- 1State Key Laboratory of Freshwater Ecology and Biotechnology, Institute of Hydrobiology, The Chinese Academy of Sciences, Wuhan, China
- 2College of Life Sciences, University of Chinese Academy of Sciences, Beijing, China
- 3Department of Bioscience, Aarhus University, Silkeborg, Denmark
- 4Sino-Danish Centre for Education and Research, University of Chinese Academy of Sciences, Beijing, China
- 5College of Water Conservancy and Hydropower Engineering, Hohai University, Nanjing, China
- 6Limnology Laboratory, Department of Biological Sciences and Centre for Ecosystem Research and Implementation, Middle East Technical University, Ankara, Turkey
- 7Institute of Marine Sciences, Middle East Technical University, Mersin, Turkey
The safety of drinking water is constantly being evaluated. In the last few decades, however, many drinking waters sources in the world, including in China, have undergone serious eutrophication and consequently water quality deterioration due to anthropogenic induced stressors such as elevated external nutrient inputs. In this study, we used the state-of-the-art complex, dynamic, mechanistic model GOTM-FABM-PCLake (a coupled one-dimensional hydrodynamic-lake ecosystem model) to quantitatively assess the impacts of external nutrient loading on the temperate Jihongtan reservoir in Shandong Province, China. Simulated values of all variables targeted in calibration (water temperature, dissolved oxygen, total nitrogen, total phosphorus, and chlorophyll a) agreed well with observations throughout the entire calibration and validation period and generally mimicked seasonal dynamics and inter-annual variations as found in the monitoring data. A series of scenarios, representing changed external nutrient loadings (both increasing and decreasing compared to the current nutrient load), were set up to quantify the effects on the reservoir water quality. Changes relative to the current external nutrient load had a significant effect on the simulated TN and TP concentrations in the reservoir. Our impact assessment indicate that TN will meet the Chinese water quality requirements of the water source (Class III) when the external nitrogen load is reduced by 70%, whereas TP will meet the requirements even if the external phosphorus load is increased by 100% relative to current loads. The model predicts progressively higher summer and autumn phytoplankton biomasses in the scenarios with increasing external phosphorus loading and potential toxic cyanobacteria will become more dominant at the expense of diatoms and other algae. Strict control of the external nutrient loading is therefore needed to maintain good drinking water quality in the reservoir.
Highlights
The state-of-the-art complex, dynamic, mechanistic model was used.
Phosphorus (P) is the key limiting factor in Jihongtan Reservoir.
Cyanobacteria are the dominant species and sensitive to changes in P.
Cyanobacteria blooms are the key water quality problem.
Introduction
In the past 200 years, many lakes have experienced increasing eutrophication due to anthropogenic increases in the external input of nutrients (point and non-point sources) (Wetzel, 1990; Wetzel, 1992). As a result of excess nutrient inputs [i.e., nitrogen (N) and phosphorus (P)], many lakes have undergone severe eutrophication and water quality deterioration, characterized by massive summer blooms of phytoplankton (especially cyanobacteria), low water transparency, reduced coverage and abundance of submerged macrophytes, dominance of plankti-benthivorous fish and a loss of biodiversity (Moss, 1998; WWF, 2018).
During the past few decades, great efforts and vast amounts of financial resources have been used globally to improve the ecological state of lakes by reducing the external nutrient loadings (Sas, 1989; Jeppesen et al., 2007). However, combating eutrophication remains a global challenge (Schindler, 2006; Carpenter, 2008; Conley et al., 2009; Wurtsbaugh et al., 2019). Multiple studies indicate that primary production is frequently limited by the supplies of N, P or both depending on their relative abundances (Elser et al., 2007). Half a century ago, phosphorus was recognized as the critical nutrient for primary production in aquatic ecosystems (Schindler, 1977), but recently focus has also been directed at the role of nitrogen in algae blooms (Trolle et al., 2008; Ma et al., 2018). Some existing paradigms regard P as the most important limiting nutrient in lakes (Schindler, 1977) while N is considered as the primary limiting nutrient in marine and terrestrial ecosystems (Vitousek and Howarth, 1991; Howarth and Marino, 2006). Nevertheless, strategies toward external nutrient control of P alone have so far had limited effect in several lakes, such as lakes Taihu and Donghu in China, lakes Apopka, George and Okeechobee in United States, and lake Kasumigaura in Japan (Havens et al., 2001; Conley et al., 2009). Recently, focus has also been on the role of nitrogen and some research indicate that controlling both N and P loads would be a more efficient management strategy for mitigation eutrophication in lakes (Lewis and Wurtsbaugh, 2008; Lewis et al., 2011; Özkundakci et al., 2011; Paerl et al., 2016; Wurtsbaugh et al., 2019). While, discussions of the roles of N and P continues, the urgent need to reduce external nutrient loadings to surface waters to protect drinking water resources and to reduce eutrophication, is widely recognized (Conley et al., 2009).
For drinking water sources, harmful algal blooms are considered one of the most serious threats associated to eutrophication (Paerl et al., 2019), promoting growth of potential toxin-producing algae such as cyanobacteria and in some cases dinoflagellates in lakes and reservoirs (Lewis et al., 2011). Harmful algal blooms produce taste, odor and toxins, potentially making waters unusable for drinking as it may threaten health (Koreiviene et al., 2014; Wurtsbaugh et al., 2019). Some of the harmful algal toxins are known to be more toxic than cobra venom (Cheung et al., 2013) and can thus harm humans, livestock, birds, and many other animal species, which may be in contact with the water (Stewart et al., 2008). Bioaccumulation of harmful algal toxins in fish and shellfish can also cause poisoning of their consumers (Carmichael and Boyer, 2016; Grattan et al., 2016). Currently, however, scientists are not able to predict when algal blooms are harmful since not all species in a particular bloom produce toxin (Wurtsbaugh et al., 2019). Hence, the only way to prevent water quality deterioration is controlling the occurrence of harmful algal blooms by reducing the external nutrient loading.
Jihongtan reservoir is a key water supply for Qingdao and the surrounding region with a population of 9.3 million inhabitants. In recent years, the external nutrient load has increased due to the pollution in the inflowing rivers. The reservoir TN:TP (mass ratio) ratio ranges from 28 to 830 (2000–2013). At present, eutrophication of the reservoir and production of microcystin toxins are the main water environment problems managers are facing. We used the state-of-the-art complex, dynamic lake ecosystem model FABM-PCLake (Hu et al., 2016) coupled with the one-dimensional, hydrodynamic General Ocean Turbulence Model (GOTM) (Burchard and Bolding, 2001) to quantitatively elucidate the impacts of changed external nutrient loadings on water quality attributes in the Jihongtan temperate drinking water reservoir in China. Our hypotheses were that 1) phosphorus concentration is the main limiting factor for Jihongtan reservoir as the TN:TP ratio is generally high, and 2) cyanobacteria blooms are the root cause of the water quality crisis in Jihongtan reservoir.
Materials and Methods
Study Area
Jihongtan reservoir is the largest man-made dam-type reservoir (the reservoir is like a pool, with all boundary formed by concrete wall) located in the northwestern part of the town Jihongtan, North of Qingdao, China (120.22°E, 36.36°N) (Figure 1). The dam is octagonal with a design water level of 14.2 m at the dam, a length of 14.2 km, a surface area of 14.1 km2, a volume of approx. 1.57 × 108 m3, a hydraulic retention time of approx. 1.4 years, a minimum water level of 6.5 m, a minimum storage capacity of 3.55 × 107 m3, and a water supply of 3.0 × 105 m3/day. The reservoir is located downstream of the Yellow River, which is its sole source of inflow through a pumping station. The reservoir began operation on 25 November, 1989. The reservoir has no natural watershed since its elevated above the surrounding environment. The reservoir bottom area is flat and the soil is mainly clay with a low permeability. The region is characterized by both a monsoon and east pacific temperate coast climate. The annual mean temperature is 12.7°C, while annual mean precipitation is 662 mm (1998–2010), with pronounced rain in summer (May to July), accounting for 57% of the annual precipitation. According to the OECD (1982) classification, the reservoir trophic state varies from mesotrophic to eutrophic.
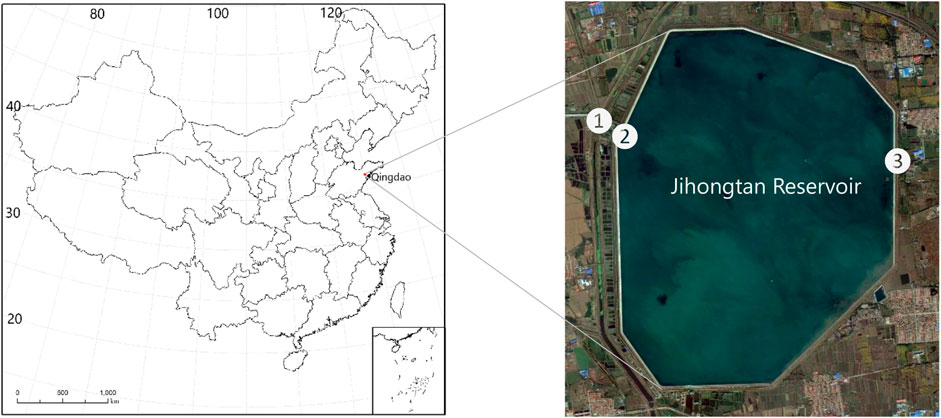
FIGURE 1. A map of the study area where ① represent the inlet pump station, ② and ③ are inflow and outflow observation locations, respectively. The map of China shows the location of the reservoir in the northwestern part of Qingdao City (red frame).
Model Description
The 1-dimensional, hydrodynamic-lake ecosystem model complex GOTM-FABM-PCLake (Bruggeman and Bolding, 2014; Hu et al., 2016) was used to simulate the time- and depth-varying distribution of water parameters in the Jihongtan reservoir. The model complex was run and the model simulations visualized through the graphical user interface QWET (formerly known as WET, Nielsen et al., 2017) version 1.3.1. Here, GOTM, the General Ocean Turbulence Model (Burchard and Bolding, 2001), is a library of traditional and state-of-the-art turbulence closure models used for the parameterization of vertical turbulent fluxes of momentum, heat, and dissolved and particulate matter (Umlauf and Burchard, 2005). FABM, the Framework for Aquatic Biogeochemical Models (Bruggeman and Bolding, 2014), is the coupling layer between the hydrodynamic model and the biogeochemical model. FABM-PCLake (Hu et al., 2016), which is a re-design of the 0-dimensional PCLake model (Janse and Liere, 1995), includes nine modules (abiotic_water, abiotic_sediment, phytoplankton_water, phytoplankton_sediment, macrophytes, zooplankton, fish, zoobenthos, and an auxiliary module). The model complex, GOTM-FABM-PCLake, has previously been applied to simulate long-term water quality dynamics in a deep and a shallow temperate lake (Chen et al., 2019; Andersen et al., 2020). The main reasons for choosing GOTM-FABM-PCLake were that the model 1) provides a detailed description of ecosystem processes that can be used to study complex aquatic ecosystems and 2) gives a detailed prediction of hydrodynamic and ecosystem components interactions.
Model Input
Boundary and forcing conditions for the model application to Jihongtan reservoir was confined to a 15-year (2000–2014) simulation period given the availability of data. Inputs consisted of a hypsograph, daily meteorological data, inflow and outflow of water, and nutrient concentrations in the inflow. The hypsographic configuration of the reservoir shape was supplied by Qingdao Hydrographic Bureau. The reservoir was divided into 11 vertical layers, the areas between layers and at the sediment-water interface were derived from a lake-specific hypsography (i.e. the relation between depth and horizontal area) with a maximum depth of 11.2 m. Meteorological forcing was represented by a three-hourly dataset of wind speed (eastward and northward component of wind at 10 m height, m/s), air pressure (hPa), air temperature (°C), relative humidity (%) and cloud cover (%), obtained from the Qingdao Meteorological Administration. Monthly average water inflow (m3/s), water outflow (m3/s), and inflow nutrient concentrations (mg/L) were used as boundary conditions based on monthly measurements of inflow, total nitrogen (TN) and phosphorous (TP) concentrations from Jihongtan Reservoir Authority (Figure 2). Input on nutrient concentrations to the model was divided into particulate organic phosphorus (PPOM, mg/L), orthophosphate (PO4, mg/L), particulate organic nitrogen (NPOM, mg/L), nitrate (NO3, mg/L), and ammonium (NH4, mg/L). The particulate organic fractions were derived from the mass balance calculations between total and inorganic nutrient concentrations.
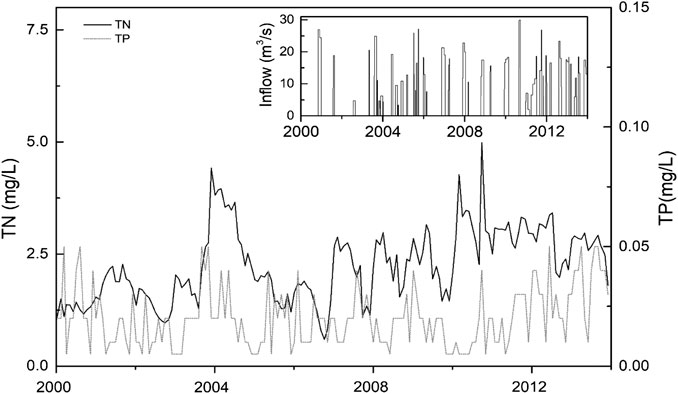
FIGURE 2. Total nitrogen (TN) and total phosphorus (TP) concentrations in the inflowing water from 2000 to 2013.
Model Calibration and Validation
Modeling were split into warm-up (2000–2003), calibration (2004–2011), and validation (2012–2013), respectively. The warm-up period was used to reduce the uncertainty associated with model initialization related to model calibration (Chen et al., 2019). Model performance was optimized against measured values of water temperature (TEMPV), dissolved oxygen (DO), total nitrogen (TN), total phosphorus (TP), and chlorophyll a (Chl-a).
The model was calibrated using the auto-calibration tool ACPy (Auto Calibration Python) developed by Bolding and Bruggemann (http://bolding-bruggeman.com/portfolio/acpy/) to perform automatic optimization of a selected subset of model parameters via value adjustments within predefined and realistic ranges in order to minimize the difference between simulated and measured values (Moras et al., 2019). ACPy utilizes the differential evolution search method and a likelihood multi-objective function to narrow and optimize parameter-specific ranges.
Predefined parameter values, based on the observed water quality in more than 40 lakes (Janse, 2005), were used initially in the calibration. Parameters subject to calibration were identified and selected from the previous sensitivity analysis described by Janse et al. (2010); Schladow and Hamilton (1997), and Nielsen et al. (2014) as well as user experience during the actual calibration process. The auto-calibration tool allowed for a faster calibration process than previous manual calibrations (Chen et al., 2019; Andersen et al., 2020), resulting in a total of 124 parameters calibrated for the Jihongtan reservoir model. All calibration parameters have been provided in the Supplementary Table S1.
Calibration was conducted successive in a five-step procedure targeting in order: physical dynamics -> oxygen dynamics -> nitrogen dynamics -> phosphorus dynamics -> phytoplankton dynamics (and higher trophic levels). During each calibration step, thousands of model runs were executed via ACPy, ultimately producing an improved parameter value range of the selected parameters to increase model performance calibrations (Andersen et al., 2020). The improved parameter ranges were then applied in the next step of the calibration procedure. After each step, the RMSE (root mean square error) and R (correlation coefficient) were computed to evaluate performance for each output variable (TEMP, DO, TN, TP, and Chl-a) (Table 1). The stepwise calibration continued until model error could no longer be appreciably minimized.
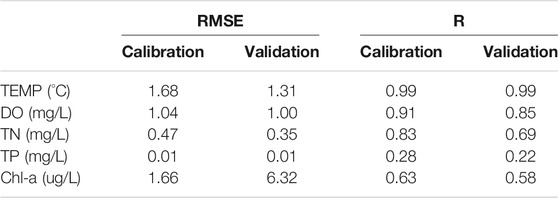
TABLE 1. Root-mean-square-error (RMSE) values and Pearson coefficients based on selected model outputs and observed data.
Scenarios
In order to predict the potential effects of a change in external nutrient loadings on water quality, a total of 60 nutrient loading scenarios were designed. Model input data (meteorological forcing, inflow and outflow of water, and inflow nutrient concentrations) from the three-year period 2007–2009 were looped until 2012, an adequate duration to be able achieve a stable state within the reservoir, because the external load is relatively stable in 2007–2009. The last three years of the scenario period were extracted for further analysis. To create different scenarios, the looped periods were subject to changes either as: 1) changes in external nitrogen loading within a range of −90 to +100% in increments of 10%, 2) changes in external phosphorus loading within a range of −90 to +100% in increments of 10%, and 3) changes in both external nitrogen and phosphorus loading within a range of −90 to 100% in increments of 10%. The environmental quality standards for surface water (GB 3838, 2002) and standards for drinking water quality (GB 5749, 2006) were applied to determine the needed nutrient load changes for acceptable simulated water quality.
Results
Calibration and Validation
Overall, all water quality parameters (TEMP, DO, TN, TP, and Chl.-a) exhibited relatively good agreement between modeled and observed data for the entire calibration (2004–2011) and validation (2012–2013) periods.
Modeled water temperatures showed excellent agreement with observed data and temperatures were reproduced accurately, with a high R and small RMSE during the calibration (R = 0.99, RMSE = 1.68) and validation (R = 0.99, RMSE = 1.31) periods. Simulated temperatures across winter and summer spanned a temperature range of approx. 0–30°C and captured both timing and inter-annual variations (Table l; Figure 3).
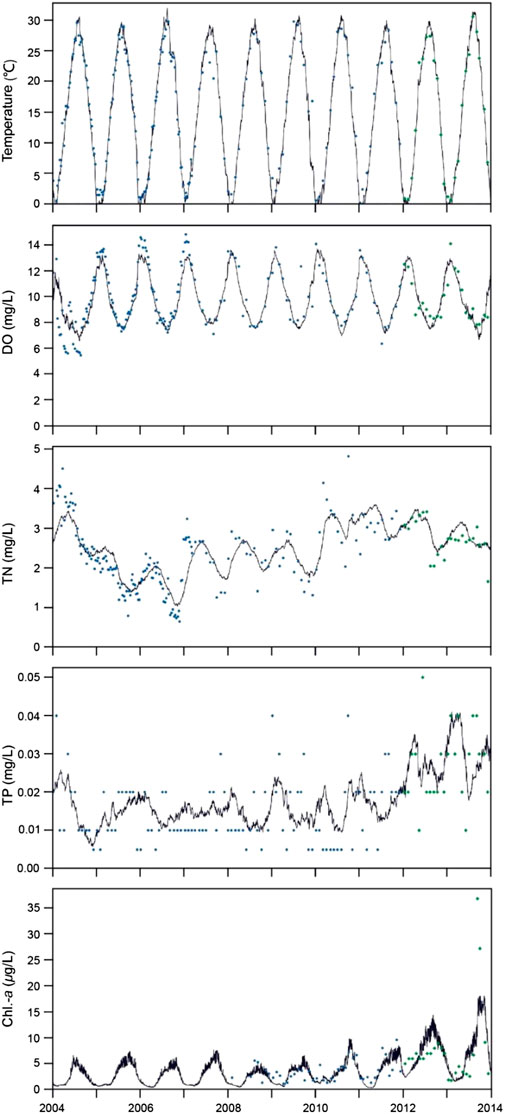
FIGURE 3. Simulated (black line) and observed values (blue and green dots, respectively) for the calibration (2004–2011) and validation (2012–2013) periods.
Comparison of simulated and observed data for DO yielded R and RMSE values of 0.91 and 0.85 and 1.04 and 1.00, respectively, indicating a good correspondence between model and field observations during both the calibration and validation period. Simulated DO generally increased in winter to around 12–14 mg/L and decreased in summer to around 6–8 mg/L. The model captured reasonably well the seasonal dynamics and inter-annual variations of DO with low RMSE, but for some years the model simulations tended to underestimate DO in winter (Table l; Figure 3).
The dynamics of in-reservoir TN were largely driven by inflow nitrate, which contributed 50–80% of TN. Generally, the TN dynamics showed good agreement with measurements from both the calibration and validation period (Table l; Figure 3), and the modeled TN also exhibited the same seasonal dynamics and inter-annual variations as the monitoring data. However, the model underestimated nitrogen in summer and overestimated it in winter by approx. 25% over the whole simulated period.
The model succeeded in capturing the seasonal patterns and inter-annual variations of TP despite the highly variable observations (Table 1; Figure 3). For both the calibration and the validation period, the performance statistics were poor most likely due to imprecize observed field concentrations that were sometimes near the detection limit (0.003 mg/L) and thus yielded low R but also low RMSE values. Over the whole simulated period, the model overestimated TP in summer and underestimated it in winter.
The GOTM-FABM-PCLake model set up in included three phytoplankton groups: cyanobacteria, diatoms, and green algae (other algae). As the optimal growth temperature of algae differ, diatoms are the dominant class in spring and cyanobacteria in summer (Chen et al., 2019). According to our calibration, the optimal growth temperature of diatoms and cyanobacteria in Jihongtan reservoir were 18.7 and 26.3°C, respectively. For Chl.-a, the model captured the observed dynamics during both the calibration and the validation period (Table 1). Simultaneously, the model successfully reproduced the seasonal dynamics and changes with time of Chl.-a (Figure 3). In the last years of the validation period (e.g., 2013), however, the model tended to underestimate Chl.-a concentrations somewhat in summer and autumn.
Simulating the Influence of Changes in External Nutrient Loading
In the Jihongtan reservoir ecosystem, total nitrogen (TN) mainly originates from inflowing streams (from Yellow River through a pumping station). Nitrate accounts for 50–80% of the in-reservoir TN. The TN content showed significant seasonal dynamics and inter-annual variations, peaking in late spring and early summer and being lowest in late summer and early autumn.
In the baseline model simulation (representing the current nutrient loading level), TN far exceeded the water quality requirements of the water source (Class III) using the environmental quality standards for surface water (Supplementary Table S2; Figure 4) (GB 3838–2002; GB 5749–2006). In terms of annual averages, the TN content in the reservoir will meet the standard for surface water when the external nitrogen loading is reduced by 70%. Seasonal changes in the external nitrogen loads have great impact on the TN concentration in the reservoir (Figure 4). In spring, summer, autumn, and winter, the TN content of the reservoir will meet the water quality requirements of the water source (Class III) in accordance with the environmental quality standards for surface water when the external nitrogen load is reduced by 80, 70, 60, and 70%, respectively (Figure 4).
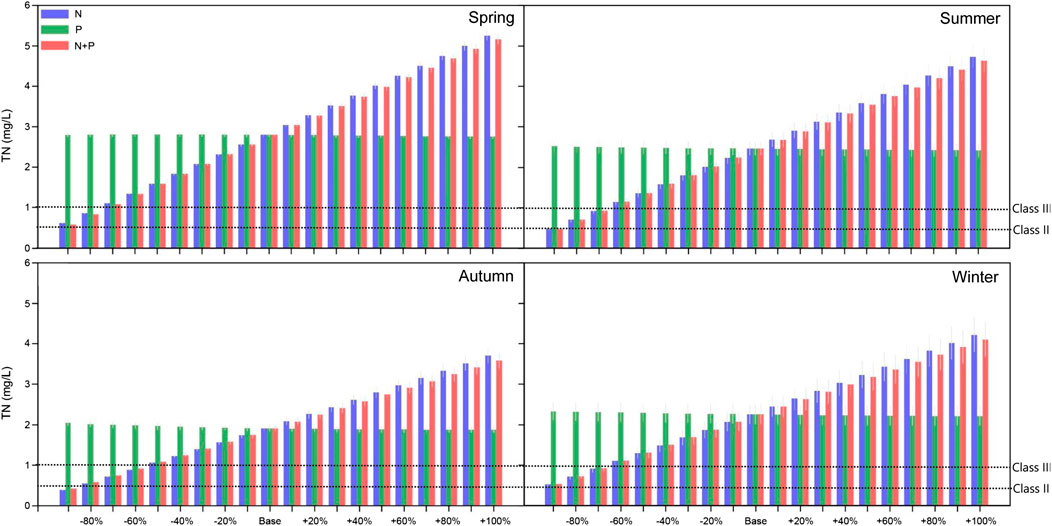
FIGURE 4. The effects of changed external nutrient loading on different seasonal mean values of TN in the Jihongtan reservoir. Errorbars represent standard deviation between years of the simulation.
As for nitrogen, the phosphorus loading to the Jihongtan reservoir mainly comes from inflowing streams (from Yellow River through a pumping station). The content of TP also showed clear seasonal dynamics and inter-annual changes. The peak and minimum value appeared in winter and summer, respectively. In the baseline model simulation, the TP content in the Jihongtan reservoir was below 0.025 mg/L throughout the year, which fulfills the water quality requirements for Class II in the environmental quality standards for surface water as well as the water quality requirements of the water source (Supplementary Table S2; Figure 5). The simulation results show that there are significant effects of the external phosphorus loading on the TP concentration in the reservoir in the different seasons (Figure 5). Nonetheless, the results of simulations from 60 different scenarios showed that even if the external phosphorus loading is increased by 100% relative to the current value, the phosphorus content of the reservoir will still meet the water quality requirement for the water source in the environmental quality standards for surface water (Class III; for water sources, the phosphorus content should be less than 0.05 mg/L, Supplementary Table S2).
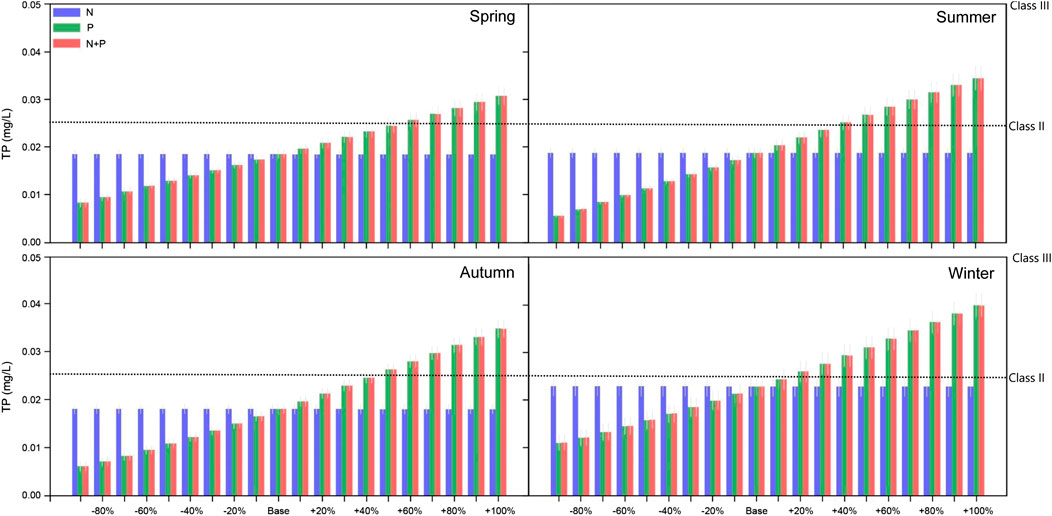
FIGURE 5. The effects of changed external nutrient loading on different seasonal mean values of TP in the Jihongtan reservoir. Errorbars represent standard deviation between years of the simulation.
The water nutrition status for oligotrophic, mesotrophic, eutrophic and ultra-eutrophic state, respectively, may be classified in terms of the Chl.-a concentration of the water body being <2.5 μg/L, 2.5∼8.0 μg/L, 8.0∼25.0 μg/L, and 25∼75 μg/L, according to the classification criteria for water nutrition status from OECD (1982). The Chl.-a concentration of Jihongtan reservoir exhibits marked seasonal dynamics and inter-annual changes with maximum values in summer and autumn and minimum values in winter. The annual average Chl.-a concentrations of the simulated scenarios indicate an oligotrophic state when the external phosphorus loading is reduced by 40% and according to the Chl.-a concentration the reservoir will remain in a mesotrophic state even if the external phosphorus loading is increased by 100%. However, there are significant difference in the influence of the external phosphorus loading on Chl.-a concentrations in the reservoir over the season (Figure 6). In spring and autumn, the reservoir Chl.-a concentration is indicative of an oligotrophic state when the external phosphorus loading is decreased by 40 and 60%, respectively. In summer, an 80% reduction of the external phosphorus loading is required before the reservoir Chl.-a concentration reach oligotrophic status, and increased by 20% the eutrophic status is reached. In winter, the reservoir Chl.-a concentration is constantly in oligotrophic status in all external nutrient loading change scenarios (from −90 to +100%) (Figure 6). It is worth noting that model predictions for phytoplankton biomass included cyanophytes, green algae, and diatoms. In summer and autumn, cyanobacteria are predicted to be the dominant group in the reservoir (constituting 90% of the phytoplankton biomass), and cyanobacteria Chl.-a is the main source of Chl.-a in water (Figure 7; Supplementary Figures S1–S6).
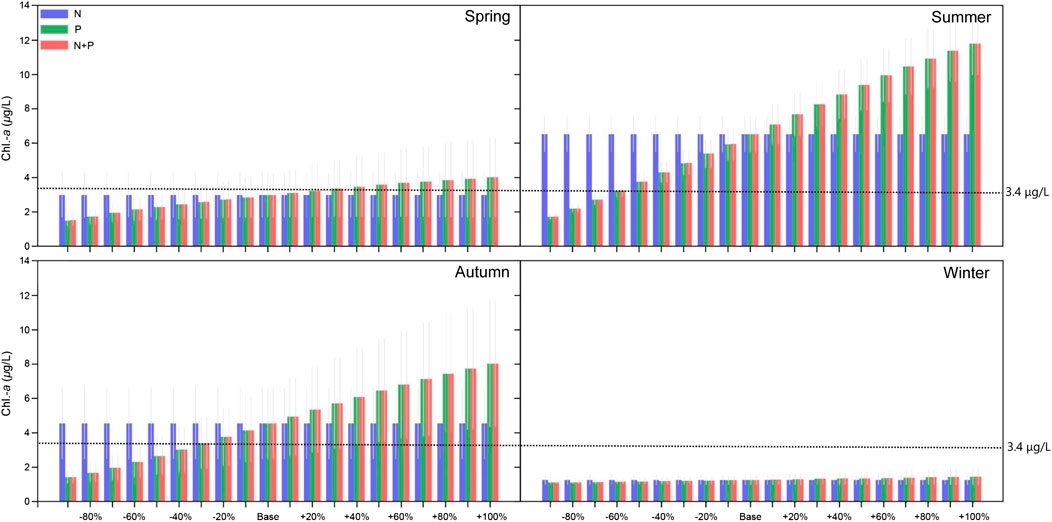
FIGURE 6. The effects of changed external nutrient loading on different seasonal mean values of Chl.-a in the Jihongtan reservoir. Errorbars represent standard deviation between years of the simulation.
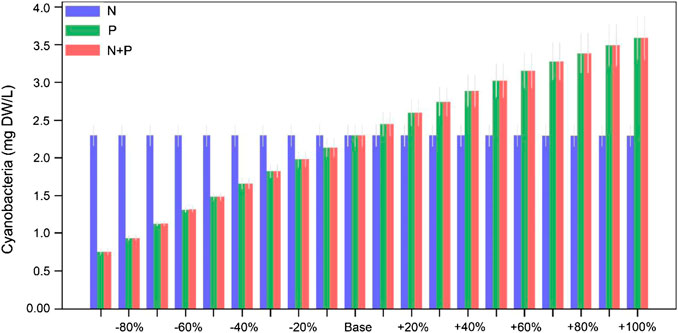
FIGURE 7. The effects of changed external nutrient loading on the summer dry weight of cyanobacteria biomass in the Jihongtan reservoir. Errorbars represent standard deviation between years of the simulation.
Discussion
Model Performance
Our study applied the coupled hydrodynamic-ecosystem model GOTM-FABM-PCLake (Hu et al., 2016) and the ACPy automatic calibration tool to a temperate reservoir ecosystem combined with a likelihood function as the objective function. Overall, with a few exceptions, the model was able to reproduce the measured data during the calibration and validation periods, which indicates that the model tracked well the seasonal dynamics and inter-annual variations. Compared with the R values of relevant water quality variables in 153 individual aquatic ecological modeling studies reviewed by Arhonditsis and Brett (2004), the present study generally performs better than 40–70% of previous studies. Therefore, we conclude that the performance of the GOTM-FABM-PCLake model complex for Jihongtan reservoir was satisfactory and can be applied in predicting and evaluating the effects of changes in the external nutrient loading on the water quality of the reservoir.
Simulating Effects of External Nutrients Loading
Although a reduction of the external nutrient loading is the key to mitigating eutrophication, quantification of the required reduction for specific lakes/reservoirs is critical (Elser et al., 2007; Cui et al., 2016). According to the Chinese Standard GB3838–2002, TN and TP of the water source should be lower than 1.0 mg/L and 0.05 mg/L (Class III; Supplementary Table S2), respectively. The model simulations showed that TN in the Jihongtan reservoir will meet the water quality requirements of the water source (Class III) when the external nitrogen loading is reduced by 70%, while TP concentrations in the reservoir will still meet the requirement (Class III) even if the external phosphorus loading is increased by 100%. The change in the external phosphorus loading had no clear effect on the TN of the reservoir; similarly, the change in the external nitrogen loading has no marked effect on the TP. Research has shown that TN:TP ratios (by mass) > 14:1 in the water indicates excessive N where P is the limiting factor for algae growth. Conversely, when TN:TP < 14:1, it is indicative of phytoplankton likely being limited in growth by available P (Downing and McCauley, 1992). We found that TN:TP was much greater than 14 in several scenarios in the Jihongtan reservoir ranging from roughly 100 to 200 during the summer for −90 to +100% NP load change (Supplementary Figures S7–S9). This is consistent with our first hypothesis. Eutrophication control in the Jihongtan reservoir should therefore focus on reducing the P load, but to meet the N standard for drinking water N load reduction is also required.
In lakes and reservoirs, a key symptom of eutrophication is algae blooms (Conley et al., 2009). Excess P in the water column leads to blooms of toxin-producing cyanobacteria that create taste and odor problems and threaten the safety of drinking water (Wurtsbaugh et al., 2019). According to the model simulations, cyanobacteria were the main component of the phytoplankton community in the reservoir (Figure 7), and cyanobacteria Chl.-a content accounted for more than 90% of the total Chl.-a content of the phytoplankton (Supplementary Figures S1–S6), corresponding to our second hypothesis. The model also predicts progressively more severe summer and autumn blooms in the scenarios of an external P loading increase (Supplementary Figures S10–S12). A likely explanation of this could be that summer and autumn not only have nutrient (including macronutrients and micronutrients) conditions suitable for algae growth, but also favorable light and temperature conditions (Otten and Paerl, 2015; Glibert and Burford, 2017). The summer and autumn Chl.-a concentrations of phytoplankton will increase and so will the biomass and dominance of cyanobacteria. Based on our simulations, cyanobacteria constitute a larger proportion of the phytoplankton community with increasing external P at the expense of diatoms and other algae, implying that the Chl.-a increase is mainly due to an increase in cyanobacterial biomass (Supplementary Figures S1–S6). This means that phytoplankton in the reservoir ecosystem respond immediately to external P loading changes, but not to changes in N loading. In the consultation draft of the lake nutrient benchmark technical report published by the Ministry of Ecology and Environment, China, the maximum Chl.-a concentration allowed to avoid a potential harmful situation is 3.4 μg/L. According to the model results, if this requirement has to be met in summer and autumn, the external P loading needs to be reduced by 60 and 30%, respectively.
The water crizes occurring worldwide warn us that algae toxins produced by cyanobacterial blooms in drinking water reservoirs needs to be considered. In 2007, the outbreak of cyanobacterial blooms in Taihu Lake in China produced a large amount of hepatoxins, causing a drinking water disaster that directly affected nearly ten million people in the Taihu Basin (Paerl et al., 2011). In 1996, cyanobacterial toxins in a reservoir in Brazil killed 76 people with the water plant’s filtration system damaged (Wurtsbaugh et al., 2019). Recent studies have also revealed that there is a significant correlation between harmful algal blooms and deaths caused by liver-related diseases (Zhang et al., 2015). In a study by Poste et al. (2013) algae toxin levels were also found to correlate with P concentrations, with variations in the types and amounts of toxins (Wurtsbaugh et al., 2019). Nevertheless, scientists cannot yet predict when algae blooms will produce toxins (Wurtsbaugh et al., 2019). Therefore, in order to prevent the occurrence of water quality crizes in the Jihongtan reservoir, the best option currently is to prevent the occurrence of potentially harmful algal blooms by reducing the external P loading and in some lakes, not least the shallow lakes, by reducing both N and P loading (Paerl et al., 2016).
Recommendations and Management Actions
Ecological models are useful tools to quantify and elucidate the potential effects of changes in the external nutrient loading, and they may thus assist managers in implementing reservoir restoration efforts (Jørgensen, 1976). Seen in light of this, our model results are important since 60 external nutrient loading scenarios indicate a potential water quality crisis. According to the GOTM-FABM-PCLake model for Jihongtan reservoir, a reduction of the external P loading will be effective at controlling algae blooms, while a reduction in N loading will not be sufficient as P is a major limiting factor for cyanobacteria in the Jihongtan reservoir. Nevertheless, a reduction in the external nitrogen load by 70% is required to meet the water quality requirements of the water source (Class III), as the current state of knowledge prevents ecologists from accurately understanding the actual role of these nutrients in the ecosystem (Elser et al., 2007). Zhang and Jørgensen (2005) suggested that the content of Chl.-a in the water of the reservoir should be kept at 4.1–10.0 µg/L or lower. So far, the Jihongtan reservoir Chl.-a content has always remained within this range, but if the external P loading increases by more than 40%, the Chl.-a will exceed this limit in summer and autumn. Therefore, the input of external N and P should be strictly controlled in order to maintain good water quality in the reservoir. This may include 1) change of the land use pattern in the Yellow River Basin, reduction in the use of fertilizers, proper handling of manure, and a strengthening of soil conservation practices; 2) construction of special wastewater treatment facilities for agriculture, industry and urban areas around the reservoir (Paerl et al., 2019); 3) establishment of a grass protection belt around the reservoir to reduce the nutrient input from rainfall and snowfall (Jeppesen et al., 2005) as control of nonpoint pollution has proved difficult and 4) construction of an artificial wetland at the water inlet of the reservoir so that particulate nutrients in the inflowing water will settle and dissolved nutrients may be taken up by the wetland plants and epiphytes (Dunne et al., 2013; Dolph et al., 2019).
Data Availability Statement
The raw data supporting the conclusions of this article will be made available by the authors, without undue reservation.
Author Contributions
QC performed model calibration, validation, scenarios simulation, analyzed data and wrote the manuscript; AN and FH contributed to model coupling; TA and WC performed sensitivity analysis; XZ provided model-driven data; TC and LN provided fund support; EJ revised the manuscript and gave important and critical input; DT planned and designed the research.
Funding
This work was supported by the National Natural Science Foundation of China (31930074, 31870446, 32071574), China Postdoctoral Science Foundation (236070) and State Key Laboratory of Freshwater Ecology and Biotechnology (2019FBZ01). QC was supported by a scholarship from the China Scholarship Council (Grant No. 201704910729) enabling a visit to Aarhus University. TA was supported by Ph.D. funding from the Sino-Danish Center for Education and Research. DT was supported through the CASHFISH project funded by the Danish Council for Independent Research. DT, AN and EJ were also supported by the WATExR project, funded through the EU JPI Climate initiative, the PROGNOS project, funded through the EU JPI Water initiative, and a project on Mechanistic Models for Water Action Planning funded by the Danish EPA. EJ was also supported by the TÜBITAK, BIDEB 2232 program (project 118C250).
Conflict of Interest
The authors declare that the research was conducted in the absence of any commercial or financial relationships that could be construed as a potential conflict of interest.
Acknowledgments
We are grateful to A. M. Poulsen for manuscript editing.
Supplementary Material
The Supplementary Material for this article can be found online at: https://www.frontiersin.org/articles/10.3389/fenvs.2021.632778/full#supplementary-material.
References
Andersen, T. K., Nielsen, A., Jeppesen, E., Hu, F., Bolding, K., Liu, Z., et al. (2020). Predicting ecosystem state changes in shallow lakes using an aquatic ecosystem model: Lake Hinge, Denmark, an example. Ecol. Appl. Eap. 2160, 1–21. doi:10.1002/eap.2160
Arhonditsis, G. B., and Brett, M. T. (2004). Evaluation of the current state of mechanistic aquatic biogeochemical modeling. Mar. Ecol. Prog. Ser. 271, 13–26. doi:10.3354/meps271013
Bruggeman, J., and Bolding, K. (2014). A general framework for aquatic biogeochemical models. Environ. Model. Software. 61, 249–265. doi:10.1016/j.envsoft.2014.04.002
Burchard, H., and Bolding, K. (2001). Comparative analysis of four second-moment turbulence closure models for the oceanic mixed layer. J. Phys. Oceanogr. 31 (8), 1943–1968. doi:10.1175/1520-0485(2001)031<1943:CAOFSM>2.0.CO;2
Carmichael, W. W., and Boyer, G. L. (2016). Health impacts from cyanobacteria harmful algae blooms: implications for the North American great lakes. Harm. Algae. 54, 194–212. doi:10.1016/j.hal.2016.02.002
Carpenter, S. R. (2008). Phosphorus control is critical to mitigating eutrophication. Proc. Natl. Acad. Sci. U.S.A. 105, 11039–11040. doi:10.1073/pnas.0806112105 |
Chen, W., Nielsen, A., Andersen, T. K., Hu, F., Chou, Q., Søndergaard, M., et al. (2019). Modeling the ecological response of a temporarily summer-stratified lake to extreme heatwaves. Water 12 (1), 1–18. doi:10.3390/w12010094
Cheung, M. Y., Liang, S., and Lee, J. (2013). Toxin-producing cyanobacteria in freshwater: a review of the problems, impact on drinking water safety, and efforts for protecting public health. J. Microbiol. 51, 1–10. doi:10.1007/s12275-013-2549-3 |
Conley, D. J., Paerl, H. W., Howarth, R. W., Boesch, D. F., Seitzinger, S. P., Havens, K. E., et al. (2009). Controlling eutrophication: nitrogen and phosphorus. Science 323, 1014. doi:10.1126/science.1167755 |
Cui, Y., Zhu, G., Li, H., Luo, L., Cheng, X., Jin, Y., et al. (2016). Modeling the response of phytoplankton to reduced external nutrient load in a subtropical Chinese reservoir using DYRESM-CAEDYM. Lake Reservoir Manag. 32 (2), 146–157. doi:10.1016/j.ecolmodel.2007.09.028
Dolph, C. L., Boardman, E., Danesh-Yazdi, M., Finlay, J. C., Hansen, A. T., Baker, A. C., et al. (2019). Phosphorus transport in intensively managed watersheds. ISO: Water Resour. Res. 55, 24009. doi:10.1029/2018WR024009
Downing, J. A., and McCauley, E. (1992). The nitrogen—phosphorus relationship in lakes. ISO: Limnol. Oceanogr. 37, 936–945. doi:10.4319/lo.1992.37.5.0936
Dunne, E. J., Coveney, M. F., Marzolf, E. R., Hoge, V. R., Conrow, R., Naleway, R., et al. (2013). Nitrogen dynamics of a large-scale constructed wetland used to remove excess nitrogen from eutrophic lake water. Ecol. Eng. 61, 224–234. doi:10.1016/j.ecoleng.2013.09.039
Elser, J. J., Bracken, M. E. S., Cleland, E. E., Gruner, D. S., Harpole, W. S., Hillebrand, H., et al. (2007). Global analysis of nitrogen and phosphorus limitation of primary producers in freshwater, marine and terrestrial ecosystems. Ecol. Lett. 10, 1135–1142. doi:10.1111/j.1461-0248.2007.01113.x |
Glibert, P. M., and Burford, M. A. (2017). Globally changing nutrient loads and harmful algal blooms recent advances, new paradigms, and continuing challenges. Oceanography 30, 58–69. doi:10.5670/oceanog.2017.110
Grattan, L. M., Holobaugh, S., and Morris, J. G. (2016). Harmful algal blooms and public health. Harm. Algae. 57, 2–8. doi:10.1016/j.hal.2016.05.003
Havens, K. E., Kukushima, T., Xie, P., Iwakuma, T., James, R. T., Takamura, N., et al. (2001). Nutrient dynamics and the eutrophication of shallow lakes Kasumigaura (Japan), Donghu (PR China), and Okeechobee (United States). Environ. Pollut. 111, 263–272. doi:10.1016/s0269-7491(00)00074-9 |
Howarth, R. W., and Marino, R. (2006). Nitrogen as the limiting nutrient for eutrophication in coastal marine ecosystems: evolving views over three decades. Limnol. Oceanogr. 51, 364–376. doi:10.4319/lo.2006.51.1_part_2.0364
Hu, F., Bolding, K., Bruggeman, J., Jeppesen, E., Flindt, M. R., van Gerven, L., et al. (2016). FABM-PCLake—linking aquatic ecology with hydrodynamics. Geosci. Model Dev. 9 (6), 1–15. doi:10.5194/gmd-9-2271-2016
Janse, J. H., and Liere, L. V. (1995). PCLake: a modelling tool for the evaluation of lake restoration scenarios. Water Sci. Technol. 31 (8), 371–374. doi:10.2166/wst.1995.0332
Janse, J. H. (2005). Model studies on the eutrophication of shallow lakes and ditches. Doctoral dissertation. Wageningen, Netherlands: Wageningen University Available at: http://edepot.wur.nl/121663 Retrieved from Informatie voor professionals in voedsel en groen.
Janse, J. H., Scheffer, M., Lijklema, L., Liere, L. V., Sloot, J. S., Mooij, W. M., et al. (2010). Estimating the critical phosphorus loading of shallow lakes with the ecosystem model PCLake: sensitivity, calibration and uncertainty. Ecol. Model. 221 (4), 654–665. doi:10.1016/j.ecolmodel.2009.07.023
Jeppesen, E., Søndergaard, M., Jensen, J. P., Havens, K. E., Anneville, O., Carvalho, L., et al. (2005). Lake responses to reduced nutrient loading—an analysis of contemporary long‐term data from 35 case studies. Freshw. Biol. 50, 1747–1771. doi:10.1111/j.1365-2427.2005.01415.x
Jeppesen, E., Sondergaard, M., Lauridsen, T. L., Kronvang, B., Bekiloglu, M., Lammens, E., et al. (2007). Danish and other European experiences in managing shallow lakes. Lake Reservoir Manag. 23, 439–451. doi:10.1080/07438140709354029
Jørgensen, S. E. (1976). A eutrophication model for a lake. Ecol. Model. 2, 147–165. doi:10.1016/0304-3800(76)90030-2
Koreiviene, J., Anne, O., Kasperoviciene, J., and Burskyte, V. (2014). Cyanotoxin management and human health risk mitigation in recreational waters. Environ. Monit. Assess. 186, 4443–4459. doi:10.1007/s10661-014-3710-0 |
Lewis, W. M., and Wurtsbaugh, W. A. (2008). Control of lacustrine phytoplankton by nutrients: erosion of the phosphorus paradigm. Int. Rev. Hydrobiol. 93, 446–465. doi:10.1002/iroh.200811065
Lewis, W. M., Wurtsbaugh, W. A., and Paerl, H. W. (2011). Rationale for control of anthropogenic nitrogen and phosphorus to reduce eutrophication of inland waters. Environ. Sci. Technol. 45, 10300–10305. doi:10.1021/es202401p |
Ma, S. N., Wang, H. J., Wang, H. Z., Li, Y., Liu, M., Liang, X. M., et al. (2018). High ammonium loading can increase alkaline phosphatase activity and promote sediment phosphorus release: a two-month mesocosm experiment. Water Res. 145, 388–397. doi:10.1016/j.watres.2018.08.043 |
WWF (2018). Living planet report—2018: aiming higher. Editors M. Grooten, and R. Almond (Gland, Switzerland: WWF).
Moras, S., Ayala, A. I., and Pierson, D. C. (2019). Historical modelling of changes in Lake Erken thermal conditions. Hydrol. Earth Syst. Sci. 2019, 1–18. doi:10.5194/hess-23-5001-2019
Nielsen, A., Bolding, K., Hu, F. J., and Dennis Trolle, D. (2017). An open source QGIS-based workflow for model application and experimentation with aquatic ecosystems. Environ. Model. Soft. 95, 358–364. doi:10.1016/j.envsoft.2017.06.032
Nielsen, A., Trolle, D., Bjerring, R., Søndergaard, M., Olesen, J. E., Janse, J. H., et al. (2014). Effects of climate and nutrient load on the water quality of shallow lakes assessed through ensemble runs by PCLake. Ecol. Appl. 24 (8), 1926–1944. doi:10.1890/13-0790.1 |
OECD (1982). Eutrophication of waters—monitoring, assessment and control. Paris, France: The Organisation for Economic Cooperation and Development (OECD).
Otten, T. G., and Paerl, H. W. (2015). Health effects of toxic cyanobacteria in U.S. drinking and recreational waters: our current understanding and proposed direction. Curr. Environ. Health Rep. 2, 75–84. doi:10.1007/s40572-014-0041-9 |
Özkundakci, D., Hamilton, D., and Trolle, D. (2011). Modelling the response of a highly eutrophic lake to reductions in external and internal nutrient loading. N. Z. J. Mar. Freshw. Res. 45 (2), 165–185. doi:10.1080/00288330.2010.548072
Paerl, H. W., Havens, K. E., Xu, H., Zhu, G., McCarthy, M. J., Newell, S. E., et al. (2019). Mitigating eutrophication and toxic cyanobacterial blooms in large lakes: the evolution of a dual nutrient (N and P) reduction paradigm. Hydrobiologia 3, 1–17. doi:10.1007/s10750-019-04087-y
Paerl, H. W., Scott, J. T., McCarthy, M. J., Newell, S. E., Gardner, W. S., Havens, K. E., et al. (2016). It takes two to tango: when and where dual nutrient (N & P) reductions are needed to protect lakes and downstream ecosystems. Environ. Sci. Technol. 50, 10805–10813. doi:10.1021/acs.est.6b02575 |
Paerl, H. W., Xu, H., McCarthy, M. J., Zhu, G., Qin, B., Li, Y., et al. (2011). Controlling harmful cyanobacterial blooms in a hyper-eutrophic lake (Lake Taihu, China): the need for a dual nutrient (N & P) management strategy. Water Res. 45, 1973–1983. doi:10.1016/j.watres.2010.09.018 |
Poste, A. E., Hecky, R. E., and Guildford, S. J. (2013). Phosphorus enrichment and carbon depletion contribute to high Microcystis biomass and microcystin concentrations in Ugandan lakes. Limnol. Oceanogr. 58, 1075–1088. doi:10.4319/lo.2013.58.3.1075
Sas, H. (1989). Lake restoration by reduction of nutrient loading: expectations, experiences, extrapolation. Limnol. Oceanogr. 35, 1412–1413. doi:10.4319/lo.1990.35.6.1412
Schindler, D. (2006). Recent advances in the understanding and management of eutrophication. Limnol. Oceanogr. 51, 356–363. doi:10.4319/lo.2006.51.1_part_2.0356
Schindler, D. W. (1977). Evolution of phosphorus limitation in lakes. Science 195, 260–262. doi:10.1126/science.195.4275.260 |
Schladow, S. G., and Hamilton, D. P. (1997). Prediction of water quality in lakes and reservoirs: part II - model calibration, sensitivity analysis and application. Ecol. Model. 96, 111–123. doi:10.1016/S0304-3800(96)00063-4
Stewart, I., Seawright, A. A., and Shaw, G. R., 2008. Cyanobacterial poisoning in livestock, wild mammals and birds--an overview, Adv. Exp. Med. Biol. 619. 613–637. doi:10.1007/978-0-387-75865-7_28 |
Trolle, D., Jørgensen, T. B., and Jeppesen, E. (2008). Predicting the effects of reduced external nitrogen loading on the nitrogen dynamics and ecological state of deep Lake Ravn, Denmark, using the DYRESM–CAEDYM model. Limnologica 38, 220–232. doi:10.1016/j.limno.2008.05.009
Umlauf, L., and Burchard, H. (2005). Second-order turbulence closure models for geophysical boundary layers. A review of recent work. Continent. Shelf Res. 25, 795–827. doi:10.1016/j.csr.2004.08.004
Vitousek, P. M., and Howarth, R. W. (1991). Nitrogen limitation on land and in the sea - how can it occur. Biogeochemistry 13, 87–115. doi:10.1007/bf00002772
Wetzel, R. G. (1992). Clean water: a fading resource. Hydrobiologia 243–244, 21–30. doi:10.1007/BF00007017 |
Wetzel, R. G. (1990). “Land-water interfaces: metabolic and limnological regulators,” in Congress of the International association of theoretical and applied limnology, (1989, Munich, Fed Rep Ger) 24, 6–24. doi:10.1080/03680770.1989.11898687
Wurtsbaugh, W. A., Paerl, H. W., and Dodds, W. K. (2019). Nutrients, eutrophication and harmful algal blooms along the freshwater to marine continuum. ISO: Wiley Interdiscip. Rev.-Water, 6, 1373. doi:10.1002/wat2.1373
Zhang, F., Lee, J., Liang, S., and Shum, C. K. (2015). Cyanobacteria blooms and non-alcoholic liver disease: evidence from a county level ecological study in the United States. Environ. Health. 14, 41. doi:10.1186/s12940-015-0026-7 |
Keywords: FABM-PCLake, Lake ecosystem model, hydrodynamic model, water quality crisis, external nutrients load, drinking water safety
Citation: Chou Q, Nielsen A, Andersen TK, Hu F, Chen W, Zhang X, Cao T, Ni L, Jeppesen E and Trolle D (2021) Assessing Impacts of Changes in External Nutrient Loadings on a Temperate Chinese Drinking Water Reservoir. Front. Environ. Sci. 9:632778. doi: 10.3389/fenvs.2021.632778
Received: 24 November 2020; Accepted: 15 January 2021;
Published: 08 March 2021.
Edited by:
Ke-Qing Xiao, University of Leeds, United KingdomReviewed by:
Ming Su, Research Center for Eco-environmental Sciences (CAS), ChinaJianfeng Feng, Nankai University, China
Copyright © 2021 Chou, Nielsen, Andersen, Hu, Chen, Zhang, Cao, Ni, Jeppesen and Trolle. This is an open-access article distributed under the terms of the Creative Commons Attribution License (CC BY). The use, distribution or reproduction in other forums is permitted, provided the original author(s) and the copyright owner(s) are credited and that the original publication in this journal is cited, in accordance with accepted academic practice. No use, distribution or reproduction is permitted which does not comply with these terms.
*Correspondence: Xiaolin Zhang, zhangxl@ihb.ac.cn