consisting of Sphingomonas, Rubellimicrobium, Staphylococcus, Acinetobacter and Massalia. Indeed, despite clear differences being reported in the human microbiome (which contributes directly to the house dust samples), a core “home microbiome” could still be detected.
Several of the bacterial genera identified as common in the home microbiome are environmentally ubiquitous (e.g., Acinetobacter, Staphylococcus, Corynebacterium) and whilst pathogenicity is species/strain-specific, and also dependent on the immune-status of the host, some like Acinetobacter spp. are increasingly of interest due to extreme drug resistance (Doughari et al., 2011). Occurrence as part of the core home microbiome is of relevance and highlights key genera for targeted further work to establish species-level identification.
Whilst several of the prominent taxa identified in the microbial communities of our household dusts were dominated by both human and non-human commensal bacteria, we also identified the presence of a region-specific core microbiome. This geospatial dependency may reflect differences in our indoor bacterial communities due to prevailing bioclimatic or country-specific factors (e.g. Paracoccus, Blastococcus, Deinococcus and Pseudomonas in the Greek samples, and Nocardiodes, Spirosoma, Streptococcus, Corynebacterium and Hymenobacter in the UK unique core). We now need to scale up this study to robustly test these hypotheses. To further understand the differences between homes, future studies should also consider including analysis of the microbiomes of the occupants (both human and animal), in parallel with key characteristics of the local environment such as the composition of the local soils, given they are key sources of input to house dust.
Data Availability Statement
The sequencing data that support the findings of this study are openly available (as raw fastq files: Target_gene 16S and Target_subfragment V4) from the European Nucleotide Archive via the study accession PRJEB46920 (individual sample accession numbers ERX6130460 to ERX6130493). URL: https://www.ebi.ac.uk/ena/browser/view/PRJEB46920.
Ethics
This study was part of the Home Biome Project, ethics approval number: 19135, Department of Geography and Environmental Science Ethics Committee, Northumbria University, UK.
Author Contributions
JE, DP, AH, AN, JW, PR, SP-V, LB, AA, and PM conceptualised the Home Biome project, input into the experimental design, and input to the manuscript. JT initiated the analyses, designed and performed the experiments and prepared the initial draft manuscript. MC developed the bioinformatic and statistical analysis pipeline, MB advised on bioinformatics analysis. ANe managed the in-house sequencing. AA provided the Greek samples. KJ was involved in experimental design and literature review. All authors contributed to and approved the final manuscript.
Funding
JE acknowledges funding from the Natural Environment Research Council (Research Grant NE/T004401/1). MB and MC acknowledge research funding from Research England’s Expanding Excellence in England (E3) Fund as part of the Hub for Biotechnology in the Built Environment. KJ acknowledges funding for her PhD research programme from Northumbria University. Open access publication fees were provide by Northumbria University.
Conflict of Interest
The authors declare that the research was conducted in the absence of any commercial or financial relationships that could be construed as a potential conflict of interest.
Publisher’s Note
All claims expressed in this article are solely those of the authors and do not necessarily represent those of their affiliated organizations, or those of the publisher, the editors and the reviewers. Any product that may be evaluated in this article, or claim that may be made by its manufacturer, is not guaranteed or endorsed by the publisher.
Supplementary Material
The Supplementary Material for this article can be found online at: https://www.frontiersin.org/articles/10.3389/fenvs.2021.754657/full#supplementary-material
References
Amend, A. S., Seifert, K. A., Samson, R., and Bruns, T. D. (2010). Indoor Fungal Composition Is Geographically Patterned and More Diverse in Temperate Zones Than in the Tropics. Proc. Natl. Acad. Sci. 107, 13748–13753. doi:10.1073/pnas.1000454107
Andrews, S. (2010). FastQC: a Quality Control Tool for High Throughput Sequence Data0. Available at http://www.bioinformatics.babraham.ac.uk/projects/fastqc2010.
Asteinberger9 (2020). asteinberger9/seq_scripts v1.1 (v1.1). Zenodo. doi:10.5281/zenodo.4270481 Available at: asteinberger9/seq_scripts v1.x.
B B Map (2020). B B Map Short Read Aligner, and Other Bioinformatic Tools. Available from: sourceforge.net/projects/bbmap/.
Baker, S. C., Ferguson, S. J., Ludwig, B., Page, M. D., Richter, O.-M. H., and van Spanning, R. J. M. (1998). Molecular Genetics of the Genus Paracoccus : Metabolically Versatile Bacteria with Bioenergetic Flexibility. Microbiol. Mol. Biol. Rev. 62 (4), 1046–1078. doi:10.1128/mmbr.62.4.1046-1078.1998
Barberán, A., Dunn, R. R., Reich, B. J., Pacifici, K., Laber, E. B., Menninger, H. L., et al. (2015). The Ecology of Microscopic Life in Household Dust. Proc. R. Soc. B. 282, 20151139. doi:10.1098/rspb.2015.1139
Barnes, C., Portnoy, J. M., Ciaccio, C. E., and Pacheco, F. (2013). A Comparison of Subject Room Dust with home Vacuum Dust for Evaluation of Dust-Borne Aeroallergens. Ann. Allergy Asthma Immunol. 110 (5), 375–379. doi:10.1016/j.anai.2013.02.010
Birzele, L. T., Depner, M., Ege, M. J., Engel, M., Kublik, S., Bernau, C., et al. (2016). Environmental and Mucosal Microbiota and Their Role in Childhood Asthma. Allergy 72 (1), 109–119. doi:10.1111/all.13002
Blanchard, P., Babichuk, N., and Sarkar, A. (2020). Evaluating the Use of Synchrotron X-ray Spectroscopy in Investigating Brominated Flame Retardants in Indoor Dust. Environ. Sci. Pollut. Res. 27 (33), 42168–42174. doi:10.1007/s11356-020-10623-4
Cameron, E. S., Schmidt, P. J., Tremblay, B. J., Emelko, M. B., and Müller, K. M. (2020). To Rarefy or Not to Rarefy: Enhancing Microbial Community Analysis through Next-Generation Sequencing. bioRxiv. doi:10.1101/2020.09.09.290049
Chaudhary, D. K., and Kim, J. (2017). Massilia Agri Sp. nov., Isolated from Reclaimed Grassland Soil. Int. J. Syst. Evol. Microbiol. 67 (8), 2696–2703. doi:10.1099/ijsem.0.002002
Colt, J. S., Gunier, R. B., Metayer, C., Nishioka, M. G., Bell, E. M., Reynolds, P., et al. (2008). Household Vacuum Cleaners vs. The High-Volume Surface Sampler for Collection of Carpet Dust Samples in Epidemiologic Studies of Children. Environ. Health 7, 6. doi:10.1186/1476-069X-7-6
Dannemiller, K. C., Mendell, M. J., Macher, J. M., Kumagai, K., Bradman, A., Holland, N., et al. (2014). Next-generation DNA Sequencing Reveals that Low Fungal Diversity in House Dust Is Associated with Childhood Asthma Development. Indoor Air 24 (3), 236–247. doi:10.1111/ina.12072
Ding, L.-J., Zhou, X.-Y., and Zhu, Y.-G. (2020). Microbiome and Antibiotic Resistome in Household Dust from Beijing, China. Environ. Int. 139, 105702. doi:10.1016/j.envint.2020.105702
Doughari, H. J., Ndakidemi, P. A., Human, I. S., and Benade, S. (2011). The Ecology, Biology and Pathogenesis of Acinetobacter spp.: an Overview. Microb. Environ. 26 (2), 101–112. doi:10.1264/jsme2.me10179
Duggan, M. J., Inskip, M. J., Rundle, S. A., and Moorcroft, J. S. (1986). Lead in Playground Dust and on the Hands of Schoolchildren. Sci. Total Environ. 44 (1), 65–79. doi:10.1016/0048-9697(85)90051-8
Ege, M. J., Mayer, M., Normand, A. C., Genuneit, J., Cookson, W. O., Braun-Fahrländer, C., et al. (2011). Exposure to Environmental Microorganisms and Childhood Asthma. N. Engl. J. Med. 364 (8), 701–709. doi:10.1056/NEJMoa1007302
Fahimipour, A. K., Ben Mamaar, S., McFarland, A. G., Blaustein, R. A., Chen, J., Glawe, A. J., et al. (2018). Antimicrobial Chemicals Associate with Microbial Function and Antibiotic Resistance Indoors. mSystems 3 (6), e00200–18. doi:10.1128/mSystems.00200-18
Frediansyah, A., Straetener, J., Brötz-Oesterhelt, H., and Gross, H. (2020). Massiliamide, a Cyclic Tetrapeptide with Potent Tyrosinase Inhibitory Properties from the Gram-Negative Bacterium Massilia Albidiflava DSM 17472T. J. Antibiot. 74 (4), 269–272. doi:10.1038/s41429-020-00394-y
Gilbert, J. A., and Stephens, B. (2018). Microbiology of the Built Environment. Nat. Rev. Microbiol. 16 (11), 661–670. doi:10.1038/s41579-018-0065-5
Hanson, B., Zhou, Y., Bautista, E. J., Urch, B., Speck, M., Silverman, F., et al. (2016). Characterization of the Bacterial and Fungal Microbiome in Indoor Dust and Outdoor Air Samples: a Pilot Study. Environ. Sci. Process. Impacts 18 (6), 713–724. doi:10.1039/c5em00639b
Haysom, I. W., and Sharp, K. (2003). The Survival and Recovery of Bacteria in Vacuum Cleaner Dust. J. R. Soc. Promot. Health 123 (1), 39–45. doi:10.1177/146642400312300114
Jeon, Y.-S., Chun, J., and Kim, B.-S. (2013). Identification of Household Bacterial Community and Analysis of Species Shared with Human Microbiome. Curr. Microbiol. 67 (5), 557–563. doi:10.1007/s00284-013-0401-y
Jiang, L.-Q., Zhang, K., Li, G.-D., Wang, X.-Y., Shi, S.-B., Li, Q.-Y., et al. (2019). Rubellimicrobium Rubrum Sp. nov., a Novel Bright Reddish Bacterium Isolated from a Lichen Sample. Antonie van Leeuwenhoek 112 (12), 1739–1745. doi:10.1007/s10482-019-01304-5
Juel Holst, G., Pørneki, A., Lindgreen, J., Thuesen, B., Bønløkke, J., Hyvärinen, A., et al. (2020). Household Dampness and Microbial Exposure Related to Allergy and Respiratory Health in Danish Adults. Eur. Clin. Respir. J. 7 (1), 1706235. doi:10.1080/20018525.2019.1706235
Kassambara, A. (2020). Ggpubr: 'ggplot2' Based Publication Ready Plots. R package version 0.4.0. Available from: https://CRAN.R-project.org/package=ggpubr.
Kembel, S. W., Meadow, J. F., O’Connor, T. K., Mhuireach, G., Northcutt, D., Kline, J., et al. (2014). Architectural Design Drives the Biogeography of Indoor Bacterial Communities. PLOS ONE 9 (1), e87093. doi:10.1371/journal.pone.0087093
Kim, S.-J., Ahn, J.-H., Weon, H.-Y., Hong, S.-B., Seok, S.-J., Kim, J.-S., et al. (2016). Spirosoma Aerophilum Sp. nov., Isolated from an Air Sample. Int. J. Syst. Evol. Microbiol. 66 (6), 2342–2346. doi:10.1099/ijsem.0.001037
Lahti, L., and Shetty, S. (2017). Tools for Microbiome Analysis in R. Available from: http://microbiome.github.com/microbiome.
Lasek, R., Szuplewska, M., Mitura, M., Decewicz, P., Chmielowska, C., Pawłot, A., et al. (2018). Genome Structure of the Opportunistic Pathogen Paracoccus Yeei (Alphaproteobacteria) and Identification of Putative Virulence Factors. Front. Microbiol. 9, 2553. doi:10.3389/fmicb.2018.02553
Lax, S., Smith, D. P., Hampton-Marcell, J., Owens, S. M., Handley, K. M., Scott, N. M., et al. (2014). Longitudinal Analysis of Microbial Interaction between Humans and the Indoor Environment. Science 345 (6200), 1048–1052. doi:10.1126/science.1254529
Leal, S. M., Jones, M., and Gilligan, P. H. (2016). Clinical Significance of Commensal Gram-Positive Rods Routinely Isolated from Patient Samples. J. Clin. Microbiol. 54 (12), 2928–2936. doi:10.1128/jcm.01393-16
Lee, M. K., Carnes, M. U., Butz, N., Azcarate-Peril, M. A., Richards, M., Umbach, D. M., et al. (2018). Exposures Related to House Dust Microbiota in a U.S. Farming Population. Environ. Health Perspect. 126 (6), 067001. doi:10.1289/ehp3145
Leung, M. H. Y., and Lee, P. K. H. (2016). The Roles of the Outdoors and Occupants in Contributing to a Potential Pan-Microbiome of the Built Environment: a Review. Microbiome 4 (1), 21. doi:10.1186/s40168-016-0165-2
Loo, E. X. L., Chew, L. J. M., Zulkifli, A. B., Ta, L. D. H., Kuo, I.-C., Goh, A., et al. (2018). Comparison of Microbiota and Allergen Profile in House Dust from Homes of Allergic and Non-allergic Subjects- Results from the GUSTO Study. World Allergy Organ. J. 11, 37. doi:10.1186/s40413-018-0212-5
Lu, J., Breitwieser, F. P., Thielen, P., and Salzberg, S. L. (2017). Bracken: Estimating Species Abundance in Metagenomics Data. Peer J. Comput. Sci. 3, e104. doi:10.7717/peerj-cs.104
Makarova, K. S., Aravind, L., Wolf, Y. I., Tatusov, R. L., Minton, K. W., Koonin, E. V., et al. (2001). Genome of the Extremely Radiation-Resistant Bacterium Deinococcus Radiodurans Viewed from the Perspective of Comparative Genomics. Microbiol. Mol. Biol. Rev. 65 (1), 44–79. doi:10.1128/mmbr.65.1.44-79.2001
McDonald, D., Clemente, J. C., Kuczynski, J., Rideout, J. R., Stombaugh, J., Wendel, D., et al. (2012). The Biological Observation Matrix (BIOM) Format or: How I Learned to Stop Worrying and Love the Ome-Ome. GigaSci 1 (1), 7. doi:10.1186/2047-217X-1-7
McMurdie, P. J., and Holmes, S. (2013). Phyloseq: an R Package for Reproducible Interactive Analysis and Graphics of Microbiome Census Data. PLoS One 8 (4), e61217. doi:10.1371/journal.pone.0061217
Meadow, J. F., Altrichter, A. E., Kembel, S. W., Kline, J., Mhuireach, G., Moriyama, M., et al. (2014). Indoor Airborne Bacterial Communities Are Influenced by Ventilation, Occupancy, and Outdoor Air Source. Indoor Air 24, 41–48. doi:10.1111/ina.12047
Morawska, L., and Salthammer, T. (2003). Indoor Environment: Airborne Particles and Settled Dust. Weinheim: Wiley-VCH, 467. ISBN: 978-3-527-30525-4.
Nygaard, A. B., and Charnock, C. (2018). Longitudinal Development of the Dust Microbiome in a Newly Opened Norwegian Kindergarten. Microbiome 6 (1), 159. doi:10.1186/s40168-018-0553-x
Oksanen, J., Blanchet, F. G., Friendly, M., Kindt, R., Legendre, P., McGlinn, D., et al. (2020). Vegan Community Ecology Package Version 2. Available at: https://cran.r-project.org, https://github.com/vegandevs/vegan (Accessed November 5–7)
Phillips, T. B., Ballard, H. L., Lewenstein, B. V., and Bonney, R. (2019). Engagement in Science through Citizen Science: Moving beyond Data Collection. Sci. Ed. 103, 665–690. doi:10.1002/sce.21501
Pruesse, E., Quast, C., Knittel, K., Fuchs, B. M., Ludwig, W., Peplies, J., et al. (2007). SILVA: a Comprehensive Online Resource for Quality Checked and Aligned Ribosomal RNA Sequence Data Compatible with ARB. Nucleic Acids Res. 35 (21), 7188–7196. doi:10.1093/nar/gkm864
Public Health England (2020). COVID-19: Cleaning in Non-healthcare Settings outside the home. Available from: https://www.gov.uk/government/publications/covid-19-decontamination-in-non-healthcare-settings/covid-19-decontamination-in-non-healthcare-settings.
R Development Core Team (2020). R: A Language and Environment for Statistical Computing. Vienna, Austria: R Foundation for Statistical Computing.
Rasmussen, P. E., Levesque, C., Chénier, M., Gardner, H. D., Jones-Otazo, H., and Petrovic, S. (2013). Canadian House Dust Study: Population-Based Concentrations, Loads and Loading Rates of Arsenic, Cadmium, Chromium, Copper, Nickel, lead, and Zinc inside Urban Homes. Sci. Total Environ. 443, 520–529. doi:10.1016/j.scitotenv.2012.11.003
Rodrigues, R. R., Rodgers, N. C., Wu, X., and Williams, M. A. (2018). COREMIC: a Web-Tool to Search for a Niche Associated Core Microbiome. PeerJ 6, e4395. doi:10.7717/peerj.4395
Round, J. L., Lee, S. M., Li, J., Tran, G., Jabri, B., Chatila, T. A., et al. (2011). The Toll-like Receptor 2 Pathway Establishes Colonization by a Commensal of the Human Microbiota. Science 332, 974–977. doi:10.1126/science.1206095
Salthammer, T. (2020). Emerging Indoor Pollutants. Int. J. Hyg. Environ. Health 224, 113423. doi:10.1016/j.ijheh.2019.113423
Shan, Y., Wu, W., Fan, W., Haahtela, T., and Zhang, G. (2019). House Dust Microbiome and Human Health Risks. Int. Microbiol. 22 (3), 297–304. doi:10.1007/s10123-019-00057-5
Slowikowski, K. (2020). Ggrepel: Automatically Position Non-overlapping Text Labels with 'ggplot2'. R package version 0.8.2. Available from: https://CRAN.R-project.org/package=ggrepel.
Täubel, M., Rintala, H., Pitkäranta, M., Paulin, L., Laitinen, S., Pekkanen, J., et al. (2009). The Occupant as a Source of House Dust Bacteria. J. Allergy Clin. Immunol. 124 (4), 834–840. doi:10.1016/j.jaci.2009.07.045
Tong, S. Y. C., Davis, J. S., Eichenberger, E., Holland, T. L., and Fowler, V. G. (2015). Staphylococcus aureus Infections: Epidemiology, Pathophysiology, Clinical Manifestations, and Management. Clin. Microbiol. Rev. 28 (3), 603–661. doi:10.1128/cmr.00134-14
Urzì, C., Brusetti, L., Salamone, P., Sorlini, C., Stackebrandt, E., and Daffonchio, D. (2001). Biodiversity of Geodermatophilaceae Isolated from Altered Stones and Monuments in the Mediterranean basin. Environ. Microbiol. 3 (7), 471–479. doi:10.1046/j.1462-2920.2001.00217.x
Wickham, H. (2009). ggplot2: Elegant Graphics for Data Analysis. New York: Springer. ISBN 978-0-387-98141-3.
Wilke, C. (2020). Cowplot: Streamlined Plot Theme and Plot Annotations for 'ggplot2. R package version 1.1.0. Available from: https://CRAN.R-project.org/package=cowplot.
Wood, D. E., Lu, J., and Langmead, B. (2019). Improved Metagenomic Analysis with Kraken 2. Genome Biol. 20 (1), 257. doi:10.1186/s13059-019-1891-0
Yoon, J-H., and Park, Y-H. (2006). “The Genus Nocardioides. P. 1099-113,” in Volume 3: The Prokaryotes: Archaea Bacteria: Firmicutes, Actinomycetes. Editors M. Dworkin, S. Falkow, E. Rosenberg, K-H. Schleifer, and E. Stackebrandt (New York, NYNew York: Springer). ISBN 978-0-387-30743-5.
Keywords: vacuum dust, bacterial assemblage, citizen science, 16S, microbiome
Citation: Thompson JR, Argyraki A, Bashton M, Bramwell L, Crown M, Hursthouse AS, Jabeen K, Marinho Reis P, Namdeo A, Nelson A, Pearce DA, Potgieter-Vermaak S, Rasmussen PE, Wragg J and Entwistle JA (2021) Bacterial Diversity in House Dust: Characterization of a Core Indoor Microbiome. Front. Environ. Sci. 9:754657. doi: 10.3389/fenvs.2021.754657
Received: 06 August 2021; Accepted: 26 October 2021;
Published: 23 November 2021.
Edited by:
Erick Bandala, Desert Research Institute (DRI), United StatesReviewed by:
Jose Luis Sanchez-Salas, University of the Americas Puebla, MexicoAngela R. Lemons, National Institute for Occupational Safety and Health (NIOSH), United States
Copyright © 2021 Thompson, Argyraki, Bashton, Bramwell, Crown, Hursthouse, Jabeen, Marinho Reis, Namdeo, Nelson, Pearce, Potgieter-Vermaak, Rasmussen, Wragg and Entwistle. This is an open-access article distributed under the terms of the Creative Commons Attribution License (CC BY). The use, distribution or reproduction in other forums is permitted, provided the original author(s) and the copyright owner(s) are credited and that the original publication in this journal is cited, in accordance with accepted academic practice. No use, distribution or reproduction is permitted which does not comply with these terms.
*Correspondence: Jane A. Entwistle, jane.entwistle@northumbria.ac.uk
†ORCID: Ariadne Argyraki, orcid.org/0000-0002-0015-2456; Matthew Bashton, orcid.org/0000-0002-6847-1525; Lindsay Bramwell, orcid.org/0000-0003-1795-8736; Matthew Crown, orcid.org/0000-0002-5091-6282; Andrew S. Hursthouse, orcid.org/0000-0003-3690-2957; Khadija Jabeen, orcid.org/0000-0003-3105-1444; Paula Marinho, orcid.org/0000-0003-0922-829; Anil Namdeo, orcid.org/0000-0002-0982-9590; Andrew Nelson, orcid.org/0000-0001-6665-4929; David A. Pearce, orcid.org/0000-0001-5292-4596; Sanja Potgieter-Vermaak, orcid.org/0000-0002-1994-7750; Pat E. Rasmussen, orcid.org/0000-0001-6748-4255; Joanna Wragg, orcid.org/0000-0003-0011-641X; Jane A. Entwistle, orcid.org/0000-0002-4375-4502