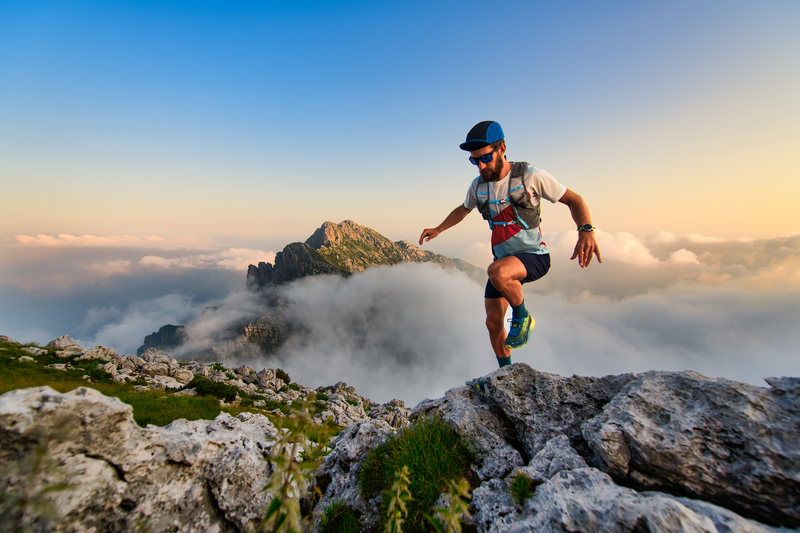
94% of researchers rate our articles as excellent or good
Learn more about the work of our research integrity team to safeguard the quality of each article we publish.
Find out more
ORIGINAL RESEARCH article
Front. Environ. Sci. , 20 January 2022
Sec. Environmental Economics and Management
Volume 9 - 2021 | https://doi.org/10.3389/fenvs.2021.745498
This article is part of the Research Topic Health in the Green Economy View all 5 articles
Seeking innovative development has become a necessity for global cities because of the accelerating consumption of natural resources and the increasing need for urgent control of environmental pollution. However, few studies have reported on the effect of innovative development on the green economic growth of resource-based cities. Therefore, this study aimed to construct the SBM model of unexpected output to measure the efficiency of green economic growth using data of 108 resource-based cities in China from 2004 to 2018, and to analyze the impact of innovative development and resource endowment on green economic growth using the systematic Generalized Method of Moments (GMM) model. The following results were obtained: first, the green economic growth efficiency of resource-based cities exhibited a general trend of fluctuation and rise, but the efficiency of different resource-based cities was different in time and space. Second, although innovative development promoted the green economic growth of resource-based cities, the impact of different resource-based cities was heterogeneous. Innovative development promoted the green economic growth of resource-based cities in eastern China, comprehensive cities, metal cities, growth cities, and renewable cities. However, it had an inhibitory effect on the green economic growth of northeast China, west China, and oil–gas and nonmetal resource–based cities, but no significant effect on the green economic growth of other resource-based cities. Third, the nonlinear effect of resource endowment on the green economic growth of resource-based cities was not significant, but resource endowment had a “curse” effect on green economic growth. Meanwhile, heterogeneity analysis showed two effects of resource endowment on the green economic growth in different resource-based cities: curse and blessing. Fourth, the impact of innovative development on the green economic growth of resource-based cities was moderated by resource endowment. The impact of innovative development on green economic growth decreased with the increase in resource endowment. Therefore, resource-based cities should not only constantly increase innovation and improve resource utilization efficiency but also actively promote coordination and cooperation of regional resource-based industries so as to achieve green and sustainable development.
Resource-based cities refer to cities that take mining and processing of mineral resources, forests, and other natural resources as the leading industries (Zhang et al., 2014; Yu et al., 2019). Among the 293 prefecture-level cities in China, 126 are resource-based cities, accounting for 43% of the total1. However, they rank among the top in China in terms of natural resources such as coal, oil, iron ore, and rare Earth. They are essential energy resource supplies and reserve bases in China and have contributed significantly to the economic development of China. However, as the exploitation of natural resources continues to run dry, some resource-based cities have entered the mature stage and the decline stage. According to the National Sustainable Development Plan for Resource-based Cities (2013–2020), the resource-based cities in the mature stage and the decline stage account for about 80% of the 262 resource-based cities in China. Some resource-based cities are even “empty cities” and “ghost cities,” and some cities are called “cities that cannot be seen on satellite.” Industrial transformation and environmental pollution seriously restrict the sustainable development of resource-based cities (Hou et al., 2019; Meng et al., 2021).
Compared with the non-resource-based cities, resource-based cities face problems such as ecological environment destruction caused by natural exploitation and industrial structure transformation required for sustainable economic development, especially the low efficiency and regional imbalance in the transformation development. The transformation and development of resource-based cities are more urgent (Chen et al., 2018; Tan et al., 2020). In recent years, as countries worldwide have gradually confirmed a timetable for carbon neutrality, environmental pollution control, and resource and energy utilization have become important development issues for all countries. Green transformation has become an inevitable choice for the sustainable development of resource-based cities to cope with environmental pollution and resource-based industry transformation (Guo et al., 2018). Green economic growth refers to promoting economic growth while nature provides resources and environmental services for human development (OECD, 2009). This means that green economic growth is an environmentally friendly “low-carbon economy” and “circular economy” that achieves sustainable economic growth through ecological protection, environmental governance, and improvement of the utilization efficiency of natural resources (Janicke, 2012). Innovation and technological progress are the main ways to improve the efficiency of economic growth (He and Wang, 2015). The strength of innovative development has become an important factor affecting regional technological progress and also an effective means to balance economic growth and environmental protection (Demirel and Kesidou, 2011). In the context of global innovation driven, the research on how natural resources regulate the impact of innovative development on green economic growth and the heterogeneity of the impact of innovative development on green economic growth of different types of resource-based cities will further expand the research on the innovative development of green transformation of resource-based cities. It will also have important policy reference value for the green transformation of resource-based cities in China and even developing countries.
The marginal contribution of this study is as follows: First, unlike previous studies that simply analyzed the relationship between technological innovation and green efficiency or explored the resource endowment and green economic growth (Liu and Dong, 2021; Zhao et al., 2021), this study has brought innovative development, resource endowment, and green economic growth into a unified framework; analyzed the impact of innovative development and resource endowment on the green economic growth of resource-based cities; and analyzed the moderating effect of resource endowment on the impact of innovative development on green economic growth. Further, it highlighted the role of innovative development in the green economic transformation of resource-based cities. Second, the impact of innovative development on the green economic growth of resource-based cities has been comprehensively analyzed in the heterogeneity test under the following three heterogeneity criteria: different life cycles, different economic regions, and different resource attributes. This approach helped avoid the limitations of previous analyses and researches on a single resource-based city or city type or simple economic region (Huang et al., 2018; Liu et al., 2018; Xie et al., 2020), enriching the heterogeneity of resource-based cities. Third, this study did not use scientific research investment, technology patent, and foreign investment to measure innovative development indicators unlike previous studies so as to avoid the limitation that a single innovative development index cannot fully reflect the real situation of urban innovative development (Xie et al., 2020; Liu and Dong, 2021). In this study, multidimensional indicators were evaluated based on the big data to represent the innovative development of cities, which could more accurately describe the real level of the innovative development of resource-based cities, effectively alleviate the selective bias of the model, and expand relevant studies on the definition and measurement of innovative development indicators.
This study has been arranged as follows. The second section presents a literature review. The third section discusses the theory and hypotheses. The fourth section introduces the model and data of this study. The fifth section analyzes the empirical results. The sixth section presents the conclusion and policy recommendations.
Some studies turned “high-energy consumption, high-pollution, and high-emission” industries in economic development into “brown economy” before the emergence of the concept of the green economy (UNEP, 2011). In the process of the rapid growth of “brown economy,” natural resources continued to be consumed; sulfur dioxide, carbon dioxide, industrial sewage, and other harmful substances continued to be discharged; and ecological damage and environmental pollution became increasingly serious. Therefore, countries around the world urgently needed to change this mode of economic development. The green economy was first proposed in the article “Blueprint for a Green Economy,” published by Pearce in 1989. He emphasized that the development of human society and economy should not be separated from the ecological environment. Pursuing economic growth while ignoring the ecological environment leads to resource depletion, making economic activities unsustainable (Pearce et al., 2013). The traditional economic growth theory only focuses on the growth of gross domestic product (GDP) but ignores the environmental cost. The United Nations Statistical Office (1993) first proposed green GDP, in which the input to the ecological environment generated by the use of natural resources was deducted from the economic aggregate. Since the United Nations issued the “Green Initiative” in 2008, the green economy began to spread widely worldwide. The OECD (2011) defined green economic growth as ensuring that natural resources and ecological environment continue to provide resources and services for human activities while promoting economic growth. The Association of Academies of Sciences in Asia (2011) conducted investigations and studies on pollution control, ecological restoration, and poverty control in Asian countries and concluded that green transformation was conducive to the sustainable development of Asian countries. Khan and Ulucak (2020) believed that the development of environmental technology was conducive to realizing green economic growth and sustainable development through data analysis of Brazil, Russia, India, China, and South African countries. Some scholars also studied green economic growth in China and believed that green economic growth has become a new growth mode to achieve sustainable development irrespective of the national or provincial level (Guo et al., 2018; Cheng et al., 2020). Compared with economic growth, green economic growth protects the environment, prevents further deterioration of the environment, and promotes the effective use of resources. On the other hand, green economic growth allows economic growth (Fernandes et al., 2021). This study argued that the connotation of green economic growth was to achieve a goal of higher economic growth at the cost of minimum ecology and environment, that is, to improve green economic growth as much as possible (Meng and Zhang, 2020). The measurement of green economic growth mainly included a single factor index and total factor index, but scholars often adopted a multi-index method because the total factor method included not only capital investment and human capital but also energy consumption and environmental pollution index (Li and Hu, 2012; Feng et al., 2018). In terms of the total factor index method, scholars mostly used the ultra-efficient SBM model with unexpected production constructed by Tone (2001) to overcome the limitations of the CCR and BBC models. Oh (2010) extended the Malmquist-Luenberger index to the Global Malmquist-Luenberger (GML) index in this technique, overcoming the deviation of the traditional ML index when formulating policies. Therefore, scholars mostly used the directional distance function method of unintended output to construct the GML index to express green economic growth (Li and Xu, 2018; Sun H. et al., 2019).
As for the research on the relationship between resource endowment and green economic growth, scholars generally believed that with the exploitation of natural resources, the effect of resource endowment on economic growth gradually changed from “resource Gospel” to “resource curse,” that is, resource-rich countries fell into low or negative economic growth (Auty and Warhurst, 1993; Hodler, 2006), and natural resources affected the regional economic growth by negatively influencing talent flow, Dutch disease, government policies, and foreign trade (Stijns, 2005; Takatsuka et al., 2015; Poncian, 2019). The resource curse research has expanded to economic growth efficiency with the deepening of research. Factors of production in resource-based countries or regions mainly flow into the resource sector, which not only destroys the local ecological environment but also drags down the regional total factor productivity (Li and Xu. 2018; Zidouemba and Elitcha, 2018). Cheng et al. (2020) studied the relationship between resource endowment and green economic growth in 30 provinces in China and found that the more abundant natural resources had a significant inhibitory effect on green economic growth. Zhang and Song (2020) found an “inverted U-shaped” relationship between resource endowment and the green transformation of the manufacturing industry. Weber (2014) found that the development of the natural gas industry in the central and southern United States actually promoted local economic growth.
Since Schumpeter (1912) put forward the concept of innovation for the first time, the research on innovative development has been constantly enriched. It includes innovation in the economic and social fields as well as in the cultural, scientific, and technological fields. Scholars tend to summarize the connotation of innovation as knowledge innovation, technological innovation, and institutional innovation. Endogenous growth theory involves the endogenous mechanism of technological progress promoting economic growth by endogenesis (Furman et al., 2002; Makkonen and Inkinen, 2013). Scholars often use patent data or regional research and development (R&D) data to study the impact of innovative development and technological progress in economic growth efficiency. Some studies found that invention patents, R&D, and product innovation have a significant positive impact on the green economy (Zhao et al., 2019; Sun et al., 2020). However, some scholars have different views on the relationship between technological innovation and green economic growth. For example, Tang and Fu (2014) used foreign direct investment (FDI) to measure technology introduction and found that technology introduction may inhibit the efficiency of economic growth. Zhao et al. (2019) believed that knowledge innovation had no significant impact on green development. Thus, it was seen that scholars still have some uncertainties regarding the relationship between innovation and green economic growth (Zhu and Li, 2019).
The exploitation of natural resources will aggravate the environmental problems in resource areas. Most of the initial industries developed in resource-based areas are initial mining industries, which cause great harm to the natural ecology. With the acceleration of industrial transformation, resource-based primary industries in resource-based areas will be upgraded to resource-based product processing and manufacturing industries, which are mostly oil and coal processing industries, and metal and non-metallic mineral products processing industries. Due to the single industrial structure and resource dependence, natural resources can both promote and inhibit the green transformation of resource-based regions. On the one hand, natural resources guarantee the sustainable economic growth of the region, especially under the effect of green policies, resource-based industries are forced to carry out green transformation and upgrading (Kesidou and Demirel, 2012; Chakraborty and Chatterjee, 2017). On the other hand, the development of resource-based industries produces problems such as rent-seeking and corruption; “crowding-out” innovative development, technological change, and human capital; aggravating the burden of ecological environment and environmental problems; and thus inhibiting the green transformation of resource-based cities (He et al., 2017; Han et al., 2020). The richer the natural resources, the lower the green total factor productivity (Cheng et al., 2020). However, some scholars have questioned the nonlinear relationship between resource endowment and a green transition, that is, natural resources do not systematically increase the “curse” and “blessing” of green development when the “crowding-out” and “forcing-out” effects of natural resources are small (Weber, 2014). At the same time, the problem of unbalanced development of global natural resources already exists in China. Resource endowment has different effects on green economic growth due to differences in resource endowment degree, regional economic development level, and resource scarcity (Dwumfour and NTOW-Gyamfi, 2018; Feng et al., 2019; Mao et al., 2019; Cheng et al., 2020). Figure 1 reflects the impact of resource endowment on green economic growth of resource-based cities. To this end, we obtained the following hypotheses:
Hypothesis 1. Resource endowment may have a nonlinear effect on green economic growth.
Hypothesis 2. Resource endowment has a “curse” effect on green growth.
Hypothesis 3..The effects of resource endowment on the green economic growth of different types of resource-based cities are heterogeneous.Endogenous economic growth theory holds that technological progress is the decisive factor to ensure sustained economic growth, and innovative development has become the key driving force for global sustainable development. Liu and Dong (2021) verified that technological innovation promoted the economic efficiency of Chinese cities. Zhang and Cui (2020) constructed innovation indicators in technological R&D and business transformation, and found that regional innovation improved the efficiency of green economic growth in China. Technological progress is the internal driving force for sustainable industrial development. Green technological innovation is conducive to enterprises’ treatment of environmental pollution and reduction of exhaust gas, wastewater, and smoke dust emissions (Peng, 2020; Feng et al., 2021). Optimizing the structure of green organizations and improving the transmission of green knowledge are conducive to improving the technology efficiency of enterprises, thus enhancing the sustainable development ability of enterprises (Matsuhashi and Takase, 2015; Mishra, 2017). Resource-based industries promote technological innovation, reduce the loss of natural resources, improve the efficiency of resource utilization, and then reduce environmental costs, so as to promote green economic growth. Meanwhile, the higher the urban governance capacity and institutional quality, the stronger the positive effect of innovative development on green economic growth (Antonioli and Mazzanti, 2017).The study of natural resources on technological innovation can be traced back to the “resource curse” (Xie et al., 2021). The richer the natural resources, the greater the crowding effect of resource-based industries on non-resource-based industries. Resource-dependent cities depend on the mining and processing of natural resources for their leading industries; the richer the resources, the more priority the local government gives to the development of the industries so as to obtain greater economic growth. However, the level of resource utilization and the limitation of resources hinder the initiative of resource-based enterprises in updating technology and inhibit the innovative development of manufacturing industry (Chen and Kung, 2016; Igna and Venturini, 2019). This causes the deterioration of the urban ecological environment and environmental pollution, increasing the environmental management costs of the government, thus limiting the green transformation (Sun H. et al., 2019; Sun and Wang, 2021). Resource-based cities can be divided into multiple groups according to different standards, and heterogeneous factors may cause deviations in the effect of innovative development on green economic growth (Wang and Chen, 2020). Cities are located in different economic regions, resulting in different geographical space positions, environmental policies, industrial policies, and economic development levels. Economically developed regions are more able to attract excellent talents and green industries due to their superior institutional and geographical advantages, thus promoting the growth of an urban green economy. Lax environmental regulations are more likely to attract polluters from rich regions to poor ones (Antweiler et al., 2001; Candau and Dienesch, 2017), but strict environmental regulations improve local environmental technology and standards, improve local environmental quality, and form a “pollution halo.” Strict or lax environmental regulations become a decision-making basis for foreign investment (Wang and Liu, 2019). Empirical research proves that eastern China attracts more foreign investments than central and western China because the institutional environment and innovation environment are better in eastern China than in central and western China (Yuan et al., 2021). Resource-based cities rely heavily on resource-based industries with their endowment characteristics, while resource-based industries are mostly low-technology-intensive industries. Cycle and resource attributes determine the uniqueness of their green economic transformation, leading to different technological biases (Acemoglu et al., 2012; Baskaran and Mehta, 2016; Altuntas et al., 2018). Therefore, these different heterogeneous factors may cause deviations in the impact of innovative development on green economic growth. Figure 2 reflects the impact of innovative development on green economic growth of resource-based cities. We have the following hypotheses:
Hypothesis 4. Innovative development promotes green economic growth in resource-based cities.
Hypothesis 5. The impact of innovative development on the green development of different types of resource-based cities is heterogeneous.
Hypothesis 6. The impact of innovative development on green economic growth is moderated by resource endowment. Innovative development has less impact on the green economic growth of resource-based cities with the increase in resource exploitation.
This study aimed to explore the impact of innovative development and resource endowment on the green economic growth of resource-based cities in China. The system GMM (Blundell and Bond, 1998) estimation method was adopted to set the model to avoid endogenous problems:
Meanwhile, to test the nonlinear relationship between resource endowment and the green economic growth of resource-based cities of China, the quadratic term of resource endowment was introduced based on Model 1:
Considering that innovative development and resource endowment do not have a single impact on the green economic growth, and the impact of innovative development on the green economic growth may be moderated by resource endowment, the interaction term Ri*Re of innovative development and resource endowment was introduced based on Models 1 and 2, and the model was set as follows:
In Models 1, 2, 3, and 4,
Green economic growth. According to the research of Oh (2010), this study constructed the GML index based on the distance function in the SBM direction to represent green economic growth. This method overcame the limitation of the CCR and BBC models, and the problem that environment and resource factors are ignored when measuring efficiency. Each resource-based city in the prefecture-level administrative region was regarded as a decision-making unit (DMU). Assuming that each DMU uses m kinds of inputs, it was counted as
Then, the super SBM model was as follows:
output and unexpected output, respectively; and
Innovative development. Patent data is the main indicator for scholars to reflect their innovative development ability. Compared with R&D, the number of invention patents can better reflect regional substantive innovation (Tong et al., 2014), but the number of patents cannot fully reflect regional innovation activities (Wang et al., 2020). Therefore, as described in Mao and Lu (2020), this study adopted the innovative undertaking development level index to depict the innovative development, using the big data analysis method, based on the microscopic enterprise data of mainland China, covering elements and multidimensional indexes, such as the subject and technology output, which can more fully reflect the innovative development of the urban development level of China. Meanwhile, the number of patents granted for invention (PAT) was used for robustness analysis to verify the rationality of the model.
Resource endowment. Due to the lack of data, this paper uses Xu (2006) to reflect the resource endowment of resource-based cities by the ratio of mining industry employees to total employment. This measurement method could better reflect the degree of resource dependence of a regional economy (Brunnschweiler and Bulte, 2008).
Industrial structure. The industry of resource-based cities is mainly the secondary industry. The main goal of industrial structure transformation is to reduce the proportion of resource-based industry in industry, promote the leading industry, and constantly evolve to the tertiary industry (Kuznets, 1957). Therefore, this study used the ratio of the tertiary industry to the secondary industry to represent the IS of resource-based cities.
Marketization level. The marketization level reflects the openness of a region, and scholars generally use the marketization index (Fan et al., 2011) or FDI proportion (James and Aadland, 2011) of regional economic openness. However, the marketization index does not have city-level data, and FDI data in some cities are seriously missing, so the data quality is not enough to explain the marketization level of cities. Therefore, the index commonly used by scholars to measure the institutional quality and market level at the city level, namely the proportion of urban private and self-employed people in the total employed population, is chosen to reflect the marketization level of the city (Ma and Tao, 2018; Song and Wu, 2018). The larger this index, the more active the private economy of the city, that is, the higher the degree of economic openness and the higher the marketization level of the city.
Government intervention. Government is an important participant in economic activities, and fiscal expenditure is one of the main means for the government to regulate industries. This study used the proportion of government fiscal expenditure in GDP for reference to the existing research to indicate the degree of GI (Lu and Chen, 2004).
Human capital. Human capital can effectively promote economic growth and innovative development. The definition of human capital is usually expressed in terms of the proportion of the educated population in the total labor population in each industry (Ciccone and Papioannou, 2009). Due to the lack of data on the number of population with educational background distribution in prefecture-level cities in China, this paper refers to the measurement method of human capital proposed by Barro and Lee (2013) and sets the schooling years of primary school, middle school, high school, and university as 6, 9, 12, and 16 years, respectively, in combination with China’s national conditions and data availability. Then
A total of 126 prefecture-level resource-based cities are present in China. This study eliminated the cities with serious data shortage due to the limitation of data updating speed and quality, and finally obtained the data of 108 resource-based cities from 2004 to 2018. The innovative development data were from the large enterprise data center of Peking University; invention patent authorization data were from the Chinese Research Data Services platform; and other data were derived from the China City Statistical Yearbook, China Regional Statistical Yearbook, Statistical Yearbook of provinces and autonomous regions of China (comprising only prefecture-level resource-based cities in the provinces and cities; does not contain other Chinese provinces and cities and regions) from 2000 to 2019, and the administrative statistical bulletin for national economic and social development. Table 1 is the statistical description of the sample variables in this study.
We used MATLAB to calculate the GML index of distance function in the SMB direction and evaluate the development status of green economic growth, green technological progress, and green technological efficiency in resource-based cities in China from time and space dimensions. Figure 3 shows the green economic growth index (GML), green technological progress index (Gtech), and green technological efficiency index (Geffch) of resource-based cities in China from 2004 to 2018. From 2004 to 2018, the green economic growth index showed a trend of fluctuation and rise. The green economic growth index mainly depended on the improvement of the green technological progress index. After 2017, the green technological efficiency index kept the same direction as the green economic growth index and began to play a positive role. The green economy growth index declined to the lowest point in 2017, but reached the highest point in 2018. This trend is consistent with the curve of green technology progress, and it keeps rising overall, indicating that the green economy of resource-based cities is developing toward higher quality. Meanwhile, the fluctuations of green economic growth index, green technological progress index, and green technological efficiency index all occurred from 2008 to 2011. The green technology progress index dropped to the lowest point in 2009 because the financial crisis had an impact on enterprises that rely on capital and increased the cost of technological innovation of enterprises (Giebel and Kraft, 2019), thus limiting the progress of urban green technology, but at the same time, the efficiency of green technology reached the highest point. This may be because most of China’s resource-based industries are owned by the state, which can quickly absorb and accept the technology and management of enterprises in developed countries affected by the financial crisis, to improve the efficiency of green technology. As the Chinese government participated in the “market rescue” and issued a four trillion yuan stimulus plan, energy-intensive industries developed rapidly, but extensive development caused environmental pollution and increased carbon emissions (Feng et al., 2021), which offset the promotion effect of this plan and brought down the green economic growth level of resource-based cities. In recent years, the Chinese government has adopted the new development philosophy of “innovative, coordinated, green, open, and shared development” and established a development path that “lucid waters and lush mountains are invaluable assets”. Since 2015, it has gradually revised and adopted the Air Pollution Prevention and Control Law, Water Pollution Prevention and Control Law, and environmental protection tax, thus reducing emissions by enterprises. In particular, emissions from polluting industries (He et al., 2020).
Different regions differ greatly in terms of resource endowment, innovation level, and economic development level due to the vast territory and complex geographical characteristics of China, resulting in the uneven development of green economy in different regions (Xiao et al., 2017; Wang and Yi, 2021). According to the “National Sustainable Development Plan for Resource-Based Cities (2013–2020)” released by the Chinese government, more than 70% of the 126 resource-based cities are in the central and western regions, which are often ecologically fragile, environmentally polluted, and economically backward (Li Y. et al., 2020). We divided resource-based cities according to the division of economic regions in the China Statistical Yearbook, namely, eastern region, northeast region, central region, and western region, to better analyze the differences in the green economic growth of resource-based cities. Figure 4 reflects the temporal variation trend and spatial difference of green economic growth efficiency, green technological progress index, and green technology efficiency index of resource-based cities in the four economic regions of China from 2004 to 2018. Overall, the green economic growth index improved by the green technological progress index. However, resource-based cities in the four major economic regions were affected by the financial crisis, and green economic growth efficiency declined in 2009. Moreover, the east, central, and west all saw big shifts in 2017. Meanwhile, the growth efficiency of the green economy changed in the same direction with the progress of green technology. In contrast, it changed in the opposite direction with the efficiency of green technology. By sector, the green economic growth efficiency of resource-based cities reached the highest point in 2008, 2011, 2010, and 2017 and the lowest point in 2011, 2012, 2017, and 2018 in eastern China, northeast China, central China, and western China, respectively. The reasons for the inconsistent time series changes of the three indexes in the four economic regions may lie in the large span of the economic regions where resource-based cities are located, different resource endowment conditions, and different marketization degrees of infrastructure, resulting in insufficient kinetic energy of technological innovation and industrial transformation (Shi et al., 2017; Qiang and Jian, 2020).
FIGURE 4. The Gml, Gtech, and Geffch indexes of resource-based cities in four economic regions in China from 2004 to 2018.
Based on the definition of main resources of different resource-based cities by Zhang et al. (2014), we divided the cities with different resource attributes into comprehensive cities, coal cities, oil and gas cities, metal cities, and nonmetal cities. Comprehensive cities referred to resource-based cities with two or more kinds of main resources. For example, the main natural resources of Baotou included coal and metal mineral deposits. Coal, oil and gas, and metal cities referred to the natural resources of the city dominated by coal, oil, and metal. Meanwhile, metallic cities referred to cities whose natural resources were dominated by ferrous metal and nonferrous metal resources. Nonmetallic cities referred to cities whose natural resources were dominated by magnesite or phosphate or potassium salt or wood. Figure 5 reflects the trend changes of green economic growth efficiency, green technology efficiency, and green technology progress index of five resource-based cities with different resource attributes from 2004 to 2018. Overall, the green economic growth index of these five cities was caused by the green technology progress index. However, after 2017, the green technology efficiency of comprehensive and metal cities began to play a positive effect. The changes in the three indexes of these five resource-based cities basically followed the general trend of resource-based cities in China, that is, “rising first, then falling, and then fluctuating and rising.” The green economic growth efficiency and green technology progress index of mixed cities reached the highest point in 2008, while the green technology efficiency declined to the lowest point in 2008 and reached the highest point in 2009, and the green economic growth efficiency reached the lowest point in 2017. The green economic growth efficiency of coal-based cities reached the highest point in 2011 and fell to the lowest point in 2017. The green technology progress index declined to the lowest point in 2009 and reached the highest point in 2011. The green technology efficiency index reached the highest point in 2009 and fell to the lowest point in 2011. The three indexes of oil and gas cities fluctuated greatly. The green economic growth efficiency fell to the lowest point in 2005 and reached the highest point in 2010; green technology progress index fell to the lowest point in 2009 and reached the highest point in 2018; and green technology efficiency fell to the lowest point in 2011 and reached the highest point in 2016. The fluctuation of the three indexes of metal cities was also obvious. The growth efficiency of the green economy reached the highest point in 2016, and then fell to the lowest point in 2017. The green technology progress also fell to its lowest point in 2017, after peaking in 2008. The green technology efficiency bottomed out in 2005 and peaked in 2009. The green economic growth efficiency and green technology progress index of nonmetallic cities fell to the lowest point in 2017, but quickly reached the maximum value in 2018, and the green technology efficiency reached the maximum value in 2014 and fell to the minimum value in 2017. The change in green economic growth efficiency of cities with different resource attributes was not consistent, which may be due to the different sensitivity of resource-based industries to environmental policies and green transformation, and the development of non-resource-based industries was squeezed out by resource-based industries (Li W. et al., 2020; Xing et al., 2021).
FIGURE 5. The GML, Gtech, and Geffch indexes for five resource-based cities with different resource properties from 2004 to 2018.
As described by Yu et al. (2019), we divided resource-based cities into mature cities, declining cities, growing cities, and regenerative cities according to their developmental stages. This classification conformed to the criteria of the Chinese government for the developmental stages of resource-based cities. Among them, mature cities referred to cities in the stable stage of resource development, declining cities referred to cities in the state of resource depletion, growth cities referred to cities in the rising stage of resource development, and regenerative cities referred to cities that basically got rid of resource dependence. Figure 6 reflects the temporal variation trends of green economy growth index, green economy technology efficiency, and green technology progress index of four resource-based cities with different life cycles from 2004 to 2018. Overall, the change trend of the green economic growth index of resource-based cities in different life cycles was basically the same as that of all resource-based cities. The green economic growth index of resource-based cities with different life cycles was all caused by the green technology progress index, but the green technology efficiency of mature cities began to play a positive effect after 2017. The green economic growth index of mature cities showed a trend of fluctuating rise from 2004 to 2010, but began to fluctuate and decline after 2010, and fell to the lowest point in 2017, and began to rise fiercely in 2018. The green economic growth of recessionary cities was generally in the shape of “M,” falling to the lowest point in 2009, reaching the highest point in 2011, and then beginning to decline after forming the highest point in 2015. The green economic growth index of growing cities presented an “M–W shape,” with peaks in 2007, 2010, and 2015 and bottoms in 2009, 2013, and 2017. The green economic growth index of recessionary cities peaked in 2008 and then declined rapidly due to the impact of the financial crisis. As the economy of China entered a new normal, the green economic growth index began to rise slowly, but fell to a minimum point in 2017 and recovered to the level of 2015 in 2018. For resource-based cities green growth has a heterogeneity index in different life cycles, the reason may be that the resources industry in different life cycles determines the direction of industry transformation of resources city is not the same (James, 2015), the growth of the city resources industry gradually expand the scale, regeneration of cities has gradually come on the reliance of the resources industry. Therefore, it has been the direction of green transformation of resource-based cities to develop continuation and non-resource industries and promote industrial diversification (Larsen, 2006; Hou et al., 2019; Liu B. et al., 2020).
FIGURE 6. The GML, Gtech, and Geffch indexes of four resource-based cities with different life cycles from 2004 to 2018.
Using the “12th 5-year” and “much starker choices- and graver consequences-in” program of the Chinese Central Government, we calculated the two stages of green growth, green technology, and green technology efficiency index of average to compare the “national resource city sustainable development planning (2013–2020)” before and after the implementation effect. The results are shown in Table 2. Overall, the green economic growth index, green technological efficiency index, and green technological progress index of all resource-based cities have been improved after implementing the plan, indicating that national planning contributed to the green economic growth of resource-based cities. However, differences existed among different types of resource-based cities. The implementation of state-level planning not only improved the level of green economic growth index of comprehensive cities, metal cities, and mature cities, but also reduced the level of green economic growth. Meanwhile, the green economic growth index of these cities and resource-based cities in the west was lower than the average level of all resource-based cities after implementing the national plan, reflecting the imbalance of green economic growth level of different types of resource-based cities. The reasons were as follows: due to the differences in economic regions, the ecological environment in western China was more fragile, the level of social and economic development was more backward than that in other regions, and the kinetic energy of western cities to improve the level of green economic growth was relatively limited (Hou et al., 2021). Resource attribute determined the development scale of resource-based industries in resource-based cities. The production of the metal industry is often accompanied by serious environmental pollution. However, resource-based industries in mature cities are more comprehensive in development and less susceptible to the influence of national planning (Meng et al., 2021).
TABLE 2. Comparison of green economic growth efficiency of resource-based cities after the implementation of national planning policies.
Table 3 reflects the full-sample regression results of Models 1, 2, and 3. From the point of estimated parameters, the AR (1) p values were less than 0.05 and the AR (2) p values were greater than 0.05, which showed that the disturbance of difference presented first-order autocorrelation, but no second-order autocorrelation. Meanwhile, the p values of Sargan statistics and Hansen statistics were both greater than 0.05, indicating that the model setting and estimation were reasonable.
From the perspective of explanatory variables, the first column presents the estimated results of model 1. The coefficient of innovative development was 0.0683, which passed the significance level test of 10%, indicating that innovative development improved the green economic growth of resource-based cities. The reason may be innovative development improved the level of technological innovation and provided the impetus for green economic growth, which proved Hypothesis 4. Muazu and Xuan (2021) also found that innovative development could reduce environmental pollution. The resource endowment coefficient was 1.2352 and passed the 1% significance level testing, showing that resource endowment hindered the green economic growth of resource-based cities. This could be because resource-based cities in the process of development of the green economic growth produced environmental pollution and were resource-dependent (Sachs and Warner, 2001), and produced the “resource curse” effect, which proved Hypothesis 2. The second column presents the estimated result of Model 2. The coefficient of RE2 was −0.2391, which did not pass the significance level test, indicating that no nonlinear “inverted U-shaped” relationship existed between resource endowment and green economic growth, proving Hypothesis 1. The reason was that the exploitation of natural resources and the development of resource-based industries in resource-based cities were often accompanied by ecological environmental damage and low efficiency of technological innovation. The resource-based cities often need to make too much investment in the ecological environment to repair the damaged ecological environment, in turn, hindering the growth of the green economy. The third column presents the estimated results of Model 3. The interaction coefficient between innovative development and resource endowment was −6.1901, which passed the significance level test of 10%. This indicated that innovative development played a smaller role in promoting the growth of the green economy with the increase in resource endowment, and the inhibitory effect of resource endowment on green economic growth was less with the improvement in innovative development level, which proved Hypothesis 6. The reason was that the high dependence on natural resources limited the level of innovative development. This also reflected that resource-based cities need to increase innovation input and promote the policy choice direction of resource-based cities to eliminate resource dependence. The fourth column introduces the interaction term between resource endowment and innovative development on the basis of Model 2. The results showed that both the interaction term and the square term failed to pass the significance level test, which verified that no nonlinear relationship existed between resource endowment and green economic growth in Model 2, which further proved Hypothesis 1. From the perspective of control variables, the coefficient symbols of IS, GI, marketization level, and HC in Models 1, 2, and 3 were the same, and the coefficient of IS was positive, indicating that the current IS of resource-based cities was conducive to the development of green economy in resource-based cities, but the IS had not passed the significance level test. The coefficient of GI was negative, indicating that the government had suppressed the growth of the regional green economy through political behavior, that is, the policies and measures of the government of resource-based cities were not conducive to the green transformation of resource-based cities, but it had not passed the significance level test. The coefficient of marketization level was positive, indicating that the higher the marketization degree, the stronger the driving effect on the green economy of resource-based cities; however, it did not pass the significance level test. The coefficient of HC was positive, indicating that the HC promoted the green economic growth of resource-based cities, and passed the significance level test in Model 3. This means that, on the one hand, strengthening talent construction is conducive to improving technological innovation level and energy and resource utilization efficiency; on the other hand, high human capital is conducive to promoting the transformation of resource-based urban economic sectors to non-resource-based industrial sectors (Kurtz and Brooks, 2011; Lee and van der Heijden, 2019).
This study carried out a robustness test of the main conclusions by replacing the explained variables and the explanatory variables to ensure the robustness of the baseline regression conclusion. It included explained variables using Getch and Geffch to replace the GML, and explanatory variables using authorized invention patents (Pat) instead of innovative development index (Id).
Table 4 reflects the replacement after explaining variable Models 1, 2, and 3 of the regression analysis results. The regression results of the model shows that the p values of AR (1), AR (2), and Sargan and Hansen statistics all indicated that the model setting was reasonable. According to Model 1, the coefficient of PAT was 0.0003, and it had passed the significance level test of 1%, indicating that technological innovation promoted the growth of the green economy. The coefficient of Re was −2.1331, which passed the significance level test of 5%, indicating that natural resources had a “curse” effect on the green economic growth of resource-based cities. The positive and negative signs of the two coefficients were the same as those of Model 1 in Table 5, which supported the robustness of the benchmark regression conclusion. According to Model 2, the coefficient of Re2 was −1.6553, which was consistent with the positive and negative signs of the coefficient of Re2 in Model 2 in Table 1, but did not pass the significance level test. This indicated that no nonlinear “inverted U-shaped” relationship existed between resource endowment and green economic growth, and the conclusion was consistent with the benchmark model. According to Model 3, the interaction term coefficient was −0.0013, which did not pass the significance level test, but was consistent with the interaction term coefficient of Model 3 in Table 1. After replacing the explanatory variable, the sign of the coefficient of the core explanatory variable was consistent with the baseline regression, which provided support for the result of the baseline regression.
Table 6 reflects the estimated results of models 1 and 3 after replacing the explained variable. From the estimated parameters of the model, the p values of AR (1) were all less than 0.05 and those of AR (2) were all greater than 0.05. Meanwhile, the p values of Sargan and Hansen statistics were all greater than 0.05, indicating that the model was set reasonably. Columns 1 and 3 are the estimated results of Model 1 after replacing variables, while columns 2 and 4 are the estimated results of Model 3 after replacing variables. The coefficients of innovative development were all positive, the coefficient of resource endowment was negative, and the coefficient of interaction term was negative, which was consistent with the estimated results of the baseline regression model. Among them, innovative development passed the significance level test in columns 3 and 4, but did not pass the significance level test in columns 1 and 2, that is, innovative development had a significant promoting effect on the change index of green technology efficiency, but its promoting effect on the green progress index was not significant. The resource endowments in columns 1, 2, and 4 all passed the significance level test, indicating that resource endowments had an obvious “curse effect” on the green technological progress index, but did not pass the significance level test in column 3. The interaction coefficients of innovative development and resource endowment were both negative, indicating that innovative development had less influence on the change index of green technology efficiency and green technology progress index with the improvement in resource endowment. The aforementioned results were consistent with the baseline regression results in Table 1, which proved that the estimation results in this study were robust.
This study aimed to analyze the impact of innovative development and resource endowment on the green economic growth of resource-based cities. Therefore, the heterogeneity test only reflected the estimated results of Model 1, mainly from the perspective of the heterogeneity of the economic geographic region that resource-based cities belonged to, the heterogeneity of resource endowment, and the heterogeneity of the life cycle of urban development.
Table 7 reflects the heterogeneity analysis results of different regions of resource-based cities. According to the p values of the estimated parameters of the model, AR (1), AR (2), and Sargan and Hansen statistics, the setting of the model was reasonable.
From the perspective of explanatory variables, regional heterogeneity was observed between innovative development and green economic growth of resource-based cities; however, no regional heterogeneity existed between resource-based green economic growth and resource endowment. The coefficients of innovative development in columns 1 and 3 of Table 2 are positive (1.2453 and 0.0446, respectively), indicating that innovative development could promote the green economic growth of resource-based cities in the eastern and central regions. However, only the innovative development variable in column 1 passed the significance level test. In columns 2 and 4, the coefficient of innovative development is negative (−1.3158 and −0.7025, respectively), and passed the significance level test. This indicated that innovative development had an inhibitory effect on the green economic growth of resource-based cities in northeast China and west China. This finding was consistent with that of Mushtaq et al. (2020), who revealed that innovation degraded the environment in the northwest region, while higher innovation improved the environmental quality in eastern and southwest regions. The reason was that the innovative development environment and development level in eastern and central China were better than those in less-developed northeast and western China, and innovative development could release the power of green economic growth more (Liu C. et al., 2020). The coefficients of resource endowment from column 1 to column 4 are all negative, indicating that resource endowment has an inhibitory effect on green economic growth of resource-based cities. This is because resource-based cities often face problems such as ecological environment destruction and environmental pollution (Meng et al., 2021), especially the resource-based industries are mostly heavily polluting industrial enterprises, such as oil, coal, steel, and other resource-based cities.
From the perspective of control variables, the coefficients of all control variables in columns 1–4 are not the same, indicating that the influence of IS, GI, marketization level, and HC on the green economic growth of resource-based cities also had regional heterogeneity. This was consistent with some previous studies (Ren et al., 2019). The IS coefficients in columns 1, 2, and 3 are negative, indicating that the IS had an inhibitory effect on the green economic growth in the eastern, northeastern, and western regions, and all of them passed the significance level test. The coefficient of IS in column 3 is positive, indicating that the IS had a promoting effect on the growth of the green economy in the central region, but it did not pass the significance level test. This is because the resource-based cities were mostly in underdeveloped areas, and the resource-based cities in the east have taken over the industries of the surrounding big cities, causing environmental pollution. The northeast and western regions have become pollution havens, as developed cities in the east and central regions shift to industries in the northeast and west. The coefficients of GI are also different, in which columns 1–3 are positive, but only columns 1 and 2 passed the significance level test, indicating that GI promoted the green economic growth of resource-based cities in the eastern, northeastern, and central regions. The coefficient of GI in the fourth column is negative, indicating that GI inhibited the green economic growth of resource-based cities in the western region, but it did not pass the significance level test. The coefficient of marketization level in columns 1–3 is negative, indicating that the marketization level hindered green economic growth in the eastern, northeastern, and central resource-based cities, and this inhibitory effect was only significant in the eastern region. The coefficient of marketization level from column 1 to column 3 is negative, indicating that marketization level hinders the green economic growth of resource-based cities in the east, northeast, and central China, and this inhibition effect is only significant in the eastern region. The coefficient of marketization level in western China is positive, but not significant. This shows that no matter in the eastern or central regions, resource-based cities in China are generally in underdeveloped areas with low marketization level. Human capital significantly promotes the resource-based cities in eastern and central green economic growth, promoting effect is not significant, in the western areas is not significant on the inhibitory effect of northeast area, the result is in China’s regional talent distribution characteristics, namely the eastern, central is relatively abundant, the west as China’s “western development” policy continues to advance. Human capital in the west has been improved, but northeast China has faced the problem of a brain drain in recent years (Yu and Chen, 2020).
Table 8 reflects the heterogeneity test results of the benchmark regression in the samples of resource-based cities with different resource attributes. According to the estimation results of the model, the p values of AR (1) were all less than 0.05, and the p values of AR (2) and Sargan and Hansen statistics were all greater than 0.05, indicating that the estimation results of the model were reasonable. From the perspective of explanatory variables, the impact of innovative development and resource endowment on the green economic growth of different resource-based attributes was heterogeneous. The coefficient of innovative development in columns 1–5 is 0.1442, 0.0315, 0.4610, 0.9877, and 0.7142, of which columns 1 and 3, and rows 4 and 5 passed the significance level inspection, showing that innovative development of integrated urban and metal mold city green had obvious roles in promoting the economic growth; oil and gas and nonmetallic cities had an obvious inhibitory effect, but no significant inhibition of coal city was found. The coefficients of resource endowment are −1.7029, −1.1320, 0.6407, −1.7573, and 6.3583, respectively, and pass the significance level test in column 1, 2, and 3, indicating that resource endowment has a restraining effect on the green economic growth of comprehensive cities and coal cities, and has a significant promoting effect on oil and gas cities. However, resource endowment has no significant effect on inhibiting and promoting metal city and non-metal city. Qian et al. (2019) also found a resource curse effect in China’s coal-mining cities. The reason may be that the oil and gas cities were in the stage of development, and the natural resources of the nonmetal cities were less polluted, but the industries of the comprehensive, coal, and metal cities had been more mature, causing greater pressure on the ecological environment (Tan et al., 2017). From the perspective of control variables, the effects of IS, GI, and marketization level on green economic growth in cities with different resource attributes were not consistent, indicating that different natural resource endowments caused the differences in green development paths of resource-based cities. However, HC had a positive influence on the green economic growth of cities with different resource-based attributes and exhibited a significant promoting effect on the green economic growth of metal cities.
Table 9 reflects the regression results of the samples of resource-based cities with different life cycles of Model 1. Overall, the p value of AR (1) in the model in columns 1–4 is less than 0.05, and the p values of AR (2) and Sargan and Hansen statistics are all greater than 0.05, indicating that the setting and estimation of the model were reasonable. From the perspective of explanatory variables, innovative development could promote the green economic growth of resource-based cities with different life cycles and had a significant promoting effect on the growth cities and regenerative cities. This finding was consistent with that by Xie et al. (2020), who found that technological innovation could promote the industrial green efficiency of different resource-based cities. The effects of resource endowment on resource-based cities with different life cycles were different, among which, resource endowment significantly promoted the green economic growth of mature and regenerative cities but hindered the green economic growth of declining and growing cities. The reason was that the mature urban resource industry was relatively mature, and the development and utilization efficiency of resources was relatively high. Although regenerative cities had eliminated the growth path of resource dependence, their industrial development still mostly relied on mature traditional resource-based industries. Growth cities were in the stage of rapid development of resource exploitation. The exploitation of resources and the production of resource-based industries do great damage to the ecological environment. In addition, the dependence of the economy on resources reduced the level of green development. The resources of recession-type cities tended to be exhausted or have been exhausted. After experiencing the growth and maturity periods, on the one hand, ecological and environmental problems are still present in history; on the other hand, industrial simplification restricted economic development. The restoration of the ecological environment and difficulties in industrial upgrading restricted the development of the green economy. From the perspective of control variables, none of the IS variables passed the significance level test. The influence of GI on resource-based cities with different life cycles was also different. Among them, GI in growing cities had a significant promoting effect on green economic growth, while the others did not pass the significance level test. The impact of the marketization level on the green economic growth of resource-based cities in different development cycles was also different. It had a significant promoting effect on the green economic growth of mature and regenerative cities, but a significant inhibitory effect on the green economic growth of declining and growing cities. HC had a significant inhibitory effect on the green economic growth of mature cities and a significant promoting effect on the green economic growth of growing cities. However, it had no obvious effect on the green economic growth of declining and regenerating cities. This may be because the development environment of resource-based cities with different life cycles had a different attraction to human resources.
We studied the impact of innovative development and resource endowment on green economic growth in resource-based cities. The following conclusions were drawn through empirical tests. First, the green economic growth efficiency index of resource-based cities was measured based on the SMB model of unexpected output. The results showed that the green economic growth efficiency of resource-based cities in China exhibited an overall trend of rising fluctuation from 2004 to 2018, and the curve trend of green technological progress was basically the same as that of the green economic value-added index. The curve trend of green technological efficiency index, green economic growth index, and green technological progress index was opposite in 2017, which indicated that the green economic growth of resource-based cities in China mainly depended on the improvement in the green technological progress index, and accelerating technological innovation was conducive to the improvement in green economic growth (Zhai and An, 2021). Moreover, resource-based cities should not only improve the level of technological progress but also improve the level of green technology efficiency. However, the green economic growth index of different types of resource-based cities showed the difference in time and space, but the curve keeps rising and fluctuating. Second, innovative development promoted the green economic growth of resource-based cities, and innovation capacity was a key factor for the sustainable development of resource-based cities (Zhang and Cui, 2020). By adding the interaction term between innovative development and resource endowment into the benchmark model, the interaction term coefficient was found to be negative, indicating that the impact of innovative development on the green economic growth of resource-based cities was moderated by resource endowment, that is, the more abundant the natural resources, the less the impact of innovative development on green economic growth. This was because rich natural resources crowded out technological innovation and HC in non-resource-based industries (Han et al., 2020). Third, resource endowment had a restraining effect on the green economic growth of resource-based cities, that is, resource endowment had a “resource curse” effect on the green economic growth, which was consistent with the conclusion of Cheng et al. (2020). Adding the quadratic term of resource endowment to the benchmark model, the empirical results showed that the inverted U-shaped relationship between resource endowment and green economic growth was not significant. Fourth, this study also tested the heterogeneous impact of innovative development and resource endowment on green economic growth in resource-based cities with different economic regions, different resource attributes, and different life cycles. The results showed that the impact of innovative development on green economic growth was different in different economic regions and resource-based cities with different resource attributes. However, it was found that resource endowment only had different effects on green economic growth with different resource attributes and different life cycles, and a “resource curse” effect existed on the green economic growth of resource-based cities in different economic regions. Resource endowment had a significant “curse” effect on the eastern region, central region, comprehensive type, coal type, and mature resource-based cities, and a significant “blessing” effect on mature resource-based cities, while other types of resource-based cities had no significant effect.
The empirical conclusion provided reliable support for dealing with the related problems of green economic growth in resource-based cities. To this end, we proposed the following policy recommendations. First, the conclusion of the GML index based on the SMB model of unintended output revealed that the green economic growth of resource-based cities promoted not only green technological progress but also green technological efficiency, which was because, in the past, the green technological efficiency of resource-based cities and green economic growth index changed in the opposite direction. The conclusion of the benchmark model also confirmed that innovative development significantly promoted the green economic growth of resource-based cities. Therefore, resource-based cities should strengthen technological innovation, especially the innovation level of green technology, optimize innovative talents, strengthen innovation support, improve technological progress index, and optimize green management and green organization structure to improve the efficiency of green technology (Zeraibi, et al., 2021) and promote industrial transformation. Promoting green economic growth in resource-based cities (Feng et al., 2019; Liang et al., 2021). Second, the conclusion of the benchmark model showed that resource endowment restrained the green economic growth level of resource-based cities, and resource endowment had a moderating effect on the relationship between innovative development and green economic growth. Therefore, on the one hand, resource-based cities should encourage and support innovation and new entrepreneurship, enhance the level of innovative development, and accelerate the development of new economy such as the continuation industry and non-resource-based industry, which were conducive to promoting the IS transformation of resource-based cities (Nikolaev and Krivovichev, 2019). In particular, the resource-based cities in less-developed areas in northeast China and western China, as well as coal-based, oil and gas, and nonmetallic resource-based cities should enhance the momentum of urban innovative development, change the previous resource-driven economic growth mode, and shift to the path of innovation-driven green economic growth (Hou et al., 2019). On the other hand, resource-based cities should enhance innovation support for non-resource-based industries, optimize talent policies, attract talents and technology to non-resource-based industries, and eliminate and reduce the crowding out effect of resource endowment on non-resource-based industries (Wang et al., 2019; Namazi and Mohammadi, 2018), so as to give full play to the maximum driving force of innovation and development to improve the level of green economic growth. Third, the heterogeneity test indicated that green economic growth in resource-based cities was different in time and space, which determined that the central government should change the previous regional economic policy of “efficiency first and fairness given consideration,” but should take both efficiency and equity into consideration to promote coordinated regional development (Sheng et al., 2014; Bong and Premaratne, 2018; Sun H. et al., 2019). According to the local development, the present situation of local government timely adjusted industrial policies and environmental policies, are both to promote local economic growth and pay more attention to the ecological environment. This required local governments to continuously optimize the local business environment, release the innovation vigor, and develop a new economy, to improve resource utilization efficiency, change IS, and realize green development. Fourth, the green economic development of resource-based cities in China is universal in resource-based cities. Our conclusion also provides a policy reference for resource-based cities in other developing countries to promote green economic growth, for example, for resource-based regions in Latin America and Africa, which are facing problems such as “resource curse” and “resource blessing” (Henri, 2019). Properly handling the relationship between innovative development, resource endowment, and green economic growth is conducive to the green and sustainable development of resource-based cities.
Although the results of this study were robust, the study had some limitations. First, this study was based on the SMB model that calculated the index of green economic growth, but the green measure of economic growth and a variety of forms, especially, were the expected output indicators. The expected output of this study contained only industrial wastewater, industrial sulfur dioxide emissions (powder), and industrial smoke dust emissions; other pollutant indexes could not be measured, such as soil pollution, noise pollution, and so on. In the future, the construction of a multiindex analysis framework would be helpful to measure green economic growth accurately. Second, this study was based on the innovative development indicators measured by Big Data, but it could not measure the subdimensions of innovative development, such as green organizations and green institutions. In the future, subdimensions of innovative development based on Big Data methods should be built, and the impact of each dimension of innovative development on green economic growth should be analyzed more accurately.
The raw data supporting the conclusion of this article will be made available by the authors, without undue reservation.
This manuscript was contributed by all authors. All the authors have ratified the manuscript. MZ compiled the manuscript; QR and TY collected data and information; TY reviewed the manuscript and provided sources of funding. All authors have participated in the work of this manuscript.
This work was supported by MOE (Ministry of Education in China) Project of Humanities and Social Science (17YJC790024), and National Natural Science Foundation of China (71973019).
Author QR is employed by Chongqing Ruiyun Technology Co., Ltd.
The remaining authors declare that the research was conducted in the absence of any commercial or financial relationships that could be construed as a potential conflict of interest.
All claims expressed in this article are solely those of the authors and do not necessarily represent those of their affiliated organizations, or those of the publisher, the editors, and the reviewers. Any product that may be evaluated in this article, or claim that may be made by its manufacturer, is not guaranteed or endorsed by the publisher.
1See National Sustainable Development Plan for Resource-Based Cities (2013–2020). Web: http://www.gov.cn/gongbao/content/2013/content_2547140.htm
Acemoglu, D., Aghion, P., Bursztyn, L., and Hemous, D. (2012). The Environment and Directed Technical Change. Am. Econ. Rev. 102 (1), 131–166. doi:10.1257/aer.102.1.131
Altuntas, S., Cinar, O., and Kaynak, S. (2018). Relationships Among Advanced Manufacturing Technology, Innovation, export, and Firm Performance. K 47 (9), 1836–1856. doi:10.1108/K-10-2017-0380
Antonioli, D., and Mazzanti, M. (2017). Towards a green Economy through Innovations: The Role of Trade union Involvement. Ecol. Econ. 131, 286–299. doi:10.1016/j.ecolecon.2016.09.003
Antweiler, W., Copeland, B. R., and Taylor, M. S. (2001). Is free trade good for the environment? Am. Econ. Rev. 91, 877–908. doi:10.1257/aer.91.4.877
Auty, R., and Warhurst, A. (1993). Sustainable development in mineral exporting economies. Res. Pol. 19, 14–29. doi:10.1016/0301-4207(93)90049-S
Barro, R. J., and Lee, J. W. (2013). A New Data Set of Educational Attainment in the World, 1950-2010. J. Dev. Econ. 104 (104), 184–198. doi:10.1016/j.jdeveco.2012.10.001
Baskaran, S., and Mehta, K. (2016). What Is Innovation Anyway? Youth Perspectives from Resource-Constrained Environments. Technovation 52-53, 4–17. doi:10.1016/j.technovation.2016.01.005
Bong, A., and Premaratne, G. (2018). Regional Integration and Economic Growth in Southeast Asia. Glob. Business Rev. 19 (6), 1403–1415. doi:10.1177/0972150918794568
Blundell, R., and Bond, S. (1998). Initial conditions and moment restrictions in dynamic panel data models. J. Econ. 87 (1), 115–143. doi:10.1016/S0304-4076(98)00009-8
Brunnschweiler, C. N., and Bulte, E. H. (2008). The Resource Curse Revisited and Revised: A Tale of Paradoxes and Red Herrings. J. Environ. Econ. Manag. 55 (3), 248–264. doi:10.1016/j.jeem.2007.08.004
Candau, F., and Dienesch, E. (2017). Pollution haven and corruption paradise. J. Environ. Econ. Manag. 85, 171–192. doi:10.1016/j.jeem.2017.05.005
Chakraborty, P., and Chatterjee, C. (2017). Does Environmental Regulation Indirectly Induce Upstream Innovation? New Evidence from India. Res. Pol. 46 (5), 939–955. doi:10.2139/ssrn.266413110.1016/j.respol.2017.03.004
Chen, T., and Kung, J. K.-S. (2016). Do land Revenue Windfalls Create a Political Resource Curse? Evidence from China. J. Dev. Econ. 123, 86–106. doi:10.1016/j.jdeveco.2016.08.005
Chen, W., Shen, Y., and Wang, Y. (2018). Evaluation of Economic Transformation and Upgrading of Resource-Based Cities in Shaanxi Province Based on an Improved TOPSIS Method. Sust. Cities Soc. 37, 232–240. doi:10.1016/j.scs.2017.11.019
Cheng, Z., Li, L., and Liu, J. (2020). Natural Resource Abundance, Resource Industry Dependence and Economic green Growth in China. Resour. Pol. 68, 101734. doi:10.1016/j.resourpol.2020.101734
Ciccone, A., and Papaioannou, E. (2009). Human Capital, the Structure of Production, and Growth. Rev. Econ. Stat. 91 (1), 66–82. doi:10.1162/rest.91.1.66
Demirel, P., and Kesidou, E. (2011). Stimulating Different Types of Eco-Innovation in the UK: Government Policies and Firm Motivations. Ecol. Econ. 70 (8), 1546–1557. doi:10.1016/j.ecolecon.2011.03.019
Dwumfour, R. A., and Ntow-Gyamfi, M. (2018). Natural Resources, Financial Development and Institutional Quality in Africa: Is There a Resource Curse? Resour. Pol. 59, 411–426. doi:10.1016/j.resourpol.2018.08.012
Fan, G., Wang, X. L., and Ma, G. R. (2011). ContIdbution of Marketization to China’s Economic Growth. Econ. Res. J. 46 (09), 4–16. in Chinese.
Feng, C., Huang, J.-B., and Wang, M. (2018). Analysis of green Total-Factor Productivity in China's Regional Metal Industry: A Meta-Frontier Approach. Resour. Pol. 58, 219–229. doi:10.1016/j.resourpol.2018.05.008
Feng, Y., Wang, X., and Liang, Z. (2021). How Does Environmental Information Disclosure Affect Economic Development and Haze Pollution in Chinese Cities? the Mediating Role of green Technology Innovation. Sci. Total Environ. 775, 145811. doi:10.1016/j.scitotenv.2021.145811
Feng, Y., Zhong, S., Li, Q., Zhao, X., and Dong, X. (2019). Ecological Well-Being Performance Growth in China (1994-2014): From Perspectives of Industrial Structure green Adjustment and green Total Factor Productivity. J. Clean. Prod. 236, 117556. doi:10.1016/j.jclepro.2019.07.031
Fernandes, C. I., Veiga, P. M., Ferreira, J. J. M., and Hughes, M. (2021). Green Growth versus Economic Growth: Do Sustainable Technology Transfer and Innovations lead to an Imperfect Choice? Bus Strat Env 30 (4), 2021–2037. doi:10.1002/bse.2730
Furman, J. L., Porter, M. E., and Stern, S. (2002). The Determinants of National Innovative Capacity. Res. Pol. 31 (6), 899–933. doi:10.1016/S0048-7333(01)00152-4
Giebel, M., and Kraft, K. (2019). External financing constraints and firm innovation. J. Industrial Econ. 67, 91–126. doi:10.1111/joie.12197
Guo, L.-l., Qu, Y., Wu, C.-y., and Wang, X.-l. (2018). Identifying a Pathway towards green Growth of Chinese Industrial Regions Based on a System Dynamics Approach. Resour. Conservation Recycling 128, 143–154. doi:10.1016/j.resconrec.2016.09.035
Han, D., Li, T., Feng, S., and Shi, Z. (2020). Does Renewable Energy Consumption Successfully Promote the Green Transformation of China's Industry? Energies 13 (1), 229. doi:10.3390/en13010229
He, S. Y., Lee, J., Zhou, T., and Wu, D. (2017). Shrinking Cities and Resource-Based Economy: the Economic Restructuring in China's Mining Cities. Cities 60, 75–83. doi:10.1016/j.cities.2016.07.009
He, X. G., and Wang, Z. L. (2015). Energy Biased Technology Progress and Greengrowth Transformation: An empiIdcal Analysis Based on 33 indus⁃tIdes of China. China IndustIdal Econ. 12 (2), 50–62. doi:10.19581/j.cnki.ciejournal.2015.02.006
He, Y., Wen, C., and Zheng, H. (2020). SDoes China’s Environmental Protection Tax Law Effectively Influence Firms? Evidence from Stock Markets. Emer. Markets Finance Trade, 1–12. doi:10.1080/1540496x.2020.1822810
Henri, P. A. O. (2019). Natural Resources Curse: A Reality in Africa. Resour. Pol. 63, 101406. doi:10.1016/j.resourpol.2019.101406
Hodler, R. (2006). The Curse of Natural Resources in Fractionalized Countries. Eur. Econ. Rev. 50 (6), 1367–1386. doi:10.1016/j.euroecorev.2005.05.004
Hou, C., Chen, H., and Long, R. (2021). Coupling and Coordination of China's Economy, Ecological Environment and Health from a green Production Perspective. Int. J. Environ. Sci. Technol. 2021, 1–20. doi:10.1007/s13762-021-03329-8
Hou, G., Zou, Z., Zhang, T., and Meng, Y. (2019). Analysis of the Effect of Industrial Transformation of Resource-Based Cities in Northeast China. Economies 7 (2), 40. doi:10.3390/economies7020040
Huang, Y., Fang, Y., Gu, G., and Liu, J. (2018). The Evolution and Differentiation of Economic Convergence of Resource-Based Cities in Northeast China. Chin. Geogr. Sci. 28 (3), 495–504. doi:10.1007/s11769-018-0962-6
Igna, I. A., and Venturini, F. (2019). The Impact of Educational Mismatch on Returns to R&D: Evidence from Manufacturing in OECD Countries. Econ. Innovation New Tech. 28 (5), 435–464. doi:10.1080/10438599.2018.1527548
James, A., and Aadland, D. (2011). The Curse of Natural Resources: An Empirical Investigation of U.S. Counties. Resource Energ. Econ. 33 (2), 440–453. doi:10.1016/j.reseneeco.2010.05.006
James, A. (2015). The Resource Curse: A Statistical Mirage? J. Dev. Econ. 114, 55–63. doi:10.1016/j.jdeveco.2014.10.006
Jänicke, M. (2012). "Green Growth": From a Growing Eco-Industry to Economic Sustainability. Energy Policy 48, 13–21. doi:10.1016/j.enpol.2012.04.045
Khan, D., and Ulucak, R. (2020). How do environmental technologies affect green growth? evidence from brics economies. Sci. Total Environ. 712, 136504. doi:10.1016/j.scitotenv.2020.136504
Kesidou, E., and Demirel, P. (2012). On the Drivers of Eco-Innovations: Empirical Evidence from the UK. Res. Pol. 41 (5), 862–870. doi:10.1016/j.respol.2012.01.005
Kurtz, M. J., and Brooks, S. M. (2011). Conditioning the "Resource Curse": Globalization, Human Capital, and Growth in Oil-Rich Nations. Comp. Polit. Stud. 44 (6), 747–770. doi:10.1177/0010414011401215
Kuznets, S. (1957). Quantitative aspects of the economic growth of nations: II. industrial distribution of national product and labor force. Econ. Dev. Cultural Change 5, 1–111. doi:10.1086/449740
Larsen, E. R. (2006). Escaping the Resource Curse and the Dutch Disease? Am. J. Econ. Sociol. 65 (3), 605–640. doi:10.1111/j.1536-7150.2006.00476.x
Lee, T., and van der Heijden, J. (2019). Does the Knowledge Economy advance the green Economy? an Evaluation of green Jobs in the 100 Largest Metropolitan Regions in the United States. Energ. Environ. 30 (1), 141–155. doi:10.1177/0958305X18787300
Li, J. L., and Xu, B. (2018). Curse or Blessing: How Does Natural Resource Abundance Affect Green Economic Growth in China? Econ. Res. J. 53 (9), 151–167.
Li, L.-B., and Hu, J.-L. (2012). Ecological Total-Factor Energy Efficiency of Regions in China. Energy Policy 46, 216–224. doi:10.1016/j.enpol.2012.03.053
Li, W., Sun, H., Tran, D. K., and Taghizadeh-Hesary, F. (2020). The Impact of Environmental Regulation on Technological Innovation of Resource-Based Industries. Sustainability 12 (17), 6837. doi:10.3390/su12176837
Li, Y., Zhang, Q., Wang, L., and Liang, L. (2020). Regional Environmental Efficiency in China: An Empirical Analysis Based on Entropy Weight Method and Non-parametric Models. J. Clean. Prod. 276, 124147. doi:10.1016/j.jclepro.2020.124147
Liang, G., Yu, D., and Ke, L. (2021). An Empirical Study on Dynamic Evolution of Industrial Structure and Green Economic Growth-Based on Data from China's Underdeveloped Areas. Sustainability 13 (15), 8154. doi:10.3390/su13158154
Liu, B., Wang, J., Jing, Z., and Tang, Q. (2020). Measurement of Sustainable Transformation Capability of Resource-Based Cities Based on Fuzzy Membership Function: A Case Study of Shanxi Province, China. Resour. Pol. 68, 101739. doi:10.1016/j.resourpol.2020.101739
Liu, C., Gao, X., Ma, W., and Chen, X. (2020). Research on Regional Differences and Influencing Factors of green Technology Innovation Efficiency of China's High-Tech Industry. J. Comput. Appl. Math. 369, 112597. doi:10.1016/j.cam.2019.112597
Liu, X., Pei, T., Zhou, C., Du, Y., Ma, T., Xie, C., et al. (2018). A Systems Dynamic Model of a Coal-Based City with Multiple Adaptive Scenarios: A Case Study of Ordos, China. Sci. China Earth Sci. 61 (3), 302–316. doi:10.1007/s11430-016-9077-5
Liu, Y., and Dong, F. (2021). How Technological Innovation Impacts Urban green Economy Efficiency in Emerging Economies: A Case Study of 278 Chinese Cities. Resour. Conservation Recycling 169, 105534. doi:10.1016/j.resconrec.2021.105534
Lu, M., and Chen, Z. (2004). Urbanization, Urban-Biased Economic Policies and Urban-Rural Inequality. Econ. Res. J. 39 (06), 50–58.
Ma, Z. X., and Tao, Y. T. (2018). Institutional Supply, Institutional Quality and Unbalanced Urban Development——EmpiIdcal Study Based on the Differences in the Development of 288 Cities after the Reform and Opening-up. Public Finance Res. 21 (06), 70–83. doi:10.19477/j.cnki.11-1077/f.2018.06.005
Makkonen, T., and Inkinen, T. (2013). Innovative capacity, educational attainment and economic development in the European Union: Causal relations and geographical variations. Eur. Plann. Stud. 21 (12), 1958–1976. doi:10.1080/09654313.2012.722968
Mao, W., Wang, W., and Sun, H. (2019). Driving patterns of industrial green transformation: A multiple regions case learning from China. Sci. Total Environ. 697, 134134. doi:10.1016/j.scitotenv.2019.134134
Mao, W. F., and Lu, J. (2020). How does land misallocation affect the quality of urban innovation and entrepreneurship in China? EmpiIdcal evidence from the city level of prefecture-level cities. IndustIdal Econ. Res. 11 (03), 17–29+126. doi:10.13269/j.cnki.ier.2020.03.002
Matsuhashi, R., and Takase, K. (2015). Green innovation and green growth for realizing an affluent low-carbon society. Lce 06 (04), 87–95. doi:10.4236/lce.2015.64010
Meng, W., and Zhang, Y. (2020). Natural resource endowment, path selection of technological progress, and green economic growth: An empirical research based on China's provincial panel data. Resour. Sci. 42 (12), 2314–2327. doi:10.18402/resci.2020.12.05
Meng, Y., Liu, L., Wang, J., Ran, Q., Yang, X., and Shen, J. (2021). Assessing the impact of the national sustainable development planning of resource-based cities policy on pollution emission intensity: evidence from 270 prefecture-level cities in China. Sustainability 13 (13), 7293. doi:10.3390/su13137293
Muazu, I., and Xuan, V. (2021). Exploring the relationships among innovation, financial sector development and environmental pollution in selected industrialized countries. J. Environ. Manage. 284, 112057. doi:10.1016/j.jenvman.2021.112057
Mushtaq, A., Chen, Z., Ud Din, N., Ahmad, B., and Zhang, X. (2020). Income inequality, innovation and carbon emission: perspectives on sustainable growth. Econ. Research-Ekonomska Istraživanja 33, 769–787. doi:10.1080/1331677x.2020.1734855
Namazi, M., and Mohammadi, E. (2018). Natural resource dependence and economic growth: A TOPSIS/DEA analysis of innovation efficiency. Resour. Pol. 59, 544–552. doi:10.1016/j.resourpol.2018.09.015
Nikolaev, A. I., and Krivovichev, S. V. (2019). Prospects for the Development of the Kola Chemical Technological Cluster in Transition from a Resource-Based Economy to an Innovative Economy. Theor. Found. Chem. Eng. 53 (5), 933–938. doi:10.1134/S0040579519050178
OECD (2011). Invention and Transfer of Environmental Technologies. Available at: https://www.oecd.org/env/consumptioninnovation/inventionandtransferofenvironmentaltechnologies.htm.
Oh, D.-h. (2010). A global Malmquist-Luenberger productivity index. J. Prod. Anal. 34 (3), 183–197. doi:10.1007/s11123-010-0178-y
Pearce, D., Markandya, A., and Barbier, E. (2013). Blueprint 1: For a green Economy. New York: Routledge. doi:10.4324/9781315070223
Peng, X. (2020). Strategic interaction of environmental regulation and green productivity growth in China: Green innovation or pollution refuge? Sci. Total Environ. 732, 139200. doi:10.1016/j.scitotenv.2020.139200
Poncian, J. (2019). Extractive resource ownership and the subnational resource curse: insights from tanzania. Extractive Industries Soc. 6 (2), 332–342. doi:10.1016/j.exis.2018.08.013
Qian, X., Wang, D., Wang, J., and Chen, S. (2021). Resource curse, environmental regulation and transformation of coal-mining cities in China. Resour. Pol. 74, 101447. doi:10.1016/j.resourpol.2019.101447
Qiang, Q., and Jian, C. (2020). Natural resource endowment, institutional quality and china's regional economic growth. Resour. Pol. 66, 101644. doi:10.1016/j.resourpol.2020.101644
Ren, M., Wang, X. M., Liu, Z. M., Liu, L., and Zhang, W. X. (2019). Spatial-temporal changes of regional ecological efficiency in China and their influencing factors. East China Econ. Manag. 97 (9), 71–79. doi:10.19629/j.cnki.34-1014/f.181112005
Sachs, J. D., and Warner, A. M. (2001). The curse of natural resources. Eur. Econ. Rev. 45 (4-6), 827–838. doi:10.1016/S0014-2921(01)00125-8
Sheng, Y., Shi, X., and Zhang, D. (2014). Economic growth, regional disparities and energy demand in China. Energy Policy 71, 31–39. doi:10.1016/j.enpol.2014.04.0010.1016/j.enpol.2014.04.001
Shi, Y., Guo, S., and Sun, P. (2017). The role of infrastructure in China's regional economic growth. J. Asian Econ. 49, 26–41. doi:10.1016/j.asieco.2017.02.004
Song, C. H., and Wu, F. X. (2018). The Impacts of Institutional Environment on the Magnitude of Urban Agglomeration Economies: Based on the Dual Perspectives of Citizenization and Market Transition. J. Yunnan Univ. Finance Econ. 34 (06), 3–15. doi:10.16537/j.cnki.jynufe.000318
Stijns, J.-P. C. (2005). Natural resource abundance and economic growth revisited. Resour. Pol. 30 (2), 107–130. doi:10.1016/j.resourpol.2005.05.001
Sun, H.-P., Sun, W.-F., Geng, Y., Yang, X., and Edziah, B. K. (2019). How does natural resource dependence affect public education spending? Environ. Sci. Pollut. Res. 26 (4), 3666–3674. doi:10.1007/s11356-018-3853-6
Sun, H., Edziah, B. K., Sun, C., and Kporsu, A. K. (2019). Institutional quality, green innovation and energy efficiency. Energy policy 135, 111002. doi:10.1016/j.enpol.2019.111002
Sun, Y., Ding, W., Yang, Z., Yang, G., and Du, J. (2020). Measuring China's regional inclusive green growth. Sci. Total Environ. 713 (15), 136367–1136367. doi:10.1016/j.scitotenv.2019.136367
Sun, Z., and Wang, Q. (2021). The asymmetric effect of natural resource abundance on economic growth and environmental pollution: Evidence from resource-rich economy. Resour. Pol. 72, 102085. doi:10.1016/j.resourpol.2021.102085
Takatsuka, H., Zeng, D.-Z., and Zhao, L. (2015). Resource-based cities and the dutch disease. Resource Energ. Econ. 40, 57–84. doi:10.1016/j.reseneeco.2015.01.003
Tan, J. T., Zhang, X. L., Liu, L., Zhao, H. B., and Chou, F. D. (2020). Research on the Urban Transformation Performance of China's Resource-Based Cities. Econ. Geogr. 40 (07), 57–64. doi:10.15957/j.cnki.jjdl.2020.07.007
Tan, J., Zhang, P., Lo, K., Li, J., and Liu, S. (2017). Conceptualizing and measuring economic resilience of resource-based cities: Case study of Northeast China. Chin. Geogr. Sci. 27 (3), 471–481. doi:10.1007/s11769-017-0878-6
Tang, W. B., and Fu, Y. H. (2014). Dynamic impacts of investmentin scientific and technological activities and spillover effect of for⁃eign direct investment on intensive economic growth: A varying pa⁃rameter test of the state-space model. China Soft Sci. Maga⁃zine 3 (9), 172–181.
The Association of Academies of Sciences in Asia (2011). Towards a Sustainable Asia – Green Transition and Innovation. Beijing: Science Press/Springer.
Tone, K. (2001). A slacks-based measure of efficiency in data envelopment analysis. Eur. J. Oper. Res. 130 (3), 498–509. doi:10.1016/s0377-2217(99)00407-5
Tong, T. W., He, W., He, Z.-L., and Lu, J. (2014). Patent regime shift and firm innovation: evidence from the second amendment to china's patent law. Amproc 2014 (1), 14174. doi:10.5465/ambpp.2014.1417410.5465/ambpp.2014.14174abstract
Unep, U. (2011). Towards a green Economy: Pathways to Sustainable Development and Poverty Eradication. Nairobi, Kenya: UNEP.
Wang, C. Y., Lan, Z. M., Zhang, C., and Hou, X. S. (2020). High-speed Rail Construction, Human Capital Migration and Regional Innovation. China IndustIdal Econ. 1 (12), 102–120. doi:10.19581/j.cnki.ciejournal.2020.12.005
Wang, K, Wu, M., Sun, Y., Shi, X., Sun, A., and Zhang, P. (2019). High-speed Rail Construction, Human Capital Migration and Regional Innovation. China IndustIdal Econ. 60, 203–214. doi:10.1016/j.resourpol.2019.01.001
Wang, H., and Liu, H. (2021). Foreign direct investment, environmental regulation, and environmental pollution: an empirical study based on threshold effects for different Chinese regions Environ. Sci. Pollut. Res. 26, 5394–5409. doi:10.1007/s11356-018-3969-8
Wang, Q., and Yi, H. (2021). New energy demonstration program and China's urban green economic growth: Do regional characteristics make a difference? Energy Policy 151, 112161. doi:10.1016/j.enpol.2021.112161
Wang, Y., and Chen, X. (2020). Natural resource endowment and ecological efficiency in China: Revisiting resource curse in the context of ecological efficiency. Resour. Pol. 66, 101610. doi:10.1016/j.resourpol.2020.101610
Weber, J. G. (2014). A decade of natural gas development: The makings of a resource curse? Resource Energ. Econ. 37, 168–183. doi:10.1016/j.reseneeco.2013.11.013
Xiao, Z., Du, X., and Wu, C. (2017). Regional difference and evolution and convergence of innovation capability in China: research on space and factorial levels. Sustainability 9 (9), 1644. doi:10.3390/su9091644
Xie, W., Yan, T., Xia, S., and Chen, F. (2020). Innovation or introduction? The impact of technological progress sources on industrial green transformation of resource-based cities in China. Front. Energ. Res. 8, 301. doi:10.3389/fenrg.2020.598141
Xie, X., Li, K., Liu, Z., and Ai, H. (2021). Curse or blessing: how does natural resource dependence affect city‐level economic development in China? Aust. J. Agric. Resour. Econ. 65 (2), 413–448. doi:10.1111/1467-8489.12423
Xing, M., Luo, F., and Fang, Y. (2021). Research on the sustainability promotion mechanisms of industries in China's resource-based cities -- from an ecological perspective. J. Clean. Prod. 315, 128114. doi:10.1016/j.jclepro.2021.128114
Xu, K. N. (2006). Natural Resources Abundance and Economic Growth: A Re-examination of the ‘Resource Curse’ Hypothesis. The J. World Economy 11, 38–47+96.
Yu, J., Li, J., and Zhang, W. (2019). Identification and classification of resource-based cities in china. J. Geogr. Sci. 29 (8), 1300–1314. doi:10.1007/s11442-019-1660-8
Yu, X., and Chen, S. K. (2020). Analysis on the Difference between Inter-provincial Population Mobility and Human Capital Mobility in China. Popul. J. 42 (01), 30–41. doi:10.16405/j.cnki.1004-129X.2020.01.003
Yuan, B., Li, C., Yin, H., and Zeng, M. (2021). Green innovation and China's CO2 emissions - the moderating effect of institutional quality. J. Environ. Plann. Manag. 25, 1–30. doi:10.1080/09640568.2021.1915260
Zeraibi, A., Balsalobre-Lorente, D., and Murshed, M. (2021). The influences of renewable electricity generation, technological innovation, financial development, and economic growth on ecological footprints in ASEAN-5 countries. Environ. Sci. Pollut. Res. 28, 51003–51021. doi:10.1007/s11356-021-14301-x
Zhai, X., and An, Y. (2021). The relationship between technological innovation and green transformation efficiency in China: An empirical analysis using spatial panel data. Tech. Soc. 64, 101498. doi:10.1016/j.techsoc.2020.101498
Zhang, F., and Song, X. N. (2020). Resource Endowment, Technological Progress and Greenness Transformation of ManufactuIdng Industry. Stat. Decis. 36 (13), 98–102.
Zhang, J., Wu, G., and Zhang, J. (2004). The Estimation of China’s provincial capital stock: 1952 —2000. Econ. Res. J. 10, 35–44.
Zhang, W. Z., Yu, J. H., Wang, D., and Zhan, L. (2014). Study on Sustainable Development of Resource-Based Cities in China. Beijing: Science Press.
Zhang, Y., and Cui, M. (2020). Determining the innovation efficiency of resource-based cities using a relational network dea model: Evidence from China. Extractive Industries Soc. 7 (4), 1557–1566. doi:10.1016/j.exis.2020.07.019
Zhao, K., Zhang, R., Liu, H., Wang, G., and Sun, X. (2021). Resource Endowment, Industrial Structure, and Green Development of the Yellow River Basin. Sustainability 13 (8), 4530. doi:10.3390/su13084530
Zhao, S., Jiang, Y., and Wang, S. (2019). Innovation stages, knowledge spillover, and green economy development: moderating role of absorptive capacity and environmental regulation. Environ. Sci. Pollut. Res. 26 (24), 25312–25325. doi:10.1007/s11356-019-05777-9
Zhu, J., and Li, Y. F. (20192019). Non-R&D innovation and regional productivity: Ananalysis based on spatial panel econometIdc model. J. Beijing Normal Univ. (Social Sciences) 05 (4), 147–160.
Keywords: innovative development, green economic growth, resource endowment, resource-based cities, green transformation
Citation: Zhang M, Yan T and Ren Q (2022) Does Innovative Development Drive Green Economic Growth in Resource-Based Cities? Evidence From China. Front. Environ. Sci. 9:745498. doi: 10.3389/fenvs.2021.745498
Received: 22 July 2021; Accepted: 13 December 2021;
Published: 20 January 2022.
Edited by:
Elias T. Ayuk, Independent Researcher, Accra, GhanaReviewed by:
Daniel Balsalobre-Lorente, University of Castilla-La Mancha, SpainCopyright © 2022 Zhang, Yan and Ren. This is an open-access article distributed under the terms of the Creative Commons Attribution License (CC BY). The use, distribution or reproduction in other forums is permitted, provided the original author(s) and the copyright owner(s) are credited and that the original publication in this journal is cited, in accordance with accepted academic practice. No use, distribution or reproduction is permitted which does not comply with these terms.
*Correspondence: Taihua Yan, eWFudGFpaHVhQGNxdS5lZHUuY24=
Disclaimer: All claims expressed in this article are solely those of the authors and do not necessarily represent those of their affiliated organizations, or those of the publisher, the editors and the reviewers. Any product that may be evaluated in this article or claim that may be made by its manufacturer is not guaranteed or endorsed by the publisher.
Research integrity at Frontiers
Learn more about the work of our research integrity team to safeguard the quality of each article we publish.