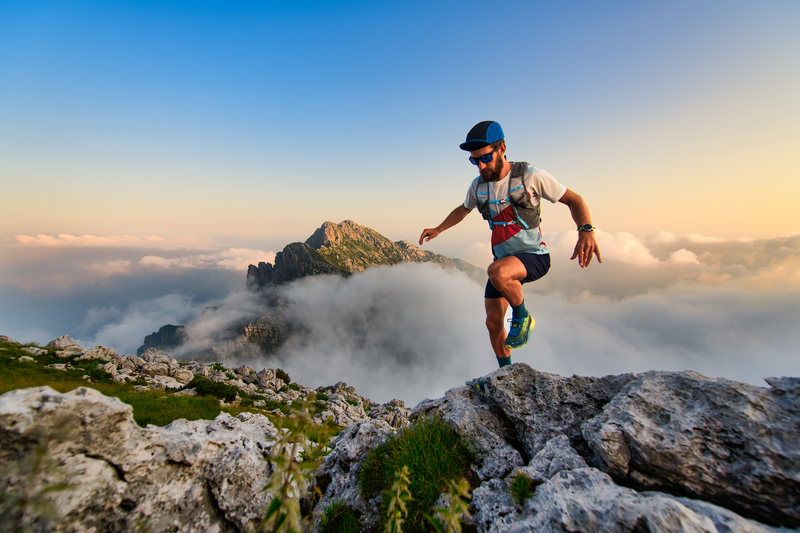
94% of researchers rate our articles as excellent or good
Learn more about the work of our research integrity team to safeguard the quality of each article we publish.
Find out more
ORIGINAL RESEARCH article
Front. Environ. Sci. , 29 March 2021
Sec. Toxicology, Pollution and the Environment
Volume 9 - 2021 | https://doi.org/10.3389/fenvs.2021.641497
This article is part of the Research Topic Soil and Sediment Pollution, Processes and Remediation View all 10 articles
Due to the irreversibility of heavy metal pollution, the presence of heavy metals in farmland soil is associated with severe ecological risks that endanger both the environment and human health. Cadmium (Cd) and mercury (Hg) are two toxic heavy metals found widely in polluted soil. Cd is not readily fixed in the soil and is therefore easily accumulated by plants, while Hg has a wide range of pollution sources. The aims of this study were to explore the spatial variation in Cd and Hg concentrations in farmland soil in Poyang Lake Plain, China, and to assess their potential ecological risks as influenced by natural and human factors. A total of 283 soil samples were obtained from Fengcheng city, central Jiangxi Province. Data were then analyzed using geostatistics, the potential ecological risk index, Pearson’s correlation analysis, and Geodetector. The results showed moderate variation in soil Cd and Hg concentrations, with a remarkable difference in their spatial distribution. Cd concentrations in the northwest and northeast of Fengcheng were below the regional background level in Jiangxi; in most remaining areas, Cd concentrations were between the regional background level and national risk screening value. Areas with Hg concentrations lower than the regional background level were largely concentrated in the south, east and north of Fengcheng, and gradually increased towards the central, where they exceeded the regional background level but were below the national risk screening value. Overall, the potential ecological risk level of Cd was predominantly low, while that of Hg was moderate. The comprehensive potential ecological risk was low in most areas for both Cd and Hg, with some scattered areas of moderate risk. Moreover, the comprehensive potential ecological risk index of both Cd and Hg was significantly correlated with soil pH, total phosphorous, elevation, distance from a river (p < 0.01), and distance from a road (p < 0.05). The most significant factor influencing the comprehensive potential ecological risk index of these two heavy metals was soil pH of 5.2–5.6, followed by total p ≤ 0.52 mg kg−1. In conclusion, moderate pollution of Cd and Hg occurred in farmland soil in Poyang Lake Plain where their comprehensive potential ecological risk level was generally low and mainly influenced by soil pH and total phosphorous.
Heavy metals are an inherent component of soil, and there are more or less heavy metals in soils with different parent materials. However, human factors such as industrial development and the application of pesticides and fertilizers have led to the accumulation of heavy metals in farmland soil and subsequent heavy metal pollution (Aelion et al., 2008; Chabukdhara and Nema, 2013; Kelepertzis, 2014). Sources of heavy metal pollution in farmland soils are diverse, possibly from industry, sewage irrigation, urban activities or general agricultural practices (Jiang et al., 2018). The level of heavy metal pollution in farmland soil is closely associated with the quality and safety of agricultural products as well as severe ecological risks related to the toxicity, persistence, and bioaccumulation characteristics of heavy metals (Barlas et al., 2005; Ihedioha et al., 2016; Zhou et al., 2020). By entering the food chain, heavy metal pollutants also have an eventual effect on human health (Mazej et al., 2010; Hu et al., 2017; Liu et al., 2019). Research on the sources, spatial distribution and risk assessment of heavy metals in soil has attracted wide attention from governments and scientists (Yuan et al., 2015; Zhao and Luo, 2015).
The spatial distribution and distribution characteristics of heavy metal content in farmland soil are not only related to soil texture, physicochemical properties and structural conditions in the region, but also closely related to human activities (An et al., 2016; Lü et al., 2018), and by studying the spatial distribution and characteristics of heavy metals in farmland, it is helpful to find the causes and future development trends of their characteristics. There are many methods to study the spatial distribution of heavy metals in soil (Loredo et al., 2003; Cai et al., 2019). In some studies, assessment of spatial distribution of heavy metals in soils using multiple linear regression and neural network genetic algorithm based on satellite images (Arman et al., 2017). In more studies, spatial interpolation methods (such as ordinary Kriging and inverse distance weight method) are used to describe the spatial distribution characteristics of heavy metals in soil. By drawing contour maps to describe the spatial distribution of metals, for example, it was found that heavy metals in urban and industrial soils were significantly higher than in residential and private areas (Imperato et al., 2003); Spatial analysis of heavy metal concentration in soil by spatial interpolation in order to further study the influencing factors of spatial distribution characteristics of heavy metals (Ding et al., 2016; Wang et al., 2019).
Risk is fuzzy, grey and uncertain. Many studies on the risk of heavy metal pollution have been carried out in different countries and regions to assess the degree of pollution in sediments by using geoaccumulation index methods (Raj and Jayaprakash, 2008); for the polluted environment, especially urban soil, the impact of soil heavy metal pollution on urban population is analyzed by health risk assessment method (Tepanosyan et al., 2017); soils in industrial cities are most vulnerable to heavy metal pollution. Single pollution index and Nemerow comprehensive pollution index are used to evaluate the degree of heavy metal pollution (Hu et al., 2018). Potential ecological risk index method is proposed by Hakanson (1980) to evaluate the ecological risk of heavy metals in aquatic sediments. It can effectively combine the ecological, environmental and toxicological effects, and consider the biological toxicity of heavy metals (Hakanson, 1980; Chen et al., 2017). It is a widely used and reliable method in heavy metal pollution assessment. Principal component analysis is often used in the study of risk factors (Facchinelli et al., 2001; Lv et al., 2015). Geodetectors are a set of statistical methods to detect spatial variability and reveal the driving forces behind them, and they also can detect both numerical data and qualitative data, and are ideal methods to explore risk factors. Geodetectors have two advantages. One is that they can detect both numerical data and qualitative data without linear assumption. The other is that they can detect the interaction of two factors with dependent variables. This method has a clear physical meaning (Wang and Xu, 2017; Qiao et al., 2019).
Poyang Lake Plain is an important area of food production in China, with the quality of farmland soil in the region closely related to the environmental quality of the lake (Jiang et al., 2018), and Fengcheng is one of the important grain production bases. With the increasing demand for food products, the rapid economic development of Poyang Lake Urban Agglomeration, the excessive use of pesticides and a large number of heavy metal sewage irrigation tillage soil have aggravated the pollution load in some regions of the region (Zhang et al., 2017; Dai et al., 2018). Heavy metal pollution in farmland is a serious threat to the sustainability and safety of food crop production in the Poyang Lake Plain, which is a potential obstacle to food trade in the region (Jiang et al., 2018). However, despite these findings, the research scope of existing studies remains extensive. Poyang Lake Plain includes Fengcheng and an additional 24 counties, all of which vary in natural conditions and artificial factors that could potentially affect the spatial variation in heavy metals in farmland soil. The ecological risk of heavy metals in these areas has also yet to be revealed, with factors influencing the potential ecological risk again varying between regions. Thus, existing research on the spatial variation in heavy metals, their potential ecological risks and associated influencing factors in farmland soil in Poyang Lake Plain is insufficient. Further research into the spatial distribution of heavy metals and identification of the factors influencing their potential ecological risks is therefore important in terms of management and control, agricultural sustainability and eco-environmental safety, as well as protection of human health on a regional scale.
In this study, we investigated the distribution and spatial differentiation characteristics of Cd and Hg in farmland soil of Fengcheng City in the Poyang Lake Plain, and simulated the spatial structure and change of heavy metals in farmland soil by geostatistics combined with semi-variogram (such as ordinary Kriging estimation), which has the advantage of visualization for the spatial distribution and uncertainty analysis of heavy metals (Ha et al., 2014). Potential ecological risk assessment of heavy metals based on biotoxicological and environmental effects using potential ecological risk index method. Compared with the general study on the factors affecting the spatial distribution of heavy metals, this study used correlation analysis and Geodetector principle to explore the risk factors and their influence degree of potential ecological risks of heavy metals, and comprehensively considered the influencing factors of natural background of farmland soil and human activities, so as to provide insight into heavy metal pollution in Poyang Lake Plain, which will aid the prevention and control of farmland soil pollution as well as the utilization and protection of agricultural resources on a regional scale.
The study was conducted in Fengcheng city, central Jiangxi Province, China, in the middle and lower reaches of Ganjiang River, at the southern margin of Poyang Lake Basin (between 115°25′–116°27′ E and 27°42′–28°27′ N). Fengcheng, a pilot city directly under provincial government control, is the main food production base in China and one of the first National Pilot Demonstration Zones under the Sustainable Agricultural Development Plan. The total area of cultivated land is about 10.87 million hm2, which is a population-intensive agricultural area. Fengcheng belongs to the subtropical humid monsoon climate, the annual average precipitation is about 1552.1 mm, the annual average temperature is 15.3–17.7°C, frost-free period is about 274 days. The terrain is high in the south and low in the north, gradually tilting from southwest to northeast. Low mountainous areas are distributed in the south, while the central part is relatively low and flat, where Ganjiang River forms a valley floodplain. Meanwhile, undulating hills dominate the northwest and southeast. The total land area of Fengcheng is 284,500 hm2. The geographic location of the study region is shown in Figure 1.
FIGURE 1. Geographical location of the study region in Poyang Lake Plain and the distribution of sampling points in Fengcheng, Jiangxi Province, China.
Soil sampling was conducted in October 2018 according to the principle of uniform distribution and increased sampling point density in typical areas, with additional consideration given to both economic efficiency and feasibility. A total of 283 sampling points were distributed among regular 1 × 1 km grids throughout the region, with surface soil samples collected at depths of 0–20 cm. Three to five replicate samples were taken from each grid and mixed to form a composite sample. A handheld global positioning system (HD8200X-GPS, Zhonghaida, Guangzhou, China) was used to record the latitude and longitude of each sampling point. The distribution of the sampling points is also shown in Figure 1.
The soil samples were air-dried, with impurities removed by hand, then ground and sieved before heavy metal analysis. Cd concentrations were measured by graphite furnace atomic absorption spectrophotometry (GFAAS-AA800, Perkin Elmer Inc., Waltham, MA, United States) and Hg concentrations were measured by cold atomic absorption spectrophotometry (AFS-9530, Haiguang Instrument Co., Ltd., Beijing, China).
Eleven outliers over three standard deviations were eliminated, giving a final 271 samples for data analysis. The Kolmogorov-Smirnov test for normality was conducted using SPSS 22.0 (IBM SPSS, Somers, NY, United States); data that did not conform to the normal distribution were transformed into normal distribution using the logarithm. Correlation analyses between heavy metal concentrations and associated influencing factors were also performed using SPSS 22.0.
Semi-variogram analysis and model fitting were performed with GS + version 7.0 (Gamma Design Software, LLC., Plainwell, United States). Optimal model parameters were obtained based on the maximum coefficient of determination (R2) and the minimum residual sum of squares (RSS). Ordinary Kriging interpolation was then performed according to the optimal model parameters, Ordinary Kriging is the most commonly used geostatistical interpolation method, which provides the parameters required for spatial interpolation, and uses the semi-variogram to quantify the spatial variation of regional variables and the spatial distribution of heavy metals in the study region was mapped using ArcGIS 10.2 (ESRI Inc., Redlands, CA, United States).
Geostatistics was used to determine the spatial variation of Cd and Hg concentrations. Geostatistics is a tool that describes the spatial correlation of regionalized variables using semi-variograms (Ha et al., 2014). The semivariogram, also known as the semivariance, represents the spatial variation and correlation with regionalized variables. It is a key parameter in studies of the spatial variation in soil properties, forming the basis for accurate ordinary Kriging interpolation (Xie et al., 2010). The formula is as follows:
where r(h) is the semivariogram, h is the separation distance, N(h) is the pairwise number of data points separated by distance h, Z(x) is the value of a regionalized variable at spatial position x, and Z (x + h) is the value of a regionalized variable at position x + h.
In the spatial structure of regionalized variables, the coefficient of variation (CV) represents dispersion between data, reflecting spatial variation in a set of samples (Kasel et al., 2017). In general, CV < 10% indicates low variation, CV = 10–100% indicates moderate variation, and CV ≥ 100% indicates high variation (Zhong et al., 2007). The nugget/sill ratio C0/(C0 + C) represents the ratio of random variation to total spatial variation, reflecting the spatial autocorrelation between variables. C0/(C0 + C) < 25% represents high spatial correlation, in which non-human structural factors play a major role; C0/(C0 + C) = 25–75% represents moderate spatial correlation affected by both natural and human factors; and C0/(C0 + C) > 75% indicates low spatial correlation, in which random factors play a major role (Lei et al., 1985; Tang, 2007).
The ecological risk of heavy metal pollution was assessed using the potential ecological risk index proposed by Hakanson (1980), which is based on the background value of heavy metals in the soil (China National Environmental Monitoring Center (CNEMC), 1990; He and Xu, 2006). The risk index value is then calculated from the biotoxicity (toxicity coefficients) and environmental effect (pollution index) of the heavy metal in question as follow:
where
In this study, the toxicity coefficients of Cd and Hg were 30 and 40 (Xu et al., 2008), regional background levels of soil Cd and Hg in Jiangxi were 0.108 and 0.084 (China National Environmental Monitoring Center (CNEMC), 1990), and the risk screening values of Cd and Hg for soil pollution of agricultural land in China were 0.3 and 0.5 (National Standard of the People's Republic of China, 2018), respectively.
Spatial variation is one of the basic characteristics of geographical phenomena. Geodetector refers to a series of statistical methods for detecting spatial differentiation and revealing the driving forces behind them (Wang and Xu, 2017). First, we adopted the factor detector to quantify the relative importance of particular factors in explaining spatial variation in the potential ecological risks of heavy metals, as measured by the q statistic:
where q is the relative influence of a particular factor on the spatial distribution of heavy metal concentrations in soil (q = 0–1), h = 1,…, L is the class of influencing factors, Nh and N are the number of units in class h and the study area, respectively, σk2 and σ2 are the variance in heavy metal concentrations in class h and the study area, respectively, and SSW and SST are the within sum of squares and the total sum of squares, respectively.
The q value satisfies the non-central F∼(L-1, N-L; λ) distribution as follows:
The risk detector was then used to determine whether there were significant differences between the attribute means of two sub-areas, as measured by the t statistic:
where
The null hypothesis was H0:
According to existing research, and taking into account data availability and accessibility, 14 factors that could influence the potential ecological risks of Cd and Hg in farmland soil in the study region were selected. These were soil properties: soil type, texture, pH, organic matter, total nitrogen (N), total phosphorous (P), and total potassium (K); topographical factors: slope and elevation; and distance factors: distance of sampling points from rivers, residents, roads, railways, and mining lands. All factors were extracted from vector data based on existing studies.
The descriptive statistics of Cd and Hg concentrations in farmland soil in Fengcheng are listed in Table 1. There was a remarkable difference between the maximum and minimum concentrations of the two heavy metals. The original Cd and Hg concentrations did not follow a normal distribution, but after processing, had an approximate lognormal and a lognormal distribution, respectively. According to their CV values, Cd and Hg concentrations in the study area show moderate variation.
The optimal models and fitting parameters of Cd and Hg concentrations in the study region are summarized in Table 2. The optimal models for both heavy metals were exponential. Nugget/sill ratios for both Between 25 and 75%, indicating that their concentrations were moderate spatial autocorrelation, and are affected by random factors such as human activities and structural factors. The range of Cd was larger than that of Hg, suggesting that Cd has greater spatial correlation than Hg. The R2 values of Hg and Cd were 0.766 and 0.609 respectively, indicating that the goodness-of-fit of the semi-variagram was better for Cd than H.
TABLE 2. Optimal models and fitting parameters for Cd and Hg concentrations in farmland soil in the study region.
The semivariogram can be used to explain the spatial structure of heavy metal concentrations, thereby reflecting spatial variation; however, the information provided by this function has limitations (Wang et al., 2014). To further characterize the spatial distribution of Cd and Hg concentrations in the study region, we therefore conducted ordinary Kriging interpolation according to the optimal semivariogram models. The spatial distributions of the two heavy metal concentrations are shown in Figure 2, revealing remarkable differences between Cd and Hg in farmland soil throughout the study region.
In ArcGIS-Geostatistical Analyst tool, the training set and validation set of sampling points are selected by subset tool, which are used to represent the cross-validation results of ordinary Kriging interpolation (Table 3). The root mean square errors of the two are 0.02, 0.03, and the interpretation coefficients are 0.76, 0.85, respectively. The test shows that the prediction accuracy is high and the interpolation results are reliable (Jiang and Guo, 2019).
It can be found from Figure 2 that the distribution differences of Cd and Hg in the whole city are obvious. A small part of Cd content less than the background value of soil elements in Jiangxi Province is mainly concentrated in the northwest and northeast of the city, and the distribution is more dispersed. The main distribution of Cd content exceeding the soil pollution risk screening value of agricultural land in China is in the central and southeast regions of the study area,it can also be seen from the map that Cd content in most areas of the city exceeded the background value of soil elements in Jiangxi Province but did not reach the level of risk screening value, mainly concentrated in the 0.136–0.185 range. Hg in Fengcheng City did not exceed the national screening value of soil pollution risk of agricultural land, but most exceeded the background value of Hg in Jiangxi Province. The high value areas were mainly distributed in the central part of Fengcheng City, mainly concentrated in the range of 0.099–0.133. Distribution of Hg within background values of soil in Jiangxi Province in southern, eastern and northern parts of the study area, most of the rest exceed the background value of Jiangxi Province, but are still in the range of risk screening values. From the spatial distribution of Cd and Hg, it can be seen that the high value area of Cd is close to the city center, which is closely related to human activities, and its influence range may pose a threat to farmland grain production and ecological environment safety around the city, however, the distribution of Hg is relatively dispersed, and it does not have a certain concentration as Cd, which is related to its main source of pesticide and fertilizer application.
Using the potential ecological risk index results, the sampling points were classified into different risk levels (Figure 3), with the number of sampling points associated with different risk levels shown in Figure 4. The risk index of both Cd and Hg was categorized into four levels each. The risk level of Cd was generally low (level 1) at 141 sampling points, accounting for 52.03% of total sampling points, with only one point showing a high level of risk (level 4). Meanwhile, the potential ecological risk level of Hg was moderate (level 2) at 161 sampling points, accounting for 59.41% of total sampling points, with only 0.74% of points represented by a high risk level (level 4).
FIGURE 4. Zonation of comprehensive potential ecological risk levels of Cd and Hg in farmland soil across the study region.
Based on the potential ecological risk index of heavy metals Cd and Hg, the comprehensive potential ecological risk index of each sample point was calculated. The zoning map of soil comprehensive potential ecological risk index in the study area was made by ArcGIS 10.2 (ESRI Inc., Redlands, CA, United States) software (Figure 4). It is not difficult to see that the comprehensive potential ecological risk index of soil in the study area is between 32.38 and 217.36. From the spatial distribution, moderate (150 ≤ RI < 300) and intensity (300 ≤ RI < 600) potential ecological risk areas are mainly distributed in the central region of the city. According to the grid reclassification statistics, the regions with slight potential ecological harm (150 ≤ RI < 300) were the majority, accounting for 86.19%. The lowest potential ecological risk index showed a relatively dispersed block distribution in spatial distribution, and the moderate (150 ≤ RI < 300) and intensity (300 ≤ RI < 600) potential ecological risk areas were also dispersed. The overall potential ecological risk of heavy metals in the soil of the study area was relatively low.
Spatial variation analysis revealed that the distribution of Cd and Hg concentrations in farmland soil in the study region was influenced by external factors; however, these associated factors are complex and diverse. Land use patterns and vegetation cover types are important factors controlling spatial distribution and accumulation of heavy metals in soils at site, county and watershed scales. To identify the different factors influencing the potential ecological risks, we first conducted correlation analysis between the risk index of Cd and Hg, the comprehensive risk index, and the 14 associated factors (Table 4). Four factors (soil texture, soil type, total N, and distance from residential areas) had no significant correlation with the risk index of Cd and Hg or the comprehensive risk index; however, the remaining 10 factors were all significantly correlated with at least one index.
TABLE 4. Correlations between the potential ecological risk of Cd and Hg and associated influencing factors in farmland soil in the study region.
The potential ecological risk coefficient of Cd was significantly correlated with pH and negatively correlated with Total K (p-values < 0.01), and the distance from the road showed a significant negative correlation (p-values < 0.05). Meanwhile, the potential ecological risk coefficient of Hg element was negatively correlated with total phosphorus, slope, elevation and distance to the river (p-values < 0.01), as well as with organic matter distance from a railway and distance from a mining area (p-values < 0.05). The comprehensive potential ecological risk index of soil showed a significant positive correlation with pH, while negative correlation were found with Total P, elevation and distance to river (p-values < 0.01), and a significant negative correlation with distance to road (p-values < 0.05).
The factor detector of Geodetector was subsequently used to measure the relative influence of the selected factors on the potential ecological risks of Cd and Hg (Table 5). The explanatory power of the top six factors influencing the potential ecological risk of Cd was ranked as follows: pH > distance from a road > distance from a river > soil texture > slope > total K; however, only the effects of pH were significant (p < 0.05). Meanwhile, the explanatory power of the top six factors influencing the potential ecological risk of Hg was as follows: distance from a railway > total P > slope > distance from a river > elevation > organic matter, but only the effect of total P and distance from a railway was significant (p < 0.05). In terms of the comprehensive potential ecological risk index of heavy metals, total P and total P had the greatest explanatory power (p < 0.05).
TABLE 5. The explanatory power and statistical significance of the top six major factors influencing the potential ecological risks of Cd and Hg in farmland soil in the study region.
The results obtained using the risk detector showed that the most significant factor influencing the potential ecological risk of Cd was a soil pH of 5.2–5.6. The most significant factor influencing the potential ecological risk of Hg was distance from a railway >6004.02 mm, while the second was total p ≤ 0.52 mg kg−1. The most significant factor influencing the comprehensive potential ecological risk was soil pH of 5.2–5.6 followed by p ≤ 0.52 mg kg−1.
Potential ecological risk index method introduces the toxic coefficient and links the ecological and environmental effects of heavy metals with their toxicology. This index can therefore be used to comprehensively reflect the potential impacts of Cd and Hg on the ecological environment of farmland soil, providing useful information for the management and protection of soil environments as well as human health. Remarkable differences in the spatial distribution of Cd and Hg concentrations were observed throughout the study region. The results show that a handful of areas with Cd concentrations below the regional background level were found in the northwest and northeast; however, most other areas had a soil Cd concentration above this background level, with only a few areas in the central and southeast possessing a soil Cd concentration higher than the national risk screening value. Meanwhile, those with a Hg concentration lower than the regional background level distributed in the southern, eastern and northern parts of the country, and remaining areas with concentrations between the regional background level and national risk screening value, gradually increasing toward the central.
According to the land-use map of Fengcheng (Figure 5), urban road traffic networks are mainly concentrated in central and northern areas, while emerging industrial parks, mainly featuring new energy, photovoltaic electronics, and machinery industries, are located in the center of the city. Cd and Hg have therefore accumulated in this region as a result of human factors, and that is consistent with Zhou's conclusion that compared with natural factors, human activities release more partition content into soil, which is more likely to affect crop growth retardation (Zhou et al., 2020; Boussen et al., 2013). The area with the highest Cd concentration was located near the dense urban roads, close to mining land. In addition to soil parent material, this Cd was therefore deemed to have originated from vehicle exhaust emissions and smelting, this is consistent with Yang’s findings that major sources of cadmium and mercury come from human activities and from transport and industrial activities (Yang et al., 2020). A small amount of Cd was thought to be related to a combination of accidental factors such as field sampling, laboratory test errors. Low Hg concentrations were found in the south and east of Fengcheng, far from industrial parks and road traffic, were there is less of an effect from human factors. These results suggest that Cd and Hg detected in farmland soil in the study region are derived from external sources, notably human activities such as industrial production, agricultural fertilization, and residential areas. Over time, these heavy metal concentrations could therefore exceed their respective regional background levels, resulting in heavy metal pollution (Shi et al., 2019).
The potential ecological risks of Cd and Hg at sampling points across the study region were categorized into four levels. For Cd, the number of sampling points with a high risk level (level 4) was low, the majority being associated with a low risk level (level 1). For Hg, the majority of sampling points were associated with a moderate risk level (level 2). The potential ecological risks of Cd and Hg in humans are well known. For example, Cd is known to enter the human body through the food chain, causing damage to the kidneys, brain, bones and nervous system, and resulting in acute or chronic poisoning and even cancer (Sriramachari and Jain, 1997; Bandara et al., 2008). Meanwhile, Hg is easily absorbed by human skin, as well as via the respiratory tract and digestive system, with bioaccumulation causing damage to the brain and liver, again posing serious health risks (Zhang et al., 2018). The Hg concentrations of farmland soil in the study region were lower than those of Cd; however, the potential ecological risk of Hg was higher. Reasonable precautions should therefore be taken to prevent Hg input and decrease the potential ecological risk level. The comprehensive potential ecological risk of both Cd and Hg was generally low in farmland soil throughout the study region; however, the comprehensive risk level in central parts was higher than in the south and north, mainly because this area is more heavily influenced by human activities such as industry and transportation.
The potential ecological risk of Cd and Hg in farmland soil in the study region was found to be influenced by both natural and human factors. Human activities can change soil pH and fertility, thereby affecting the migration and transformation of heavy metals in the soil, and ultimately influencing the potential ecological risk. Based on a long-term field experiment, Chen et al. revealed that the application of P fertilizer was the main factor leading to an increase in Cd and Hg concentrations in farmland soil (Chen and Dong, 2005). In addition, topographic factors such as slope were also found to have a direct effect on the distribution and redistribution of water and thermal resources (Wei and Shao, 2009), in turn affecting the conversion and sequestration, and potential ecological risk, of heavy metals in soil. Heavy metals emitted from automobile exhausts and those present in vehicle tires are also deposited in farmland, driven by climatic factors such as rainfall, further affecting the accumulation of Cd and Hg. In line with this, our results are similar to a previous study (Liu et al., 2016; Turkyilmaz et al., 2018), whereby distance from a road was considered one of the most important factors influencing the potential ecological risks of Cd and Hg in farmland soil. Moreover, industrial wastewater and domestic sewage discharge into rivers used for agricultural irrigation was also found to be another important reason for increasing concentrations and potential ecological risk levels of heavy metals in farmland soil. Furthermore, the occurrence of heavy metals varies across soil types and textures; thus, the potential ecological risk of Cd and Hg in farmland soil is also affected, to a certain extent, by soil texture. Explanatory power q of the selected factors varied little in terms of the potential ecological risk of Cd and Hg, suggesting that both heavy metals are jointly affected by multiple factors. Using Geodetector, the two most significant factors influencing the potential ecological risk of Cd were a pH of 5.2–5.6 and Distance from a road, while for Hg, distance from a railway >6004.02 m and total p ≤ 0.52 mg kg−1 were most significant. These results highlight the need for future management and control of heavy metal pollution in farmland soil in this and similar regions.
In this study, the concentrations of Cd and Hg in farmland soil in Fengcheng both showed moderate variation, influenced by both natural and human factors. Both human and natural factors have a comprehensive effect on the spatial distribution of the two heavy metals. Moreover, while concentrations of Cd and Hg in most areas exceeded the regional background level in Jiangxi, they did not reach the national risk screening value for soil pollution of agricultural land in China. The most significant factor influencing the potential ecological risk of Cd was a pH of 5.2–5.6. Meanwhile, the most significant factor affecting Hg was distance from a railway >6004.02 m, followed by total p ≤ 0.52 mg kg−1. The most significant factor affecting the comprehensive potential ecological risk index was soil pH of 5.2–5.6 followed by total p ≤ 0.52 mg kg−1. Overall, the potential ecological risk of heavy metals in the study region was low. These results provide insight into heavy metal pollution in Poyang Lake Plain, which will aid the prevention and control of farmland soil pollution as well as the utilization and protection of agricultural resources on a regional scale.
The raw data supporting the conclusion of this article will be made available by the authors, without undue reservation.
All authors listed have made a substantial, direct, and intellectual contribution to the work and approved it for publication. XH and HY contributed equally to this work.
This research was supported by the National Key R and D Program of China (No. 2017YFD0301603).
The authors declare that the research was conducted in the absence of any commercial or financial relationships that could be construed as a potential conflict of interest.
Aelion, C., Davis, H. T., McDermott, S., and Lawson, A. (2008). Metal concentrations in rural topsoil in South Carolina: potential for human health impact. Sci. Total Environ. 402, 149–156. doi:10.1016/j.scitotenv.2008.04.043
An, J., Gong, X., Chen, H., and Wei, S. (2016). Temporal and spatial characteristics and health risk assessments of heavy metal pollution in soils of Shenfu irrigation area. J. Agro-Environ. Sci. 35 (1), 37–44. doi:10.11654/jaes.2016.01.005
Arman, N., Mohammad, A., Babak, K., and Mohammad, S. (2017). Assessment of spatial distribution of soil heavy metals using ANN-GA, MSLR and satellite imagery. Environ. Monit. Assess. Int. J. 185 (5), 214
Bandara, J., Senevirathna, D., Dasanayake, D., Herath, V., Bandara, J., Abeysekara, T., et al. (2008). Chronic renal failure among farm families in cascade irrigation systems in Sri Lanka associated with elevated dietary cadmium levels in rice and freshwater fish (Tilapia). Environ. Geochem. Health 30 (5), 465–478. doi:10.1007/s10653-007-9129-6
Barlas, N., Akbulut, N., and Aydoğan, M. (2005). Assessment of heavy metal residues in the sediment and water samples of Uluabat Lake, Turkey. Bull. Environ. Contam. Toxicol. 74 (2), 286–293. doi:10.1007/s00128-004-0582-y
Boussen, S., Soubrand, M., Bril, H., Ouerfelli, K., and Abdeljaouad, S. (2013). Transfer of lead, zinc and cadmium from mine tailings to wheat (Triticum aestivum) in carbonated mediterranean (Northern Tunisia) soils. Geoderma 192, 227–236. doi:10.1016/j.geoderma.2012.08.029
Cai, L., Wang, Q., Wen, H., Luo, J., and Wang, S. (2019). Heavy metals in agricultural soils from a typical township in Guangdong province, China: occurrences and spatial distribution. Ecotoxicol. Environ. Saf. 168, 184–191. doi:10.1016/j.ecoenv.2018.10.092
Chabukdhara, M., and Nema, A. (2013). Heavy metals assessment in urban soil around industrial clusters in Ghaziabad, India: probabilistic health risk approach. Ecotoxicol. Environ. Saf. 87, 57–64. doi:10.1016/j.ecoenv.2012.08.032
Chen, F., and Dong, Y. (2005). Variation of soil heavy metal contents in a long-term fertilization experiment. Soil 37 (3), 308–311. doi:10.13758/j.cnki.tr.2005.03.015
Chen, M., Ding, S., Zhang, L., Li, Y., Sun, Q., and Zhang, C. (2017). An investigation of the effects of elevated phosphorus in water on the release of heavy metals in sediments at a high resolution. Sci. Total Environ. 575, 330–337. doi:10.1016/j.scitotenv.2016.10.063
China National Environmental Monitoring Centre (CNEMC) (1990). The background values of Chinese soils. Beijing: Environmental Science Press of China, 101–501.
Dai, L., Wang, L., Li, L., Liang, T., Zhang, Y., Ma, C., et al. (2018). Multivariate geostatistical analysis and source identification of heavy metals in the sediment of Poyang lake in China. Sci. Total Environ. 621, 1433–1444. doi:10.1016/j.scitotenv.2017.10.085
Ding, Q., Cheng, G., Wang, Y., and Zhuang, D. (2016). Effects of natural factors on the spatial distribution of heavy metals in soils surrounding mining regions. Sci. Total Environ. 578, 577–585. doi:10.1016/j.scitotenv.2016.11.001
Facchinelli, A., Sacchi, E., and Mallen, L. (2001). Multivariate statistical and gis-based approach to identify heavy metal sources in soils. Environ. Pollut. 114 (3), 313–324. doi:10.1016/S0269-7491(00)00243-8
Ha, H., Olson, J., Bian, L., and Rogerson, P. (2014). Analysis of heavy metal sources in soil using kriging interpolation on principal components. Environ. Sci. Technol. 48 (9), 4999–5007. doi:10.1021/es405083f
Hakanson, L. (1980). An ecological risk index for aquatic pollution control.a sedimentological approach. Water Res. 14, 975–1001. doi:10.1016/0043-1354(80)90143-8
He, J., and Xu, G. (2006). Study on background value of soil environment in Jiangxi province. Beijing, China: China Environmental Science Press, 314.
Hu, B., Jia, X., Hu, J., Xu, D., Xia, F., and Li, Y. (2017). Assessment of heavy metal pollution and health risks in the soil-plant-human system in the Yangtze river delta, China. Int. J. Environ. Res. Public Health 14 (14), 1–18. doi:10.3390/ijerph14091042
Hu, B., Zhao, R., Chen, S., Zhou, Y., Jin, B., Li, Y., et al. (2018). Heavy metal pollution delineation based on uncertainty in a coastal industrial city in the Yangtze river delta, China. Int. J. Environ. Res. Public Health 15 (4), 710. doi:10.3390/ijerph15040710
Ihedioha, J. N., Ukoha, P., and Ekere, N. (2016). Ecological and human health risk assessment of heavy metal contamination in soil of a municipal solid waste dump in uyo, nigeria. Environ. Geochem. Health 39 (3), 497–515. doi:10.1007/s10653-016-9830-4
Imperato, M., Adamo, P., Naimo, D., Arienzo, M., Stanzione, D., and Violante, P. (2003). Spatial distribution of heavy metals in urban soils of Naples city (Italy). Environ. Pollut. 124 (2), 247–256. doi:10.1016/S0269-7491(02)00478-5
Jiang, Y., Rao, L., Sun, K., Han, Y., and Guo, X. (2018). Spatio-temporal distribution of soil nitrogen in Poyang lake ecological economic zone (South-China). Sci. Total Environ. 626, 235–243. doi:10.1016/j.scitotenv.2018.01.087
Jiang, Y., and Guo, X. (2019). Multivariate and geostatistical analyses of heavy metal pollution from different sources among farmlands in the Poyang lake region, China. J. Soil. Sediment. 19, 2472–2484. doi:10.1007/s11368-018-2222-x
Kasel, S., Bennett, L. T., Aponte, C., Fedrigo, M., and Nitschke, C. R. (2017). Environmental heterogeneity promotes floristic turnover in temperate forests of south-eastern Australia more than dispersal limitation and disturbance. Landsc. Ecol. 32, 1613–1629. doi:10.1007/s10980-017-0526-7
Kelepertzis, E. (2014). Accumulation of heavy metals in agricultural soils of Mediterranean: insights from Argolida basin, Peloponnese, Greece. Geoderma 221–222 (27), 82–90. doi:10.1016/j.geoderma.2014.01.007
Lei, Z., Yang, S., Xu, Z., and Vachaud, G. (1985). Preliminary investigaion of the spatial variability of soil properties. J. Hydraulic Eng. (09), 10–21. doi:10.13243/j.cnki.slxb.1985.09.002
Liu, H. L., Zhou, J., Li, M., Hu, Y. M., Liu, X., and Zhou, J. (2019). Study of the bioavailability of heavy metals from atmospheric deposition on the soil-pakchoi (Brassica chinensis l.) system. J. Hazard. Mater. 362, 9–16. doi:10.1016/j.jhazmat.2018.09.032
Liu, Y., Ma, Z., Lv, J., and Bi, J. (2016). Identifying sources and hazardous risks of heavy metals in topsoils of rapidly urbanizing East China. J. Geogr. Sci. 26 (6), 735–749. doi:10.1007/s11442-016-1296-x
Loredo, J., Pereira, A., and Ordonez, A. (2003). Untreated abandoned mercury mining works in a scenic area of asturias (Spain). Environ. Int. 29 (4), 481–491. doi:10.1016/S0160-4120(03)00007-2
Lü, J., Jiao, W.-B., Qiu, H.-Y., Chen, B., Huang, X.-X., and Kang, B. (2018). Origin and spatial distribution of heavy metals and carcinogenic risk assessment in mining areas at You'xi county Southeast China. Geoderma 310, 99–106. doi:10.1016/j.geoderma.2017.09.016
Lv, J., Liu, Y., Zhang, Z., Dai, J., Dai, B., and Zhu, Y. (2015). Identifying the origins and spatial distributions of heavy metals in soils of Ju country (Eastern China) using multivariate and geostatistical approach. J. Soil. Sediment. 15 (1), 163–178. doi:10.1007/s11368-014-0937-x
Lv, J., Zhang, Z., Li, S., Liu, Y., Sun, Y., and Dai, B. (2013). Assessing spatial distribution, sources, and potential ecological risk of heavy metals in surface sediments of the Nansi lake, Eastern China. J. Radioanal. Nucl. Chem. 299 (3), 1671–1681. doi:10.1007/s10967-013-2883-2
Mazej, Z., Al Sayegh-Petkovsek, S., and Pokorny, B. (2010). Heavy metal concentrations in food chain of lake velenjsko jezero, slovenia: an artificial lake from mining. Arch. Environ. Contam. Toxicol. 58 (4), 998–1007. doi:10.1007/s00244-009-9417-5
National Standard of the People's Republic of China (2018). Soil environmental quality. Risk control standard for soil contamination agricultural land (trial)GB15618-2018, 2–3.
Qiao, P., Yang, S., Lei, M., Chen, T., and Dong, N. (2019). Quantitative analysis of the factors influencing spatial distribution of soil heavy metals based on geographical detector. Sci. Total Environ. 664, 392–413. doi:10.1016/j.scitotenv.2019.01.310
Raj, S. M., and Jayaprakash, M. (2008). Distribution and enrichment of trace metals in marine sediments of bay of bengal, off ennore, south-east coast of india. Environ. Geol. 56 (1), 207–217. doi:10.1007/s00254-007-1156-1
Shi, R., Zhang, Y., Xu, M., Zhang, X., and Zhao, Z. (2019). Pollution evaluation and source apportionment of heavy metals in soils from tianjin suburbs, China. J. Agro-Environ. Sci. 38 (5), 1069–1078. doi:10.11654/jaes.2018-1152
Sriramachari, S., and Jain, A. K. (1997). Trace elements in human nutrition and health. Indian J. Med. Res. 105 (5), 246–247. doi:10.1177/026010609601100206
Tang, L. (2007). Effects of soil properties on crop cd uptake and prediction of cd concentration in grains. J. Agro-Environ. Sci. 26 (2), 699–703.
Tepanosyan, G., Maghakyan, N., Sahakyan, L., and Saghatelyan, A. (2017). Heavy metals pollution levels and children health risk assessment of yerevan kindergartens soils. Ecotoxicol. Environ. Saf. 142, 257–265. doi:10.1016/j.ecoenv.2017.04.013
Turkyilmaz, A., Cetin, M., Sevik, H., Isinkaralar, K., and Saleh, E. A. A. (2018). Variation of heavy metal accumulation in certain landscaping plants due to traffic density. Environ. Dev. Sustain. 22 (3), 2385. doi:10.1007/s10668-018-0296-7
Wang, J., and Xu, C. (2017). Geodetector: principle and prospective. Acta Geogr. Sin. 72 (01), 116–134. doi:10.11821/dlxb201701010
Wang, S., Cai, L. M., Wen, H. H., Luo, J., Wang, Q. S., and Liu, X. (2019). Spatial distribution and source apportionment of heavy metals in soil from a typical county-level city of guangdong province, China. Sci. Total Environ. 655, 92–101. doi:10.1016/j.scitotenv.2018.11.244
Wang, Y., Bai, Y., and Wang, J. (2014). Distribution of soil heavy metal and pollution evaluation on the different sampling scales in farmland on Yellow River irrigation area of Ningxia: a case study in Xingqing County of Yinchuan City. Huan Jing Ke Xue 35 (7), 2714. doi:10.13227/j.hjkx.2014.07.039
Wei, X., and Shao, M. (2009). Distribution of micronutrients in soils as affected by landforms in a Loessial Gully Watershed. Environ. Sci. Tech. 30 (9), 2741–2746. doi:10.13227/j.hjkx.2009.09.009
Xie, X. J., Kang, J. C., Yan, G. D., Zhang, J. P., and Zhu, W. W. (2010). Heavy metal concentration in agricultural soils around the upper-middle reaches of Huangpu river. China Environ Sci. 30 (8), 1110–1117. doi:10.3724/SP.J.1088.2010.00432
Xu, Z. Q., Ni, S. J., Tuo, X. G., and Zhang, C. J. (2008). Calculation of heavy metals' toxicity coefficient in the evaluation of potential ecological risk index. Environ. Sci. Tech. 31 (2), 112–115. doi:10.19672/j.cnki.1003-6504.2008.02.030
Yang, A., Wang, Y. H., Hu, J., Liu, X. L., and Li, J. (2020). Evaluation and source of heavy metal pollution in surface soil of Qinghai-Tibet plateau. Huan Jing Ke Xue 41 (2), 886–894. doi:10.13227/j.hjkx.201907195
Yuan, R., Yu, G., Qiu, X., Zong, L., and Zheng, L. (2015). Regionally spatial variation of soil heavy metals and their influences on vegetable quality: a case study of Baguazhou and Jiangxinzhou, Nanjing, China. J. Agro-Environment Sci. 34 (8), 1498–1507. doi:10.11654/jaes.2015.08.010
Zhang, H., Jiang, Y., Ding, M., and Xie, Z. (2017). Level, source identification, and risk analysis of heavy metal in surface sediments from river-lake ecosystems in the Poyang lake, China. Environ. Sci. Pollut. Res. Int. 24, 21902–21916. doi:10.1007/s11356-017-9855-y
Zhang, P., Qin, C., Hong, X., Kang, G., Qin, M., Yang, D., et al. (2018). Risk assessment and source analysis of soil heavy metal pollution from lower reaches of yellow river irrigation in China. Sci. Total Environ. 633, 1136–1147. doi:10.1016/j.scitotenv.2018.03.228
Zhao, Q., and Luo, Y. (2015). The macro strategy of soil protection in China. Bull. Chin. Acad. Sci. 30 (4), 452–458. doi:10.16418/j.issn.1000-3045.2015.04.003
Zhong, X. L., Zhou, S. L., Li, J. T., and Zhao, Q. G. (2007). Spatial variability of soil heavy metals contamination in the Yangtze river delta--a case study of Taicang city in Jiangsu Province. Acta. Pedol. Sin. 44 (1), 33–40. doi:10.11766/trxb200508260106
Zhou, J., Zhang, C., Du, B., Cui, H., Fan, X., Zhou, D., et al. (2020). Effects of zinc application on cadmium (cd) accumulation and plant growth through modulation of the antioxidant system and translocation of cd in low- and high-cd wheat cultivars. Environ. Pollut. 265 (Pt A), 115045. doi:10.1016/j.envpol.2020.115045
Keywords: farmland, soil heavy metal, spatial variation, potential risk, impacting factors
Citation: Huang X, Yu H, Zhao X, Guo X, Ye Y and Xu Z (2021) Spatial Variation in Cadmium and Mercury and Factors Influencing Their Potential Ecological Risks in Farmland Soil in Poyang Lake Plain, China. Front. Environ. Sci. 9:641497. doi: 10.3389/fenvs.2021.641497
Received: 14 December 2020; Accepted: 18 February 2021;
Published: 29 March 2021.
Edited by:
Jun Zhou, University of Massachusetts Lowell, United StatesReviewed by:
Xuchao Zhu, Institute of Soil Science (CAS), ChinaCopyright © 2021 Huang, Yu, Zhao, Guo, Ye and Xu. This is an open-access article distributed under the terms of the Creative Commons Attribution License (CC BY). The use, distribution or reproduction in other forums is permitted, provided the original author(s) and the copyright owner(s) are credited and that the original publication in this journal is cited, in accordance with accepted academic practice. No use, distribution or reproduction is permitted which does not comply with these terms.
*Correspondence: Xiaomin Zhao, emhhb3htODg5QDEyNi5jb20=
Disclaimer: All claims expressed in this article are solely those of the authors and do not necessarily represent those of their affiliated organizations, or those of the publisher, the editors and the reviewers. Any product that may be evaluated in this article or claim that may be made by its manufacturer is not guaranteed or endorsed by the publisher.
Research integrity at Frontiers
Learn more about the work of our research integrity team to safeguard the quality of each article we publish.