- 1Institute for Finance and Economics, Central University of Finance and Economics, Beijing, China
- 2Department of Economics and Finance, The Hang Seng University of Hong Kong, Hong Kong, China
Greenhouse gases, especially carbon dioxide (CO2) emissions, are viewed as one of the core causes of climate change, and it has become one of the most important environmental problems in the world. This paper attempts to investigate the relation between CO2 emissions and economic growth, industry structure, urbanization, research and development (R&D) investment, actual use of foreign capital, and growth rate of energy consumption in China between 2000 and 2018. This study is important for China as it has pledged to peak its carbon dioxide emissions (CO2) by 2030 and achieve carbon neutrality by 2060. We apply a suite of machine learning algorithms on the training set of data, 2000–2015, and predict the levels of CO2 emissions for the testing set, 2016–2018. Employing rmse for model selection, results show that the nonlinear model of k-nearest neighbors (KNN) model performs the best among linear models, nonlinear models, ensemble models, and artificial neural networks for the present dataset. Using KNN model, sensitivity analysis of CO2 emissions around its centroid position was conducted. The findings indicate that not all provinces should develop its industrialization. Some provinces should stay at relatively mild industrialization stage while selected others should develop theirs as quickly as possible. It is because CO2 emissions will eventually decrease after saturation point. In terms of urbanization, there is an optimal range for a province. At the optimal range, the CO2 emissions would be at a minimum, and it is likely a result of technological innovation in energy usage and efficiency. Moreover, China should increase its R&D investment intensity from the present level as it will decrease CO2 emissions. If R&D reinvestment is associated with actual use of foreign capital, policy makers should prioritize the use of foreign capital for R&D investment on green technology. Last, economic growth requires consuming energy. However, policy makers must refrain from consuming energy beyond a certain optimal growth rate. The above findings provide a guide to policy makers to achieve dual-carbon strategy while sustaining economic development.
Introduction
Greenhouse gases, especially carbon dioxide (CO2) emissions, are viewed as one of the core causes of climate change, and it has become one of the most important environmental problems in the world (Rehman et al. (2021a)). At the press conference on WMO State of the Climate 2019 Report, António Guterres, UN Chief, reported that 2019 was the second hottest year on record during his opening remarks. According to the World Meteorological Organization’s (WMO) flagship State of the Global Climate report, the global average temperature in 2020 was about 1.2°C above preindustrial level.
To mitigate the threat of runaway climate change, the Paris Agreement calls for limiting global warming to well below 2 and preferably to 1.5°C, compared to preindustrial levels. This requires global emissions to peak as soon as possible, with a rapid fall of 45 percent from 2010 levels by 2030, and to continue to drop off steeply to achieve net zero emissions by 2050 (Bertram et al., 2021). The world is way off track in meeting this target at the current level of nationally determined contributions. Global greenhouse gas emissions of developed countries and economies in transition have declined by 6.5 percent over the period 2000–2018. Meanwhile, the emissions of developing countries are up by 43.2 percent from 2000 to 2013. The rise is largely attributable to increased industrialization and enhanced economic output measured in terms of GDP.
Carbon dioxide emissions have been the primary source of extreme environmental pollution (Rehman et al. (2021a)). With the rapidly growing agriculture and farm mechanization, agricultural sector has become a factor in the surge in CO2 emissions and other greenhouse gases in the globe (Rehman et al. (2021b)).
Economic, social, and environmental suitability are the three core pillars of the UN’s Sustainable Development Goals (SDG) declarations (Rehman et al. (2021a)). In September 2019, Heads of State and Government gathered in the SDG Summit at the United Nations Headquarters in New York to follow up and comprehensively review progress in the implementation of the 2030 Agenda for Sustainable Development and the 17 Sustainable Development Goals (SDGs). The summit resulted in the adoption of the Political Declaration and its core message is to take action to respond to climate emergencies. Relevant research shows that if economic growth and climate and environmental sustainability are achieved at the same time, emission reduction policies need to be incorporated into the economic growth policies of various countries (Murshed et al. (2020), Li et al. (2021), Rehman et al. (2021a)).
As the world’s second largest economy, the Chinese government strives to achieve environmental sustainability through a series of policies and measures. At the General Debate of the 75th session of the United Nations General Assembly on 22nd September 2020, President Xi Jinping of China announced that China will scale up its Intended Nationally Determined Contributions by adopting more vigorous policies and measures. It also aims to have CO2 emissions peak before 2030 and achieve carbon neutrality before 2060.
Generally speaking, various economic activities will affect carbon emissions, Liu et al. (2021). They include industrial structure (Shen et al., 2021), energy consumption, trade, and urbanization (Kasman and Duman, 2015), consumption structure of fossil fuel and cleaner fuel (Murshed et al., 2020), foreign investment (Elliott and Sun, 2013), and technology advancement (Yu and Du, 2018).
Based on this background, this paper studies the relation between China’s economic growth, industrial structure, urbanization, R&D investment, foreign investment, energy consumption growth, and CO2 emissions from 2000 to 2018 and predicts it.
Choosing China as an ideal case to study driving factors on CO2 emissions is because China has accounted for the highest level of CO2 emissions across the globe in 2017 (Ma et al., 2021). President Xi Jinping addressed the General Assembly of United Nations and declared China’s national goal of turning carbon neutral by 2060. China is an important country to play a key role in achieving the 2030 Sustainable Development Agenda of the United Nations. In order to achieve the 2030 Sustainable Development Agenda of the United Nations and the Paris Agreement at the same time, China must achieve the carbon emissions peak by 2030 and the carbon neutrality by 2060 while sustaining a certain economic growth. To this end, China has formulated a “dual-carbon” strategy. Therefore, it is vital to study the drivers that influence CO2 emissions. Economic growth and CO2 emissions go hand in hand as economic activities give rise to CO2 emissions. Therefore, economic growth is the core factor affecting CO2 emissions. Industrialization and urbanization are the two main lines of China’s economic and social development that includes the CO2 emissions of the production side and the consumption side, respectively (Cao et al., 2016; Han et al., 2019). Industrialization and urbanization are compound factors affecting carbon emissions. It is because the process of industrialization and urbanization includes the factors driving CO2 emissions and limiting CO2 emissions. Industrialization has brought the change of industrial structure, and the CO2 emissions of different industries are different. On the one hand, urbanization has an impact on the CO2 emissions caused by residents’ consumption, which is quite different between urban residents and rural residents. On the other hand, urbanization is the movement of industries and population in different areas. Therefore, urbanization also reflects the different performance of carbon emissions in urban area and rural area. Technological progress, foreign investment, and energy consumption are the specific factors of CO2 emissions, technological progress reduce CO2 emissions by exploring and usage of clean energy, foreign investment reflects the pollution haven (tax environmental regulation, good market access to high-income countries, and corruption opportunities) (Candau and Dienesch, 2017), and energy consumption determines the quantity of CO2 emissions.
This paper contributes to the literature in two ways. 1) This is a comprehensive research; we try to build a framework which includes three levels of six driving factors on CO2 emissions as shown in Figure 1. The most important factors include economic growth, industrialization, urbanization, technology progress, foreign direct investment, and energy consumption. 2) Most of the existing studies are based on OLS framework to explore the relation between carbon emissions and related factors. It is difficult to avoid the omission of variables or endogeneity issues, Kasman and Duman (2015). An increasing number of recent studies (Li et al., 2021; Liu et al., 2021) have been using cross-sectionally augmented autoregressive distributed lag (CS-ARDL) approach developed by Chudik and Pesaran (2015) for short- and long-term CO2 emissions forecast. This research applies a suite of machine learning algorithms in predicting CO2 emissions using the factors discussed. Machine learning avoids omission of variables and endogeneity issues. In addition, the trends and relation between CO2 emissions and various factors are predicted.
The rest of this paper is organized as follows. Literature Review provides a literature review on CO2 emissions. Data and the Variables describes the data and variables under study. Methodology describes the machine learning algorithms deployed for predicting the level of CO2 emissions. Results compares the accuracy of predictions among various machine learning algorithms. Discussions discusses the results using the best performing model, while Conclusion and Policy Implications concludes the paper.
Literature Review
Economic scale, economic structure, and technological level are the three major factors affecting the environment (Grossman and Krueger, 1995). Economic scale is the output of the economy; more economic output means more pollution. It is because that economic growth needs more resources investment and more energy consumption. Economic structure is industry structure. The change of industry structure will reduce the pollution. With economic developing, percentage of secondary industry, especially energy-intensive industry, will reduce percentage of tertiary industry, and energy consumption will increase, so the pollution will be reducing. Technology progress will realize the usage of resource efficiency and reduce the energy consumption. So, technological level is an important factor which influences the energy intensity and pollution. Many research studies are based on these three environment factors and extend them accordingly. The research can be classified into relation between economic growth and CO2 emissions, industry structure, technology, and CO2 emissions, and urbanization and CO2 emissions. But results differ from research focus, theories, and methods. There are three parallel literatures on factors what will influence CO2 emissions.
The first group of studies has investigated the relation between CO2 emission, economic growth, and energy consumption. Environmental Kuznets Curve (EKC) is often used to discuss the relation between environmental pollution and economic growth, which is also the main method to analyze the relation between CO2 emissions and economic growth (Lin and Jiang, 2009). Grossman and Krueger (1991) found the U-shaped relation between economic growth and CO2 emissions. But the result is opposite if CO2 is used as the environmental indicator. Holtz-Eakin and Selden (1995), Sachs et al. (1999), Friedl and Getzner (2003), and Galeotti et al. (2006) found that the relation between CO2 emission and economic growth is inverted U-shape. It is opposite in the study of Shafik (1994), Martin (2008) and Murshed and Dao (2020) which find that per capita CO2 emission increased in parallel with per capita income, and there is no turning point. Moomaw and Unruh (1997), Martinez-Zarzoso and Bengochea-Morancho (2004), Friedl and Getzner (2003), and Akpan and Chuku (2011) found that the relation between CO2 emission and economic growth is N-shape. Saidi and Hammami (2015) examined the effect of energy use and the CO2 emissions on economic growth for 58 countries, and their empirical results showed that CO2 emissions negatively affected economic growth. Rahman et al. (2020), Liu et al. (2012), and Lantz and Feng (2006) found that per capita GDP has no relation with CO2 emission.
Environmental Kuznets Curve describes the economic growth in developed countries and the inverted U-shaped relation between environmental pollution, consciously or unconsciously, as for the developed countries to adjust economic structure and the energy consumption structure and achieve a faster pace of the inverted U-shaped path, the overall environmental quality as economic growth accumulation showed a trend of deterioration before improvement (Lin and Jiang, 2009). Acheampong (2018) found that energy consumption has a negative impact on economic growth in global level, economic growth has a negative impact on CO2 emission, and CO2 emission has positive impact on economic growth. In the Asia-Pacific region, economic growth does not cause CO2 emissions. But in Caribbean-Latin America, there is a feedback causality between economic growth and carbon emissions.
The second group of studies has investigated the relation between CO2 emissions, industry structure, and technology progress. Bernardini and Galli (1993) found that the decline in energy intensity shows a decline trend with the increase in income. The three reasons behind the relationship descent are the following. First of all, with the development of the economy, the final demand structure changes with changes in the stage of industrialization. In the preindustrial stage, agriculture is the leading industry in economic development, and economic growth is driven by basic needs, which can be met with low energy intensity. In the stage of industrialization, the infrastructure network needs to be built up to facilitate large-scale production and consumption. The primitive accumulation of capital stock related to industrialization can increase energy intensity, but it eventually reached the saturation point. At this time, the consumption of materials tended to replace durables rather than create durables. In the postindustrialized stage, the decline of manufacturing industry in relation between services and energy intensity in service-based economies is smaller than that in manufacturing-oriented economies. Shahbaz et al. (2018) and Khan et al. (2019) found that financial development helps control CO2 emissions in both France and China. However, Liu et al. (2021) found that with 1% financial development, CO2 emissions increased 0.17–0.52%.
Technology progress is the dominant factor of long-run economic growth with scarce resources. Technology change has a positive influence on energy efficiency and negative influence on energy intensity (Lin and Du, 2014; Sadorsky, 2013; Yu et al., 2021). Ang (2009) used the framework to combine modern growth theoretically, which can analyze the role of R&D activity and technology progress in reducing pollution. Technology progress is the result of R&D investment, which contributes to energy intensity reduction (Young, 1998). Wei et al. (2010) extended Antweiler’s model (Antweiler et al., 2001) to analyze the influence factors of CO2 emissions. The study found that GDP, industrialization, and free trade have positive influence on CO2 emissions, but independent research and development and technology import contribute to reducing CO2 emissions.
One source of technology progress is independent innovation; another source is FDI and trade. FDI and trade are latecomer advantage of countries, which develops later. Elliott and Sun (2013) found that FDI has negative influence on energy intensity. The last study (Khan et al., 2021) investigates the roles of export diversification and composite country risks in carbon emissions abatement. The researchers found that lowering country risks, undergoing renewable energy transition, and enhancing environmental-related technological innovations assist in reducing CO2 emissions in the long run.
The third group of studies has investigated the relation between CO2 emissions and urbanization. At present, there are a large number of literatures on urbanization and its impact on carbon dioxide (CO2) emissions for reference. A lot of research have directly investigated the positive impact of urbanization on carbon dioxide emissions (Behera and Dash, 2017; York et al., 2003; Zhang and Lin, 2012). Shahbaz et al. (2017) provided evidence showing that the development of urbanization leads to higher demands for food, housing, transportation, land usage, and energy consumption and causes serious environmental degradation problems. For instance, traffic congestion, waste management, and poor sanitation could cause pollution and health problems in most urban areas.
A number of studies have tested the linear impact of urbanization on global carbon dioxide emissions. You can find contributions that support it, such as those by York et al. (2003), Cole and Neumayer (2004), Liddle and Lung (2010), Wang et al. (2012), and Behera and Dash (2017), or those that refute it, such as those by Hossain (2011) and Liu and Bae (2018). Specifically, York et al. (2003) used panel data from 143 countries to record the positive impact of urbanization on CO2. Cole and Neumayer (2004) and Liddle and Lung (2010) reached similar findings using panel data and Stochastic Impacts by Regression on Population, Affluence, and Technology (STIRPAT). Wang et al. (2012) applied PLS with STIRPAT model in Beijing, China, and concluded that urbanization is the most influential factor that has adverse impact on environmental quality. Subsequently, Wang et al. (2013) found that urbanization, industrial growth, income levels, and population stimulate CO2 emissions during a provincial study. Behera and Dash (2017) used panel cointegration test to study the positive impact of urbanization on carbon emissions in South and Southeast Asian countries. Conversely, several studies proposed uncertain results and reported the negligible impact of urbanization on CO2 emissions (Hossain, 2011; Liu and Bae, 2018).
To a large extent, the level of economic development of the country may alleviate the nature of the relation between urbanization and pollution (Fan et al., 2006; Li and Lin, 2015; Poumanyvong and Kaneko, 2010). However, higher urbanization growth rates and development rates can improve the environment by promoting technological innovation in energy usage and efficiency, increasing awareness of environmental issues, and using green technologies, Bekhet and Othman (2017). Urbanization has an inverted U-shaped relation with CO2 emissions in Asia (Fan et al., 2020). However, Zhu et al. (2012) found there is limited support for inverted U-shaped relation between CO2 emissions and urbanization in 20 emerging economies. There was a long-run bidirectional positive relation between CO2 emissions, urbanization, and energy consumption in MENA countries. However, the long-run relation is based on the countries’ income and development (Al-mulalia et al., 2013). Urbanization has a positive influence on CO2 emissions; in the stage of urbanizing, it needs more energy consumption which will increase CO2 emissions (Lin and Du, 2013). CO2 emissions are higher in big cities or urban agglomeration areas, because of the high energy consumption on residential electricity consumption, residential gas consumption, residential heating consumption, and residential transportation energy consumption (Bai et al., 2019). In east and central China, the center and surroundings featured high levels (high-high cluster) of total CO2 emissions and low levels (low-low-cluster) of per-unit-GDP CO2 emission in urban agglomerations. The Yangtze-River-Delta, the Beibu-Gulf, and the Guangdong-Hong Kong-Macao UAs were more efficient at emission reduction with the cities’ rising scales, while cities of the Beijing-Tianjin-Hebei UA and the Chengdu-Chongqing UA performed less efficiently (Cui et al., 2020).
Two main methodologies are used by three groups of studies. One of the methodologies is econometrics. Econometric methods include spatial autocorrelation analysis, semiparametric fixed effect (Zhu et al., 2012), panel threshold regression (Zi et al., 2016), panel threshold regression (Du and Xia, 2018), autoregressive distributed lag model and vector-error correction model (Bekhet and Othman, 2017), two-stage least squares (2SLS) and augmented Stochastic Impacts by Regression on Population, Affluence, and Technology (STIRPAT) model (Bai et al., 2019), and autoregressive distributed lag (ARDL) (Ang, 2009). Econometric methods have been used to estimate the long-run relationship and the short-run dynamics for environmental pollution and its determinants. To address the issues of multicollinearity and overfitting, a recent study introduced the least absolute shrinkage and selection operator (LASSO) regression model which can pinpoint the most important determinants to investigate the driving factors influencing household carbon emissions (Shi et al., 2020). Another study on methods called cross-sectionally augmented autoregressive distributed lags (CS-ARDL) can account for cross-sectional dependency, slope heterogeneity, and structural break issues in the data (Li et al., 2021; Ma et al., 2021). The other methodology is calculating the quantity of CO2 emissions. Many research studies are based on Kaya identity and Logarithmic Mean Divisa Index (LMDI) (Ang and Zhang, 2000). Using these methods, researchers calculate the industrial CO2 emissions, regional CO2 emissions, and national CO2 emissions (Yang and Li, 2017). Based on LMDI, index decomposition analysis (IDA) is developed and becomes one of the most popular methods. However, IDA calculates the technology efficiency of economy system, not the efficiency of energy usage (Lin and Du, 2013). Wang (2011) developed the method based on production-theory decomposition approach (PDA), which is based on output-oriented distance function to decompose the energy production to technology efficiency, technology program, and input alternative. Lin and Du (2014) gave a complex framework (L-D framework) of index decomposition and production theory. Then, Yang et al. (2019) used L-D framework to calculate CO2 emissions of major industries.
There are two gaps in the above literature. Factor choice is confused by economic methods which do not support all factors (Shi et al., 2020). So, the studies always try to select one or two important factors. Actually, factors framework is a hierarchical structure, and they inevitably influence each other. Methodologies reviewed above are very useful and have been adopted with many successes. However, there are many restrictions such as collinearity and causality issues of variables. On the other hand, it is not necessary to consider these issues in machine learning. Machine learning is a method of data analysis that automates analytical model building. It is a branch of artificial intelligence based on the idea that systems can learn from data, identify patterns, and make decisions with minimal human intervention. Machine learning aims to develop algorithms that can learn and create statistical models for data analysis and prediction. The ML algorithms should be able to learn by themselves, based on data provided, and make accurate predictions, without having been specifically programmed for a given task.
Data and the Variables
CO2 Emissions
The International Panel on Climate Change (IPCC) had introduced three methods of calculating CO2 emissions (Y) from fossil fuel combustion in both stationary and mobile sources. “Method 1” is based on the amount of fuel burned and the emission factor, and it is achievable (Wang et al., 2010). Thus, this method is adopted by this paper accordingly. The method is specified as follows:
In Eq. 1, CO2 represents the amount of carbon dioxide emissions to be estimated; i represents various energy fuels, including coal, coke, coke oven gas, blast furnace gas, converter gas, other gas, crude oil, gasoline, kerosene, diesel, fuel oil, and liquefied petroleum, natural gas, and liquefied natural gas; Ei represents the combustion consumption of various energy sources; NCVi is the average low calorific value of various energy sources, used to convert various energy consumption into energy units (TJ); CEFi represents carbon dioxide emission factor of the energy consumption, which is calculated by Eq. 2:
In Eq. 2, CCi is the carbon content of energy sources. COFi is the carbon oxidation factor of energy sources; usually, the value is 1, which means that the energy is completely oxidized. In this paper, coal and coke are set to 0.99 and the rest is 1 (Chen, 2011). (44/12) is the molecular weight ratio of carbon dioxide to carbon. The CO2 emissions related data are derived from China Energy Statistical Yearbook (2001–2019) and Report of IPCC (2006).
Industrial Structure Rationalization Index
Industrial structure rationalization (X1) reflects the coordination of different industries; moreover, it reflects the efficiency of energy usage (Gan et al., 2011). The Theil index measures the industrial structure rationalization (Gan et al., 2011). The Theil index is defined as the equation below:
TL is the Theil index, Y is GDP, L is employment, i represents industries, and n represents industry sectors. When economy is equilibrium, TL = 0, industrial structure is rational. The industrial structure rationalization index related data are derived from Chinese Statistical Yearbook (2001–2019).
Other Variables and Data
This paper also includes other important variables. They are GDP, urbanization, research and development (R&D) investment, actual use of foreign capital, and growth rate of energy consumption. Data for GDP (X2) and actual use of foreign capital (X5) are derived from Chinese Statistical Yearbook (2001–2019) and statistical yearbook of 30 provinces from 2000 to 2018. Data on urbanization (X3), the share of urban population, is derived from Statistical Yearbooks (2001–2019) for the 30 provinces. Data for R&D reinvestment intensity (X4) are from Statistical Communique on National Science and Technology Expenditures (2000–2018). Data for growth rate of energy consumption (X6) are from China Energy Statistical Yearbook (2001–2019). In summary, there are one output variable and six input variables and annual data of these seven variables of 30 provinces are obtained for 2000–2018 from the sources stated above.
Methodology
This study uses a number of machine learning algorithms, or function, f, to map the output variable (Y) from input variables (X1, X2, … , X6) so that Y = f(X1, X2, … , X6). Several types of algorithms have been adopted in this study, and they are briefly described here.
Linear Models–Linear Regression, Lasso, and ElasticNet
When we make assumptions to the learning process, we can simplify the process a lot. However, they can also limit what can be learned. Algorithms that simplify the function to a known form are called linear models. Examples of this class include linear regression and logistic regression. In this study, we tested three linear models, and they are linear regression (LR), least absolute shrinkage and selection operator (LASSO), and Elastic Net (EN) that adds regularization penalties to the loss function during training. Linear models provide the benchmark to measure other machine learning algorithms. However, it is expected that linear models would not provide good prediction because CO2 emissions are complicated and depend on many factors. Furthermore, it is not expected that those factors relate to CO2 emissions linearly.
Nonlinear Models: Classification and Regression Tree, Support Vector Regression, and k-Nearest Neighbors Regression
When we do not make strong assumptions about the form of the mapping function, the algorithms are called nonlinear models. Examples of this class include Classification and Regression Tree (CART), Support Vector Regression (SVR), and k-Nearest Neighbors (KNN). These models are useful for problems involving datasets with large number of features, many of which may be correlated. As the name implied, CART works for both classification and regression problems. For SVR, as the name suggests, it is a regression algorithm, and it should not be confused with Support Vector Machine (SVM) which is for classification. The major difference between the two is there is only one slack variable in SVM and there are two slack variables in SVR during its optimization for locating the hyperplane. For KNN algorithm, it can be applied for both classification and regression problems. In classification, the algorithm tries to predict the class to which the output variable belongs by computing the local probability, while it tries to predict the values of the output variable by using a local average in regression. One of the strengths of machine learning is that it can work with nonlinear data. If a system is nonlinear (i.e., a system that contains CO2 emissions and its six input variables), nonlinear models would be more appropriate.
Ensemble Methods
Traditionally, machine learning application consisted of a single learner (say, a Decision Tree). Then, ensemble methods were born, which involve using many learners to enhance the performance of any single one of them individually.
Bagging Methods: Random Forest and Extra Trees
Bagging is a method of merging the same type of predictions. The idea of bagging is then simple: we want to fit several independent models and “average” their predictions in order to obtain a model with a lower variance. In bagging, weak learners are trained in parallel using randomness, and each model receives an equal weight. Bagging decreases variance, not bias, and solves overfitting issues in a model.
Boosting Methods: XGBoost, AdaBoost, and Gradient Boost
Boosting models fall inside this family of ensemble methods. Boosting is a method of merging different types of predictions. Boosting decreases bias, not variance. In boosting, models are weighted based on their performance. Boosting should not be confused with bagging. In boosting, the weak learners are trained sequentially.
AdaBoost is a specific boosting algorithm developed for classification problems. The weakness is identified by the weak estimator’s error rate.
Gradient boosting approaches the problem a bit differently. Instead of adjusting weights of data points, Gradient boosting focuses on the difference between the prediction and the ground truth.
XGBoost builds the model by calculating similarity scores between the observations that end up in a node. Also, XGBoost allows for regularization, reducing the possible overfitting of individual trees and therefore of the ensemble model.
Artificial Neural Networks
Neural networks consist of nodes connected by links. They have three types of layers: an input layer with a node for each input, hidden layers where learning occurs in training and inputs are processed on trained nets, and an output layer with a node for each target variable, which passes information outside the network. Learning takes place in the hidden layer nodes, each of which consists of a summation operator and an activation function. Note that for neural networks, the inputs should be scaled (i.e., standardized) to account for differences in the units of the data. This is important as scaling could improve the performance by a considerable margin (Chaudhari, 2019).
In recent times, ANNs have become popular and helpful model for classification, clustering, and pattern recognition in many disciplines (Abiodun et al., 2018). With its versatility, one would expect it will work well. However, neural networks usually require much more data than traditional machine learning algorithms. In fact, the amount of data required depends both on the complexity of the problem and on the complexity of chosen algorithm. Given that the present study has only 400 + rows of panel data, whether this would impose any limitation on the accuracy of this method remains to be seen.
Results
To get the best results, it is necessary to understand the data first by inspecting their descriptive statistics and plotting their histograms. The descriptive statistics and histogram of the original data between 2000 and 2015 are shown in Figure 2A; Table 1, respectively.
Looking at the data, it is revealed that better results could be obtained by taking the logarithm of X2, X4, X5, and Y. The descriptive statistics and histograms of the logarithms of X2, X4, X5, and Y are shown in Figure 2B; Table 2, respectively.
Data scaling is important for some machine learning algorithms, e.g., KNN and ANN, and less critical for some others such as linear regression. For consistency and easy comparison, the second step of data preparation is standardization of data with its mean and standard deviation rather than normalization of data with its maximum and minimum vales. It is because the data are Gaussian-like than bounded by a maximum and minimum as shown in the histograms.
Linear Models: Linear Regression, Lasso, and ElasticNet
Three linear models have been applied to the scaled dataset using k-fold cross validation. There is no formal rule for the choice of k. In the present study, we set k = 5 so that the length of the validation data match that of the testing set (i.e., 2016-2018). The box-and-whisker plot of mean and standard deviation of each validation for the three models are shown in Figure 3. It can be seen that the mean and standard deviation for linear regression model is tighter than the other two linear models. However, after Lasso and ElasticNet models are tuned for their hyperparameters and used to fit the whole set of training data (i.e., without k-fold cross validation), the rmse between the prediction and the actual data of the training set (2000–2015) are all the same at 0.5482. Furthermore, when they are applied to the testing set (2016–2018), the rmse among the three models are practically the same at 0.6732. To show how good the models are, we plot the actual against the prediction in Figure 4. For a good fit, the points should be close to the dotted line. As it can be seen, we can hardly describe that linear models are able to predict CO2 emissions. This prompts us to apply non-linear models accordingly.
Nonlinear Models: Classification and Regression Tree, Support Vector Regression, and k-Nearest Neighbors Regression
Similar to linear models, we applied k-fold cross validation to the three nonlinear models. The box-and-whisker plot of the three models is shown in Figure 5. It can be seen that the performance of SVR and KNN is better than that of CART. The graphs of actual against prediction for SVR and KNN are shown in Figures 6, 7, respectively. It can be seen in Figures 6, 7 that nonlinear models, especially KNN, have done much better in predicting CO2 emissions. In particular, if you compare the actual against prediction graph, you can see the points are much tighter and closer to the dotted lines. The rmse are 0.1750 and 0.3641 for the training set and testing set of data when the number of neighbors is set to 2. This is a remarkable improvement over the linear models.
Ensemble Methods
In this study, five ensemble methods, 2 bagging and 3 boosting algorithms, are applied. As k-fold cross validation randomly divided the dataset, the box-and-whisker plots change every time we run. Figure 8 shows the typical results for four runs. It can be seen that Extra Trees consistently outperformed the other four models in the present study. If we apply Extra Trees algorithm to fit the combined training and validation dataset, we can see it can fit the prediction almost perfectly with the actual data as shown in Figure 9A. However, when it is applied to the testing data in Figure 9B, it gives a rmse of 0.4128 when the number of trees (or estimators) is 20. One thing to note for ET model is that the rmse are relatively stable with respect to the number of trees: the values of rmse are 0.4370, 0.4128, and 0.4155 when the number of trees is 10, 20, and 50, respectively. Though ET model the best among the five ensemble models under study, its performance is not as good as the KNN model discussed above.
Artificial Neural Network
As mentioned in Artificial Neural Networks, there are three types of layers in ANN. To apply ANN, one needs to determine the number of layers and number of neurons used in each layer. On top of the k-fold cross validation that introduces randomness, the stochastic nature of the model results in different output every time we run the model. Therefore, it is necessary to experiment the combination of these parameters to get the best results. As the number of instances of our dataset is only slightly over 400, one hidden layer is sufficient after experimentation. After random search, it is found that the number of neurons should be between 6 and 15 in both input layer and hidden layer. Then, we run the model at least 10 times for each combination of neurons in the input layer and hidden layer. It is found that the best combination is six neurons in the input layer and 10 neurons in the hidden layer. With this configuration of the network, we run the model 30 times. Then, we take the average of the results, which is shown in Figure 10. The values of the rmse of the training and testing data are 0.2430 and 0.4849, respectively. It can be seen that though ANN model performs better than linear models, it is not as good as nonlinear models. Comparatively, its accuracy is only a distant second to KNN model.
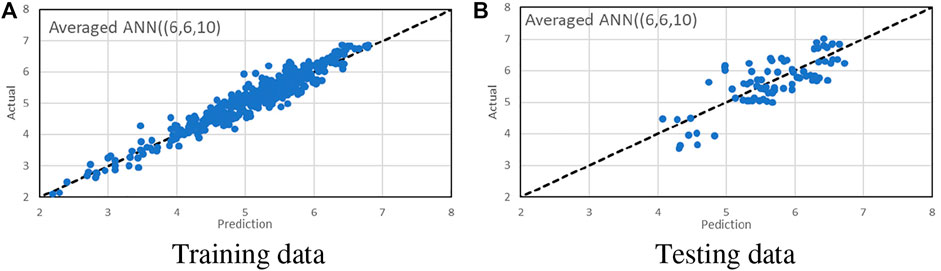
FIGURE 10. Actual vs. prediction of ANN (first layer: six inputs, six neurons; second layer: 10 neurons).
Based on rmse for model selection, the results presented above shows that KNN model performed the best, ANN model achieved a distant second and ET came third in predicting CO2 emissions with the dataset described in Data and the Variables. In the next section, we shall make use of KNN model and perform sensitivity analysis that would enable policy makers in setting policies to reduce CO2 emissions.
Discussions
Having established that KNN model performs the best in the dataset, we attempt to use KNN model to perform sensitivity analysis of independent variables on CO2 emissions. We would like to determine how the target variable, CO2 emissions, is affected based on changes in other input variables. As there are six input variables, we need to select a base case before we conduct sensitivity analysis. The base case consists of the input variables with the most common values. The procedure is described below.
From the histograms, we have divided each variable into 10 bins of equal width that cover the minimum and maximum. For each variable, say X1–Industrial Structure Rationalization, we pick the midpoint value, X1M, of the bin that contains the highest number of data. With six variables, we have X1M, X2M, … and X6M accordingly. Let us call this the “centroid” of input variables.
Now, we can vary the value of one variable, say X1—Industrial Structure Rationalization, from minimum to maximum while keeping the values of other five variables constant at their midpoint values of the highest bin. In this way, we can inspect the sensitivity of variable, X1—Industrial Structure Rationalization around the centroid. We can repeat this analysis to other input variables and form a more complete picture about the six variables affect the CO2 emission around the centroid. The result is shown in Figure 11.
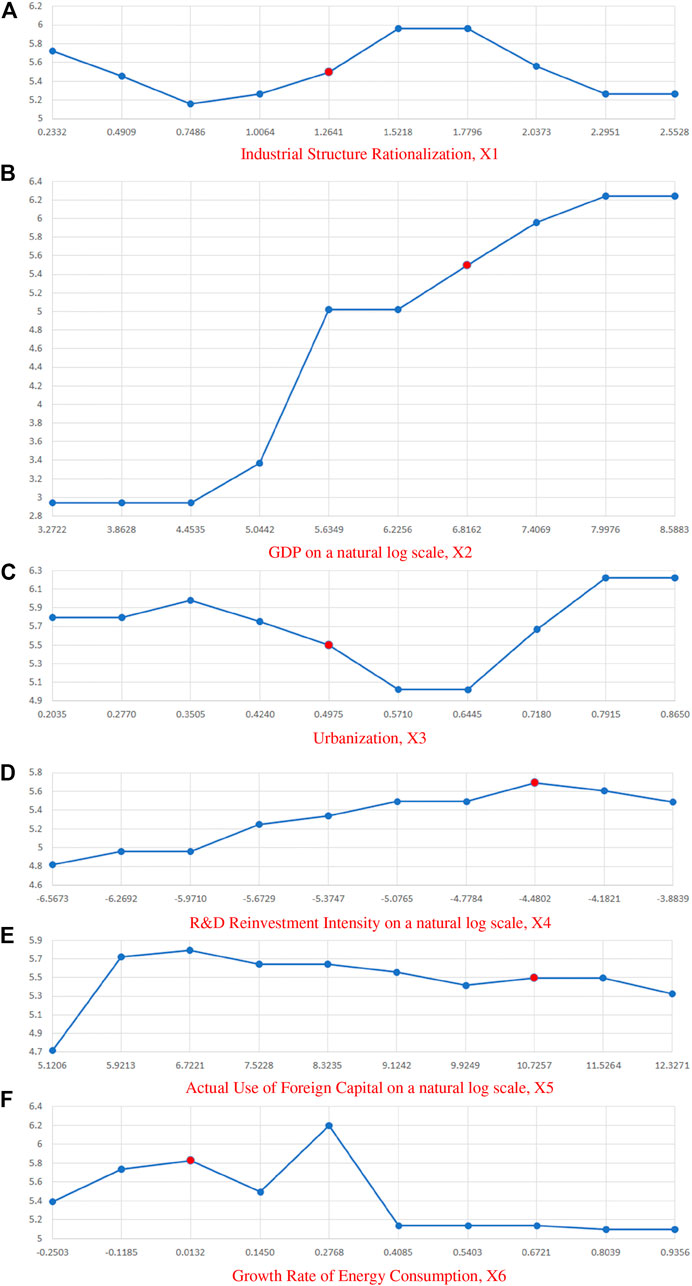
FIGURE 11. Sensitivity analysis of variables around the centroid of KNN (2). represents the centroid of the data ‒ the most populated bin of the data Only one variable varies while keeping the other variables unchanged at centroid values.
First, when all the six variables are at centroid, the predicted Ln(CO2 emissions),Y, is 5.4960 (or equivalent to 543.70 million tonnes CO2 emissions), shown with symbol ○ in Figure 11. Then, when we adjust one of the variables, the change of Ln(CO2 emissions), Y, is summarized in the following.
Industrial Structure Rationalization, X1: The effect on industrial structure rationalization on CO2 emissions is shown in Figure 11A. It can be seen that their relationship is nonlinear and nonmonotonic. It exactly demonstrates the strength of machine learning is able to pick up the nonlinearity of the variables and make better predictions. It can be seen that when the industrial structure rationalization increases from 0.7486 to 1.6507, CO2 emissions increase. Beyond that range, its effect is the opposite. It can be interpreted that industrial structure is not the only target for the policy makers. Industrial rationalization index is the equilibrium in economy; it includes output value, sectors of branch of industry employment, and industrial rationalization. If the industrial structure is rationalized, the industry, especially the output of second and third industry, should be in equilibrium, and the regional disparity should be continuously decreased. But the negative influence of industrialization will lead to the increase of CO2 emissions. It means that the CO2 emissions decreasing not only need economic equilibrium but also need the balance between the industrialization and harmful gas emission. Therefore, policy makers should develop economy of each province as rapid as possible. It is because the CO2 emission will eventually decrease after the saturation point at the postindustrialization stage as explained by Bernardini and Galli (1993). On the other hand, the industrial structure rationalization should stay at 0.7486 for some provinces as their CO2 emissions would be at minimum.
GDP on a natural log scale, X2: The effect on GDP on CO2 emissions is shown in Figure 11B. It can be seen that CO2 emissions are the most sensitive when the range of GDP is from exp(5.0442) to exp(5.6349) (or equivalent to 155.12 billion dollars to 280.03 billion dollars). As mentioned at the beginning of Methodology, X2 is taken logarithmically. Each interval of the x-axis represents 1.8 times of the previous level. Every country would like to develop their economy. Therefore, it would be unlikely that a country would sacrifice economic growth to curb CO2 emissions. Figure 11B shows that CO2 emissions will increase when economy grows. It will definitely harm the environment. Furthermore, China cannot simply grow its economy without considering CO2 emissions. It is because one of the pledges China has committed in Paris Accord is to peak CO2 emissions by 2030. However, with the advancement of technology, it is possible to reduce emissions without economic sacrifice. One thing that must be noted is that in Figure 11B, there is no inverted U-shaped relation between CO2 emissions and economic growth as found by Galeotti et al. (2006). However, we can see that when GDP grows beyond exp(8.5883) (or equivalent to 5,368 billion dollars), CO2 emissions would level off and they could even come down. It means China can fulfill its Paris Accord’s pledges.
Urbanization, X3: The impact of urbanization on CO2 emissions is very mixed and complicated as shown in literature reviewed in Literature Review. While most of the previous studies indicate a positive relationship between urbanization and CO2 emissions, in this study, it is found that a flanged U-shape is observed as shown in Figure 11C. Given that the urbanization is 0.4975 at centroid now, policy makers of China can aim to reduce its CO2 emissions by increasing urbanization to the trough region of 0.571 and 0.6445. This decrease is likely a result of technological innovation in energy usage and efficiency, increasing awareness of environmental issues, and using green technologies (Bekhet and Othman, 2017).
R&D Reinvestment Intensity on a Natural Log Scale, X4: R&D reinvestment intensity stimulates technological advancement, and it also affects economic growth. It can be seen from Figure 11D that CO2 emissions increase mildly when reinvestment intensity increases from −6.5673 to its centroid position of −4.4802. Afterwards, it decreases mildly. Looking at the figure, the reinvestment intensity is at critical moment now at its centroid position. If it decreases from its current position, CO2 emissions would decrease too. But it is likely to be accompanied by a decrease of economic growth. The implication is that China should increase its reinvestment intensity so that it could advance technology more rapidly, increase energy usage and efficiency, and make contribution in reducing CO2 emissions accordingly.
Actual Use of Foreign Capital on a Natural Log Scale, X5: The impact of actual use of foreign capital on CO2 emissions is shown in Figure 11E. It is observed that CO2 emissions increase rapidly when actual use of foreign capital increases from 5.1206 to 5.9213. Then, it levels and even decreases gradually, afterwards. According to pollution haven hypothesis, foreign firms in dirty sectors are more likely to relocate pollution activities from developed countries to poorly regulated developing countries to avoid domestic environmental control cost, which directly undermines the environmental interests of recipient countries like China. This implies that the higher the actual use of foreign capital (FDI), the higher the CO2 emissions, termed “direct” mechanism. However, there is “indirect” mechanism that affect the CO2 emissions. Foreign capital could act as a channel for environmentally friendly technologies, more stringent environmental regulations can be designed and implemented in low-emissions provinces to attract clean foreign capital. So the two mechanisms have the opposite effect on CO2 emissions. In China, actual use of foreign capital of most of the provinces has well passed 5.9212 and reached its centroid position of 10.7257 already. The implication is that the impact of actual use of foreign capital is not significant as the CO2 emissions is quite stable around that position.
Growth Rate of Energy Consumption, X6: When the economy is robust and growing, more energy is consumed. Therefore, it will result in higher CO2 emissions. Energy consumption is in an interesting situation now. It is because CO2 emissions are at a local maximum when the growth rate of energy consumption is at 0.0132 as shown in Figure 11F. Interestingly, when the growth rate was higher than 0.0132 during the period under study, CO2 emissions decreased unless the growth rate was too rapid beyond 0.145 level. It could be explained that China has made good use of foreign capital and R&D investment. Therefore, it is expected that cleaner and greener energy such as hydropower and nuclear power have been used when the growth rate increases from 0.0132 to 0.145. Last, but not least, policy makers should refrain from consuming energy beyond a growth rate of 0.145. It is because it can be seen that CO2 emissions increases sharply beyond 0.145 level. Also, the results between 0.5403 and 0.9356 can be ignored as there are only one or two (or even zero) pieces of data of that range.
Noting that the above analysis applies to the centroid position, it provides an overall picture for China as a whole. This approach can also be applied to other position that might be more relevant to individual province.
Conclusion and Policy Implications
Following the pledges China has committed in Paris Accord is to peak CO2 emissions by 2030 and the declaration of the 2060 carbon-neutrality goal of Chinese government; it requires proactive measures to be undertaken to reduce carbon emissions while maintaining continuous economic growth and improving in living standards. Against this background, this paper analyzed the effects of industrial structure rationalization index, GDP, urbanization, R&D reinvestment, actual use of foreign capital, and growth rate of energy consumption on forecasting CO2 emissions.
Data across 30 provincial administrative regions of China from 2000 to 2018 are used for the study. Data from 2000 to 2015 are used as training set, and data from 2016 to 2018 are used as testing set. We apply a suite of machine learning algorithms on the testing set and predict the levels of CO2 emissions for the testing set. Machine learning algorithms include linear and nonlinear models, ensemble methods with boosting and bagging, and artificial neural networks. Employing rmse for model selection, results show that k-nearest neigbors (KNN) model performs the best when the number of neighbors is set to two for the present dataset.
Using KNN model, we conducted a sensitivity analysis of CO2 emissions around its centroid position on its dependent variables. The overall findings revealed that economic growth measured by GDP, X2, contribute to higher CO2 emissions. As China needs to maintain its economic growth to continuously improve living standards, it brings several implications for policy makers when setting policies concerning other variables. First, in terms of industrial structure rationalization, X1, not all provinces should develop its industrialization. Some provinces should stay at relatively mild industrialization stage that their CO2 emissions would be at minimum. For other provinces, they should develop their economy as rapidly as possible. It is because CO2 emissions will eventually decrease after saturation point. Therefore, the duration of high CO2 emissions that comes with industrialization would be as short as possible. Second, in terms of urbanization, X3, there is an optimal range for a province. To minimize CO2 emissions, provinces should try to achieve urbanization around 0.571 and 0.6445. With the range, the CO2 emissions would be at minimum and the decrease is likely a result of technological innovation in energy usage and efficiency. It also suggests that a province should not be too densely populated. Third, the result of R&D reinvestment intensity, X4, suggests that China should increase its reinvestment intensity further. At present, there is a positive relationship between CO2 emissions and reinvestment intensity. Therefore, it seems that monies for R&D reinvestment have not been put, or not enough, into green technology yet. Only when there is a further increase of R&D reinvestment intensity into green technology, there will be a decrease of CO2 emissions. Fourth, it is found that the impact of the actual use of foreign capital, X5, on CO2 emissions is insignificant, relatively speaking, when compared with other variables. If we assume that R&D reinvestment is associated with actual use of foreign capital, policy makers should prioritize the use of foreign capital for R&D investment on green technology. That would reduce CO2 emissions while maintaining economic growth. Last, it is possible to increase the growth rate of energy consumption, X6, gradually if R&D reinvestment and use of foreign capital are directed towards cleaner and green energy sources such as hydropower and nuclear power. Policy makers must refrain from consuming energy beyond a growth rate of 0.1450 for economic growth. Otherwise, CO2 emissions would increase rapidly and may jeopardize the pledges China committed in Paris Accord and the 2060 carbon-neutrality declaration. In summary, the above policy implications provide a blueprint for policy makers for ensuing environmentally sustainability economic development in China.
It is worth noting that the approach applied in this study can easily be replicated for other countries to make better forecasting of CO2 emissions for the future. The major constraint of this approach is the data limitation. For successful application of machine learning, the number of data required is usually more than traditional econometric models. With more data, more advanced machine learning algorithms can be applied to further check the robustness of the findings.
Data Availability Statement
Publicly available datasets were used and analyzed in this study. The sources of data are listed in Data and the Variables section of this paper in details.
Author Contributions
All authors listed have made a substantial, direct, and intellectual contribution to the work and approved it for publication.
Funding
The paper is supported by Program for Innovation Research in Central University of Finance and Economics and by General Project of Beijing Social Science Foundation Research Base (18JDLJB001), Research on Beijing Urban Governance Path Based on the Optimization of Urban Multi-dimensional Spatial Structure.
Conflict of Interest
The authors declare that the research was conducted in the absence of any commercial or financial relationships that could be construed as a potential conflict of interest.
Publisher’s Note
All claims expressed in this article are solely those of the authors and do not necessarily represent those of their affiliated organizations, or those of the publisher, the editors, and the reviewers. Any product that may be evaluated in this article, or claim that may be made by its manufacturer, is not guaranteed or endorsed by the publisher.
References
Abiodun, O. I., Jantan, A., Omolara, A. E., Dada, K. V., Mohamed, N. A., and Arshad, H. (2018). State-Of-The-Art In Artificial Neural Network Applications: A Survey. Heliyon 4, e00938. doi:10.1016/j.heliyon.2018.e00938
Acheampong, A. O. (2018). Economic Growth, CO2 Emissions and Energy Consumption: What Causes what and where? Energ. Econ. 74, 677–692. doi:10.1016/j.eneco.2018.07.022
Akpan, U. F., and Chuku, A. (2011). Economic Growth and Environmental Degradation in Nigeria: Beyond the Environmental Kuznets Curve. Mpra Paper 8 (5), 568–577. doi:10.1108/14777830710778328
Al-mulali, U., Fereidouni, H. G., Lee, J. Y. M., and Sab, C. N. B. C. (2013). Exploring the Relationship between Urbanization, Energy Consumption, and CO2 Emission in MENA Countries. Renew. Sustain. Energ. Rev. 23, 107–112. doi:10.1016/j.rser.2013.02.041
Ang, B. W., and Zhang, F. Q. (2000). A Survey of index Decomposition Analysis in Energy and Environmental Studies. Energy 25 (12), 1149–1176. doi:10.1016/s0360-5442(00)00039-6
Ang, J. B. (2009). CO2 Emissions, Research and Technology Transfer in China. Ecol. Econ. 68 (10), 2658–2665. doi:10.1016/j.ecolecon.2009.05.002
Antweiler, W., Copeland, B. R., and Taylor, M. S. (2001). Is Free Trade Good for the Environment? Am. Econ. Rev. 91, 877–908. doi:10.1257/aer.91.4.877
Bai, Y., Deng, X., Gibson, J., Zhao, Z., and Xu, H. (2019). How Does Urbanization Affect Residential CO2 Emissions? an Analysis on Urban Agglomerations of China. J. Clean. Prod. 209, 876–885. doi:10.1016/j.jclepro.2018.10.248
Behera, S. R., and Dash, D. P. (2017). The Effect of Urbanization, Energy Consumption, and Foreign Direct Investment on the Carbon Dioxide Emission in the SSEA (South and Southeast Asian) Region. Renew. Sustain. Energ. Rev. 70, 96–106. doi:10.1016/j.rser.2016.11.201
Bekhet, H. A., and Othman, N. S. (2017). Impact of Urbanization Growth on Malaysia CO2 Emissions: Evidence from the Dynamic Relationship. J. Clean. Prod. 154, 374–388. doi:10.1016/j.jclepro.2017.03.174
Bernardini, O., and Galli, R. (1993). Dematerialization: Long-Term Trends in the Intensity of Use of Materials and Energy. Futures 25, 431–448. doi:10.1016/0016-3287(93)90005-e
Bertram, C., Keywan Riahi, K., Hilaire, J., Bosetti, V., Drouet, L., Fricko, O., et al. (2021). Energy System Developments and Investments in the Decisive Decade for the Paris Agreement Goals. Environ. Res. Lett. 16 (7). doi:10.1088/1748-9326/ac09ae
Candau, F., and Dienesch, E. (2017). Pollution haven and Corruption paradise. J. Environ. Econ. Manage. 85, 171–192. doi:10.1016/j.jeem.2017.05.005
Cao, Z., Wei, J., and Chen, H. B. (2016). CO2 Emissions and Urbanization Correlation in China Based on Threshold Analysis. Ecol. Indicators 61, 193–201. doi:10.1016/j.ecolind.2015.09.025
Chaudhari, P. (2019). Importance of Feature Scaling for Artificial Neural Networks and K-Nearest Neighbors. Available at: https://medium.com/@piyush.kailash.chaudhari/importance-of-feature-scaling-for-artificial-neural-networks-and-k-nearest-neighbors-4b7aa618d5ea. (Accessed June 5, 2021).
Chen, S. (2011). The Fluctuation and Decrease Mode of China's Carbon Emission Intensity and its Economic Explanation. The J. World Economy 34 (04), 124–143. Available at: https://kns.cnki.net/kcms/detail/detail.aspx?dbcode=CJFD&dbname=CJFD2011&filename=SJJJ201104009&v=PKVIHlLP1q%25mmd2FhF%25mmd2FkK6z80YIy5msaiQr1ZOQco3gj65bo4cCpLmeL1cPGQf9VKfY9q. (Accessed June 5, 2021). doi:10.1111/j.1467-9701.2011.01370.x
Chudik, A., and Pesaran, M. H. (2015). Common Correlated Effects Estimation of Heterogeneous Dynamic Panel Data Models with Weakly Exogenous Regressors. J. Econom. 188 (2), 393–420. doi:10.1016/j.jeconom.2015.03.007
Cole, M. A., and Neumayer, E. (2004). Examining the Impact of Demographic Factors on Air Pollution. Popul. Environ. 26, 5–21. doi:10.1023/b:poen.0000039950.85422.eb
Cui, C., Cai, B., and Wang, G. Z. (2020). Decennary Spatial Pattern Changes and Scaling Effects of CO2 Emissions of Urban Agglomerations in China. Cities 105, 102818. doi:10.1016/j.cities.2020.102818
Du, W. C., and Xia, X. H. (2018). How Does Urbanization Affect GHG Emissions? A Cross-Country Panel Threshold Data Analysis. Appl. Energ. 229, 872–883. doi:10.1016/j.apenergy.2018.08.050
Elliott, R. J. R., Sun, P. S., and Chen, S. (2013). Energy Intensity and Foreign Direct Investment: A Chinese City-Level Study. Energ. Econ. 40, 484–494. doi:10.1016/j.eneco.2013.08.004
Fan, H., Hashmi, S. H., Habib, Y., and Ali, M. (2020). How Do Urbanization and Urban Agglomeration Affect CO2 Emissions in South Asia? Testing Non-linearity Puzzle with Dynamic STIRPAT Model. Chin. J. Urban Environ. Stud. 08 (01), 205000308. doi:10.1142/s2345748120500037
Fan, Y., Liu, L.-C., Wu, G., and Wei, Y.-M. (2006). Analyzing Impact Factors of CO2 Emissions Using the STIRPAT Model. Environ. Impact Assess. Rev. 26 (4), 377–395. doi:10.1016/j.eiar.2005.11.007
Friedl, B., and Getzner, M. (2003). Determinants of CO2 Emissions in a Small Open Economy. Ecol. Econ. 45, 133–148. doi:10.1016/s0921-8009(03)00008-9
Galeotti, M., Lanza, A., and Pauli, F. (2006). Reassessing the Environmental Kuznets Curve for CO2 Emissions: a Robustness Exercise. Ecol. Econ. 57 (1), 152–163. doi:10.1016/j.ecolecon.2005.03.031
Gan, C., Zheng, R., and Yu, D. (2011). An Empirical Study on the Effects of Industrial Structure on Economic Growth and Fluctuations in China. Econ. Res. J. 46 (05), 4–16+31. Available at: https://kns.cnki.net/kcms/detail/detail.aspx?dbcode=CJFD&dbname=CJFD2011&filename=JJYJ201105002&v=jXi17UDWTaaqRHWJh8aSKBVxDK77Pz%25mmd2FC3DruT4vnekzOQ8DZnDbFnuQFv3dcUcCh. (Accessed June 5, 2021).
Grossman, G. M., and Krueger, A. B. (1991). Environmental Impacts of a North American Free Trade Agreement. National Bureau of Economic Research Working Paper. No. 3914. doi:10.3386/w3914 Available at: https://www.nber.org/papers/w3914 (Accessed August 15, 2021).
Grossman, G. M., and Krueger, A. B. (1995). Economic Growth and the Environment. Q. J. Econ. 110 (2), 353–377. doi:10.2307/2118443
Han, X., Cao, T., and Sun, T. (2019). Analysis on the Variation Rule and Influencing Factors of Energy Consumption Carbon Emission Intensity in China's Urbanization Construction. J. Clean. Prod. 238, 124605. doi:10.1016/j.jclepro.2019.117958
Holtz-Eakin, D., and Selden, T. M. (1995). Stoking the Fires? CO2 Emissions and Economic Growth. J. Public Econ. 57, 85–101. doi:10.1016/0047-2727(94)01449-x
Hossain, M. S. (2011). Panel Estimation for CO2 Emissions, Energy Consumption, Economic Growth, Trade Openness and Urbanization of Newly Industrialized Countries. Energy Policy 39 (11), 6991–6999. doi:10.1016/j.enpol.2011.07.042
Kasman, A., and Duman, Y. S. (2015). CO2 Emissions, Economic Growth, Energy Consumption, Trade and Urbanization in New EU Member and Candidate Countries: A Panel Data Analysis. Econ. Model. 44, 97–103. doi:10.1016/j.econmod.2014.10.022
Khan, Z., Murshed, M., Dong, K., and Yang, S. (2021). The Roles of export Diversification and Composite Country Risks in Carbon Emissions Abatement: Evidence from the Signatories of the Regional Comprehensive Economic Partnership Agreement. Appl. Econ., 1–19. doi:10.1080/00036846.2021.1907289
Khan, Z., Sisi, Z., and Siqun, Y. (2019). Environmental Regulations an Option: Asymmetry Effect of Environmental Regulations on Carbon Emissions Using Non-linear ARDL. Energy Sourc. A: Recovery, Utilization, Environ. Effects 41, 137–155. doi:10.1080/15567036.2018.1504145
Lantz, V., and Feng, Q. (2006). Assessing Income, Population, and Technology Impacts on CO2 Emissions in Canada: Where's the EKC? Ecol. Econ. 57, 229–238. doi:10.1016/j.ecolecon.2005.04.006
Li, K., and Lin, B. (2015). Impacts of Urbanization and Industrialization on Energy Consumption/CO2 Emissions: Does the Level of Development Matter? Renew. Sustain. Energ. Rev. 52, 1107–1122. doi:10.1016/j.rser.2015.07.185
Li, Z.-Z., Li, R. Y. M., Malik, M. Y., Murshed, M., Khan, Z., and Umar, M. (2021). Determinants of Carbon Emission in China: How Good Is Green Investment? Sustainable Prod. Consumption 27, 392–401. doi:10.1016/j.spc.2020.11.008
Liddle, B., and Lung, S. (2010). Age-structure, Urbanization, and Climate Change in Developed Countries: Revisiting STIRPAT for Disaggregated Population and Consumption-Related Environmental Impacts. Popul. Environ. 31 (5), 317–343. doi:10.1007/s11111-010-0101-5
Lin, B., and Du, K. (2014). Understanding the Changes in China's Energy Intensity: a Comprehensive Decomposition Framework. J. World Economy 37 (04), 69–87. Available at: https://kns.cnki.net/kcms/detail/detail.aspx?filename=SJJJ201404005&dbcode=CJFQ&dbname=CJFD2014&v=7ZdISdpBS7Ocxj7t_Kly5sUetQA6bDAudZuqf7tsBLFTt4L-55mxiTThz9tEW3Jy. (Accessed June 5, 2021).
Lin, B., and Du, K. (2013). What Is the Driving Force of My Country's Energy Productivity Growth: Based on the Decomposition of Distance Function. J. Financial Res. (09), 84–96. Available at: https://kns.cnki.net/kcms/detail/detail.aspx?filename=JRYJ201309007&dbcode=CJFQ&dbname=CJFD2013&v=gazb_TmM57RsImpyvmwXBe67SvuxDMQJVNSt68dYl5hNcsOm3uxVUvRoF-W2Cott. (Accessed June 5, 2021).
Lin, B., and Jiang, L. (2009). Prediction of Environmental Kuznets Curve of Carbon Dioxide in China and Analysis of Influencing Factors. Manage. World 4, 2736. Available at: https://kns.cnki.net/kcms/detail/detail.aspx?dbcode=CJFD&dbname=CJFD2009&filename=GLSJ200904005&v=hnvCX%25mmd2Bnv89Jb8ZEhkoTGxX8Ufj8SzOC2I8xzaqyApuknmDeyvWbifRExmNIGtEQn. (Accessed June 5, 2021).
Liu, J., Murshed, M., Chen, F., Shahbaz, M., Kirikkaleli, D., and Khan, Z. (2021). An Empirical Analysis of the Household Consumption-Induced Carbon Emissions in China. Sustain. Prod. Consumption 26, 943–957. doi:10.1016/j.spc.2021.01.006
Liu, X., and Bae, J. (2018). Urbanization and Industrialization Impact of CO2 Emissions in China. J. Clean. Prod. 172, 178–186. doi:10.1016/j.jclepro.2017.10.156
Liu, Z., Geng, Y., Lindner, S., and Guan, D. (2012). Uncovering China's Greenhouse Gas Emission from Regional and Sectoral Perspectives. Energy 45 (1), 1059–1068. doi:10.1016/j.energy.2012.06.007
Ma, Q., Murshed, M., and Khan, Z. (2021). The Nexuses between Energy Investments, Technological Innovations, Emission Taxes, and Carbon Emissions in china. Energy Policy 155 (30), 112345. doi:10.1016/j.enpol.2021.112345
Martin, W. (2008). The Carbon Kuznets Curve: A Cloudy Picture Emitted by Bad Econometrics? Resource Energ. Econ. v30, 388–408.
Martinez-Zarzoso, I., and Bengochea-Morancho, A. (2004). Pooled Mean Group Estimation for an Environmental Kuznets Curve for CO2. Econ. Lett. 82, 121–126.
Moomaw, W. R., and Unruh, G. C. (1997). Are Environmental Kuznets Curves Misleading Us? the Case of CO2 Emissions. Envir. Dev. Econ. 2, 451–463. doi:10.1017/s1355770x97000247
Murshed, M., and Dao, N. T. T. (2020). Revisiting the CO2 Emission-Induced EKC Hypothesis in South Asia: the Role of Export Quality Improvement. GeoJournal (8). doi:10.1007/s10708-020-10270-9
Murshed, M., Ali, S. R., and Banerjee, S. (2020). Consumption of Liquefied Petroleum Gas and the EKC Hypothesis in South Asia: Evidence from Cross-Sectionally Dependent Heterogeneous Panel Data with Structural Breaks. Energ. Ecol. Environ. 6 (4), 353–377. doi:10.1007/s40974-020-00185-z
Poumanyvong, P., and Kaneko, S. (2010). Does Urbanization lead to Less Energy Use and Lower CO2 Emissions? A Cross-Country Analysis. Ecol. Econ. 70 (2), 434–444. doi:10.1016/j.ecolecon.2010.09.029
Rahman, M. M., Saidi, K., and Mbarek, M. B. (2020). Economic Growth in South Asia: the Role of CO2 Emissions, Population Density and Trade Openness. Heliyon 6 (5), e03903. doi:10.1016/j.heliyon.2020.e03903
Rehman, A., Ulucak, R., Murshed, M., Ma, H., and Isik, C. (2021b). Carbonization and Atmospheric Pollution in China: The Asymmetric Impacts of Forests, Livestock Production, and Economic Progress on CO2 Emissions. J. Environ. Manage. 294, 1–10. doi:10.1016/j.jenvman.2021.113059
Rehman, A., Ma, H., Ozturk, I., Murshed, M., and Dagar, V. (2021a). The Dynamic Impacts of CO2 Emissions from Different Sources on Pakistan’s Economic Progress: a Roadmap to Sustainable Development. Environ. Dev. Sustain.. doi:10.1007/s10668-021-01418-9
Sachs, J., Panayotou, T., and Peterson, A. (1999). Developing Countries and the Control of Climate Change: A Theoretical Perspective and Policy Implications. CAER Ⅱ Discussion Paper. No. 44. Available at: https://www.semanticscholar.org/paper/Developing-Countries-and-the-Control-of-Climate-%3A-A-Sachs-Panayotou/4a052fa713252eadb020ced77323cbf5c0d7a360 and https://www.earth.columbia.edu/sitefiles/file/Sachs%20Writing/1999/HIIDCAERII_DevelopingCountriesandtheControlofClimateChange_Nov1999.pdf. (Accessed August 15, 2021).
Sadorsky, P. (2013). Do urbanization and Industrialization Affect Energy Intensity in Developing Countries? Energ. Econ. 37, 52–59. doi:10.1016/j.eneco.2013.01.009
Saidi, K., and Hammami, S. (2015). The Impact of CO2 Emissions and Economic Growth on Energy Consumption in 58 Countries. Energ. Rep. 1, 62–70. doi:10.1016/j.egyr.2015.01.003
Shafik, N. (1994). Economic Development and Environmental Quality: an Econometric Analysis. Oxford Econ. Pap. 46, 757–773. doi:10.1093/oep/46.supplement_1.757
Shahbaz, M., Chaudhary, A. R., and Ozturk, I. (2017). Does Urbanization Cause Increasing Energy Demand in Pakistan? Empirical Evidence from STIRPAT Model. Energy 122, 83–93. doi:10.1016/j.energy.2017.01.080
Shahbaz, M., Nasir, M. A., and Roubaud, D. (2018). Environmental Degradation in France: the Effects of FDI, Financial Development, and Energy Innovations. Energ. Econ. 74, 843–857. doi:10.1016/j.eneco.2018.07.020
Shen, X., Chen, Y., and Lin, B. (2021). The Impacts of Technological Progress and Industrial Structure Distortion on China’s Energy Intensity. Econ. Res. J. (02), 157–173. Available at: http://kns.cnki.net/kcms/detail/11...F.20210406.1417.020.html.
Shi, X, K. Wang., Cheong, T. S., and Zhang, H. (2020). Prioritizing driving factors of household carbon emissions: An application of the LASSO model with survey da, 104ta. Energ. Econ. 92942, 1–13. doi:10.1016/j.eneco.2020.104942
Wang, C. (2011). Sources of Energy Productivity Growth and its Distribution Dynamics in China. Resource Energ. Econ. 33 (1), 279–292. doi:10.1016/j.reseneeco.2010.06.005
Wang, F., Wu, L., and Yang, C. (2010). Driving Factors for Growth of Carbon Dioxide Emissions during Economic Development in China. Econ. Res. J. 45 (02), 123–136. Available at: https://kns.cnki.net/kcms/detail/detail.aspx?dbcode=CJFD&dbname=CJFD2010&filename=JJYJ201002011&v=O6IUlfr0xi3lU0SXtaGeZhrF%25mmd2FUmFUiFJAc%25mmd2BkKLVVy2pyXHnkjBYtLEQTuMQiPFLT. (Accessed August 15, 2021).
Wang, P., Wu, W., Zhu, B., and Wei, Y. (2013). Examining the Impact Factors of Energy-Related CO2 Emissions Using the STIRPAT Model in Guangdong Province, China. Appl. Energ. 106, 65–71. doi:10.1016/j.apenergy.2013.01.036
Wang, Z., Yin, F., Zhang, Y., and Zhang, X. (2012). An Empirical Research on the Influencing Factors of Regional CO2 Emissions: Evidence from Beijing City, China. Appl. Energ. 100, 277–284. doi:10.1016/j.apenergy.2012.05.038
Wei, W. X., Yang, F., and Iang, F. (2010). Impact of Technology advance on Carbon Dioxide Emission in China. Stat. Res. 27 (07), 36–44. doi:10.19343/j.cnki.11-1302/c.2010.07.006
Yang, L., Zhu, J., and Jia, Z. (2019). Influencing Factors and Current Challenges of CO2 Emission Reduction in China: a Perspective Based on Technological Progress. Econ. Res. J. 54, 118–132. Available at: https://kns.cnki.net/kcms/detail/detail.aspx?dbcode=CJFD&dbname=CJFDLAST2020&filename=JJYJ201911009&v=ffsbFCXZ%25mmd2BXHaBhuX5uzpMjUQQJLF2u2ENdBwXz9hFkKQ1VS5NSK9k258gY9lErsg. (Accessed June 5, 2021).
Yang, L., and Li, Z. (2017). Technology advance and the Carbon Dioxide Emission in China - Empirical Research Based on the Rebound Effect. Energy Policy 101 (FEB.), 150–161. doi:10.1016/j.enpol.2016.11.020
York, R., Rosa, E. A., and Dietz, T. (2003). STIRPAT, IPAT and ImPACT: Analytic Tools for Unpacking the Driving Forces of Environmental Impacts. Ecol. Econ. 46 (3), 351–365. doi:10.1016/s0921-8009(03)00188-5
Young, A. (1998). Growth without Scale Effects. J. Polit. Economy 106 (1), 41–63. doi:10.1086/250002
Yu, Y., and Du, Y. (2018). Impact of Technological Innovation on CO2 Emissions and Emissions Trend Prediction on ‘new normal' Economy in China. Atmos. Pollut. Res. 10, 152–161. doi:10.1016/j.apr.2018.07.005/
Yu, J., Shi, X., Guo, D., and Yang, L. (2021). Economic Policy Uncertainty (EPU) and Firm Carbon Emissions: Evidence Using a China Provincial EPU Index. Energy Economics 94, 105071.
Zhang, C., and Lin, Y. (2012). Panel Estimation for Urbanization, Energy Consumption and CO2 Emissions: A Regional Analysis in China. Energy Policy 49, 488–498. doi:10.1016/j.enpol.2012.06.048
Zhu, H.-M., You, W.-H., and Zeng, Z.-f. (2012). Urbanization and CO2 Emissions: A Semi-parametric Panel Data Analysis. Econ. Lett. 117 (3), 848–850. doi:10.1016/j.econlet.2012.09.001
Keywords: Machine learning, CO2 emissions, economic growth, industry structure, forecasting
Citation: Li S, Siu YW and Zhao G (2021) Driving Factors of CO2 Emissions: Further Study Based on Machine Learning. Front. Environ. Sci. 9:721517. doi: 10.3389/fenvs.2021.721517
Received: 07 June 2021; Accepted: 22 July 2021;
Published: 23 August 2021.
Edited by:
Xunpeng (Roc) Shi, University of Technology Sydney, AustraliaReviwed by:
Muntasir Murshed, North South University, BangladeshJianfu Shen, Hong Kong Polytechnic University, Hong Kong, China
Feng Dong, China University of Mining and Technology, China
Copyright © 2021 Li, Siu and Zhao. This is an open-access article distributed under the terms of the Creative Commons Attribution License (CC BY). The use, distribution or reproduction in other forums is permitted, provided the original author(s) and the copyright owner(s) are credited and that the original publication in this journal is cited, in accordance with accepted academic practice. No use, distribution or reproduction is permitted which does not comply with these terms.
*Correspondence: Yam Wing Siu, ywsiu@hsu.edu.hk