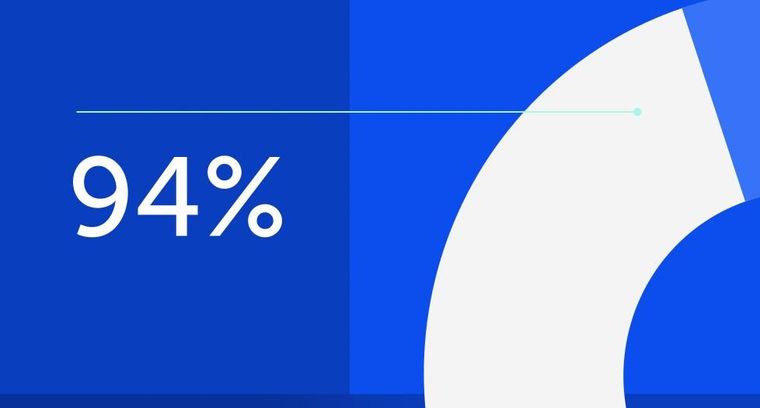
94% of researchers rate our articles as excellent or good
Learn more about the work of our research integrity team to safeguard the quality of each article we publish.
Find out more
ORIGINAL RESEARCH article
Front. Environ. Sci., 16 March 2021
Sec. Environmental Informatics and Remote Sensing
Volume 9 - 2021 | https://doi.org/10.3389/fenvs.2021.555216
This article is part of the Research TopicSpatial Downscaling of Remotely Sensed Hydrological Cycle Components: Algorithms Development, Evaluation and ApplicationView all 4 articles
DISPATCH is a disaggregation algorithm of the low-resolution soil moisture (SM) estimates derived from passive microwave observations. It provides disaggregated SM data at typically 1 km resolution by using the soil evaporative efficiency (SEE) estimated from optical/thermal data collected around solar noon. DISPATCH is based on the relationship between the evapo-transpiration rate and the surface SM under non-energy-limited conditions and hence is well adapted for semi-arid regions with generally low cloud cover and sparse vegetation. The objective of this paper is to extend the spatio-temporal coverage of DISPATCH data by 1) including more densely vegetated areas and 2) assessing the usefulness of thermal data collected earlier in the morning. Especially, we evaluate the performance of the Temperature Vegetation Dryness Index (TVDI) instead of SEE in the DISPATCH algorithm over vegetated areas (called vegetation-extended DISPATCH) and we quantify the increase in coverage using Sentinel-3 (overpass at around 09:30 am) instead of MODIS (overpass at around 10:30 am and 1:30 pm for Terra and Aqua, respectively) data. In this study, DISPATCH is applied to 36 km resolution Soil Moisture Active and Passive SM data over three 50 km by 50 km areas in Spain and France to assess the effectiveness of the approach over temperate and semi-arid regions. The use of TVDI within DISPATCH increases the coverage of disaggregated images by 9 and 14% over the temperate and semi-arid sites, respectively. Moreover, including the vegetated pixels in the validation areas increases the overall correlation between satellite and in situ SM from 0.36 to 0.43 and from 0.41 to 0.79 for the temperate and semi-arid regions, respectively. The use of Sentinel-3 can increase the spatio-temporal coverage by up to 44% over the considered MODIS tile, while the overlapping disaggregated data sets derived from Sentinel-3 and MODIS land surface temperature data are strongly correlated (around 0.7). Additionally, the correlation between satellite and in situ SM is significantly better for DISPATCH (0.39–0.80) than for the Copernicus Sentinel-1-based (−0.03 to 0.69) and SMAP/S1 (0.37–0.74) product over the three studies (temperate and semi-arid) areas, with an increase in yearly valid retrievals for the vegetation-extended DISPATCH algorithm.
Soil moisture (SM) is an important element in the hydrologic cycle, especially influencing precipitation, infiltration, and runoff (Hamlet et al., 2007). SM is thus useful for different applications such as meteorology (Dirmeyer, 2000), climatology (Douville, 2004), hydrology (Chen et al., 2011) and agriculture (Guérif and Duke, 2000). SM has a very high spatio-temporal variability and to approximate such a variability of SM, in situ measurements are not applicable on a global basis. Instead remote sensing techniques have a strong potential to provide SM estimates at multiple scales globally.
Currently, L-band radiometry is acknowledged as one of the most efficient technique to retrieve the surface SM on a global scale. Based on L-band radiometer, Soil Moisture and Ocean Salinity (SMOS, Kerr et al., 2012) satellite was launched by European Space Agency (ESA) on November 2, 2009 and Soil Moisture Active Passive (SMAP, Entekhabi et al., 2010) was launched by National Aeronautics and Space Administration (NASA) on January 31, 2015. Both satellites provide SM at a sensing depth of 3–5 cm with a spatial resolution of about 40 km and a revisit cycle of about 3 days on a global basis. Since L-band emission is highly sensitive to SM and relatively less sensitive to soil roughness and vegetation optical-depth (Wigneron et al., 2017), it can be used to derive SM with high precision.
Passive (including L-band) microwave-derived SM products are regularly evaluated and are found to be suitable for hydro-climatic applications (Wanders et al., 2014; Lievens et al., 2015). But for most of the hydrological and agricultural purposes, SM data are required at a much higher (i.e., at least kilometric) spatial resolution. Active microwave (e.g., Synthetic Aperture Radar) can be used to derive SM at kilometric or sub-kilometric spatial resolution (Wegmuller and Werner, 1997; Bauer-Marschallinger et al., 2018). However, the major disadvantage of the radar techniques is the high sensitivity of the surface backscatter to disturbing factors such as notably vegetation structure (Waite and MacDonald, 1971), soil roughness (Verhoest et al., 2008) and topography (Atwood et al., 2014). Consequently, to overcome the limitation of both microwave techniques, various researches have been done in the past to combine active and passive microwave data (Narayan et al., 2006; Piles et al., 2009; Das et al., 2010). In particular, based on this technique, NASA recently developed a method that provides SM at 9 and 3 km resolution from SMAP data (Jagdhuber et al., 2017; Lievens et al., 2017; Das et al., 2018).
Alternatively, optical/thermal sensors such as Moderate resolution Imaging Spectroradiometer (MODIS) are extensively used to retrieve SM proxies from land surface temperature (LST) and normalized vegetation index (NDVI) (Peng et al., 2015a; Zhang and Zhou, 2016). The triangle (Carlson, 2007) or trapezoid (Moran et al., 1994) method is built by assuming that it covers the sensitivity of LST for fully vegetated areas and bare soil conditions. The fully dry and well-watered surface conditions can be determined as edges of the LST-NDVI feature space. Based on the LST-NDVI approach, various moisture index methods have been proposed like the crop water stress index (CWSI, Moran et al., 1994), the vegetation condition index (VCI, Kogan, 1995), the normalized difference water index (NDWI, Gao, 1996) and the temperature vegetation dryness index (TVDI) (Sandholt et al., 2002). The TVDI in particular is a land surface dryness index used to calculate water-stress condition.
Further, an optical-derived SM proxy is used to disaggregate passive microwave derived SM data by establishing a link between LST and SM through the evapotranspiration process (Merlin et al., 2005; Kim and Hogue, 2012; Peng et al., 2015a; Peng et al., 2015b). Based on this, the DISPATCH method (Merlin et al., 2012; Merlin et al., 2013) was developed. DISPATCH estimates the soil evaporative efficiency (SEE, defined as the ratio of actual to potential soil evaporation) from optical/thermal data and expresses the disaggregated SM through a downscaling relationship between the SM observed at low resolution (LR) and the SEE derived at high resolution (HR). Given that SEE has a mostly linear relationship with soil temperature (Merlin et al., 2013), SEE is estimated as the optical-derived soil temperature normalized by its maximum and minimum values corresponding to dry and wet soil conditions in the LST-NDVI feature space (Merlin et al., 2012). For routine application of DISPATCH, the C4DIS processor was implemented at the Centre Aval de Traitement des données SMOS (CATDS) as a level-4 SM product (Molero et al., 2016). C4DIS processor provides SM at 1 km resolution product on a daily-global basis using LR SMOS SM and HR MODIS data. The C4DIS was recently adapted to integrate SMAP and Sentinel-3 data in replacement of SMOS and MODIS data, respectively.
Various researches have shown that the application of DISPATCH to SMAP or SMOS data provides a 1 km resolution SM product with satisfying accuracy in arid and semi-arid regions (Malbéteau et al., 2016; Molero et al., 2016; Colliander et al., 2017; Mishra et al., 2018). However DISPATCH, like all the optical-based SM disaggregation methods, has two main intrinsic limitations: 1) the soil surface temperature that is related to the surface (0–5 cm) SM cannot be retrieved underneath the vegetation cover and 2) optical/thermal data are unavailable in cloudy conditions. Such constraints significantly reduce the spatio-temporal coverage of optical-disaggregated SM images, which potentially hinders several applications requiring data at high temporal frequency.
In this context, the paper aims to partly overcome the above mentioned limitations by testing three significant changes in the DISPATCH algorithm. Firstly, over densely vegetated pixels, the DISPATCH downscaling relationship is implemented using the TVDI (Sandholt et al., 2002), by assuming over those areas a link between the surface SM (as sensed by SMOS/SMAP) and the TVDI-derived root zone SM. Secondly, the enhanced vegetation index (EVI) is used in place of NDVI to improve the robustness of disaggregated SM over vegetated region. In fact, EVI is expected to be more sensitive to vegetation density and to correct for inaccuracies due to atmospheric and soil conditions. Thirdly, Sentinel-3 LST is tested as input to DISPATCH in place of MODIS LST by assuming that an earlier optical/thermal observation is generally less affected by clouds (Georgiana Stefan et al., 2018).
The main objective of this paper is therefore to improve the spatio-temporal coverage and the robustness over vegetated areas of the 1 km resolution of DISPATCH SM. The approach is applied to SMAP SM data and tested over three 50 km by 50 km study areas in France and Spain with one temperate and two semi-arid regions. Results of the new vegetation-extended DISPATCH algorithm is assessed against in situ measurements collected in all three study areas, as well as against the 1 km resolution, Copernicus SM data derived from Sentinel-1 radar data and SMAP/S1 data derived from Sentinel-1 and SMAP.
Three study areas of 50 km by 50 km are selected in the South-West of France (ICOS sites), and in the West (REMEDHUS sites) and East (dryland sites) of Spain (location is shown in Figure 1). The extent of each study area is defined in order to encompass at least one SMAP pixel and to represent the sub-pixel variability at the 1 km resolution. The main objective for the selection of study areas is two-fold 1) to evaluate the performance of DISPATCH under different climatic conditions including temperate and semi-arid, and 2) to evaluate the performance of DISPATCH over different land cover types such as agricultural land and dryland areas. A detailed description of the SM monitoring sites within each of the three study areas is provided below.
i) ICOS network (South-West of France): It includes Auradé (43°32′58.81 N, 01°06′22.08 E) and Lamasquère (43°50′05 N, 01°24′19 E), which are located near Toulouse at a distance of 12 km from each other. The study area has temperate climatic conditions with an annual average precipitation of 700 mm. The land is mainly covered by agricultural field. Soil texture is clay loam for Auradé with clay and sand contents of 32.3 and 20.6%, respectively, while soil texture for Lamasquère is clay with clay and sand fraction contents of 54 and 12%, respectively. SM is measured by CS616 (Campbell Scientific Inc., Logan, UT, USA) probes at depths of 0.05, 0.10, and 0.30 m. CS616 probe uses soil dielectric permittivity to measure the volumetric soil water content. The in situ SM data collected at 5 cm depth for years 2017 and 2018 are used in this study. Detailed information about field instrumentation and agricultural practices can be found in Béziat et al. (2009) and Tallec et al. (2013).
ii) REMEDHUS network (West of Spain): REMEDHUS is a very dense network, consisting of 20 stations located near the center of Duero basin (41.1–41.5°N, 5.1–5.7°W). The study area has semi-arid Mediterranean climate with an annual average precipitation 385 mm. In this paper 13 stations are used. The land is mainly covered by croplands, shrublands, forests and pasture. Soil type is silty and clayey sand. SM is measured by a dielectric sensor (hydra probe and Stevens water monitoring system), which measures the volumetric SM at a depth of 0.00–0.05 m. The in situ SM collected for 2017 are used in this study is obtained from the International Soil Moisture Network (ISMN) (Dorigo et al., 2011).
iii) Dryland sites (East of Spain): Dryland areas are selected from the Tarragona province of Catalunya, Spain. Land is mainly covered by rainfed crops. Soil texture of this area is clayey. The monitoring network consists of seven stations. SM is measured at a depth of 5 cm by Teros sensor 10. The in situ SM data collected from June to November 2019 are used in this study. The dryland sites area exhibits a semi-arid Mediterranean climate, which is dry and warm in summer and cold and wet in winter. The average annual precipitation is 385 mm with an elevation of 700–900 m above sea level.
FIGURE 1. Location of the three study areas: (A) ICOS network in South Western France (B) Dryland network in Eastern Spain and (C) REMEDHUS network in Western Spain.
SMAP is a L-band satellite mission, launched in January 2015, that combines 1 km resolution radar and 36 km resolution radiometer observations to provide SM at 9 km resolution. But due to improper functioning of SMAP radar, currently SMAP provides SM at 36 km resolution (radiometer) on a global-daily basis. SMAP satellite has a near-polar sun-synchronous orbit with an altitude of 658 km. The SMAP swath is about 1,000 km width with a revisit cycle of 2–3 days. In this paper, SMAP level-3 daily SM product (named as L3SMP A/D, version 005) with an ascending and descending overpass of 6 pm/6 am is used separately as an input to DISPATCH algorithm. These products are in HDF format and cylindrically projected on the EASE grid version 2.0. SMAP data can be downloaded from https://nsidc.org/data/SPL3SMP/versions/5. In addition to this, SMAP/S1 (named as L2_SM_SP) provides SM at 1 km resolution is used for the statistical analysis with DISPATCH data. SMAP/S1 use Sentinel-1A and Sentinel-1B to disaggregate SMAP (∼36 km) data at 1 km resolution.
The C4DIS processor presented in Molero et al. (2016) uses the MODIS version 6 optical/thermal data. The Terra overpass (10:30 am)—named as MOD11A- and Aqua overpass (1:30 pm)—named as MYD11A1—gives 1 km resolution LST data on a daily basis. In DISPATCH algorithm, 6 MODIS LST products (1 day before, same day and 1 day after the SMAP overpass) are used as an input for each SMAP ascending and descending overpass. MODIS version 6 MOD13 is used to monitor canopy structure, leaf area index and vegetation greenness extent, and contains two vegetation indices—NDVI and EVI—as sub-datasets. MODIS NDVI and EVI products provide data continuously at 1 km spatial and 16-day temporal resolution for global vegetation coverage. NDVI is used in the original DISPATCH algorithm for disaggregation of SM at HR (Merlin et al., 2012). NDVI is defined as:
where
The main limitation of NDVI is that it is very sensitive to canopy background (Huete et al., 2002) and gets saturated in conditions of high biomass (Gitelson, 2004). Another limitation is that it shows a non-linear behavior like ratio-based indices (Jiang et al., 2006) and is affected by atmospheric noise (Liu and Huete, 1995). EVI was thus developed to improve the sensitivity to high biomass conditions. It also decouples the canopy background signal (Huete et al., 2002) and reduces atmospheric influence (Matsushita et al., 2007). EVI is computed as:
where,
DISPATCH relies on the fractional vegetation cover
where
Similarly,
where
EVI aims to provide a VI with reduced sensitivity to (daily) atmospheric effects and to (constant) background soil effects, while no such correction for soil or atmospheric effects is undertaken for NDVI. The time series and scatter plot comparisons between NDVI and EVI are hence presented to visually assess the possible artifacts in the temporal and in spatial pattern in NDVI that may occur due to atmospheric disruptive effects and/or to the background soil variability between study areas. Figure 2 presents the time series of the 1 km resolution fractional vegetation cover derived from NDVI and EVI at Auradé site (ICOS study area), BA (dryland area) and F11 (REMEDHUS network study area) (left side) and also presents the scatter plot comparison of both fractional vegetation cover estimates extracted over ICOS, dryland, and REMEDHUS study areas (right side). The time series evolution in Figure 2 observed a relatively smoother dynamics of EVI compared to NDVI, suggesting that the atmospheric effects are reduced in EVI data. The effect of different soil types is observed in the spatial scatter plot of Figure 2 (right side) between the fractional vegetation cover derived from NDVI and EVI over each of the three study areas. Relative differences are more significant over the dryland sites. Since the dryland sites are covered by vineyards, a big jump of NDVI is not observed in the middle of the season. It is therefore observed that NDVI and EVI behave differently in different areas depending on meteorological conditions, canopy structure and soil type. This is the rationale for evaluating the performance of DISPATCH using EVI and NDVI vegetation indices separately for different regions.
FIGURE 2. Times series of fractional vegetation cover derived from NDVI and EVI (left) and a spatial scatterplot of fractional vegetation cover derived from NDVI and EVI (right) for Auradé (ICOS) site, 2017 (A,B); BA (dryland) site, 2019 (C,D); and L03 (REMEDHUS) site, 2017 (E,F).
Sentinel-3 satellite was launched by ESA in February 2016. Sentinel-3 has a sun-synchronous polar orbit with an altitude of 815 km and an overpass at 09:30 a.m. The LST Sentinel-3 product is derived from data collected by the Sea and Land Surface Temperature Radiometer (SLSTR) instrument. SLSTR is a multi-channel radiometer with nine spectral bands including three thermal bands, which are used to derive LST from the split-window method with an accuracy better than 1 K (Sobrino et al., 2015). Despite the earlier overpass of Sentinel-3 and the expected enhanced accuracy of Sentinel-3 LST data, SLSTR LST data are pretty similar to MODIS LST data in terms of spatio-temporal resolution: 1 km resolution with 1- or 2-day revisit time. In this paper, the daily Sentinel-3 LST product named SL2LST downloaded from https://scihub.copernicus.eu is used as input to DISPATCH algorithm in place of MODIS LST. In the DISPATCH algorithm, three Sentinel-3 LST images are used (1 day before, same day, and 1 day after) as input for each SMAP ascending and descending overpass. Note that in this application, the NDVI/EVI data are still derived from MODIS to focus on the DISPATCH output differences associated with the input data of (MODIS or Sentinel-3) LST.
GTOPO 30 digital elevation model (DEM) data at 30 arc second resolution are used to correct the 1 km resolution MODIS/Sentinel-3 LST for topographic effects, before its use for SEE/TVDI estimates within DISPATCH (Merlin et al., 2013). The DEM can be downloaded from https://lta.cr.usgs.gov/GTOPO30.
Copernicus global land service provides a SM product over Europe at 1 km resolution from 2016. The change detection method from Technological University of Vienna (TU-Wien) is used to derive daily relative SM estimates from the C-band Sentinel-1 backscatter time series collected in Interferometric Wade Swath (IW) and VV-polarization mode (Bauer-Marschallinger et al., 2018). In practice, the Copernicus Sentinel-1 relative SM (expressed in percentage from 0 to 100%) is derived from the angle-normalized backscattering coefficient linearly scaled between wet and dry conditions at each location individually. Data can be downloaded at https://land.copernicus.eu/global/products/ssm.
For comparing the performance of Copernicus and DISPATCH SM data sets at the validation sites, the Copernicus relative SM is converted into volumetric SM from the extreme SM values estimated at the site level using texture information:
Where
The DISPATCH downscaling equation relies on HR optical-derived SM proxy (SEE in the current version of DISPATCH) to disaggregate LR (SMOS or SMAP) SM at HR:
where
where
where,
As fully described in Merlin et al. (2012), the retrieval of soil temperature in Eq. 8 depends on the estimation of vegetation temperature, which depends on the location of the associated HR pixel in the LST-fvg feature space. As illustrated in Figure 3A, the LST-fvg feature space is divided in four zones A, B, C, and D. In zone D, where the LST is mainly controlled by the vegetation temperature, the retrieved soil temperature is assumed to be constant meaning that SEE is uniform with the SMOS/SMAP pixel and the downscaling relationship is not applied. In summary, the use of SEE in the downscaling relationship of Eq. 6 implies that disaggregation is only possible in zones A, B, and C. No disaggregated SM value is provided by DISPATCH for the HR pixels located in zone D.
FIGURE 3. Illustration of DISPATCHclassic(A) and DISPATCHveg–ext(B) polygons in the LST-fvg feature space for ICOS study area on DOY 204, 2018.
Note that Eq. 6 is applied to all SMOS/SMAP pixels over which the cloud cover percentage is lower than a given threshold (named the cloud cover threshold) set to 33% in the current DISPATCH version. Under cloud cover, the SEE values are set to the average SEE within the LR pixel, but no disaggregated SM value is provided.
More details on the DISPATCH method are provided in Merlin et al. (2012) and Merlin et al. (2013). Note that the original DISPATCH algorithm as described by the above equations and implemented in the current C4DIS processor (Molero et al., 2016) is named DISPATCHclassic in this paper.
The objective of the new DISPATCH version is to apply the downscaling relationship of Eq. 6 to zone D, where the variability of LST for a given
TVDI is defined as
where,
It is reminded that DISPATCHclassic operates only on zones A, B, C of the LST-fvg feature space where the soil temperature can be retrieved but DISPATCHveg–ext algorithm additionally operates on zone D where vegetation temperature is dominant. Replacing SEE by TVDI in Eq. 6 thus involves modifying the algorithm for estimating temperature endmembers. SEE is more sensitive to the surface soil moisture via the soil temperature retrieved over bare or partially vegetated pixels, while TVDI is more sensitive to the root zone soil moisture via the vegetation temperature retrieved over vegetated pixels. As a matter of fact, SEE cannot be retrieved from satellite over densely vegetated areas. Therefore, TVDI has the advantage over SEE to provide vegetation water stress information over densely vegetated areas that can be translated into soil moisture variabilities within DISPATCH. However, the main issue with the use of TVDI instead of SEE is the link between the root zone soil moisture (information provided by TVDI) and the surface soil moisture (as retrieved from SMAP), which might not be linear (Albergel et al., 2008; Ford et al., 2013).
Figure 3 gives an illustration of the temperature endmembers retrieved from the DISPATCHclassic (A) and DISPATCHveg–ext (B) algorithms for a given date within a given SMAP pixel. In this example, the SMAP pixel over the ICOS study area was selected. Note that the values of temperature endmembers are calibrated and will be different for each SMAP pixel and for each SMAP overpass time. Visually, it is observed from the graph in Figure 3B that the polygonal envelop for DISPATCHveg–ext includes all pixel LST values (represented by black dots). This is not the case for DISPATCHclassic as illustrated in the graph of Figure 3A where several pixel LST values are clearly located above the dry edge. Moreover, if we consider zone D uniquely (represented by a grey area), the pixel LST values that come under zone D are discarded from the disaggregation in DISPATCHclassic (Figure 3A), whereas the pixel LST values in zone D are included for disaggregation in DISPATCHveg–ext (Figure 3B). The DISPATCHveg–ext algorithm thus extends the applicability of DISPATCH to zone D as well as improves the robustness of dry/wet edges determination. The main difference between DISPATCHclassic and DISPATCHveg–ext for estimating temperature endmembers is two-fold: 1) instead of using directly the maximum and minimum observed LST values (DISPATCHclassic) to determine respectively the dry bare soil and wet full cover vertices, DISPATCHveg–ext iteratively ensures that most of the data points are kept within the polygon, and 2) the maximum to minimum vegetation temperature difference is forced to be equal to or larger than half the maximum to minimum soil temperature difference. The rationale behind the second requirement is that the maximum to minimum soil temperature difference and the maximum to minimum vegetation temperature difference should be rather close from the energy budget perspective (Stefan et al., 2015; Yang et al., 2015). It has been demonstrated that the uncertainty in dry and wet boundaries is reduced and the accuracy in the associated SM proxies is increased when the second requirement is satisfied.
In practice, the new algorithm for estimating
(1) First guess estimates of temperature endmembers are provided by DISPATCHclassic algorithm (Figure 3A for illustration).
(2) An offset is applied to the first guess dry (wet) edge to make it pass through the point corresponding to the maximum (minimum) observed LST.
(3) An additional constraint is applied to the maximum vegetation temperature. In case the maximum to minimum vegetation temperature difference is lower than half the maximum to minimum soil temperature difference, the final maximum temperature is set to:
The above requirement is especially useful over relatively wet SMOS/SMAP pixels where water-stressed vegetation conditions do not occur, i.e., where the first guess maximum vegetation temperature is not sufficiently representative.
In this section, the DISPATCHveg–ext algorithm is evaluated over the study sites and its performance is assessed compared to the DISPATCHclassic algorithm and to the Copernicus Sentinel-1-based SM retrieval method. DISPATCHveg–ext is run in different modes with the use of MODIS EVI instead of MODIS NDVI, and the use of Sentinel-3 LST instead of MODIS LST. The objective is to quantify the potential increase in the spatial coverage of DISPATCH data and its accuracy over vegetated regions under temperate and semi-arid conditions.
DISPATCHclassic and DISPATCHveg–ext algorithms are run with the same input data to compare the spatial extent of their 1 km resolution disaggregated SM output. Readers are reminded that DISPATCHveg–ext is an extension of the DISPATCHclassic algorithm to include vegetated areas (disaggregation is undertaken in all zones in the LST- feature space, including zone D). Figure 4 illustrates the visual comparison of 1 km resolution disaggregated SM images obtained after running DISPATCHclassic and DISPATCHveg–ext algorithms on DOY 112 for 2018 over the H18V04 MODIS tile. Note that there are multiple void regions in the output of DISPATCHclassic disaggregated SM. These void regions appear in the output images when the corresponding input pixels belong to zone D (densely vegetated areas). The DISPATCHclassic disaggregation algorithm does not give disaggregated SM values over those areas. On the other hand, DISPATCHveg–ext disaggregated image fills the void region attributed to vegetation cover by using TVDI in DISPATCH algorithm. The void regions are still visible in the output of DISPATCHveg–ext image. Note that SMAP does not retrieve SM at high altitude which is covered by snow such as alps and pyrenees (O’Neill et al., 2018). Due to this, there is no SM retrieval in this region and the regions remains void. The void regions in high altitude are consistently observed across DISPATCHclassic, DISPATCHveg–ext, and NDVI images.
FIGURE 4. Images over the MODIS tile H18V04 of 1 km resolution disaggregated SM derived from DISPATCHclassic(left top) and DISPATCHveg–ext(right top) and NDVI (left bottom) on DOY 112, 2018.
Different cloud cover thresholds (10, 30, 50 and 70%) are also used separately in DISPATCHveg–ext and DISPATCHclassic algorithms to examine the effect on the spatial coverage of valid pixels. Such a comparison is made on a yearly basis (2017) over distinct spatial extents: the MODIS tiles H17V04 and H18V04 and the 50 km by 50 km REMEDHUS, ICOS, and dryland study areas. From Table 1 it is observed that there is an overall increase in the percentage of valid pixels in DISPATCHveg–ext disaggregated SM. The increase is about 3–6% in semi-arid areas and 6–9% in the temperate area as compared to DISPATCHclassic, for different cloud-free threshold values. The increase in coverage is more evident in temperate sites because agricultural areas generally have a larger NDVI than semi-arid/dryland areas. For the MODIS tile extent, the relative increase in coverage over vegetated areas (from 58 to 86% depending on the cloud cover threshold) is very significant. The threshold values of cloud cover have a rather small effect at the 50 km by 50 km scale for both DISPATCH algorithms. However, a significant improvement can be seen over the entire MODIS tile extent.
TABLE 1. Percentage of valid pixels within the 1 km resolution disaggregated SM images using DISPATCHclassic and DISPATCHveg–ext algorithm for i) the entire MODIS tile extent H18V04, ii) the union of both semi-arid areas and iii) the temperate study area separately, for different cloud cover thresholds in 2017.
Statistical results of correlation (R), slope of the linear regression (Slope), mean bias (MB), and root mean square difference (RMSD) between disaggregated and in situ SM with DISPATCHclassic and DISPATCHveg–ext algorithm are calculated separately and presented in Table 2. The overall temporal correlation of DISPATCHveg–ext SM is in the range of 0.40–0.70, compared to 0.37–0.70 for the DISPATCHclassic case. The slight increase in the temporal correlation over vegetated areas is mainly attributed to the increase in the spatio-temporal coverage of DISPATCHveg–ext SM data set associated with the inclusion of vegetated areas. The MB and RMSD values remain approximately the same for both algorithms, with a maximum difference of 0.02 m3/m3. This indicates that the inclusion of vegetated areas in DISPATCH algorithm using the TVDI approach does not degrade the disaggregation performance. It is also observed that the slope of the linear regression between disaggregated and in situ SM is more stable for the new algorithm because of the modified calculation of temperature endmembers.
TABLE 2. Statistical results in terms of correlation (R), slope of the linear regression (Slope), mean bias (MB), RMSD between 1 km resolution disaggregated and in situ SM for (NDVI-based) DISPATCHclassic and DISPATCHveg–ext algorithm.
The robustness of the DISPATCHveg–ext algorithm over vegetated areas is further tested by using NDVI and EVI vegetation indices as input. The analysis is done by comparing the NDVI-based DISPATCHveg–ext (Table 2) and EVI-based DISPATCHveg–ext (Table 3) performance in terms of correlation (R), slope of the linear regression (Slope), mean bias (MB) and RMSD between disaggregated and in situ SM. The overall (all sites) temporal correlation of disaggregated SM vs. in situ SM ranges from 0.41 to 0.73 as compared to 0.40–0.70 for the EVI and NDVI case, respectively.
TABLE 3. Statistical results in terms of correlation (R), slope of the linear regression (Slope), mean bias (MB) and RMSD between EVI-based DISPATCHveg–ext 1 km resolution satellite product and in situ SM.
The inclusion of EVI improves the performance of the DISPATCH algorithm over the temperate and semi-arid study areas. Note that the overall percentage increase—as compared to the NDVI-based DISPATCH SM—in temporal correlation for the ICOS network (agricultural land) is 7% and for REMEDHUS and dryland networks is 1 and 4%. The increase in temporal correlation of disaggregated SM using EVI over agricultural areas is attributed to the sensitivity of EVI to
Cloud cover strongly limits the availability of optical/thermal data. The non-availability of optical/thermal data at HR is the main reason for voids in DISPATCH disaggregated SM products. The cloud cover generally differs according to the time of day. Hence, in spite of having the same spatial and temporal resolution, MODIS LST and Sentinel-3 LST data sets may be affected by clouds differently. The cloud mask applied to the MODIS LST images extracts the LST pixels with quality control (QC) equal to 0 or 17, which corresponds to an uncertainty in LST lower than 1 K and a maximum emissivity error equal to 0.01 and 0.02, respectively. The cloud mask applied to the Sentinel-3 LST images extracts the LST pixels with a QC equal to 0, which corresponds to an uncertainty in LST lower than 1 K. The Sentinel-3 LST QC does not include other criteria of data quality (Ghent, 2017). Note that in this study, only the valid SMAP pixels (with valid SMAP SM retrievals) were considered in the computation of gap percentages within the disaggregated images so that the computed data gaps correspond to the actual cloud cover on optical images, plus the SMAP pixels with a cloud cover larger than a threshold value of 50% over which disaggregation is not applied.
Figure 5 shows a qualitative analysis between MODIS-based (left) and Sentinel-3-based (right) DISPATCHveg–ext disaggregated SM images on DOY 204 for 2018 over the MODIS tile H18V04. A large data gap can be seen in the MODIS-based disaggregated SM image due to cloud cover. Most of these voids are filled by using Sentinel-3 LST in the disaggregation of SM products, thereby significantly increasing the spatial coverage of disaggregated SM. In our study, the cloud cover percentage is analyzed by calculating the valid pixels in the 1 km resolution disaggregated SM images using Sentinel-3 LST and MODIS LST as an input. Such an analysis is undertaken for data in 2018 over two distinct extents: the MODIS tile H18V04 and ICOS study area. It is observed that there is an increase in the number of valid pixels from 21% in MODIS LST disaggregated SM to 65% in Sentinel-3 LST disaggregated SM for MODIS tile H18V04 for 2018. Similarly, there is an increase in the number of valid pixels from 40% in MODIS LST disaggregated SM to 92% in Sentinel-3 LST disaggregated SM for ICOS study areas for 2018. We assume that the reason for the increase in spatial coverage is the overpass time of Sentinel-3 (9:30 am), which is earlier than MODIS Terra (10:30 am).
FIGURE 5. DISPATCHveg–ext 1 km resolution SM images for MODIS (left) and Sentinel-3 (right) LST input data on DOY 204, 2018.
Further quantitative analysis is done in order to analyze the performance of disaggregated SM using Sentinel-3 instead of MODIS LST. Figure 6 shows a scatterplot between disaggregated SM products using Sentinel-3 LST and MODIS LST as an input for Auradé (ICOS), BA (dryland) and L03 (REMEDHUS) sites separately. Both datasets are significantly correlated with an average overall correlation coefficient of about 0.7. The mean bias (MB) is very small (0.01 m3/m3) between both products, given that they both rely on the same LR SMAP observations. It is observed that the range of SM values is similar in both products so that the main difference remains the spatial coverage, which is significantly larger for Sentinel-3 LST data.
FIGURE 6. Scatterplot between Sentinel-3 and MODIS-based 1 km resolution disaggregated SM at Auradé (ICOS) site, 2018 (A); BA (dryland) site, 2019 (B) and L03 (REMEDHUS) site, 2017 (C).
Sentinel-1 radar data are not affected by clouds. The objective here is to compare the DISPATCHveg–ext and Copernicus Sentinel-1 and SMAP/S1 1 km resolution SM data sets in terms of 1) the number of valid pixels and 2) their accuracy at all the validation sites of the three study areas.
Figure 7 compares DISPATCHveg–ext disaggregated SM, Copernicus SM, and SMAP/S1 SM data on DOY 202, 2018 over the MODIS tile H18V04. It is clear from the visual comparison that, on one hand DISPATCH and Copernicus data have their spatial coverage limited due to cloud cover and Sentinel-1 field of view, respectively. On the other hand, the spatial coverage of SMAP/Sentinel-1 L2 product, which combines both SMAP and Sentinel-1 data, is determined by the field of view overlap at the concurrent overpass time of Sentinel-1 and SMAP. Tables 3–5 report the number of DISPATCH, Copernicus and SMAP/S1 observations concurrent with the in situ SM measurements collected at each monitoring station. The number of valid retrievals for DISPATCHveg-ext is 22% (50%) larger for the temperate study area and 57% (72%) larger for both semi-arid study areas as compared to Copernicus (and SMAP/S1) products, respectively.
FIGURE 7. SM images at 1 km resolution from DISPATCHveg–ext(A), Copernicus Sentinel-1 (B), SMAP/S1 (C) on DOY 204, 2018.
TABLE 4. Statistical results in terms of correlation (R), slope of the linear regression (Slope), mean bias (MB) and RMSD between Copernicus Sentinel-1 1 km resolution satellite product and in situ SM.
TABLE 5. Statistical results in terms of correlation (R), slope of the linear regression (Slope), mean bias (MB) and RMSD between SMAP/S1 1 km resolution satellite product and in situ SM.
Table 4 presents the correlation (R), slope of the linear regression (Slope), mean bias (MB) and RMSD between Copernicus Sentinel-1-based SM and in situ SM. It is reminded that for statistical comparison, Copernicus Sentinel-1 SM (%) is converted into volumetric SM (m3/m3) from Eq. 5. Statistical results in Tables 3 and 4 used to quantitatively assess the performance of DISPATCHveg–ext relative to the Copernicus SM retrieval approach. The correlation between satellite and in situ measurement is generally closer to 1 for DISPATCH than for Copernicus product ranging from 0.39 to 0.80 and from −0.03 to 0.69, respectively. The poorer statistics for the Copernicus Sentinel-1-based SM can be attributed to two factors. In temperate regions, the vegetation cover with a leaf area index larger than 0.6 is likely to drastically reduce the sensitivity of C-band backscatter to SM. In semi-arid regions, the effective soil roughness seen by active sensors has been shown to increase in dry conditions due to volume scattering (Escorihuela and Quintana-Seguí, 2016; Ojha et al., 2020), thus artificially increasing the Sentinel-1-retrieved SM. As an illustration of both possible effects, Figure 8 presents a scatterplot of DISPATCHveg–ext vs. in situ SM and of Copernicus vs. in situ SM for Auradé (ICOS), HA1 (dryland) and L03 (REMEDHUS) sites separately. In particular, the bi-modal behavior of the Sentinel-1-retrieved SM can be attributed to volume scattering in very dry conditions. It can be the reason for the negative correlation and slope of Copernicus Sentinel-1 based SM for HA1 site. The limitation of the bi-modal behavior of Copernicus Sentinel-1 based SM for dryland areas is overcome by DISPATCHveg–ext algorithm and exhibits a better representation of SM at 1 km resolution.
FIGURE 8. Scatterplot of Copernicus Sentinel-1 vs. in situ SM (left) and DISPATCHveg–ext vs. in situ SM (right) for Auradé (ICOS) site, 2018 (A,B); HA1 (dryland) site, 2019 (C,D) and L03 (REMEDHUS) site, 2017 (E,F).
The performance of optical-based (DISPATCH) and radar-based (SMAP/S1) SMAP disaggregated SM products is assessed by the statistical comparison presented in Tables 3 and 5. The correlation between satellite and in situ measurements is in the range of 0.39–0.80 and 0.22–0.82 for DISPATCH and SMAP-S1 product, respectively. The overall statistical difference between SMAP/S1 and DISPATCH 1 km disaggregated products is relatively small for all three study areas. DISPATCH and SMAP/S1 perform well over the dryland semi-arid sites with a mean correlation larger than 0.7 with however, a significant negative bias (−0.04 m3/m3) on SMAP/S1. The statistical metrics for SMAP/S1 are slightly poorer as compared to DISPATCH in the temperate study area. This could be explained by a lower sensitivity to SM of SMAP/S1 product over vegetated areas where the vegetation water content is greater than 3 kg/m2 (Das et al., 2019). Nonetheless, the performance of both disaggregated products over all three study areas remains rather similar and the main advantage of DISPATCH compared to the official SMAP product is the larger spatio-temporal coverage. SMAP/S1 1 km product is limited by the temporal frequency of Sentinel-1 with an interval of 6 days in Europe.
DISPATCH is a well-known optical/thermal-based disaggregation method of passive microwave-derived SM data. It is usually implemented using MODIS LST/NDVI and SMOS/SMAP SM data to provide 1 km resolution disaggregated SM images. The application of DISPATCH to large areas at high frequency is however limited by 1) the loss of sensitivity of LST to the surface SM over densely vegetated areas and 2) the unavailability of optical data under cloud cover. To improve the spatio-temporal coverage of 1 km resolution DISPATCH SM data, a new algorithm named DISPATCHveg–ext algorithm is proposed. DISPATCHveg–ext differs from DISPATCHclassic in mainly one aspect: the use of TVDI in the DISPATCH downscaling relationship to apply the disaggregation to densely vegetated areas. Moreover, DISPATCHveg–ext is tested using Sentinel-3 LST instead of MODIS LST as input, in order to assess the impact of the thermal observation time on the output data gaps due to cloud cover. This approach is evaluated by comparing the disaggregated SM with in situ measurements over a temperate and two semi-arid regions.
First, the comparison is done between DISPATCHclassic and DISPATCHveg–ext disaggregated SM at 1 km resolution. Visual analysis indicates a significant increase in the spatial coverage of DISPATCHveg–ext disaggregated SM images due to the inclusion of densely vegetated areas. In addition, the temporal correlation between satellite and in situ SM is increased by 9% and the RMSD is decreased by 6% in the temperate region. Similarly, for the semi-arid regions, the temporal correlation is increased by 7–8% and the RMSD is decreased by 6–18%. Furthermore, the use of EVI instead of NDVI improves the robustness of the disaggregated SM over vegetated areas by increasing the correlation by 7% over the temperate region and by up to 4% over the semi-arid regions.
Second, the use of Sentinel-3 LST (09:30 am overpass) in place of MODIS LST (10:30 am and 1:30 pm overpass) to disaggregate SM at 1 km resolution very significantly increases the spatial coverage of disaggregated SM at 1 km resolution. Both MODIS- and Sentinel-3-based disaggregated SM data sets are found to be significantly correlated. However, as a caveat, one should keep in mind that the overpass time of thermal data is a compromise between 1) the overall cloud cover, which may be less early in the morning but also 2) the coupling between LST and SM, which is stronger at solar noon. Instead of polar orbit satellites, geostationnary satellites have been also used to downscale SMOS SM data (Piles et al., 2016; Tagesson et al., 2018). The point is that the currently available geostationary thermal sensors have a spatial resolution of about 2–3 km at nadir, corresponding to a resolution of 4–5 km at the latitudes of our study areas. In the future, the possible advent of high-resolution geostationary thermal infrared satellites will be very useful in DISPATCH to significantly reduce the gaps in disaggregated SM images due to cloud cover.
Third, the accuracy of DISPATCHveg–ext is evaluated by comparison with Copernicus Sentinel-1 SM and SMAP/S1 SM products separately, which both have the same (1 km) spatial resolution. DISPATCHveg–ext is generally more accurate than the Copernicus product and its spatio-temporal coverage is significantly larger than that of SMAP/S1 product. Such a comparison opens up a new research towards the development of synergies between thermal-based (DISPATCH) and Sentinel-1-based SM (Amazirh et al., 2019). The high spatio-temporal resolution together with the all-weather capability of Sentinel-1 data are essential assets for that purpose. In particular, the SMOS/SMAP SM can be disaggregated at 100 m resolution using DISPATCH and Landsat thermal data (Ojha et al., 2019). At such high spatial resolution however, the revisit time of current thermal sensors is relatively long (16 days for Landsat). Therefore, the synergy between DISPATCH and Sentinel-1 data is expected to be very useful at high spatial resolution, especially before the advent of future thermal missions like TRISHNA (Lagouarde et al., 2019) and LSTM (Koetz et al., 2018).
The original contributions presented in the study are included in the article/Supplementary Material, further inquiries can be directed to the corresponding author.
NO: implementation, validation, investigation, data curation and writing original draft of the paper; OM: supervision, reviewing and editing; CS and ME: reviewing and editing.
This study was supported by the European Commission Horizon 2020 Program for Research and Innovation (H2020) in the context of the Marie Sklodowska-Curie Research and Innovation Staff Exchange 445 (RISE) action (ACCWA project, Grant Agreement No.: 823965), and by the Partnership for Research and 446 Innovation in the Mediterranean Area (PRIMA) IDEWA project.
Author ME is employed by the company isardSAT.
The remaining authors declare that the research was conducted in the absence of any commercial or financial relationships that could be construed as a potential conflict of interest.
Soil Moisture measurements at Lamasquère (FR-Lam) and Auradé (FR-Aur) were mainly funded by ICOS ERIC. These experimental sites also benefited from the support and facilities of the Regional Spatial Observatory (OSR), CNRS (Centre National de la Recherche Scientifique), CNES (Centre National d’Etudes Spatiales), University of Toulouse. The author also thank International soil moisture network (ISMN) for providing in situ soil moisture measurements for REMEDHUS network and Pere Quintana Segui (Observatori de l’Ebre, URL-CSIC) for providing in situ soil moisture measurements for dryland areas of Spain.
Albergel, C., Rüdiger, C., Pellarin, T., Calvet, J.-C., Fritz, N., Froissard, F., et al. (2008). From near-surface to root-zone soil moisture using an exponential filter: an assessment of the method based on in-situ observations and model simulations. Hydrol. Earth Syst. Sci. 12, 1323–1337. doi:10.5194/hess-12-1323-2008
Amazirh, A., Merlin, O., and Er-Raki, S. (2019). Including sentinel-1 radar data to improve the disaggregation of modis land surface temperature data. ISPRS J. Photogramm. Remote Sens. 150, 11–26. doi:10.1016/j.isprsjprs.2019.02.004
Atwood, D. K., Andersen, H.-E., Matthiss, B., and Holecz, F. (2014). Impact of topographic correction on estimation of aboveground boreal biomass using multi-temporal, l-band backscatter. IEEE J. Sel. Top. Appl. Earth Obs. Remote Sens. 7, 3262–3273. doi:10.1109/jstars.2013.2289936
Bauer-Marschallinger, B., Freeman, V., Cao, S., Paulik, C., Schaufler, S., Stachl, T., et al. (2018). Toward global soil moisture monitoring with sentinel-1: harnessing assets and overcoming obstacles. IEEE Trans. Geosci. Remote Sens. 57, 520–539. doi:10.1109/TGRS.2018.2858004
Béziat, P., Ceschia, E., and Dedieu, G. (2009). Carbon balance of a three crop succession over two cropland sites in south west France. Agric. For. Meteorol. 149, 1628–1645. doi:10.1016/j.agrformet.2009.05.004
Brisson, N., and Perrier, A. (1991). A semiempirical model of bare soil evaporation for crop simulation models. Water Resour. Res. 27, 719–727. doi:10.1029/91wr00075
Carlson, T. (2007). An overview of the “triangle method” for estimating surface evapotranspiration and soil moisture from satellite imagery. Sensors 7, 1612–1629. doi:10.3390/s7081612
Chen, F., Crow, W. T., Starks, P. J., and Moriasi, D. N. (2011). Improving hydrologic predictions of a catchment model via assimilation of surface soil moisture. Adv. Water Resour. 34, 526–536. doi:10.1016/j.advwatres.2011.01.011
Cleugh, H. A., Leuning, R., Mu, Q., and Running, S. W. (2007). Regional evaporation estimates from flux tower and modis satellite data. Remote Sens. Environ. 106, 285–304. doi:10.1016/j.rse.2006.07.007
Colliander, A., Fisher, J. B., Halverson, G., Merlin, O., Misra, S., Bindlish, R., et al. (2017). Spatial downscaling of smap soil moisture using modis land surface temperature and ndvi during smapvex15. IEEE Geosci. Remote Sens. Lett. 14, 2107–2111. doi:10.1109/lgrs.2017.2753203
Cosby, B., Hornberger, G. M., Clapp, R. B., and Ginn, T. R. (1984). A statistical exploration of the relationships of soil moisture characteristics to the physical properties of soils. Water Resour. Res. 20, 682–690. doi:10.1029/wr020i006p00682
Das, N. N., Entekhabi, D., Dunbar, R. S., Colliander, A., Chen, F., Crow, W., et al. (2018). The smap mission combined active-passive soil moisture product at 9 km and 3 km spatial resolutions. Remote Sens. Environ. 211, 204–217. doi:10.1016/j.rse.2018.04.011
Das, N. N., Entekhabi, D., Dunbar, S., Chaubell, M., Jagdhuber, T., Colliander, A., et al. (2019). The SMAP and copernicus sentinel 1A/B microwave active-passive high resolution surface soil moisture product and its applications.
Das, N. N., Entekhabi, D., and Njoku, E. G. (2010). An algorithm for merging smap radiometer and radar data for high-resolution soil-moisture retrieval. IEEE Trans. Geosci. Remote Sens. 49 (5), 1504–1512. doi:10.1109/TGRS.2010.2089526
Dirmeyer, P. A. (2000). Using a global soil wetness dataset to improve seasonal climate simulation. J. Clim. 13, 2900–2922. doi:10.1175/1520-0442(2000)013<2900:uagswd>2.0.co;2
Dorigo, W., Wagner, W., Hohensinn, R., Hahn, S., Paulik, C., Xaver, A., et al. (2011). International soil moisture network: a data hosting facility for global in situ soil moisture measurements. Hydrol. Earth Syst. Sci. 15, 1675–1698. doi:10.5194/hess-15-1675-2011
Douville, H. (2004). Relevance of soil moisture for seasonal atmospheric predictions: is it an initial value problem?. Clim. Dyn. 22, 429–446. doi:10.1007/s00382-003-0386-5
Entekhabi, D., Njoku, E. G., O’Neill, P. E., Kellogg, K. H., Crow, W. T., Edelstein, W. N., et al. (2010). The soil moisture active passive (SMAP) mission. Proc. IEEE 98, 704–716. doi:10.1109/jproc.2010.2043918
Escorihuela, M. J., and Quintana-Seguí, P. (2016). Comparison of remote sensing and simulated soil moisture datasets in mediterranean landscapes. Remote Sens. Environ. 180, 99–114. doi:10.1016/j.rse.2016.02.046
Ford, T., Harris, E., and Quiring, S. (2013). Estimating root zone soil moisture using near-surface observations from SMOS. Hydrol. Earth Syst. Sci. Discuss. 10, 8325–8364. doi:10.5194/hessd-10-8325-2013
Gao, B.-C. (1996). NDWI-a normalized difference water index for remote sensing of vegetation liquid water from space. Remote Sens. Environ. 58, 257–266. doi:10.1016/s0034-4257(96)00067-3
Georgiana Stefan, V., Escorihuela, M. J., Merlin, O., Chihrane, J., Ghaout, S., and Piou, C. (2018). “Using Sentinel-3 land surface temperature to derive high resolution soil moisture estimates for desert locust management,” in EGU general assembly conference abstracts, Vienna, Austria, April 8–13, 2018 (Vienna, Austria: AGU), Vol. 20, 13752.
Ghent, D. (2017). S3 validation report–slstr, s3mpc.uol.vr.029-i1r0-slstr l2 land validation report.docx. Available at: https://sentinel.esa.int/web/sentinel/technical-guides/sentinel-3-slstr/cal-val-activities/validation.
Gitelson, A. A. (2004). Wide dynamic range vegetation index for remote quantification of biophysical characteristics of vegetation. J. Plant Physiol. 161, 165–173. doi:10.1078/0176-1617-01176
Guérif, M., and Duke, C. L. (2000). Adjustment procedures of a crop model to the site specific characteristics of soil and crop using remote sensing data assimilation. Agric. Ecosyst. Environ. 81, 57–69. doi:10.1016/s0167-8809(00)00168-7
Gutman, G., and Ignatov, A. (1998). The derivation of the green vegetation fraction from NOAA/AVHRR data for use in numerical weather prediction models. Int. J. Remote Sens. 19, 1533–1543. doi:10.1080/014311698215333
Hamlet, A. F., Mote, P. W., Clark, M. P., and Lettenmaier, D. P. (2007). Twentieth-century trends in runoff, evapotranspiration, and soil moisture in the western United States. J. Clim. 20, 1468–1486. doi:10.1175/jcli4051.1
Huete, A., Didan, K., Miura, T., Rodriguez, E. P., Gao, X., and Ferreira, L. G. (2002). Overview of the radiometric and biophysical performance of the modis vegetation indices. Remote Sens. Environ. 83, 195–213. doi:10.1016/s0034-4257(02)00096-2
Jagdhuber, T., Entekhabi, D., Das, N. N., Link, M., Montzka, C., Kim, S., et al. (2017). “Microwave covariation modeling and retrieval for the dual-frequency active-passive combination of sentinel-1 and SMAP,” in 2017 IEEE international geoscience and remote sensing symposium (IGARSS), Fort Worth, TX, July 23–28, 2017 (IEEE), 3996–3999. doi:10.1109/IGARSS.2017.8127876
Jiang, Z., Huete, A. R., Li, J., and Chen, Y. (2006). An analysis of angle-based with ratio-based vegetation indices. IEEE Trans. Geosci. Remote Sens. 44, 2506–2513. doi:10.1109/tgrs.2006.873205
Kerr, Y. H., Waldteufel, P., Richaume, P., Wigneron, J. P., Ferrazzoli, P., Mahmoodi, A., et al. (2012). The SMOS soil moisture retrieval algorithm. IEEE Trans. Geosci. Remote Sens. 50, 1384–1403. doi:10.1109/tgrs.2012.2184548
Kim, J., and Hogue, T. S. (2012). Evaluation and sensitivity testing of a coupled landsat-modis downscaling method for land surface temperature and vegetation indices in semi-arid regions. J. Appl. Remote Sens. 6, 063569. doi:10.1117/1.jrs.6.063569
Koetz, B., Bastiaanssen, W., Berger, M., Defourney, P., Del Bello, U., Drusch, M., et al. (2018). “High spatio-temporal resolution land surface temperature mission-a copernicus candidate mission in support of agricultural monitoring,” in IGARSS 2018-2018 IEEE international geoscience and remote sensing symposium, Valencia, Spain, July 22–27, 2018 (IEEE), 8160–8162. doi:10.1109/IGARSS.2018.8517433
Kogan, F. N. (1995). Application of vegetation index and brightness temperature for drought detection. Adv. Space Res. 15, 91–100. doi:10.1016/0273-1177(95)00079-t
Kumar, S. V., Reichle, R. H., Koster, R. D., Crow, W. T., and Peters-Lidard, C. D. (2009). Role of subsurface physics in the assimilation of surface soil moisture observations. J. Hydrometeorol. 10, 1534–1547. doi:10.1175/2009jhm1134.1
Lagouarde, J.-P., Bhattacharya, B., Crébassol, P., Gamet, P., Adlakha, D., Murthy, C., et al. (2019). “Indo-French high-resolution thermal infrared space mission for earth natural resources assessment and monitoring-concept and definition of trishna,” in ISPRS-GEOGLAM-ISRS joint international workshop on “earth observations for agricultural monitoring” New Delhi, India, Vol. 42, 403.
Lievens, H., Reichle, R. H., Liu, Q., De Lannoy, G. J. M., Dunbar, R. S., Kim, S. B., et al. (2017). Joint sentinel-1 and SMAP data assimilation to improve soil moisture estimates. Geophys. Res. Lett. 44, 6145–6153. doi:10.1002/2017gl073904
Lievens, H., Tomer, S. K., Al Bitar, A., De Lannoy, G. J. M., Drusch, M., Dumedah, G., et al. (2015). SMOS soil moisture assimilation for improved hydrologic simulation in the murray darling basin, Australia. Remote Sens. Environ. 168, 146–162. doi:10.1016/j.rse.2015.06.025
Liu, H. Q., and Huete, A. (1995). A feedback based modification of the NDVI to minimize canopy background and atmospheric noise. IEEE Trans. Geosci. Remote Sens. 33, 457–465. doi:10.1109/tgrs.1995.8746027
Malbéteau, Y., Merlin, O., Molero, B., Rüdiger, C., and Bacon, S. (2016). Dispatch as a tool to evaluate coarse-scale remotely sensed soil moisture using localized in situ measurements: application to SMOS and AMSR-E data in southeastern Australia. Int. J. Appl. Earth Obs. Geoinf. 45, 221–234. doi:10.1016/j.jag.2015.10.002
Matsushita, B., Yang, W., Chen, J., Onda, Y., and Qiu, G. (2007). Sensitivity of the enhanced vegetation index (EVI) and normalized difference vegetation index (NDVI) to topographic effects: a case study in high-density cypress forest. Sensors 7, 2636–2651. doi:10.3390/s7112636
Merlin, O., Chehbouni, A. G., Kerr, Y. H., Njoku, E. G., and Entekhabi, D. (2005). A combined modeling and multispectral/multiresolution remote sensing approach for disaggregation of surface soil moisture: application to SMOS configuration. IEEE Trans. Geosci. Remote Sens. 43, 2036–2050. doi:10.1109/tgrs.2005.853192
Merlin, O., Escorihuela, M. J., Mayoral, M. A., Hagolle, O., Al Bitar, A., and Kerr, Y. (2013). Self-calibrated evaporation-based disaggregation of SMOS soil moisture: an evaluation study at 3km and 100m resolution in Catalunya, Spain. Remote Sens. Environ. 130, 25–38. doi:10.1016/j.rse.2012.11.008
Merlin, O., Rudiger, C., Al Bitar, A., Richaume, P., Walker, J. P., and Kerr, Y. H. (2012). Disaggregation of SMOS soil moisture in southeastern Australia. IEEE Trans. Geosci. Remote Sens. 50, 1556–1571. doi:10.1109/tgrs.2011.2175000
Merlin, O., Stefan, V. G., Amazirh, A., Chanzy, A., Ceschia, E., Er-Raki, S., et al. (2016). Modeling soil evaporation efficiency in a range of soil and atmospheric conditions using a meta-analysis approach. Water Resour. Res. 52, 3663–3684. doi:10.1002/2015wr018233
Mishra, V., Ellenburg, W. L., Griffin, R. E., Mecikalski, J. R., Cruise, J. F., Hain, C. R., et al. (2018). An initial assessment of a smap soil moisture disaggregation scheme using tir surface evaporation data over the continental United States. Int. J. Appl. Earth Obs. Geoinform. 68, 92–104. doi:10.1016/j.jag.2018.02.005
Molero, B., Merlin, O., Malbéteau, Y., Al Bitar, A., Cabot, F., Stefan, V., et al. (2016). SMOS disaggregated soil moisture product at 1 km resolution: processor overview and first validation results. Remote Sens. Environ. 180, 361–376. doi:10.1016/j.rse.2016.02.045
Moran, M., Clarke, T., Inoue, Y., and Vidal, A. (1994). Estimating crop water deficit using the relation between surface-air temperature and spectral vegetation index. Remote Sens. Environ. 49, 246–263. doi:10.1016/0034-4257(94)90020-5
Mu, Q., Heinsch, F. A., Zhao, M., and Running, S. W. (2007). Development of a global evapotranspiration algorithm based on MODIS and global meteorology data. Remote Sens. Environ. 111, 519–536. doi:10.1016/j.rse.2007.04.015
Narayan, U., Lakshmi, V., and Jackson, T. J. (2006). High-resolution change estimation of soil moisture using l-band radiometer and radar observations made during the smex02 experiments. IEEE Trans. Geosci. Remote Sens. 44, 1545–1554. doi:10.1109/tgrs.2006.871199
Ojha, N., Merlin, O., and Escorihuela, M. J. (2020). “Towards synergies between thermal-disaggregated and Sentinel1-based soil moisture data sets,” in 2020 Mediterranean and Middle-East geoscience and remote sensing symposium (M2GARSS), Tunis, Tunisia, March 9–11, 2020 (IEEE).
Ojha, N., Merlin, O., Molero, B., Suere, C., Olivera-Guerra, L., Ait Hssaine, B., et al. (2019). Stepwise disaggregation of SMAP soil moisture at 100 m resolution using landsat-7/8 data and a varying intermediate resolution. Remote Sens. 11, 1863. doi:10.3390/rs11161863
O’Neill, P., Chan, S., Njoku, E., Jackson, T., and Bindlish, R. (2018). Algorithm theoretical basis document level 2 & 3 soil moisture (passive) data products; revision b. Pasadena, CA: Jet Propulsion Lab., California Institute of Technology.
Peng, J., Loew, A., Zhang, S., Wang, J., and Niesel, J. (2015a). Spatial downscaling of satellite soil moisture data using a vegetation temperature condition index. IEEE Trans. Geosci. Remote Sens. 54, 558–566. doi:10.1109/TGRS.2015.2462074
Peng, J., Niesel, J., and Loew, A. (2015b). Evaluation of soil moisture downscaling using a simple thermal-based proxy–the REMEDHUS network (Spain) example. Hydrol. Earth Syst. Sci. 19, 4765–4782. doi:10.5194/hess-19-4765-2015
Piles, M., Entekhabi, D., and Camps, A. (2009). A change detection algorithm for retrieving high-resolution soil moisture from SMAP radar and radiometer observations. IEEE Trans. Geosci. Remote Sens. 47, 4125–4131. doi:10.1109/tgrs.2009.2022088
Piles, M., Petropoulos, G. P., Sánchez, N., González-Zamora, Á., and Ireland, G. (2016). Towards improved spatio-temporal resolution soil moisture retrievals from the synergy of SMOS and MSG SEVIRI spaceborne observations. Remote Sens. Environ. 180, 403–417. doi:10.1016/j.rse.2016.02.048
Sandholt, I., Rasmussen, K., and Andersen, J. (2002). A simple interpretation of the surface temperature/vegetation index space for assessment of surface moisture status. Remote Sens. Environ. 79, 213–224. doi:10.1016/s0034-4257(01)00274-7
Sobrino, J. A., Jimenez-Munoz, J. C., Soria, G., Brockmann, C., Ruescas, A., Danne, O., et al. (2015). “A prototype algorithm for land surface temperature retrieval from Sentinel-3 mission,” in Sentinel-3 for science workshop Venice, Italy, Vol. 734, 38.
Stefan, V. G., Merlin, O., Er-Raki, S., Escorihuela, M.-J., and Khabba, S. (2015). Consistency between in situ, model-derived and high-resolution-image-based soil temperature endmembers: towards a robust data-based model for multi-resolution monitoring of crop evapotranspiration. Remote Sens. 7, 10444–10479. doi:10.3390/rs70810444
Tagesson, T., Horion, S., Nieto, H., Zaldo Fornies, V., Mendiguren González, G., Bulgin, C. E., et al. (2018). Disaggregation of SMOS soil moisture over West Africa using the temperature and vegetation dryness index based on SEVIRI land surface parameters. Remote Sens. Environ. 206, 424–441. doi:10.1016/j.rse.2017.12.036
Tallec, T., Béziat, P., Jarosz, N., Rivalland, V., and Ceschia, E. (2013). Crops’ water use efficiencies in temperate climate: comparison of stand, ecosystem and agronomical approaches. Agric. For. Meteorol. 168, 69–81. doi:10.1016/j.agrformet.2012.07.008
Verhoest, N. E., Lievens, H., Wagner, W., Álvarez-Mozos, J., Moran, M., and Mattia, F. (2008). On the soil roughness parameterization problem in soil moisture retrieval of bare surfaces from synthetic aperture radar. Sensors 8, 4213–4248. doi:10.3390/s8074213
Waite, W. P., and MacDonald, H. C. (1971). “Vegetation penetration” with K-band imaging radars. IEEE Trans. Geosci. Electron. 9, 147–155. doi:10.1109/tge.1971.271487
Wanders, N., Bierkens, M. F., de Jong, S. M., de Roo, A., and Karssenberg, D. (2014). The benefits of using remotely sensed soil moisture in parameter identification of large-scale hydrological models. Water Resour. Res. 50, 6874–6891. doi:10.1002/2013wr014639
Wegmuller, U., and Werner, C. (1997). Retrieval of vegetation parameters with SAR interferometry. IEEE Trans. Geosci. Remote Sens. 35, 18–24. doi:10.1109/36.551930
Wigneron, J.-P., Jackson, T., O’Neill, P., De Lannoy, G., De Rosnay, P., Walker, J., et al. (2017). Modelling the passive microwave signature from land surfaces: a review of recent results and application to the L-band SMOS & SMAP soil moisture retrieval algorithms. Remote Sens. Environ. 192, 238–262. doi:10.1016/j.rse.2017.01.024
Yang, Y., Guan, H., Long, D., Liu, B., Qin, G., Qin, J., et al. (2015). Estimation of surface soil moisture from thermal infrared remote sensing using an improved trapezoid method. Remote Sens. 7, 8250–8270. doi:10.3390/rs70708250
Keywords: soil moisture, DISPATCH, TVDI, EVI, SMAP, Sentinel-3
Citation: Ojha N, Merlin O, Suere C and Escorihuela MJ (2021) Extending the Spatio-Temporal Applicability of DISPATCH Soil Moisture Downscaling Algorithm: A Study Case Using SMAP, MODIS and Sentinel-3 Data. Front. Environ. Sci. 9:555216. doi: 10.3389/fenvs.2021.555216
Received: 24 April 2020; Accepted: 01 February 2021;
Published: 16 March 2021.
Edited by:
Zheng Duan, Lund University, SwedenReviewed by:
Lingmei Jiang, Beijing Normal University, ChinaCopyright © 2021 Ojha, Merlin, Suere and Escorihuela. This is an open-access article distributed under the terms of the Creative Commons Attribution License (CC BY). The use, distribution or reproduction in other forums is permitted, provided the original author(s) and the copyright owner(s) are credited and that the original publication in this journal is cited, in accordance with accepted academic practice. No use, distribution or reproduction is permitted which does not comply with these terms.
*Correspondence: Nitu Ojha, b2poYW5AY2VzYmlvLmNuZXMuZnI=
Disclaimer: All claims expressed in this article are solely those of the authors and do not necessarily represent those of their affiliated organizations, or those of the publisher, the editors and the reviewers. Any product that may be evaluated in this article or claim that may be made by its manufacturer is not guaranteed or endorsed by the publisher.
Research integrity at Frontiers
Learn more about the work of our research integrity team to safeguard the quality of each article we publish.