- 1Department of Electronics and Communication Engineering, Faculty of Engineering and Technology, SRM Institute of Science and Technology, Delhi- NCR Campus, Ghaziabad, India
- 2Department of Computer Science and Engineering, Gandhi Institute of Engineering and Technology University, Gunupur, India
- 3School of Computer Engineering, Kalinga Institute of Industrial Technology Deemed to Be University, Bhubaneswar, India
- 4Department of Civil, Environmental and Natural Resources Engineering, Lulea University of Technology, Lulea, Sweden
- 5Department of Electronics and Communication Engineering, Krishna Institute of Engineering and Technology, Group of Institutions, Ghaziabad, India
- 6Department of Computer Science, SRM Institute of Science and Technology, NCR Campus, Ghaziabad, India
- 7Department of Applied Sciences and Humanities, Teerthankar Mahaveer University, Moradabad, India
- 8Institute of Research and Development, Duy Tan University, Da Nang, Vietnam
- 9Geotechnical Engineering and Artificial Intelligence Research Group (GEOAI), University of Transport Technology, Hanoi, Vietnam
The Yamuna river has become one of the most polluted rivers in India as well as in the world because of the high-density population growth and speedy industrialization. The Yamuna river is severely polluted and needs urgent revival. The Yamuna river in Dehradun is polluted due to exceptional tourist activity, poor sewage facilities, and insufficient wastewater management amenities. The measurement of the quality can be done by water quality assessment. In this study, the water quality index has been calculated for the Yamuna river at Dehradun using monthly measurements of 12 physicochemical parameters. Trend forecasting for river water pollution has been performed using different parameters for the years 2020–2024 at Dehradun. The study shows that the values of four parameters namely, Temperature, Total Coliform, TDS, and Hardness are increasing yearly, whereas the values of pH and DO are not rising heavily. The considered physicochemical parameters for the study are TDS, Chlorides, Alkalinity, DO, Temperature, COD, BOD, pH, Magnesium, Hardness, Total Coliform, and Calcium. As per the results and trend analysis, the value of total coliform, temperature, and hardness are rising year by year, which is a matter of concern. The values of the considered physicochemical parameters have been monitored using various monitoring stations installed by the Central Pollution Control Board (CPCB), India.
Introduction
Due to historical, geographical, religious, political, and sociocultural reasons, India has a unique place in the world Agarwal et al., 2016. Pollution-causing activities have caused severe changes in aquatic environments over the last few decades. Serious questions have been raised in context to the safe use of river water for drinking and other purposes in recent times. Numerous contaminants are playing a major role in polluting the river water. It is one of the main concerns for most of the metropolitan cities of developing nations. Rivers play a vital role in shaping up the natural, cultural, and economic aspects of any country (Rafiq, 2016). The Yamuna river is one such river. The Yamuna river provides sustenance to ecology and is therefore considered holy by the people of India. It derives from the glacier called Yamunotri in the Himalayan ranges. States through which the Yamuna river flows are the Uttarakhand, Himachal Pradesh, Uttar Pradesh, Haryana, and Delhi. The Yamuna river is also divided into several tributaries such as the Hindon, Tons, Giri, Rishiganga, Hanuman Ganga, Sasur Khaderi, Chambal, Betwa, Ken, Sindh, and Baghain as it is flowing through several cities. These cities are the Yamuna Nagar, Delhi, Faridabad, Mathura, Agra, Etawah, and Prayagraj. It is a tributary of the river Ganges in India. Two of them together have had substantial importance in shaping up the history and geography of our country. The river on which our research primarily focuses is the Yamuna river. It passes through several states such as Uttar Pradesh, Himachal Pradesh, Uttarakhand, Haryana, and Delhi. It has a length of approximately 1,380 km. More than 600 lakh people are dependent on their living and income on this river (Census Reports of India 2001, 1971–1991). Such is the greatness of this river. Our research is based on the Yamuna river in Dehradun in Uttarakhand.
The process, in which the people from rustic areas shift to the town areas in search of a brighter future, thus resulting in a drastic increment in the population of people living in cities, is called urbanization. As a result, the number of cities and towns increases exponentially. There is an atrocious amount of stress on the weakening natural resources. As it is, the natural resources are facing major deterioration issues considering the unthoughtful plundering by the people. In the last few decades, the rate of spread in various segments of the world has been unprecedented and unimaginable. The proportion of the rate of infrastructure expansion has not been able to match up to the pace of urbanization in most cities. The amplified requirement of water, deficiency of sewage facilities, and scarce wastewater treatment facilities rigorously affect the water resources, and change the environment and ecology. Agricultural lands, rural unpaved areas, and natural wetlands are converted into paved and impervious urban areas, during urbanization. Augmented impervious land surface in urbanized areas leads to severe and radical changes in the natural order of things (Ahmad et al., 2017). There has been a drastic decline in the Yamuna river water quality since the last few years. The water is highly polluted, and it is a joint responsibility of the government and all the citizens to make sure that the Yamuna river is clean again. The primary step toward understanding and deliberating about the sorts of water pollution and developing effective reduction strategies is monitoring (Marale, 2012). Physical, chemical, and biological compositions determine the quality of water (Allee and Johnson, 1999). The substances such as heavy metals, pesticides, detergents, and petroleum form the chemical composition (Tiwari et al., 2020). Turbidity, color, and temperature comprise the physical composition, whereas the biological arrangement includes pigments and planktons. Observation and analysis of these water quality parameters need sampling from extensively distributed locations, which is time consuming and requires a lot of field and lab efforts to come up with statistical results (Wang et al., 2004; Icaga, 2007; Kazi et al., 2009; Amandeep, 2011; Duong, 2012; Singh et al., 2013; Nazeer and Nichol, 2015; Shi et al., 2018).
Conventionally, monitoring-based methods are used to find out the water quality parameters. They involve wide-ranging field sampling and expensive lab analysis, which is time inefficient and can only be accomplished for areas that are smaller (Song et al., 2012). Hence, these restraints and drawbacks make the conventional methods challenging for continuous water quality prediction at spatial scales (Panwar et al., 2015; Chabuk et al., 2017). For observing and analyzing water quality parameters, such as turbidity, chlorophyll, temperature, and suspended inorganic materials, techniques, such as optical remote sensing, are being used (Pattiaratchi et al., 1994; Fraser, 1998; Kondratyev et al., 1998). To calculate the measure of solar irradiance at varied wavelength bands reflected by the surface water, remote sensing satellite sensors are used (Zhang et al., 2003; Dwivedi and Pathak, 2007; Girgin et al., 2010; Ronghang et al., 2019). Amplified demand for water, poor sewage facility, and insufficient wastewater management amenities, relentlessly affect the resources of water resources. Models such as hydrological models have been used to evaluate the effect of numerous factors in rain-related procedures of the cosmopolitan areas (Trombadore et al., 2020). Knowledge and information about interconnections between climate, population, and ecology are essential for understanding and promoting sustainable development (Sharma et al., 2020). It also requires better knowledge of equipment and methodical planning. Proper management will reduce the degradation of rivers (Shukla et al., 2018). In this study, we focus on trying to find out contaminants in the river, finding the water pollution index, and subsequently enforcing measures to curb water pollution.
Contribution of the Study:
1. In the present study, water samples were collected every year from the Yamuna river canal in Dehradun, Uttarakhand, India.
2. The samples have been analyzed for 12 different physicochemical attributes like ph, BOD, COD, Total Coliform, Temp, DO, Alkalinity, Chlorides, Calcium, Magnesium, and Hardness as Calcium Carbonate and TDS.
3. The measurement of the water quality index has been taken into consideration for the years 2017, 2018, and 2019.
4. Forecasting the pollution trend for the Yamuna river water from 2016 to 2024.
Materials and Methods
Mathematical Model
In this research paper, the water sample of the Yamuna river is considered for analysis. The 12 physicochemical parameters in the water are studied and analyzed. The water sample of the Holy River called the Yamuna river is considered for a certain period. The ratio of water components mainly Temperature, Total Coliform, TDS, and Hardness are varied irregularly at various locations of India. Due to the abrupt changes in the water component, the water quality is also changed. In this research paper, a sampling distribution-based analytical model called Equipoise Evaluator (EE) is proposed for the discrete parameter value of the water components. The EE model is suitable to analyze random discrete parameters. The EE model can be applied for any kind of sample analysis where the analysis is based on sample molecules. To analyze the discrete sample in the form of the symmetric normal distribution for a particular location, the EE model is applied. In this research paper, the water sample varies based on the molecules of e water components. This EE model is also applicable for the analysis of metallurgy to detect the impurity of the metal. In this research paper, the EE model is deployed for the water sample of the Yamuna river.
Sampling distribution is proposed to transform the variable at different levels.
As per the linear transformation
Now, by applying the Jacobian transformation on a non-singular matrix M,
From Equation (2), the relational equation for all connected differential elements is defined as Equation (3)
As M is considered as an orthogonal matrix, hence Z = MW, which transformed into a quadratic form of preserving from the standard value.
To determine the dissimilarity distance from a standard sample value, the partitioning matrix is deployed.
Assume that matrix M is partitioned into qth numbers, then .
As per the partitioning matrix, all q sub-matrices are orthogonal to each other except orthogonal themselves. Now, Equation (1) is rewritten as
where, M1,…., Mq are an exclusive subset of the tested variables.
Applying transformation in Equation (7)
where,
Equation (8) determines the transformation of each partition into quadratic form with exclusive subsets of tested parameters. In this analysis, M is considered as fully orthogonal, with each row orthogonal to every other row. The result of transforming all the variables to test bed data variables of D is then,
It is considered that the water molecules of the sample water have symmetric normally distributed for a particular location. The mean of the water molecules is .
As per orthogonal transformation
where and .
u1 and σ are independently distributed. The sample mean and sample variance of the experimented sample water are independently distributed.
Water Quality Index and Trend Analysis
The primary focus of this study is to measure and analyze the drastic changes in the Yamuna river water quality at Dehradun, Uttarakhand. Standardized and the universally accepted water quality index (WQI) has been adopted to measure the variation in water quality of the Yamuna river at the prime location of the study—Dehradun over 3 years. The standard method has been used to examine and evaluate the water quality for 12 Physicochemical parameters (TDS, Chlorides, Alkalinity, DO, Temperature, COD, BOD, pH, Magnesium, Hardness, Total Coliform, and Calcium). In this study, the water quality index has been calculated using the different Physicochemical parameters documented and verified from the monitored locations. The water quality index (WQI) is stated by
where Ii signifies the ith water quality parameters, the weight associated and related to the parameters is denoted by Wi, and p notifies us about the number of water quality parameters. This WQI is based on the index introduced by the NSF (National Sanitation Foundation) (Bhutiani et al., 2016). This index is established by the Central Pollution Control board with different advancements in terms of water quality criteria. The Water quality index is supported and developed by the National Sanitation Foundation (NSF) (Brown et al., 1970). It is also known as NSF-WQI. This water quality index is denoted as
where P denotes the ith parameter measured values, quality rating is denoted by qi, and the relative weight of the ith parameters is denoted by wi.
The water quality index arithmetic index was presented (Cude, 2001). It is a very popular and standard method used by many investors and researchers in their studies (Ramakrishniah et al., 2009; Ahmad et al., 2012).
In this study, the quality rating can be calculated using the following equation:
where qi signifies the ith parameter quality rating for n water quality parameters, water.
The quality parameters' actual and definite value is denoted by Vactual, the parameters ideal value is symbolized by Videal, and the standard value of the parameters, which is suggested by the WHO, is denoted by Vstandard. The ideal values for DO and pH are 14.6 and 7 mg/L, whereas for the other parameters, it is equal to zero. After the calculation of quality rating, (relative weight), Wi has to be calculated by inversing the standard value of the parameter. Finally, the following equation was used to calculate the overall water quality index (WQI):
Here, signifying the relative weight and quality rating is symbolized by Wi and qi.
Trend Analysis
In this study, to forecast the pollution trend analysis, the linear regression model has been used. According to the linear regression model, the relationship between the two variables a and b can be expressed as:
Where x and y are the model parameters, which are known as regression coefficients, and B is the dependent variable. A is known as an independent variable, and € is the error variable. For making a prediction using a linear regression model is
The parameters x and y are calculated using the following equations:
Methodology
The flow chart for the methodology used is shown in Figure 1. The water quality index is calculated using the weight arithmetic water quality index method, which has been discussed in the Water Quality Index and Trend Analysis section.
The value of the water quality index has been compared with the standard values of WQI, which is shown in Table 1. The water quality rating is divided into five categories. The range from 0 to 25 is coming under (A) grading with excellent water quality, the range from 26 to 50 is for grading (B) with good water quality, and respectively, (C), (D), and (E) gradings are categorized for different WQI values (Chauhan and Singh, 2010).
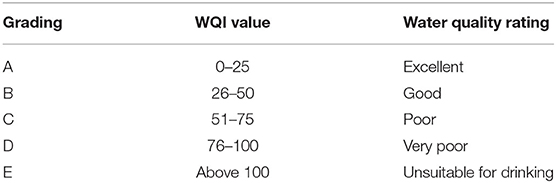
Table 1. The standard values of water quality index (WQI) using weight arithmetic water quality index method.
Dataset Collection
The most populous city of Uttarakhand is Dehradun also spelled Dear Doon. It is the capital of Uttrakhand, which is one among the 28 states in India (Figure 2). It is famous for its Doon Basmati Rice. Dehradun city has famous institutions like IMA (Indian Military Academy) regarded as one of the best officer training academies in India, Forest Research Institute, Indian Institute of Petroleum, and the famous ONGC training institute. This city is also famous among the tourists. It has many adventurous activities like rafting, bungee jumping, paragliding, etc. (Rafiq, 2016). The city is located about 255 km from New Delhi and 168 km from Chandigarh. The climate condition of Dehradun is humid, subtropical, and a summer temperature can reach a maximum of 44°C. This city is also located very close to Nainital, which has the famous Jim Corbett National Park attracting many tourists (Bhutiani et al., 2015).
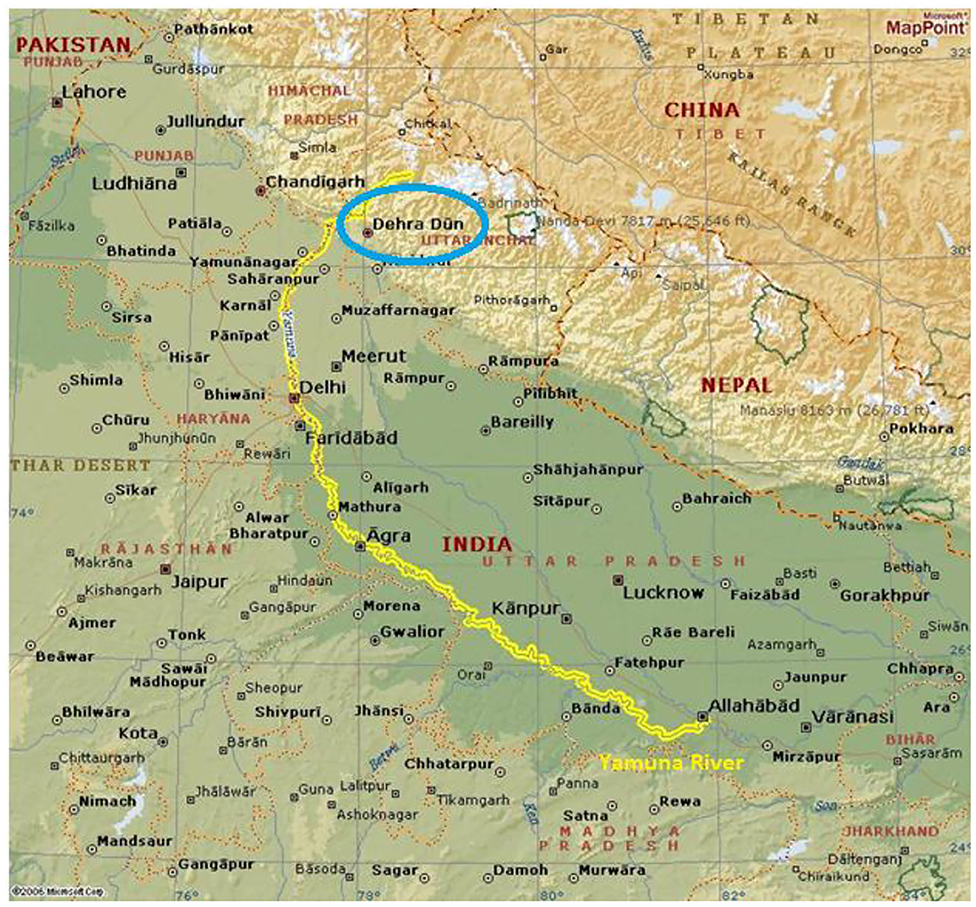
Figure 2. Map for considering location for Yamuna, Dehradun (https://www.bcmtouring.com/forums/thread).
The present study was undertaken for a period of 3 years from 2017 to 2019 to check the water quality analysis for the physicochemical attributes below. In the present study, water samples were collected on a yearly basis from the Yamuna river canal in Dehradun, Uttarakhand, India. The samples were analyzed for 12 different physicochemical attributes like ph, BOD, COD, Total Coliform, Temp, DO, Alkalinity, Chlorides, Calcium, Magnesium, and Hardness as Calcium Carbonate, and TDS (Tyagi et al., 2020). The Yamuna river plays a very crucial role in Dehradun's geography. The Yamuna river is severely polluted and needs urgent revival. The river passes through Uttarakhand. Uttarakhand has always been a tourist spot and experiences heavy tourists perennially, and Dehradun, being the capital city, also bears the brunt. The Yamuna river in Dehradun is polluted due to the exceptional tourist activities. Dehradun is also famous for the Kumaoni Holi, Jhanda Fair, Tapkeshwar Mela, and Bissu Mela. A lot of waste materials are dumped into the Yamuna river, and they contaminate the river. Water might be untreated for long spans of time. Also, a lot of industries–primarily biotechnology and food processing, are set up in Dehradun; they also mindlessly dump their waste in the Yamuna river. Industrial waste is not fully responsible for the pollution, but some poor sewage systems and human activities are also responsible for it (Bhutiani and Khanna, 2007).
Dehradun is a home to many agricultural and horticulture activities such as rice, litchi, and tea plantations. Agricultural waste also plays a major role in polluting the Yamuna river in Dehradun. The pollution is also increased by the excessive usage of insecticides and pesticides (Tiwari et al., 2020). There are also people who wash their clothes, utensils, and defecate in or around the river, thus leading to pollution. The stretch of the Yamuna river in Dehradun thus has a lot of coliform bacteria. Government projects such as road construction might also be responsible for dumping waste, although rules have been drastically upgraded in the last two decades or so. Some cattle washing activities and religious activities also polluted the Yamuna river (Bhutiani et al., 2018).
Results and Discussion
The study aims to examine the alteration in the quality of water of the Yamuna river at Dehradun in the year 2017. Water quality index (WQI) is going to be used in the study so that the changes and variations in the quality of water of the Yamuna river can be measured. The conventional method by which inspection can be done for the water quality has 12 physicochemical parameters (TDS, Chlorides, Alkalinity, DO, Temperature, COD, BOD, pH, Magnesium, Hardness, Total Coliform, and Calcium). These parameters will be measured carefully, and their respective value will be found. So, the standard value and observed value will be compared with each other, and the variation is going to be measured between them. By this variation, identification of the quality of water can be done.
Measurement of Physicochemical Parameters at Dehradun for 2017
Water samples have been taken at different months for the year 2017 (Table 2). The mean and standard deviation for the measured values have been also calculated. The mean is the number found by summing every data point and dividing by the number of data points. It is also called average. The standard deviation is defined as the number that is going to tell about the measurements for a group that is spread out from the mean or expected value. A low standard deviation signifies that many numbers are very close to the mean (Bisht et al., 2017). A high standard deviation signifies that the numbers are very much spread out. So, the accurate value for the quality of water can be found out easily using this.
The maximum value of pH is in the month of January when the water is a little more basic, and the minimum value is in July when it is less basic. The mean pH is 7.735, and the standard deviation is 0.086986589. The maximum value of the biochemical oxygen demand (BOD) is in January, which indicates more polluted water, and the minimum value is in the months of April, July, and October, which indicates less polluted water. The mean of BOD is 1.05, and the standard deviation is 0.1. The maximum value of COD is in January and October, which indicates a large quantity of oxidizable organic materials in the sample, and the minimum value is in April and July which indicates a lesser quantity of oxidizable organic materials in the sample. The mean COD is 5 and the standard deviation is 1.154700538.
The maximum value of Total Coliform is in July, which indicates that the water-borne illness is increased, and the minimum value is in October which indicates that the water-borne illness is decreased. The mean of Total Coliform is 65, and the standard deviation is 17.32050808. The maximum value of Temp is in July, which indicates increased chemical reactions generally, and the minimum value is in January, which indicates decreased chemical reactions. The mean of Temp is 17.75, and the standard deviation is 2.62995564. The maximum value of DO is in October, and the minimum value is in January, April, and July. The mean of DO is 8.7, and the standard deviation is 0.2. The maximum value of Alkalinity/visual titration CaCO3 is in July, which indicates greater buffering capacity against pH changes, and the minimum value is in April, which indicates lesser buffering capacity against pH changes. The mean of Alkalinity/visual titration CaCO3 is 64, and the standard deviation is 5.887840578. The maximum value of Chlorides is in July, which indicates body-related diseases, and the minimum value is in April and October. The mean of Chlorides is 5.75, and the standard deviation is 0.9574271078. The maximum value of Calcium as CaCO3 is in July, which has a positive effect on the body, and the minimum value is in April, which has a lesser positive effect on the body. The mean of CaCO3 is 41, and the standard deviation is 4.760952286. The maximum value of Magnesium as CaCO3 is in July, which has a positive effect on the body, and the minimum value is in January, which has a lesser positive effect on the body. The mean of Magnesium as CaCO3 is 32.5, and the standard deviation is 2.516611478. The maximum value of Hardness as CaCO3 is in July, which has a good effect on the body, and the minimum value is in April. The mean of Hardness as CaCO3 is 73.5, and the standard deviation is 6.191391874. The maximum value of TDS is in July, which specifies more toxic minerals, and the minimum value is in April, which specifies less toxic minerals. The mean of TDS is 83.75, and the standard deviation is 14.7507062. Water quality index (WQI) was used for the evaluation of the variation in the water quality of the Yamuna river at Dehradun over 3 years. The standard and prescribed methods have been used to analyze the water quality for 12 physicochemical parameters (TDS, Chlorides, Alkalinity, DO, Temperature, COD, BOD, pH, Magnesium, Hardness, Total Coliform, and Calcium). Calculations have been performed using the standardized formula and mathematical models. Detailed calculations and methodology have been used to find the water quality index as accurately as possible. The WQI of the Yamuna river in Dehradun for the year 2017 was 42.87 (Table 2). According to WHO, the WQI should be below 60 for its quality to be at least fair. Here, it can be easily concluded that the Yamuna river is polluted, but it is still revivable. Developmental and maintaining efforts can be adopted to make the Yamuna river clean again and improve the WQI drastically.
Total coliform is positively correlated with CaCO3, chlorides, and hardness of CaCO3.Temp is positively correlated with the magnesium of CaCO3 and TDS and negatively correlated with pH, BOD, and COD. DO is positively correlated with COD and negatively correlated with chlorides. Alkalinity is positively correlated with chlorides, TDS, hardness, and the magnesium of CaCO3 and negatively correlated with pH. Chlorides are positively correlated with calcium and hardness of CaCO3 and negatively correlated with pH and DO. Magnesium (CaCO3) is positively correlated with hardness and TDS, and negative with pH, BOD, and COD. Hardness (CaCO3) is positive for TDS, Chlorides, Magnesium, and negative for pH. TDS is negative for pH and positive for all. The dendrogram and graphical representation for physicochemical parameters at Dehradun for 2017 are plotted between the months (January, April, July, and October) and the parameters [TDS, Total Coliform (MPN/100 ml), Alkalinity/visual titration CaCO3, Hardness as CaCO3, Calcium as CaCO3, Magnesium as CaCO3, Temp, BOD, pH, DO, COD, and Chlorides] (Figures 3, 4).
Cluster 1 (blue) represents lightly polluted, and the parameters include TDS, Total Coliform (MPN/100 ml), Alkalinity/visual titration CaCO3, and Hardness as CaCO3. Cluster 2 (red) represents moderately polluted, and the parameters include Calcium as CaCO3, Magnesium as CaCO3, Temp, BOD, pH, DO, COD, and Chlorides. Cluster 3 (black) represents heavily polluted and the parameters include Total Coliform (MPN/100 ml), Alkalinity/visual titration CaCO3, Hardness as CaCO3, Calcium as CaCO3, Magnesium as CaCO3, and Temp.
Measurement of Physicochemical Parameters at Dehradun for 2018
Water samples have been taken in different months for the year 2018 (Table 3). Mean and standard deviation for the measured values have been also calculated. The maximum value of pH is in October so the water is a little more basic, and the minimum value is in January, which means the water is less basic. The mean pH is 7.6325, and the standard deviation is 0.420585. The maximum value and minimum value of BOD are equal every month. The mean of BOD is 1, and the standard deviation is 0.
The maximum value of COD is in April indicating a large quantity of oxidizable organic material in the sample, and the minimum value is in January, July, and October indicating a lesser quantity of oxidizable organic materials in the sample. The mean of COD is 4.5, and the standard deviation is 1. The maximum value of Total Coliform is in July indicating the water-borne illness is increased, and the minimum value is in January, April, and October indicating the water-borne illness is decreased. The mean of Total Coliform is 50, and the standard deviation is 20. The maximum value of Temp is in July indicating increased chemical reactions generally, and the minimum value is in January indicating decreased chemical reactions. The mean of Temp is 18.25, and the standard deviation is 1.707825. The maximum value of DO is in April, and the minimum value is in January and July. The mean of DO is 8.85, and the standard deviation is 0.251661. The maximum value of Alkalinity/visual titration CaCO3 is in July indicating higher buffering capacity against pH changes, and the minimum value is in April indicating lower buffering capacity against pH changes. The mean of Alkalinity/visual titration CaCO3 is 64.5, and the standard deviation is 6.608076.
The maximum value of Chlorides is in January, July, and October indicating body-related diseases, and the minimum value is in April. The mean of Chlorides is 5.75, and the standard deviation is 0.5. The maximum value of Calcium as CaCO3 is in July, which has a positive effect on the body, and the minimum value is in January, April, and October, which has a less positive effect on the body. The mean of CaCO3 is 41.5, and the standard deviation is 3. The maximum value of Magnesium as CaCO3 is in July, which has a positive effect on the body, and the minimum value is in April, which has a less positive effect on the body. The mean of Magnesium as CaCO3 is 33, and the standard deviation is 2.581989. The maximum value of Hardness as CaCO3 is in July, which has a good effect on the body, and the minimum value is in April. The mean of Hardness as CaCO3 is 74.5, and the standard deviation is 5.259911. The maximum value of TDS is in July specifying the presence of toxic minerals, and the minimum value is in January specifying the presence of less toxic minerals. The mean of TDS is 87.5, and the standard deviation is 15.60983.
Water quality index (WQI) was used in the evaluation of the variation in water quality of the Yamuna river at Dehradun over 3 years. The standard and prescribed method has been used to analyze the water quality for the 12 physicochemical parameters (TDS, Chlorides, Alkalinity, DO, Temperature, COD, BOD, pH, Magnesium, Hardness, Total Coliform, and Calcium). Calculations have been performed using the standardized formula and mathematical models. Detailed calculations and methodology have been used to find the water quality index as accurately as possible. The WQI of the Yamuna river in Dehradun for the year 2018 was 40.47 (Table 3). According to WHO, the WQI should be below 60 for its quality to be at least fair. Here, it can be easily concluded that the Yamuna river is polluted, but it is still revivable. Developmental and maintaining efforts can be adopted to make the Yamuna river clean again and improve the WQI severely. Moreover, it is a positive sign that the WQI of the Yamuna river has improved greatly for the year 2018 compared to the year 2017.
The dendrogram and graphical representation for the physicochemical parameters at Dehradun for 2018 are plotted between the months (January, April, July, and October) and also the parameters [TDS, Total Coliform (MPN/100 ml), Alkalinity/visual titration CaCO3, Hardness as CaCO3, Calcium as CaCO3, Magnesium as CaCO3, Temp, BOD, pH, DO, COD, and Chlorides] (Figures 5, 6). Cluster 1 (blue) represents lightly polluted and the parameters include Temp, BOD, pH, DO, COD, and Chlorides.
Cluster 2 (red) represents moderately polluted, and the parameters include Total Coliform (MPN/100 ml), Calcium as CaCO3, Magnesium as CaCO3, TDS, Alkalinity/visual titration CaCO3, Hardness as CaCO3. Cluster 3 (black) represents heavily polluted, and the parameters include BOD, pH, DO, COD, Chlorides, Total Coliform (MPN/100 ml), Alkalinity/visual titration CaCO3, Hardness as CaCO3, Calcium as CaCO3, and Magnesium as CaCO3.
Measurement of Physicochemical Parameters at Dehradun for 2019
Water samples have been taken in different months for the year 2019 (Table 4). The mean and standard deviation for the measured values have been also calculated. The mean is the number found by summing every data point and dividing by the number of data points. Standard deviation is defined as the number that is going to tell about the measurements for a group that is spread out from the mean or expected value. Comparing the values of this year with those of the previous years leads to the outcomes being observed.
The maximum value of pH is in the month of January when the, water is a little more basic and the minimum value is in the month of October when the water is less basic. The mean pH is 7.6225, and the standard deviation is 0.411208. The maximum value of BOD is in July when there is a large quantity of polluted water, and the minimum value is in January, April, and October when there is less quantity of polluted water. The mean of BOD is 1.05, and the standard deviation is 0.1. The maximum value of COD is in July and October indicating a greater amount of oxidizable organic materials in the sample, and the minimum value is in January and April indicating a lesser amount of oxidizable organic materials in the sample. The mean of COD is 5, and the standard deviation is 1.154701. The maximum value of Total Coliform is in April indicating that water-borne illness is increased, and the minimum value is in January indicating that water-borne illness is decreased. The mean of Total Coliform is 182.5, and the standard deviation is 93.22911. The maximum value of Temp is in October indicating increased chemical reactions generally, and the minimum value is in January indicating decreased chemical reactions. The mean of Temp is 18.5, and the standard deviation is 1.290994. The maximum value of DO is in April, and the minimum value is in July. The mean of DO is 8.9, and the standard deviation is 0.258199. The maximum value of Alkalinity/visual titration CaCO3 is in April indicating higher buffering capacity against pH changes, and the minimum value is in October indicating lower buffering capacity against pH changes. The mean of Alkalinity/visual titration CaCO3 is 68, and the standard deviation is 5.416026.
The maximum value of Chlorides is in October indicating body-related diseases, and the minimum value is in January, July, and April. The mean of Chlorides is 7.5, and the standard deviation is 3. The maximum value of Calcium as CaCO3 is in October, which has a positive effect on the body, and the minimum value is in April, the month which has a less positive effect on the body. The mean of CaCO3 is 49.5, and the standard deviation is 8.386497. The maximum value of Magnesium as CaCO3 is in April, which has a positive effect on the body, and the minimum value is in October, which has a less positive effect on the body. The mean of Magnesium as CaCO3 is 29, and the standard deviation is 7.745967. The maximum value of Hardness as CaCO3 is in April and October, which has a good effect on the body, and the minimum value is in July. The mean of Hardness as CaCO3 is 78.5, and the standard deviation is 1.914854. The maximum value of TDS is in July specifying the presence of toxic minerals, and the minimum value is in October specifying the presence of less toxic minerals. The mean of TDS is 99.25, and the standard deviation is 12.84199.
Water quality index (WQI) was used to evaluate the variation in water quality of the Yamuna river at Dehradun over 3 years. The standard and prescribed method has been used to analyze the water quality for 12 physiochemical parameters (TDS, Chlorides, Alkalinity, DO, Temperature, COD, BOD, pH, Magnesium, Hardness, Total Coliform, and Calcium). Calculations have been performed using the standardized formula and mathematical models. Detailed calculations and methodology have been used to find the water quality index as accurately as possible. The WQI of the Yamuna river in Dehradun for the year 2019 was 40.82 (Table 4). According to WHO, the WQI should be below 60 for its quality to be at least fair. Here, it can be easily concluded that the Yamuna river is polluted, but it is still revivable. Developmental and maintaining efforts can be adopted to make the Yamuna river clean again and improve the WQI drastically. Moreover, it is a positive sign that the WQI of the Yamuna river has improved for year 2019 compared to the year 2017, whereas the WQI has increased again in 2019 compared to 2018. It can be documented that the Yamuna river was the cleanest in the year 2018, and its water quality in 2019 has improved in collation to the year 2017.
The correlation coefficients between the inspected parameters of the Yamuna river water at Dehradun in the year 2019 are shown in Table 5. Ph is positive for TDS, alkalinity, and Magnesium (CaCO3) and negative for COD, Temp, and Calcium (CaCO3). BOD is positive for COD and TDS and negative for DO and hardness (CaCO3). COD is positive for Temp, Chlorides, and Calcium and negative for DO, magnesium (CaCO3), and alkalinity. Temp is positive for calcium (CaCO3) and negative for alkalinity. DO is positive for Magnesium (CaCO3). Alkalinity is positive for TDS and negative for Calcium, Magnesium, and hardness of CaCO3. The dendrogram and graphical representation for physicochemical parameters at Dehradun for 2017 are plotted between months (January, April, July, and October) and parameters (TDS, Total Coliform (MPN/100 ml), Alkalinity/visual titration CaCO3, Hardness as CaCO3, Calcium as CaCO3, Magnesium as CaCO3, Temp, BOD, pH, DO, COD, and Chlorides) (Figures 7, 8).
Cluster 1 (blue) represents lightly polluted, and the parameters include BOD, pH, DO, COD, Chlorides, Temp, and Magnesium as CaCO3. Cluster 2 (red) represents moderately polluted, and the parameters include Total Coliform (MPN/100 ml), TDS, Calcium as CaCO3, Alkalinity/visual titration CaCO3, and Hardness as CaCO3. Cluster 3 (black) represents heavily polluted, and the parameters include COD, Chlorides, Temp, Magnesium as CaCO3, Total Coliform (MPN/100 ml), and TDS.
In Table 6, the variations in the 12 physicochemical parameter values for Yamuna water at Dehradun for 2017, 2018, and 2019 are shown.
Trend Forecasting
This section is briefing about the Yamuna river water pollution trend in the next 4 years. The study demonstrates the trend of six physicochemical parameters for the years 2020 to 2024. The considered parameters for calculating the trend forecasting are Temp, Total Coliform, TDS, Hardness, pH, and DO. The forecasting for the said parameters are shown in Figures 9–11.
According to the trend analysis, the values of four parameters named Temperature, Total Coliform, TDS, and Hardness are increasing yearly, whereas the values of pH and DO are not rising year by year. The trend forecasting is verifying whether the exceptional tourist activity, poor sewage facility, and insufficient wastewater management amenities, is degrading the water of the Yamuna river at Dehradun year by year.
Discussion
The dendrogram of the mean is plotted between years (2017, 2018, and 2019) and parameters [TDS, Total Coliform (MPN/100 ml), Alkalinity/visual titration CaCO3, Hardness as CaCO3, Calcium as CaCO3, Magnesium as CaCO3, Temp, BOD, pH, DO, COD, and Chlorides] (Figure 12). Cluster 1 (blue) represents lightly polluted, and the parameters include Total Coliform (MPN/100 ml), TDS, Alkalinity/visual titration CaCO3, and Hardness as CaCO3. Cluster 2 (red) represents moderately polluted, and the parameters include Calcium as CaCO3 and Magnesium as CaCO3. Cluster 3 (black) represents heavily polluted, and the parameters include TDS, Alkalinity/visual titration CaCO3, Hardness as CaCO3, Calcium as CaCO3, Magnesium as CaCO3, and Temp. Cluster 4 (green) represents equal parameters, and it includes Temp, BOD, pH, DO, COD, and Chlorides.
The variation in observed values, quality rating, and Wiqi can be analyzed using Tables 2, 4, 6.
According to WHO, the WQI should be below 60 for its quality to be at least fair. If it is more than 60, then the quality of the water is surely poor. If the WQI is <30, then the water quality is good. The WQI of the Yamuna river in Dehradun for the year 2017 was 42.87. It can be easily said that the Yamuna river was quite polluted back then. Developmental and maintaining plans were implemented to make the Yamuna river clean again and improve the WQI drastically. The WQI of the Yamuna river in 2017 was the highest in collation to the subsequent years. This must have set the alarm bells ringing for the government and the citizens. The government has introduced many measures to curb water pollution and revive the Yamuna river as quickly as possible. It is a positive sign that the WQI of the Yamuna river has improved significantly for the years 2018 to 40.47. It was a marked difference in comparison to that of the year 2017. Joint efforts and collaboration by the government and the citizens ensured that the Yamuna river is much cleaner than before, although in 2019, the WQI rose by a small margin to 40.82. It is a sign of relief that it is still much better than the quality of the water in the year 2017. If the measures of the government and corporation by the citizens continue to go hand in hand, the results will be for everyone to see. Even regions in the west would emulate the policies adopted to revive the rivers. Policies included a big budget for the revival project, strict norms for the industries, and appropriate penalties for the defaulters. A common concern for the degrading water quality index of the Yamuna river resulted in some swift actions from the citizens as well as from those who became more aware and conscious. It can be easily and comfortably said that the Yamuna river would be much cleaner and in a much-improved condition by the year 2025.
A comparative analysis is shown in Table 7. A comparison in description and limitations with previously published approaches are organized in this table.
Conclusion
Due to historical, geographical, religious, political, and sociocultural reasons, India has a unique place in the world. Pollution-causing activities have caused severe changes in aquatic environments over the last few decades. This paper aims to calculate the water quality index of the Yamuna river in Uttarakhand using 12 physicochemical parameters for a while for 3 years from 2017 to 2019. The values of the considered physicochemical parameters have been monitored using the various examining stations installed by the Central Pollution Control Board (CPCB), India. According to WHO, the WQI should be below 60 for its quality to be at least fair. If it is more than 60, then the quality of the water is surely poor. If the WQI is <30, then the water quality is good. The WQI of the Yamuna river in Dehradun for the year 2017 was 42.87. It can be easily said that the Yamuna river was quite polluted back then. Developmental and maintaining plans were implemented to make the Yamuna river clean again and improve the WQI drastically. The WQI of the Yamuna river in 2017 was the highest in collation to the subsequent years. This must have set the alarm bells ringing for the government and the citizens.
According to the trend analysis, the values of four parameters named Temperature, Total Coliform, TDS, and Hardness are increasing yearly, whereas the values of pH and DO are not rising year by year. The trend forecasting verifies whether the exceptional tourist activity, poor sewage facilities, and insufficient wastewater management amenities is degrading the water of the Yamuna river at Dehradun year by year. It is a positive sign that the WQI of the Yamuna river has improved significantly for the years 2018 to 40.47. It was a marked difference in comparison to the year 2017. Joint efforts and collaboration by the government and the citizens ensured that the Yamuna river is much cleaner than before, although in 2019, the WQI raised by a small margin to 40.82. It is a sign of relief that it is still much better than the quality of the water in the year 2017. If the measures of the government and corporation by the citizens continue to go hand in hand, the results will be for everyone to see. Even regions in the west would emulate the policies adopted to revive the rivers. A common concern for the degrading water quality index of the Yamuna river resulted in some swift actions from the citizens as well as those who became more aware and conscious.
Data Availability Statement
The datasets presented in this study can be found in online repositories. The names of the repository/repositories and accession number(s) can be found in the article/supplementary material.
Author Contributions
Data curation was done by RS and RK. Formal analysis was made by RS, RK, and SS. The investigation was done by RS, RK, and KS. The methodology was done by RS, NA-A, and RM. Project administration was performed by HL and AA. BP was in charge of the resources and software. BP and NA-A supervised the study. Visualization was done by BP and NA-A. RS, AA, RM, and BP wrote the original draft. All authors contributed to the article and approved the submitted version.
Conflict of Interest
The authors declare that the research was conducted in the absence of any commercial or financial relationships that could be construed as a potential conflict of interest.
References
Agarwal, A., Rafique, F., Rajesh, E., and Ahmed, S. (2016). Urban flood hazard mapping using change detection on wetness transformed images. Hydrol. Sci. J. 61, 816–825. doi: 10.1080/02626667.2014.952638
Ahmad, I. K., Salih, N. M., and Nzar, Y. H. (2012). Determination of water quality index (WQI) for Qalyasan stream in Sulaimani city/Kurdistan region of Iraq. Int. J. Plant Anim. Environ. Sci. 2, 148–157.
Ahmad, S., Farooq, S., Zahoor-Ul-Islam Khan Md., A., Zaidiand, W. A., and Matloob, H. (2017). Impact of urbanization on hydro logical regime in Indian cities. J. Environ. Res. Develop. 2, 594–604.
Allee, R. J., and Johnson, J. E. (1999). Use of satellite imagery to estimate surface chlorophyll a and Secchi disc depth of Bull Shoals Reservoir, Arkansas, USA. Int. J. Remote Sens. 20, 1057–1072. doi: 10.1080/014311699212849
Amandeep, V. (2011). Identification of land and water regions in a satellite image: a texture based approach. Int. J. Comput. Sci. Eng. Technol. 1, 361–365.
Bhutiani, R., Ahamad, F., Tyagi, V., and Ram, K. (2018). Evaluation of water quality of River Malin using water quality index (WQI). Environ. Conserv. J. 19, 191–201. doi: 10.36953/ECJ.2018.191228
Bhutiani, R., and Khanna, D. R. (2007). Ecological status of river Suswa: modelling DO and BOD. Environ. Monit. Assess. 125, 183–195. doi: 10.1007/s10661-006-9251-4
Bhutiani, R., Khanna, D. R., Kulkarni, D. B., and Ruhela, M. (2016). Assessment of Ganga river ecosystem at Haridwar, Uttarakhand, India with reference to water quality indices. Appl. Water Sci. 6, 107–113. doi: 10.1007/s13201-014-0206-6
Bhutiani, R., Khanna, D. R., Tyagi, B., Tyagi, P. K., and Kulkarni, D. B. (2015). Assessing environmental contamination of River Ganga using correlation and multivariate analysis. Pollution 1, 265–273.
Bisht, A. K., Singh, R., Bhutiani, R., Bhatt, A., and Kumar, K. (2017). Water quality modelling of the River Ganga using artificial neural network with reference to the various training functions. Environ. Conserv. J. 18, 41–48. doi: 10.36953/ECJ.2017.181206
Brown, R. M., McClelland, N. I., Deininger, R. A., and Tozer, R. G. (1970). Water quality index-do we dare? Water Sew. Works 117, 339–343.
Census Reports of India 2001. (1971–1991). Available online at: https://censusindia.gov.in/
Chabuk, A., Al-Ansari, N., Hussain, H. M., Knutsson, S., Pusch, R., and Laue, J. (2017). Combining GIS applications and method of multi-criteriadecision-making (AHP) for landfill siting in Al-HashimiyahQadhaa, Babylon, Iraq. Sustainability 9, 19–32. doi: 10.3390/su9111932
Chauhan, A., and Singh, S. (2010). Evaluation of Ganga water for drinking purpose by water quality index at Rishikesh, Uttarakhand, India. Rep. Opin. 2, 53–61.
Cude, C. G. (2001). Oregon water quality index: a tool for evaluating water quality management effectiveness. J. Am. Water Resour. Assoc. 37, 125–137. doi: 10.1111/j.1752-1688.2001.tb05480.x
Duong, D. N. (2012). Water body extraction from multi spectral image by spectral pattern analysis. J. Photogramm Remote Sens. Spat. Inf. Sci. Melb. XXXIX-B 8, 248–259. doi: 10.5194/isprsarchives-XXXIX-B8-181-2012
Dwivedi, S. L., and Pathak, V. A. (2007). Preliminary assignment of water quality index to Mandakini river, Chitrakoot. Indian J. Environ. Prot. 27, 1036–1038.
Fraser, R. N. (1998). Multispectral remote sensing of turbidity among Nebraska Sand Hills Lakes. Int. J. Remote Sens. 19, 3011–3016. doi: 10.1080/014311698214406
Girgin, S., Kazanci, N., and Dügel, M. (2010). Relationship between aquatic insects and heavy metals in an urban stream using multivariate techniques. Int. J. Environ. Sci. Technol. 7, 653–664. doi: 10.1007/BF03326175
Icaga, Y. (2007). Fuzzy evaluation of water quality classification. Ecol. Indic J. Elsevier 7, 710–718. doi: 10.1016/j.ecolind.2006.08.002
Kazi, T. G., Arain, M. B., Jamali, M. K., Jalbani, N., Afridi, H. I., Sarfraz, R. A., et al. (2009). Assessment of water quality of polluted lake using multivariate statistical techniques: a case study. Ecotoxicol. Environ. Saf. J. 72, 301–309. doi: 10.1016/j.ecoenv.2008.02.024
Kondratyev, K. Y., Pozdnyakov, D. V., and Pettersson, L. H. (1998). Water quality remote sensing in the visible spectrum. Int. J. Remote Sens. 19, 957–979. doi: 10.1080/014311698215810
Marale, S. (2012). Shifting role of ecology in solving global environmental problems: selected practical tools. Environ. Develop. Sustain. 14, 869–884. doi: 10.1007/s10668-012-9362-8
Nazeer, M., and Nichol, J. E. (2015). Combininglandsat TM/ETM + and HJ-1 A/B CCD sensors for monitoring coastal water quality in Hong Kong. IEEE Geosci. Remote Sens. Lett. 12, 1898–1902. doi: 10.1109/LGRS.2015.2436899
Panwar, A., Bartwal, S., Dangwal, S., Aswal, A., Bhandari, A., and Rawat, S. (2015). Water quality assessment of River Ganga using remote sensing and GIS technique. Int. J. Adv. Remote Sens. GIS 4, 1253–1261. doi: 10.23953/cloud.ijarsg.116
Pattiaratchi, C. B., Lavery, P., Wyllie, A., and Hick, P. (1994). Estimates of water-quality in coastal waters using multi-date Landsat Thematic Mapper data. Int. J. Remote Sens. 15, 84–1571. doi: 10.1080/01431169408954192
Ramakrishniah, C. R., Sadashivaiah, C., and Ranganna, G. (2009). Assessment of water quality index for the groundwater in Tumkur Taluk. E-J Chem. 6, 523–530. doi: 10.1155/2009/757424
Ronghang, M., Gupta, A., Mehrotra, I., Kumar, P., Patwal, P., Kumar, S., et al. (2019). Riverbank filtration: a case study of four sites in the hilly regions of Uttarakhand, India. Sustain. Water Resour. Manag. 5, 831–845. doi: 10.1007/s40899-018-0255-3
Sharma, P., Sood, S., and Mishra, S. K. (2020). Development of multiple linear regression model for biochemical oxygen demand (BOD) removal efficiency of different sewage treatment technologies in Delhi, India. Sustain. Water Resour. Manag. 6:29. doi: 10.1007/s40899-020-00377-9
Shi, L., Mao, Z., and Wang, Z. (2018). Retrieval of total suspended matter concentrations from high resolution WorldView-2 imagery: a case study of inland rivers. IOP Conf. Ser. Earth Environ. Sci. 121:032036. doi: 10.1088/1755-1315/121/3/032036
Shukla, S., Khire, M. V., and Godan, S. S. (2018). Effects of urbanization on surface and subsurface hydrologic variables of upper bhima river basin, Maharashtra, India. Model. Earth Syst. Environ. 4, 699–728. doi: 10.1007/s40808-018-0446-9
Singh, A., Jakubowski, A. R., Chidister, I., and Townsend, P. A. (2013). A MODIS approach to predicting stream water quality in Wisconsin. Remote Sens. Environ. 128, 74–86. doi: 10.1016/j.rse.2012.10.001
Song, K. S., Li, L., Li, S., Tedesco, L., Hall, B., and Li, L. H. (2012). Hyperspectral remote sensing of total phosphorus (TP) in three central Indiana water supply reservoirs. J. Water Air Soil Pollut. 223, 1481–1502. doi: 10.1007/s11270-011-0959-6
Tiwari, S. K., Gupta, A. K., and Asthana, A. K. L. (2020). Evaluating CO2 flux and recharge source in geothermal springs, Garhwal Himalaya, India: stable isotope systematics and geochemical proxies. Environ. Sci. Pollut. Res. 27, 14818–14835. doi: 10.1007/s11356-020-07922-1
Trombadore, O., Nandi, I., and Shah, K. (2020). Effective data convergence, mapping, and pollution categorization of ghats at Ganga River Front in Varanasi. Environ. Sci. Pollut. Res. 27, 15912–15924. doi: 10.1007/s11356-019-06526-8
Tyagi, S., Dubey, R. C., Bhutiani, R., and Ahamad, F. (2020). Multivariate Statistical analysis of river ganga water at Rishikesh and Haridwar, India. Anal. Chem. Lett. 10, 195–213. doi: 10.1080/22297928.2020.1756405
Wang, Y. P., Xia, H., Fu, J. M., and Sheng, G. Y. (2004). Water quality change in reservoirs of Shenzhen, China: detection using LANDSAT/TM data. Sci. Total Environ. 328, 195–206. doi: 10.1016/j.scitotenv.2004.02.020
Keywords: water quality index, Yamuna river, physico-chemical parameters, water pollution, Dehradun city
Citation: Sharma R, Kumar R, Satapathy SC, Al-Ansari N, Singh KK, Mahapatra RP, Agarwal AK, Le HV and Pham BT (2020) Analysis of Water Pollution Using Different Physicochemical Parameters: A Study of Yamuna River. Front. Environ. Sci. 8:581591. doi: 10.3389/fenvs.2020.581591
Received: 09 July 2020; Accepted: 11 November 2020;
Published: 11 December 2020.
Edited by:
Senthil Kumar Ponnusamy, SSN College of Engineering, IndiaReviewed by:
Rakesh Bhutiani, Gurukul Kangri Vishwavidyalaya, IndiaLalit Garg, University of Malta, Malta
Copyright © 2020 Sharma, Kumar, Satapathy, Al-Ansari, Singh, Mahapatra, Agarwal, Le and Pham. This is an open-access article distributed under the terms of the Creative Commons Attribution License (CC BY). The use, distribution or reproduction in other forums is permitted, provided the original author(s) and the copyright owner(s) are credited and that the original publication in this journal is cited, in accordance with accepted academic practice. No use, distribution or reproduction is permitted which does not comply with these terms.
*Correspondence: Nadhir Al-Ansari, nadhir.alansari@ltu.se; Hiep Van Le, levanhiep2@duytan.edu.vn; Binh Thai Pham, binhpt@utt.edu.vn