- 1Institute of Hydrobiology and Aquatic Ecosystem Management (IHG), University of Natural Resources and Life Sciences, Vienna, Austria
- 2Clinical Division for Fish Medicine, University of Veterinary Medicine, Vienna, Austria
Water temperature is one of the most important abiotic parameters in rivers having direct and indirect effects on fish. Especially cold-water species like the brown trout (Salmo trutta) are limited by high temperatures. Beside direct physiological stress, higher water temperatures also reinforce the emergence of diseases. In this study we investigate thermal regimes of rivers based on a large-scale dataset covering Austria (~70,000 km2). The analyses aim to clarify to what extent water temperatures support the emergence of proliferative kidney disease (PKD) and assemble physiological stress for brown trout under current and future climate conditions. Data from 274 gauging stations at 184 rivers were used to calibrate a water temperature model and to investigate critical water temperature thresholds. Therefore, we developed a risk assessment scheme to identify river reaches that already have or will develop critical thermal regimes for brown trout in respect of PKD emergence and thermal physiological stress. The results revealed severe changes in the thermal regimes of the investigated rivers under climate change. Furthermore, the variable characterizing riparian vegetation played a vital role to explain cooling of the water in downstream direction. In respect of PKD, the amount of river reaches having unlikely outbreaks of PKD decreased from 72.6% under current conditions to 37.7% in the far future RCP8.5 scenario. Within small rivers that currently showed optimal thermal regimes over large extents (10,244 km), the habitat suitability will be reduced by combined effects of PKD and physiological stress to 6,554 km. In general, suitable habitats of S. trutta will shift upstream, thus to higher altitudes, and to smaller, alpine rivers in Austria. The warming leads to physiological stress that induces a diminished growth due to the non-positive transition of caloric values to growth as well as cardiac dysfunction in brown trout. These factors will further restrict the distribution of brown trout. However, the results also underline the enormous importance of the alpine region as a future refuge for brown trout in Central Europe. Thus, this study will help to inform managers to timely develop robust conservation strategies.
Introduction
Climate change represents a major driver of biodiversity change (Markovic et al., 2014). Riverine ecosystems are especially vulnerable to the impacts of climate change as warming strongly alters the habitat conditions of fish (Comte et al., 2013). Meanwhile, around 30% of the European freshwater fishes are recognized as susceptible to climate change (Jarić et al., 2019). However, most studies still focus solely on direct effects mediated by temperature increase although climate change and the associated warming will have indirect effects, such as a pronounced emergence of pathogens, too.
In aquatic ecosystems, water temperature is one of the most important abiotic parameters controlling life (Magnuson et al., 1979; Caissie, 2006). As fish are obligate poikilotherm animals, their body temperature and metabolism are regulated by the surrounding water. Fry (1971) described a 5-fold effect of temperature on fish. Besides the controlling and limiting factors of temperature that directly affect the fish, masking factors, are hardly addressed in the context of climate change impact yet. Most fish show preferences to certain temperature ranges that can be tolerated or not (Coutant, 1977). Especially cold-water species like the brown trout, Salmo trutta (Linnaeus 1758), are limited by high temperatures. Thus, brown trout are highly sensitive to climate change impacts as the thermal regimes of their habitats are affected by warming. Future scenarios predict a reduction of up to 64% of suitable stream reaches for brown trout in Europe till the 2080s (Filipe et al., 2013).
Besides direct thermal effects like physiological stress, higher water temperatures can also prompt masking and indirect effects on fish such as a more common emergence of specific diseases (Karvonen et al., 2010). Here, a weakened immune response (Bowden, 2008; Bettge et al., 2009a) and faster development of pathogens in warmer environments play a prominent role. The proliferative kidney disease (PKD) is a widespread parasitic disease that especially affects salmonid fish species. PKD can lead to the death of S. trutta affecting the wild populations in North America and Europe. Several studies highlighted declines in wild salmonid populations due to PKD (e.g., Sterud et al., 2007; Burkhardt-Holm, 2009). In Austria, PKD is a newly discovered but widespread disease (Gorgoglione et al., 2016; Lewisch et al., 2018; Waldner et al., 2019).
As PKD is strongly temperature-dependent climate change will probably enhance the further emergence of the disease (Okamura et al., 2011). Its emergence is related to the occurrence of prolonged periods of warmer water temperatures that support a faster growth of the Bryozoan host (Okamura et al., 2011). Thus, promoting the development of infections in Bryozoan colonies caused by the parasitic myxozoa Tetracapsuloides bryosalmonae (Tops et al., 2009), which is further leading to the clinical outbreak of PKD and mortality in brown trout (Bettge et al., 2009a).
Although much evidence exists on both, the physiological stress induced by water temperature increases and the temperature-dependency of PKD outbreaks in trout, from laboratory studies, less knowledge exists on water temperature related effects on trout populations in the wild. Physiologically, the upper thermal boundary for growth is at about 19.5°C for brown trout (Elliott and Elliott, 2010) due to the non-positive transition of caloric values to growth (Elliott, 1976). With increasing temperature, the energy needed for the metabolism (e.g., swimming and digestion) is exponentially increased as described by the van 't Hoff rule (Ege and Krogh, 1914). With further increasing temperature the physiologic response of the fish intensifies. Subsequently, the cardiac function of S. trutta is limited above 20.9°C consequently leading to disturbed cardiac rhythmicity. In vivo experiments showed that above 23.5°C, the heart rate gets critical up to heart arrest (Vornanen et al., 2014).
The thermal regimes of rivers are strongly related to atmospheric conditions and to energy input by solar radiation. Environmental factors changing the radiation inputs like shading effects of the terrain topography or the riparian vegetation, therefore affect water temperatures. Moreover, the volume of the water body (i.e., discharge, width, depth, and velocity) influences the daily amplitude of water temperature; for example, wide and shallow rivers heat faster than large rivers or small streams (Caissie, 2006). As the water temperature is highly linked to the same driving forces as air temperature, climate change will lead to further warming (Mohseni et al., 2003). Therefore, most river water temperature regression models rely on air temperature data, as they are linked to the same driving forces (Caissie, 2006).
Based on data from European rivers, Webb (1996) described an increase of +1°C in water temperatures in the last century (1901–1990). In the river Rhine, a 2°C-increase for the summer period was observed from 1978 to 2011 (ICPR, 2013). In the Danube and some of its Austrian tributaries, a temperature increase of about 0.3°C per decade since 1900 was observed (Dokulil, 2018).
Existing field-studies on PKD primarily focus on selected river systems or fish populations (e.g., Waldner et al., 2019). However, analyses on a larger spatial extent are needed to identify the actual and future risks related to PKD and physiological stress on S. trutta in general. Most studies address the importance of thermal regimes and climate change for the prevalence, outbreak and mortality of PKD but there is less knowledge about the spatial dimensions of the combined future effects.
We hypothesize that different thermal characteristics are relevant for PKD emergence and thermal stress in brown trout. We assume that both factors will increase with the progress of climate change. Accordingly, we address the following research questions: (1) Which parts of the river network feature a thermal regime supporting the emergence of PKD under current conditions? (2) Which parts of the river network feature a thermal regime supporting thermal stress for brown trout under current conditions? (3) How does the spatial distribution of both implications change under future climate conditions?
Materials and Methods
Our analyses included data on water temperature, river characteristics (e.g., slope, catchment size, glaciated area, etc.), and climate covering an area of around 70,000 km2 and a river network length of 23,819 km. The environmental information used in the analyses was backed up by evidence-based information from literature on the thermal preferences of S. trutta as well as temperature thresholds supporting the emergence and outbreak of PKD in trout.
Water Temperature Data
We used data from 274 river gauging stations in Austria that continuously record water temperature. This was available from the hydrographic services of the federal states (BMLFUW, 2017; Figure 1). The dataset for water temperature modeling included 1,831 WTQ95 values (95% quantile of daily mean water temperature in 1 year) from 184 rivers covering the years 2000–2011. This time period was used because of the overlay with a national climate dataset (Chimani et al., 2016).
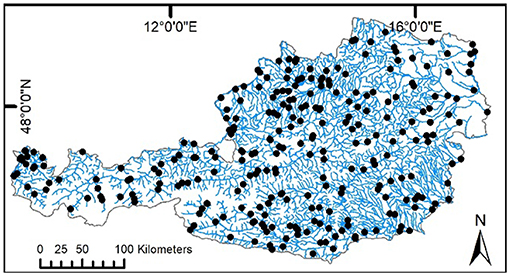
Figure 1. Location of the 274 Austrian river gauging stations (black dots) and the river network (blue lines) included in the water temperature model.
A larger water temperature dataset covering the years 2000–2015 with 3,000 WTQ95 values from 336 river gauging stations of 221 rivers was used to analyze water temperature thresholds relevant for PKD and thermal stress.
Both datasets did not contain gauging stations from lakes, lake outflows, artificial channels, the Danube, sites above 1,500 m.a.s.l., sites with missing spatial data and sites with manual or not clearly determined water temperature measurement.
River Network and Investigation Segments
The national river network of Austria (BMLFUW, 2016) represented the spatial basis for the analyses on the river segment resolution. It covers all rivers with a catchment size larger than 10 km2. We processed the river network in ArcGIS 10.4 (ESRI, 2016) to create regular 5 km (n = 5,985) reaches that represent the investigation segments. To consider the heterogeneity within the 5 km segments, we further queried all descriptors used in the modeling on basis of 1 km segments and summarized them for each 5 km segment. We excluded river segments with Strahler order <3 and an elevation above 1,500 m.a.s.l. from the dataset as the available water temperature data from the gauging stations did not sufficiently cover these areas.
For each segment, we queried Strahler order (“Strahler”), catchment size (“Catchment”), river slope (“Slope”), hillshade (“HillSha”), tree cover (“TreeDens”), and glaciated area in the catchment (“Glacier”). An overview of all parameters can be found in Table 1.
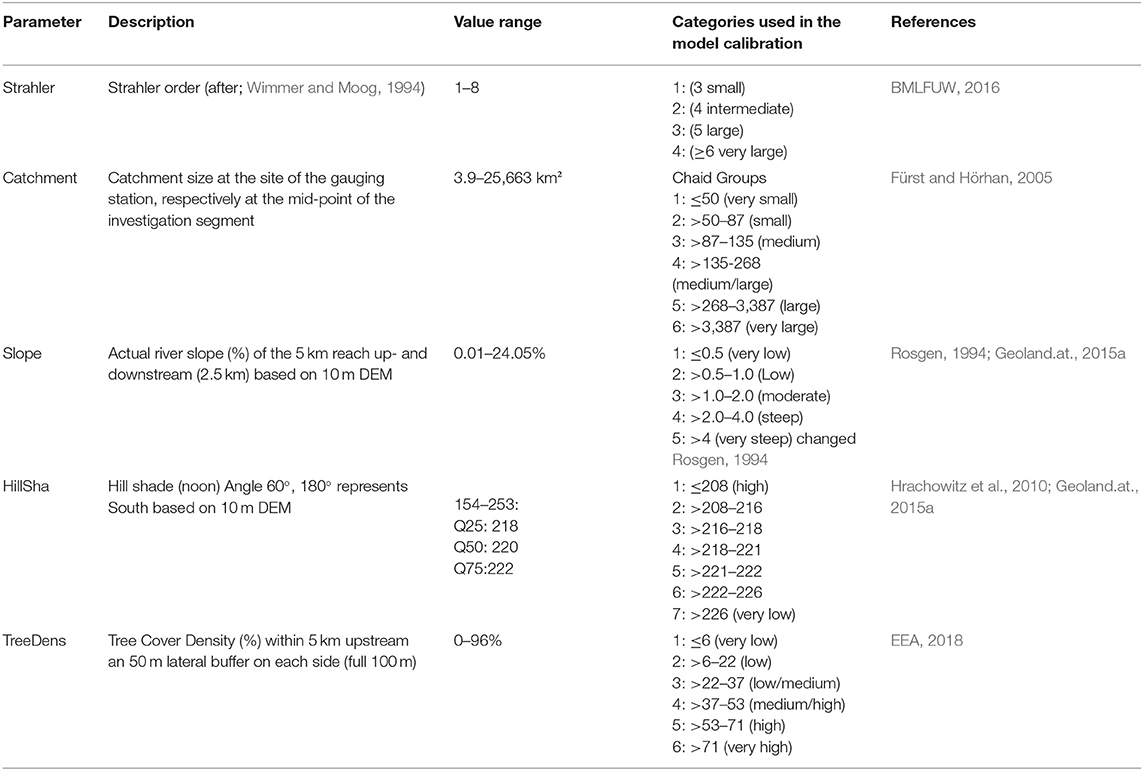
Table 1. Overview and explanation of the descriptors used in the water temperature model, as well as the value ranges in the training data (Value range), the categorized values (Categories used in the model calibration), and the data of the dataset.
Strahler order for the Austrian river network according to Wimmer and Moog (1994) was available as a line shapefile. We queried Strahler order for each investigation segment. To consider river size effects relevant for the thermal regime, we classified the temperature gauging stations and the investigation segments into four groups covering small to large rivers. Accordingly, for each type, a regression model was calibrated.
The catchment size was calculated on the basis of the Austrian sub-catchments (Fürst and Hörhan, 2005). We located the midpoint of the investigation segments to the according sub-catchment. Then consequently the upstream sub-catchment area was summed up for each midpoint. Categories were classified by a decision tree (Chaid) into 6 groups with WTQ95 as the predicting variable.
River slope was calculated within the 5 km segments as the height difference between starting and endpoint of the segment based on a 10 m digital elevation model available from Geoland.at. (2015a), and then classified into 5 groups after Rosgen (1994).
Two descriptors, hillshade (HillSha) and tree cover density (TreeDens), were used to consider shading effects on the river. Hillshade combines the information on terrain slope and aspect, and thus provides information on topographical shading effects. Tree cover density quantifies the direct shading effects of vegetation along the rivers. Both parameters help to consider the intensity of potential solar radiation onto the water surface.
Calculation of hillshade was based on the elevation and direction angle of the light source, i.e., based on the sun's position on the sky. We selected an angle of 60° as elevation (=summer) and 180° as direction (=South), representing an Austrian summer day (maximum elevation angle in Vienna is 65.2° on June 21st; Geoland.at., 2015b). The continuous hillshade values were classified into 7 categories by a decision tree (Chaid) with WTQ95 as the predicting variable.
Tree cover density was calculated based on the high-resolution layer for tree cover density (EEA, 2018). The density values were queried in a 5 km longitudinal buffer upstream along the river network and 50 m on each side of the river segment line. The derived mean tree cover density was grouped into six categories ranging from very low (≤6%) to very high (>71%).
Glaciated areas within the catchment were derived from the Austrian Glacier inventory 3 (Fischer et al., 2015). Summer cooling effects by glaciers were observed for rivers in several studies (Collins, 2009; Fellman et al., 2014; Williamson et al., 2019). Future scenarios for the development of central European glaciers were derived from Marzeion et al. (2012). The classification of the glacier effects was based on characteristic hydrological regimes of Austrian rivers (Mader et al., 1996; Table 2 and Table S1).
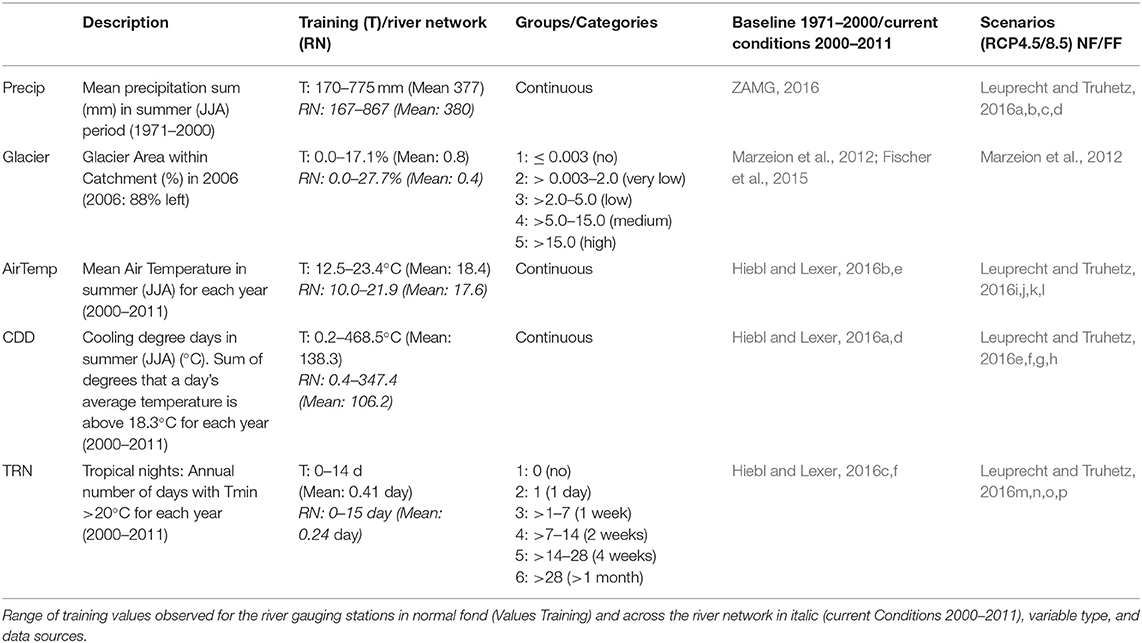
Table 2. Description of independent variables used in the water temperature model that change under climate change scenarios.
Discharge also plays a role in the thermal regime, but no adequate dataset was available for further modeling. Therefore, we tested correlations at the river gauging stations of summer precipitation amounts, low flow events and water temperature (WTQ95). Within the years 2000–2011 the mean discharge (MQ) and low discharge events (MNQ7) correlated very low with the water temperature with r = −0.04 and r = −0.05, respectively. Furthermore, the yearly amount of summer precipitation (Precep_Y) divided by the long-term amount of summer precipitation (Precep) correlated with the annual low flow events (MNQ7/MQ) by r = −0.42. We included precipitation to characterize the trend of changing hydrological conditions in our analyses as run-off models are majorly driven by precipitation as input, and another run-off model would further increase the uncertainties.
Classification of input variables was conducted to reduce the extrapolation effects of outliers in the prediction dataset not represented in the input dataset.
Thermal- and PKD-Related Risk for Brown Trout
We developed a risk assessment to identify both, (1) thermal regimes that support a higher PKD prevalence in brown trout, and (2) prospective thermal regimes that create thermally stressful conditions for S. trutta. Thus, critical temperature thresholds in respect of PKD outbreak and general thermal habitat suitability were identified through a literature review.
Overall, the most indicative parameters in the literature were based on consecutive days (further shortened as “ConsD”) and hours (further shortened as “ConsH”) exceeding specific mean water temperature values. In detail, a possible PKD outbreak is considered to appear at ≥14 ConsD over 15°C (Burkhardt-Holm, 2009). Low mortalities in field studies occurred at ≥29 ConsD over 15°C and a minimum of additional 10 non-consecutive days (≥15°C) following up to autumn (Schmidt-Posthaus et al., 2015). High mortalities with clinical signs of PKD appear at ≥26 ConsD over 18°C in laboratory conditions (Bettge et al., 2009a). These thresholds were assembled into four classes of temperature-related risk for PKD outbreak (TR-PKD): no outbreak (0), possible outbreak (1), low mortality (2), high mortality (3) (for more details see Table 3).
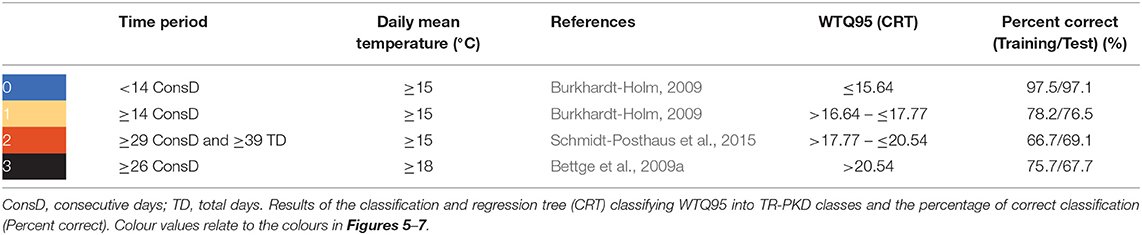
Table 3. The categories of temperature-related risk of PKD (TR-PKD) with related time periods exceeding daily mean water temperature thresholds (°C).
High water temperatures are not only threatening S. trutta in terms of emerging diseases, they also induce physiological stress. Critical temperature thresholds were therefore identified for diminished growth (water temperature ≥19.5°C; Elliott and Elliott, 2010) and for the occurrence of cardiac dysfunction in brown trout (water temperature ≥23.5°C; Vornanen et al., 2014). The temperature threshold for diminished growth was set when the 95% quantile was ≥19.5°C, expressing roughly 18 days with thermal conditions for diminished growths. As cardiac dysfunction in S. trutta can occur at single (hourly) events, the threshold for our model was an exceedance of the maximum hourly water temperature ≥23.5°C.
Water Temperature Modeling
Water temperature models were based on linear regression models that have been calibrated for four different river types ranging from small to large rivers (Table 1, Figure 2). The differentiation of river types was based on Strahler order. As dependent variable, we modeled the 95% quantile of daily mean water temperature (WTQ95). Accordingly, this value is indicating an exceedance of the given value by the 18 hottest days in the year.
The included independent variables were tested for multicollinearity based on the variance inflation factor (VIF <10). Homoscedasticity, linearity and normal distribution of residuals were checked visually based on scatter plots, histograms and distribution curves. The best models were selected by highest adjusted R2 values (Table 4).
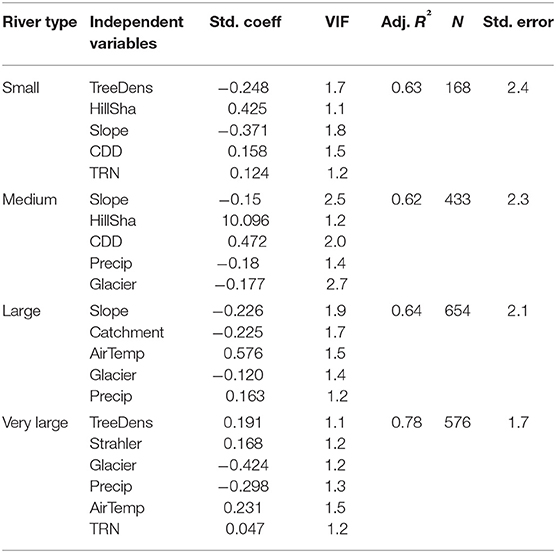
Table 4. Included variables in the linear regression models of the four rivers types (small to large rivers), including standardized coefficients (Std. Coeff), variance inflation factor (VIF), adjusted R2 (Adj. R2), the count (N), and the standard error (Std. Error).
As all the identified thresholds for TR-PKD were related to hot events, WTQ95 was tested to be suitable to predict TR-PKD. Therefore, a classification and regression tree (CRT), including a split-sample validation (training 70%, test 30%), was applied using TR-PKD as dependent and WTQ95 as independent parameter. The specifications for the CRT were a maximum tree depth of 5 and a minimum of 50/30 cases in parent/child node.
For the physiological thresholds a second CRT without split validation was applied using daily maximum temperatures ≥23.5°C [TD ≥23.5°C (max)] as dependent and WTQ95 as the independent parameter. The dataset for both CRTs included 3,000 values covering the years 2000–2015.
All statistical analyses were conducted in IBM SPSS 21 (IBM Corp., 2012).
Current, Baseline, and Future Climate
We used two data sources to describe the climate conditions in our analyses. The first dataset was used to describe the current conditions (period 2000–2011) that was also covered by the water temperature data from the gauging stations. The second dataset was used for future predictions including a baseline scenario and current conditions (Tables 1, 2). The spatial properties (grain size and coverage) were the same for both, i.e., covering the full investigation area with a 1*1 km resolution.
Future climate conditions originated from a national dataset (Chimani et al., 2016) that has been specifically established as a basis for climate change impact research in Austria. For future predictions, we used two timesteps (“near future” covering the period from 2021 to 2050, and “far future” covering 2071–2100) and two Representative Concentration Pathways (RCPs): RCP4.5 (“effective measures”) and RCP8.5 (“business as usual”). The RCPs describe different climate futures depending on the volume of greenhouse gases emitted in the years to come (IPCC, 2014). Among the different scenarios, RCP4.5 is a stabilization scenario and assumes that climate policies are invoked to achieve the goal of limiting emissions and radiative forcing. In turn, RCP8.5 is characterized by increasing greenhouse gas emissions over time, leading to high greenhouse gas concentration levels in absence of climate change policies. Compared to the total set RCPs corresponds to the pathway with the highest greenhouse gas emissions.
Results
The Current State of Temperature-Dependent PKD Risk and Thermal Stress for Brown Trout
The CRT predicted 86.5% of the WTQ95 into the correct TR-PKD class. The validation with 30% of the data showed similar results with 85.2% correct classification. The predicted thresholds are listed in Table 3. Both, ConsD ≥15°C and ≥18°C were highly correlated with WTQ95 by r = 0.85 and r = 0.71, respectively. Similarly, total days (TD) >15°C (r = 0.92) and >18°C (r = 0.8) showed high correlations with WTQ95. The limit for cardiac dysfunction (≥23.5°C) was exceeded in 455 cases between 2000 and 2015. The CRT showed that at WTQ95 >20.7°C, 99.1% of the river gauging stations (n = 232) had at least 1 day with maximum daily water temperature above 23.5°C (Figure S1).
Under current conditions (2000–2011) (Bas2011) the thermal habitat conditions for S. trutta were adequate in 98.2% of the river network (Figure 6). On average, no possible outbreak for PKD (TR-PKD = 0) was found in 72.8% of the river reaches. The TR-PKD increased with increasing Strahler order and ranged from 82.5% (TR-PKD = 0) in small rivers to 52.7% in very large rivers.
Water Temperature Modeling and Future Water Temperature
The input variables explained overall 68.9% of the variance in WTQ95 (Figure 3). The adjusted R2 ranged from 0.62 to 0.78 for small to large rivers (Table 4). The mean increases of WTQ95 ranged from +0.6 to 0.9°C in the near future and from +1.3 to 3.8°C in the far future compared to the current period 2000–2011 (Figure 4). In the baseline (1971–2000), mean WTQ95 was −0.7°C lower than in the current period 2000–2011.
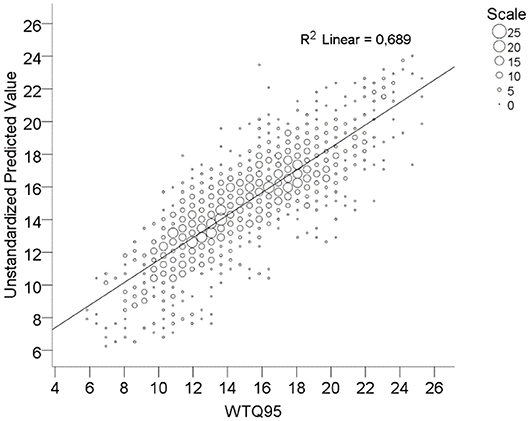
Figure 3. Linear relation of observed and predicted (unstandardized) WTQ95 values (n = 1,831) in (°C).
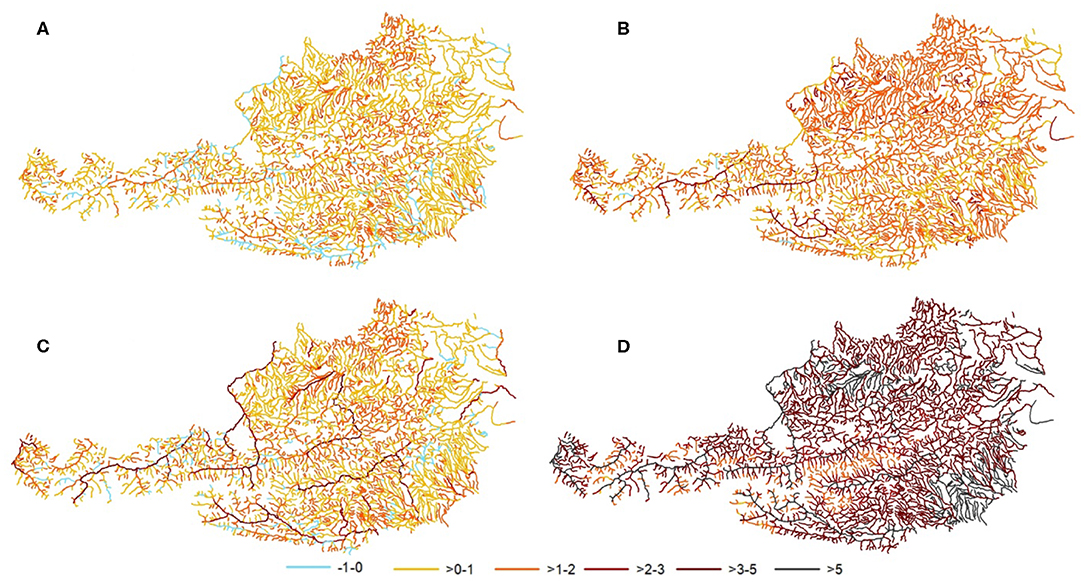
Figure 4. Signal of change of the water temperature model for WTQ95 compared to the current conditions (2000–2011) in degree Celsius (°C) for (A) near future RCP4.5, (B) far future RCP4.5, (C) near future RCP8.5, and (D) far future RCP8.5.
Future Changes of Temperature-Dependent PKD Risk and Thermal Stress for Brown Trout
The results of the modeling revealed severe changes in the thermal regimes of the rivers (Figure 5). The river reaches without a possible outbreak of PKD decreased from 72.8% (Bas2011) to 68.9–63.4% (RCP4.5–RCP 8.5) in near future and 60.1–37.8% in far future. In the past (1971–2000) 82.3% of the river length were without risk concerning possible outbreaks of PKD.
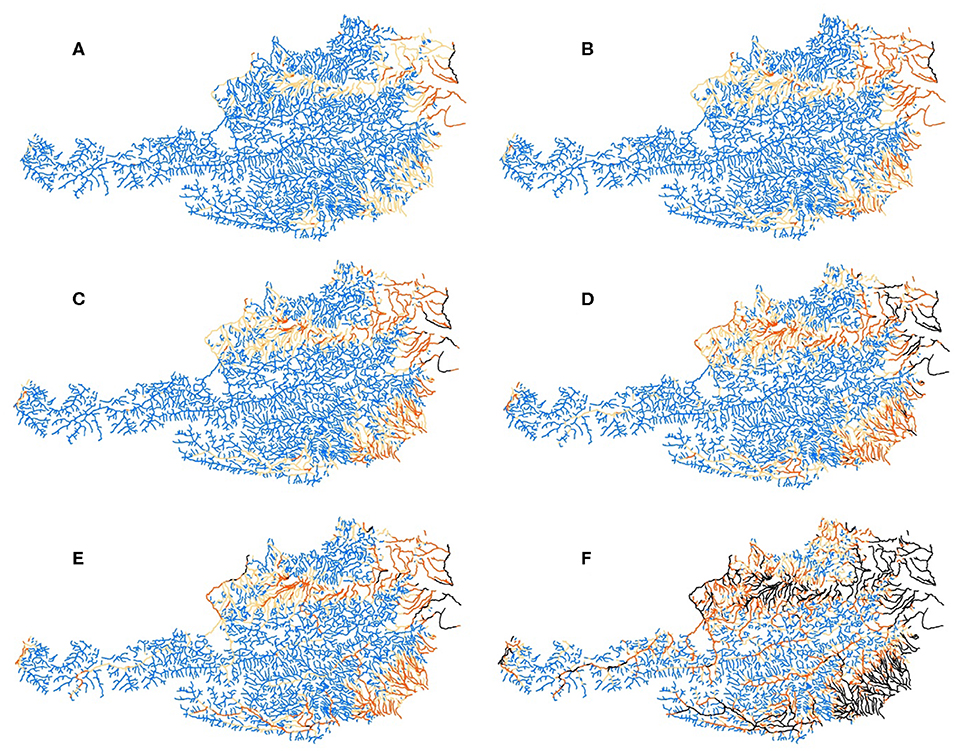
Figure 5. TR-PKD in Austrian rivers for (A) in the past (1971–2000), (B) under current conditions (2000–2011), as well as for the future scenarios (C) near future RCP4.5, (D) far future RCP4.5, (E) near future RCP8.5, and (F) far future RCP8.5. Color codes: No outbreak, blue; possible outbreak, orange; low mortality, red; and high mortality, black.
In small rivers (Strahler order = 3) the reaches without a risk of a possible PKD outbreak decreased from 82.5% (Bas2011) to 78.4–77.8% in near future, and to 71.3–52.8% in far future. The TR-PKD for low and high mortality ranged between 12.5–29.9% in far future compared to 4.8% under current conditions (2000–2011). The mean altitude of small rivers without a risk for PKD outbreak was 860–957 m.a.s.l (SD: 319.7–294.3) in far future compared to 808 m.a.sl (SD: 332) under current conditions. For medium-sized rivers the mean altitude was 896–1,035 m.a.s.l (SD: 283–238) in far future compared to 835 (SD: 301) under current conditions. This represents a shift of up to 200 m in altitude of segments without a risk for possible PKD outbreak.
The river reaches without suitable thermal habitat conditions for brown trout in respect of physiological thresholds (diminished growths) increased from 2.0% (2000–2011) to 2.9–5.3% in near future, and 5.3–31.9% in far future (Figure 6). Daily maximum temperature related to cardiac dysfunction increased from 0.1% in 2000–2011 to 0.5–1.2% in near future and to 2.4–22.3% in far future. For Strahler order 3 this effect is less pronounced with an increase from 0% for 2000–2011 to 0.3–0.4% in near future and 1.9–15.2% in far future (Table 5).
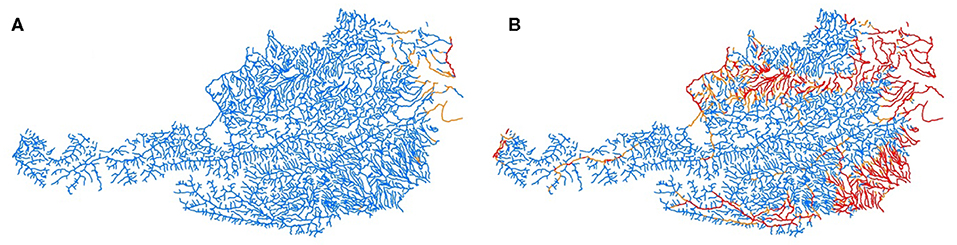
Figure 6. Characterization of thermal stress for brown trout in Austrian rivers (A) under current conditions (2000–2011), and (B) for the far future RCP8.5 scenario. Color codes: No stress, blue; diminished growth, orange; and cardiac dysfunction, red.
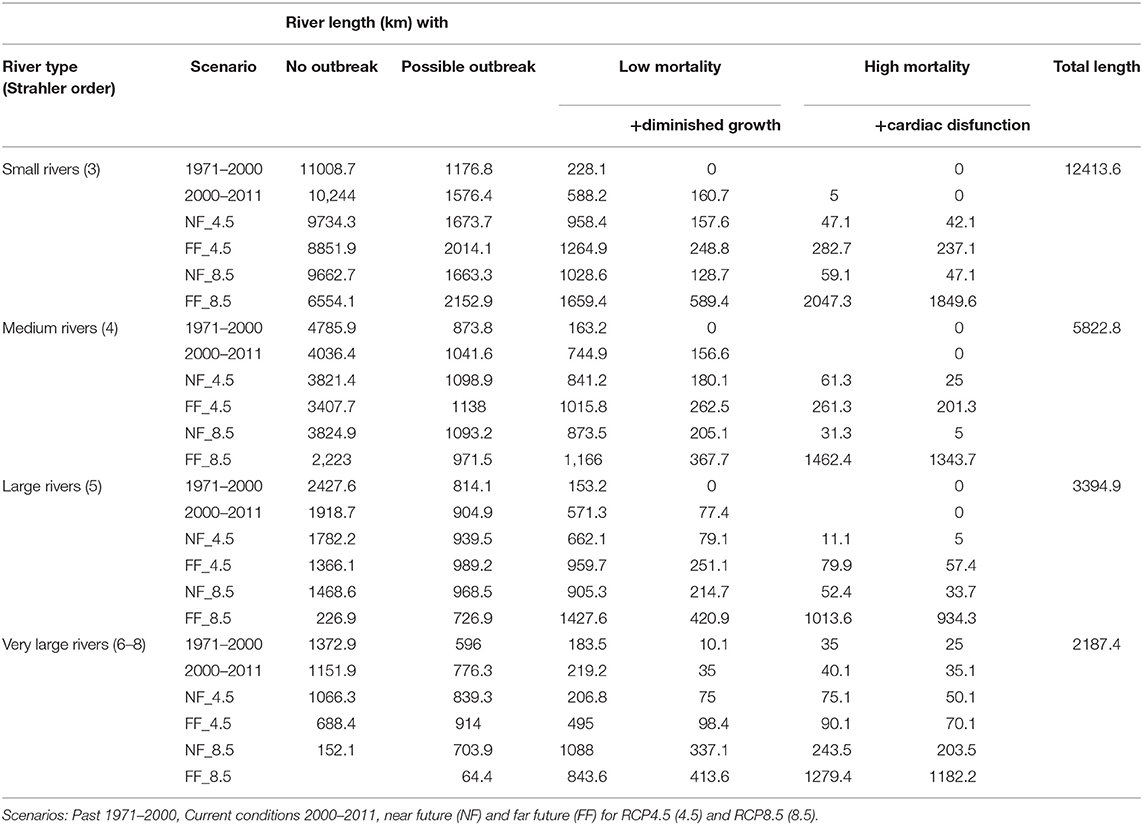
Table 5. River network (km) with combined effects of outbreak and mortality due to PKD and thermally stressful conditions for S. trutta.
Combined Effects
The combined effects of disease emergence and thermally stressful conditions for S. trutta showed a drastic increase especially under RCP 8.5 in far future (Figure 7). Combined effects of diminished growth and low mortality due to PKD (TR-PKD = 2) increased from 1.8% (2000–2011) to 2.1–3.7% in near future and to 3.6–7.5% in far future. The combined effects of cardiac dysfunction and high mortality due to PKD (TR-PKD = 3) increased from 0.1% (2000–2011) to 0.5–1.2% in near future and 2.4-22.3% in far future.
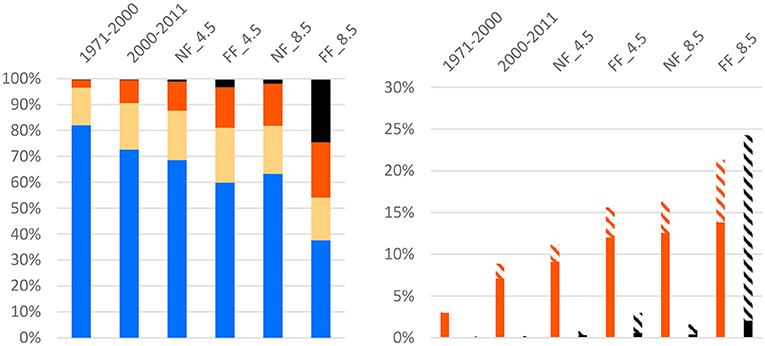
Figure 7. Shares of river reaches with different levels of TR-PKD in Austrian rivers; Left panel: No outbreak (blue), possible outbreak (yellow), low mortality (orange), high mortality (black). Combined effects of TR-PKD and physiological thresholds; Right panel: Low mortality and diminished growth (orange striped), high mortality and cardiac dysfunction (black striped). With Scenarios: Past = 1971–2000, Current conditions = 2000–2011, and abbreviations for the different scenarios: NF, near future; FF, far future; 4.5 = RCP4.5 and 8.5 = RCP8.5 (8.5).
In small rivers (Strahler order = 3) 237–1,850 km river length will be affected by combined impacts of high mortality due to PKD and cardiac dysfunction in far future compared to 0 km in 2000–2011 (Table 5). Additionally, 589 km river length of the total 1,659 km with TR-PKD = 2 (low mortality) showed a thermal regime with diminished growth in far future (RCP8.5). This indicates that within small rivers, that to a large extent (10,244 km), currently showed highly suitable thermal regimes the habitat suitability will be reduced by combined effects of PKD and physiological thresholds to 8,852–6,554 km for both RCP scenarios in far future.
Discussion
Our results highlight how thermal regimes are currently distributed in a pre-alpine to alpine river network and how the potential impacts of climate change will affect the emergence of PKD and increase thermal stress for brown trout. Here, we modeled water temperature of the rivers based on general river descriptors and climatic variables sensitive to climate change. Model calibration was based on a high-resolution long-term water temperature dataset covering a large spatial extent. Our regression model was able to explain nearly 70% of the variance in the observed water temperature (represented by WTQ95). This is in line with other regression models for water temperature, e.g., Arscott et al. (2001) modeled average daily summer temperature in a single alpine river catchment based on elevation, azimuth and water depth with R2 = 0.71. However, our dataset covers a much larger variety of rivers including four river size classes, and thus allows a broader view on how climate change will affect the thermal regimes of rivers in future.
Water Temperature Model
Our water temperature model covered three groups of main factors controlling the thermal regime of rivers: (1) topography, (2) atmospheric conditions, and (3) stream discharge (Caissie, 2006). In the different river size classes that were used for the modeling of the river water temperature these groups were described by different variables. For small rivers, topography was covered by the parameters riparian vegetation (TreeDens) and hillshade (HillSha) representing the shading effects on rivers. Atmospheric conditions were covered by two variables describing air temperature (CDD, TRN). The stream discharge and hydrologic conditions were reflected by precipitation (Precip) and river slope.
The model results showed that especially in small rivers shading effects played a crucial role in the variation of the water temperature compared to medium-sized and large rivers (Table 4) as the full river width can be covered by riparian vegetation or by surrounding hills. This is for example underlined by Hrachowitz et al. (2010) who found lowest summer maximum temperatures in small forested streams. Besides canopy, Arscott et al. (2001) found slope as an important variable for the diel temperature variation. We conclude that in small rivers that increasing the amount of riparian vegetation could strongly mitigate climate change effects, by cooling the water in downstream direction, respectively reduce the summation effect along the river network.
For small to large rivers, we found a general water temperature increase with a decrease in river slope. River slope can be used as a surrogate variable to describe the energy budget of the river, and thus characterizing the flow velocities. Basically, river slope is dependent on the terrain relief, and therefore highly correlated to altitude in alpine regions (r = 0.64). The effect of river slope and flow velocity on water temperature is related to the contact time of solar radiation with the water surface that is reduced in fast running rivers.
We also considered the effects of glaciers located further upstream in the river network that was found as an important descriptor in the thermal regime of medium-sized to large rivers. Larger glaciated areas within the catchment of a river reach led to lower water temperatures in line with several studies that observed summer cooling effects in rivers by glaciers (Collins, 2009; Fellman et al., 2014; Williamson et al., 2019). However, this cooling effect is related to a certain glaciated coverage within the catchment as Fellman et al. (2014) showed very low effects in river catchments with <2% glaciated area and very high effects with more than 30% of glaciated area.
The atmospheric conditions expressed by the air temperature variables (CDD, TRN, and AirTemp) were positively correlated with water temperature. Generally, the solar radiation is the direct energy input and driver of temperature increase for both, air and water. In our models, we used air temperature variables (CDD, TRN, and AirTemp) focusing on two aspects. Firstly, the average thermal conditions represented by mean temperature. Secondly, we aimed to describe heat waves during the summer period and thus implemented cooling degree days (CDD) and number of tropical nights (TRN). The variable cooling degree days describes hot conditions during daytime and the variable number of tropical nights characterizes nocturnal hot conditions. Especially in respect of heat stress and the emergence of diseases, the duration of heat events and the continuing exceedance of critical temperature thresholds is important for brown trout. Thus, it is important to consider the effects of less cooling during night as the thermal regimes of rivers normally cools during this daytime.
In respect of water quantity, precipitation was negatively correlated with water temperature in medium-sized and large rivers. As the relative yearly summer precipitation (Precep_Y/Precep) correlated negatively with the annual low flow (MNQ7/MQ) events, we conclude that especially summer precipitation influences summer low flow events in the rivers resulting in potential effects on the thermal regime. Furthermore, including elevation into the regression model would further increase the explanatory power of the model. However, this environmental descriptor only represents current conditions and stays stable in future and under climate change impacts.
Temperature-Dependent PKD Risk and Thermal Habitats of Brown Trout
Our approach to use a single water temperature parameter (WTQ95) to describe different heat periods in the thermal regime of rivers, was highly suitable. Exceedance periods of critical water temperatures are important to describe extreme events in the thermal regime. Turschwell et al. (2017) identified consecutive days above temperature thresholds as the best predictor for suitable habitats of a cold-water species. Using such a parameter, we were able to classify the river segments into different risk categories for PKD outbreak and physiological temperature stress. Accordingly, WTQ95 can be seen as a representative water temperature value for hot events and extreme hot events (e.g., ≥23.5°C) during summer.
Related to PKD, several authors describe the importance of warm periods in the emergence of the disease. On the one hand, life-cycle fulfillment of PKD is supported by warm temperatures, on the other hand, the progression of the disease itself in brown trout is more serious under warm conditions (Bettge et al., 2009a; Burkhardt-Holm, 2009; Gallana et al., 2013; Schmidt-Posthaus et al., 2015; Bruneaux et al., 2017). Furthermore, warm temperatures enhance the spore production in the bryozoan host, and therefore increase the infection rate (Tops et al., 2009; Okamura et al., 2011). Bryozoans, as the primary host, are organisms with a seasonal life-history; colonies increase in spring and expand prolifically during summer, leading to a peak of infective spore release and resulting in infection of trout (Gallana et al., 2013). Accordingly, Tops et al. (2009) expect that climate change will increase the geographic range of PKD as a result of the combined responses of T. bryosalmonae and its bryozoan hosts to warming and higher temperatures.
The combined effects of the disease and additional thermally stressful conditions for S. trutta are highly relevant, especially because of the weakened fitness of infected fish, the additional thermal stress on fish physiology, and an enforced immune response of fish in sub-optimal thermal conditions (Bowden, 2008; Bettge et al., 2009b). The proposed temperature-related risk assessment (TR-PKD) covers these major aspects to assess the potential spread and outbreak of PKD in S. trutta.
Future Scenarios of Water Temperature, Changes in PKD Risk, and Additional Physiological Stress for Brown Trout
Water temperature is highly linked to the same driving forces as air temperature. Thus, climate change will lead to further warming in rivers (Mohseni et al., 2003). This is in line with our results with a projected increase of WTQ95 ranging from +0.6 to +0.9°C in the near future, and from +1.3 to +3.8°C in the far future compared to the current conditions (Figure 4). Similarly, Dokulil (2018) analyzed long-term annual water temperature records of the Danube River and some tributaries (also represented in our dataset) indicating a temperature increase of about 0.3°C per decade since 1900.
Under both future concentration pathway scenarios (RCP4.5 and RCP8.5), our model showed an increase of temperature-related risk for PKD and a decrease of suitable thermal habitat for S. trutta. Interestingly, the distribution of PKD-risk for the near future under RCP4.5 is already comparable to the risk found under current conditions (2000–2011). This underlines the progressive and already observable change of the environmental conditions due to climate change. Not surprisingly, downstream sections of rivers are affected first by PKD as these river sections are generally warmer than the upstream parts. In Switzerland PKD-infected fish were mainly found below 800 m.a.s.l. (Wahli et al., 2008). This altitudinal threshold is in line with our findings with a mean altitude of 808 m.a.sl (SD: 332.1) for the risk class indicating no PKD outbreak.
In the far future RCP8.5 scenario, the amount of thermally suitable habitats for S. trutta will be drastically reduced. For small rivers that currently show highly suitable thermal regimes for a river length of more than 10,000 km, the length of river reaches with suitable habitats will be reduced to ~6,500 km due to the combined effects of PKD and physiological stress. The additional harm due to combined effects of high physiological stress (exceedance of thermal threshold for cardiac dysfunction) and potential high mortality due to PKD increases from 0.1% under current conditions up to 22.3% in the far future RCP8.5 scenario within the whole river network (Figure 7). In small rivers, this represents nearly 2,000 river kilometers with a total loss of thermally suitable habitats. This drastic reduction of habitats is in line with Filipe et al. (2013) who modeled a reduction of up to 64% of suitable stream reaches for brown trout in Europe till the 2080s due to climate change. Furthermore, a decline of S. trutta is already observed in the alpine region (Zimmerli et al., 2007).
The future distribution of S. trutta will be restricted to small and medium-sized alpine and pre-alpine rivers in Austria indicated by a elevational upward shift of around 200 m of rivers without the risk for a possible PKD outbreak in the far future RCP8.5 scenario compared to current conditions. Since the temperature thresholds for PKD-risk are below the physiological thresholds, the habitat reduction of S. trutta could be largely determined by the prevalence, outbreak and mortality due to PKD.
However, the results also underline that suitable habitats will remain in the alpine area, even under severe future climate change scenarios such as RCP8.5. This underlines the enormous importance of the alpine region as refuge for brown trout in Europe. Thus, it is highly important to firstly protect and restore existing habitats in these areas, and to secondly consider newly arising pressures on wild brown trout populations due to further human impacts and/or alterations induced by climate change.
The further habitat fragmentation can be a possible effect of climate change. The increasing number of unsuitable habitats also leads to disconnections of river sections that offer suitable habitats. Due to the loss of migration routes the populations can be further fragmented. This problem may be attenuated during the cold season as S. trutta could access areas in this time that are unsuitable during summer (Bentley et al., 2015). Furthermore, climate change will also lead to new land use patterns along rivers with potential impacts on wild brown trout populations. In this context the alpine habitats for brown trout must be conserved and managed in a careful and sustainable manner as in wide areas of Europe environmentally suitable habitats can be lost due to climate change (Filipe et al., 2013).
Our risk assessment approach represents a first step to gain more insights how PKD is distributed in rivers over large spatial extents. Thus, the risk assessment provides a basis to set up a screening and monitoring network. More insights into the temporal dynamics of parasite load and PKD symptoms in the wild are needed to further develop robust management and conservation strategies.
Data Availability Statement
The datasets generated for this study are available on request to the corresponding author.
Author Contributions
FB, GU, KW, ME-M, and TB contributed to the conception and design of this study. FB und TB developed the water temperature models and the risk assessment, and wrote the first draft of the manuscript. TB ran the analyses. FB, KW, and GU provided feedback on analysis. GU, SA, ME-M, and KW provided revisions and editing. All authors read and approved the submitted version.
Funding
This work was supported by the project ClimateTrout—Newly emerging impacts in riverine ecosystems: combined effects of climate change and malacosporean infections on brown trout (Project No. B670143) funded by the Austrian Climate Research Programme (ACRP) of the Klima-und Energiefonds.
Conflict of Interest
The authors declare that the research was conducted in the absence of any commercial or financial relationships that could be construed as a potential conflict of interest.
Acknowledgments
We thank two reviewers for their comments that helped to improve the manuscript. We also thank for the support of the BOKU Vienna Open Access Publishing Fund.
Supplementary Material
The Supplementary Material for this article can be found online at: https://www.frontiersin.org/articles/10.3389/fenvs.2020.00059/full#supplementary-material
References
Arscott, D. B., Tockner, K., and Ward, J. V. (2001). Thermal heterogeneity along a braided floodplain river (Tagliamento River, northeastern Italy). Can. J. Fish Aquat. Sci. 58, 2359–2373. doi: 10.1139/f01-183
Bentley, K. T., Schindler, D. E., Armstrong, J. B., Cline, T. J., and Brooks, G. T. (2015). Inter-tributary movements by resident salmonids across a boreal riverscape. PLoS ONE 10:e0136985. doi: 10.1371/journal.pone.0136985
Bettge, K., Segner, H., Burki, R., Schmidt-Posthaus, H., and Wahli, T. (2009a). Proliferative kidney disease (PKD) of rainbow trout: temperature- and time-related changes of tetracapsuloides bryosalmonae DNA in the kidney. Parasitology 136, 615–625. doi: 10.1017/S0031182009005800
Bettge, K., Wahli, T., Segner, H., and Schmidt-Posthaus, H. (2009b). Proliferative kidney disease in rainbow trout: time- and temperature-related renal pathology and parasite distribution. Dis. Aqu. Organ. 83, 67–76. doi: 10.3354/dao01989
BMLFUW (2017). eHYD. Vienna: Bundesministerium für Land- und Forstwirtschaft, Umwelt und Wasserwirtschaft.
Bowden, T. J. (2008). Modulation of the immune system of fish by their environment. Fish Shellfish Immunol. 25, 373–383. doi: 10.1016/j.fsi.2008.03.017
Bruneaux, M., Visse, M., Gross, R., Pukk, L., Saks, L., and Vasemägi, A. (2017). Parasite infection and decreased thermal tolerance: impact of proliferative kidney disease on a wild salmonid fish in the context of climate change. Function. Ecol. 31, 216–226. doi: 10.1111/1365-2435.12701
Burkhardt-Holm, P. (2009). Klimawandel und Bachforellenrückgang – gibt es einen Zusammenhang? Resultate aus der Schweiz. Environ. Sci. Eur 21, 177–185. doi: 10.1007/s12302-009-0043-7
Caissie, D. (2006). The thermal regime of rivers: a review. Freshwater Biol. 51, 1389–1406. doi: 10.1111/j.1365-2427.2006.01597.x
Chimani, B., Heinrich, G., Hofstätter, M., Kerschbaumer, M., Kienberger, S., Leuprecht, A., et al. (2016). ÖKS15 – Klimaszenarien für Österreich. Daten, Methoden und Klimaanalyse. Projektendbericht, CCCA Data Centre, Vienna.
Collins, D. (2009). “Seasonal variations of water temperature and discharge in rivers draining ice-free and partially-glacierised Alpine basins,” in 17th International Northern Research Basins Symposium and Workshop. 17 (Iqaluit, NU: Iqaluit-Pangnirtung-Kuujjuaq), 67–74.
Comte, L., Buisson, L., Daufresne, M., and Grenouillet, G. (2013) Climate-induced changes in the distribution of freshwater fish: observed predicted trends: climate change freshwater fish. Freshw. Biol. 58, 625–39. doi: 10.1111/fwb.12081
Coutant, C. C. (1977). Compilation of temperature preference data. Can. J. Fish. Aquat. Sci. 34, 739–745. doi: 10.1139/f77-115
Dokulil, M. (2018). Climate warming affects water temperature in the River Danube and tributaries– present and future perspectives. Geomorphologica Slovaca et Bohemica 18, 57–63.
EEA (2018). High Resolution Layer: Tree Cover Density (TCD) 2012 Copernicus Initial Operations 2011-2013 Land Monitoring Services High-resolution land cover characteristics of 5 main land cover types. European Environment Agency.
Ege, R., and Krogh, A. (1914). On the relation between the temperature and the respiratory exchange in fishes. Internal. Rev. Hydrobiol. Hydrograph. 7, 48–55. doi: 10.1002/iroh.19140070105
Elliott, J. (1976). The energetics of feeding, metabolism and growth of brown trout (Salmo trutta L.) in relation to body weight, water temperature and ration size. J. Anim. Ecol. 1976, 923–948. doi: 10.2307/3590
Elliott, J. M., and Elliott, J. A. (2010). Temperature requirements of Atlantic salmon Salmo salar, brown trout Salmo trutta and Arctic charr Salvelinus alpinus: predicting the effects of climate change. J. Fish Biol. 77, 1793–1817. doi: 10.1111/j.1095-8649.2010.02762.x
Fellman, J. B., Nagorski, S., Pyare, S., Vermilyea, A. W., Scott, D., and Hood, E. (2014). Stream temperature response to variable glacier coverage in coastal watersheds of Southeast Alaska. Hydrol. Process. 28, 2062–2073. doi: 10.1002/hyp.9742
Filipe, A. F., Markovic, D., Pletterbauer, F., Tisseuil, C., Wever, A., Schmutz, S., et al. (2013). Forecasting fish distribution along stream networks: brown trout (Salmo trutta) in Europe. Divers. Distrib. 19, 1059–1071. doi: 10.1111/ddi.12086
Fischer, A., Seiser, B., Stocker-Waldhuber, M., and Abermann, J. (2015). The Austrian glacier inventory GI 3, 2006 in ArcGIS (shapefile) format. In supplement to: Fischer, Andrea; Seiser, Bernd; Stocker-Waldhuber, Martin; Mitterer, Christian; Abermann, Jakob (2015): Tracing glacier changes in Austria from the little ice age to the present using a lidar-based high-resolution glacier inventory in Austria. Cryosphere 9, 753–766. doi: 10.5194/tc-9-753-2015
Fry, F. E. J. (1971). The effect of environmental factors on the physiology of fish. Fish Physiol.1971, 1–98. doi: 10.1016/S1546-5098(08)60146-6
Fürst, J., and Hörhan, F. (2005). “Einzugsgebietsgliederung,” in Digitaler Hydrologische Atlas Österreichs, 2. Lieferung, ed. Bundesministerium für Land- und Forstwirtschaft UuW (Wien: Österreichischer Kunst- und Kulturverlag).
Gallana, M., Ryser-Degiorgis, M.-P., Wahli, X., and Segner, H. (2013). Climate change and infectious diseases of wildlife: altered interactions between pathogens, vectors and hosts. Curr. Zool. 59, 427–437. doi: 10.1093/czoolo/59.3.427
Gorgoglione, B., Kotob, M. H., Unfer, G., and El-Matbouli, M. (2016). First proliferative kidney disease outbreak in austria, linking to the aetiology of black trout syndrome threatening autochthonous trout populations. Dis. Aqua. Organ. 119, 117–128. doi: 10.3354/dao02993
Hiebl, J., and Lexer, A. (2016a). ÖKS15 30y-mean of Temperature Indices: cdd_1971_2000_seasonal_mean, Version 1. Vienna: CCCA Data Centre.
Hiebl, J., and Lexer, A. (2016b). ÖKS15 30y-mean of Temperature Indices: tm_1971_2000_seasonal_mean, Version 1. Vienna: CCCA Data Centre, Vienna.
Hiebl, J., and Lexer, A. (2016c). ÖKS15 30y-mean of Temperature Indices: tr20_1971_2000_annual_mean, Version 1. Vienna: CCCA Data Centre, Vienna.
Hiebl, J., and Lexer, A. (2016d). ÖKS15 Temperature Climate Indices: cdd_1961_2011_seasonal, Version 1. Vienna: CCCA Data Centre.
Hiebl, J., and Lexer, A. (2016e). ÖKS15 Temperature Climate Indices: tm_1961_2011_seasonal, Version 1. Vienna: CCCA Data Centre.
Hiebl, J., and Lexer, A. (2016f). ÖKS15 Temperature Climate Indices: tr20_1961_2011_annual, Version 1. Vienna: CCCA Data Centre.
Hrachowitz, M., Soulsby, C., Imholt, C., Malcolm, I. A., and Tetzlaff, D. (2010). Thermal regimes in a large upland salmon river: a simple model to identify the influence of landscape controls and climate change on maximum temperatures. Hydrol. Process. 24, 3374–3391. doi: 10.1002/hyp.7756
ICPR (2013). Development of Rhine Water Temperatures Based on Validated Temperature Measurements Between 1978 and 2011. Koblenz: International Commission for the Protection of the Rhine, editor. Report No. 209.
IPCC (2014). “Climate change 2014: synthesis Report,” in Contribution of Working Groups I, II III to the Fifth Assessment Report of the Intergovernmental Panel on Climate Change, eds Core Writing Team, R. K. Pachauri, and L. A. Meyer (Geneva: IPCC), 151.
Jarić, I., Lennox, R. J., Kalinkat, G., Cvijanović, G., and Radinger, J. (2019). Susceptibility of European freshwater fish to climate change: species profiling based on life-history environmental characteristics. Glob. Change. Biol. 25, 448–58. doi: 10.1111/gcb.14518
Karvonen, A., Rintamäki, P., Jokela, J., and Valtonen, E. T. (2010). Increasing water temperature and disease risks in aquatic systems: climate change increases the risk of some, but not all, diseases. Int. J. Parasitol. 40, 1483–1488. doi: 10.1016/j.ijpara.2010.04.015
Leuprecht, A., and Truhetz, H. (2016a). ÖKS15 Climate Change Signal of Precipiation Indices: rr - rcp4.5 - far future - jja, Version 1. Vienna: CCCA Data Centre.
Leuprecht, A., and Truhetz, H. (2016b). ÖKS15 Climate Change Signal of Precipiation Indices: rr - rcp4.5 - near future - jja, Version 1. Vienna: CCCA Data Centre.
Leuprecht, A., and Truhetz, H. (2016c). ÖKS15 Climate Change Signal of Precipiation Indices: rr - rcp8.5 - far future - jja, Version 1. Vienna: CCCA Data Centre.
Leuprecht, A., and Truhetz, H. (2016d). ÖKS15 Climate Change Signal of Precipiation Indices: rr - rcp8.5 - near future - jja, Version 1. Vienna: CCCA Data Centre.
Leuprecht, A., and Truhetz, H. (2016e). ÖKS15 Climate Change Signal of Temperature Indices: cdd - rcp4.5 - far future - jja, Version 1. Vienna: CCCA Data Centre.
Leuprecht, A., and Truhetz, H. (2016f). ÖKS15 Climate Change Signal of Temperature Indices: cdd - rcp4.5 - near future - jja, Version 1. Vienna: CCCA Data Centre.
Leuprecht, A., and Truhetz, H. (2016g). ÖKS15 Climate Change Signal of Temperature Indices: cdd - rcp8.5 - far future - jja, Version 1. Vienna: CCCA Data Centre.
Leuprecht, A., and Truhetz, H. (2016h). ÖKS15 Climate Change Signal of Temperature Indices: cdd - rcp8.5 - near future - jja, Version 1. Vienna: CCCA Data Centre.
Leuprecht, A., and Truhetz, H. (2016i). ÖKS15 Climate Change Signal of Temperature Indices: tm - rcp4.5 - far future - jja, Version 1. Vienna: CCCA Data Centre.
Leuprecht, A., and Truhetz, H. (2016j). ÖKS15 Climate Change Signal of Temperature Indices: tm - rcp4.5 - near future - jja, Version 1. Vienna: CCCA Data Centre.
Leuprecht, A., and Truhetz, H. (2016k). ÖKS15 Climate Change Signal of Temperature Indices: tm - rcp8.5 - far future - jja, Version 1. Vienna: CCCA Data Centre.
Leuprecht, A., and Truhetz, H. (2016l). ÖKS15 Climate Change Signal of Temperature Indices: tm - rcp8.5 - near future - jja, Version 1. Vienna: CCCA Data Centre.
Leuprecht, A., and Truhetz, H. (2016m). ÖKS15 Climate Change Signal of Temperature Indices: tr20 - rcp4.5 - far future - annual, Version 1. Vienna: CCCA Data Centre.
Leuprecht, A., and Truhetz, H. (2016n). ÖKS15 Climate Change Signal of Temperature Indices: tr20 - rcp4.5 - near future - annual, Version 1. Vienna: CCCA Data Centre.
Leuprecht, A., and Truhetz, H. (2016o). ÖKS15 Climate Change Signal of Temperature Indices: tr20 - rcp8.5 - far future - annual, Version 1. Vienna: CCCA Data Centre.
Leuprecht, A., and Truhetz, H. (2016p). ÖKS15 Climate Change Signal of Temperature Indices: tr20 - rcp8.5 - near future - annual, Version 1. Vienna: CCCA Data Centre.
Lewisch, E., Unfer, G., Pinter, K., Bechter, T., and El-Matbouli, M. (2018). Distribution and prevalence of T. bryosalmonae in Austria: a first survey of trout from rivers with a shrinking population. J. Fish Dis. 41, 1549–1557. doi: 10.1111/jfd.12863
Mader, H., Steidl, T., and Wimmer, R. (1996). Abflußregime österreichischer Fließgewässer: Beitrag zu einer bundesweiten Fließgewässertypologie, Vol 82. Wien: Umweltbundesamt.
Magnuson, J. J., Crowder, L. B., and Medvick, P. A. (1979). Temperature as an ecological resource. Am. Zool. 19, 331–343. doi: 10.1093/icb/19.1.331
Markovic, D., Carrizo, S., Freyhof, J., Cid, N., Lengyel, S., Scholz, M., et al. (2014). Europe's freshwater biodiversity under climate change: distribution shifts and conservation needs. Divers. Distrib. 20, 1097–1107. doi: 10.1111/ddi.12232
Marzeion, B., Jarosch, A. H., and Hofer, M. (2012). Past and future sea-level change from the surface mass balance of glaciers. Cryosphere 6, 1295–1322. doi: 10.5194/tc-6-1295-2012
Mohseni, O., Stefan, H. G., and Eaton, J. G. (2003). Global warming and potential changes in fish habitat in US streams. Clim. Change 59, 389–409. doi: 10.1023/A:1024847723344
Okamura, B., Hartikainen, H., Schmidt-Posthaus, H., and Wahli, T. (2011). Life cycle complexity, environmental change and the emerging status of salmonid proliferative kidney disease. Freshwater Biol. 56, 735–753. doi: 10.1111/j.1365-2427.2010.02465.x
Rosgen, D. L. (1994). A classification of natural rivers. Catena 22, 169–199. doi: 10.1016/0341-8162(94)90001-9
Schmidt-Posthaus, H., Hirschi, R., and Schneider, E. (2015). Proliferative kidney disease in brown trout: infection level, pathology and mortality under field conditions. Dis. Aquat. Organ. 114, 139–146. doi: 10.3354/dao02855
Sterud, E., Forseth, T., Ugedal, O., Poppe, T. T., Jorgensen, A., Bruheim, T., et al. (2007). Severe mortality in wild Atlantic salmon Salmo salar due to proliferative kidney disease (PKD) caused by Tetracapsuloides bryosalmonae (myxozoa). Dis. Aquat. Organ. 77, 191–198. doi: 10.3354/dao01846
Tops, S., Hartikainen, H.-L., and Okamura, B. (2009). The effects of infection by Tetracapsuloides bryosalmonae (Myxozoa) and temperature on Fredericella sultana (Bryozoa). Int. J. Parasitol. 39, 1003–1010. doi: 10.1016/j.ijpara.2009.01.007
Turschwell, M. P., Balcombe, S. R., Steel, E. A., Sheldon, F., and Peterson, E. E. (2017). Thermal habitat restricts patterns of occurrence in multiple life-stages of a headwater fish. Fresh. Sci. 36, 402–414. doi: 10.1086/691553
Vornanen, M., Haverinen, J., and Egginton, S. (2014). Acute heat tolerance of cardiac excitation in the brown trout (Salmo trutta fario). J. Exp. Biol. 217, 299–309. doi: 10.1242/jeb.091272
Wahli, T., Bernet, D., Segner, H., and Schmidt-Posthaus, H. (2008). Role of altitude and water temperature as regulating factors for the geographical distribution of Tetracapsuloides bryosalmonae infected fishes in Switzerland. J. Fish Biol. 73, 2184–2197. doi: 10.1111/j.1095-8649.2008.02054.x
Waldner, K., Bechter, T., Auer, S., Borgwardt, F., El-Matbouli, M., and Unfer, G. (2019). A brown trout (Salmo trutta) population faces devastating consequences due to proliferative kidney disease and temperature increase: a case study from Austria. Ecol. Fresh. Fish. doi: 10.1111/eff.12528. [Epub ahead of print].
Webb, B. W. (1996). Trends in stream and river temperature. Hydrol. Process. 10, 205–226. doi: 10.1002/(SICI)1099-1085(199602)10:2<205::AID-HYP358>3.0.CO;2-1
Williamson, R. J., Entwistle, N. S., and Collins, D. N. (2019). Meltwater temperature in streams draining Alpine glaciers. Sci. Total Environ. 658, 777–786. doi: 10.1016/j.scitotenv.2018.12.215
Wimmer, R., and Moog, O. (1994). Flußordnungszahlen österreichischer Fließgewässer: Umweltbundesamt (Vienna).
ZAMG (2016). ÖKS15 Precipitation Climate Indices: rr_1961_2010_seasonal, Version 1. Vienna: CCCA Data Centre.
Keywords: river, Salmo trutta, water temperature, proliferative kidney disease, Alps, thermal niche
Citation: Borgwardt F, Unfer G, Auer S, Waldner K, El-Matbouli M and Bechter T (2020) Direct and Indirect Climate Change Impacts on Brown Trout in Central Europe: How Thermal Regimes Reinforce Physiological Stress and Support the Emergence of Diseases. Front. Environ. Sci. 8:59. doi: 10.3389/fenvs.2020.00059
Received: 11 February 2020; Accepted: 28 April 2020;
Published: 27 May 2020.
Edited by:
Jonas Schoelynck, University of Antwerp, BelgiumReviewed by:
Teresa Ferreira, University of Lisbon, PortugalGuillaume Grosbois, Swedish University of Agricultural Sciences, Sweden
Copyright © 2020 Borgwardt, Unfer, Auer, Waldner, El-Matbouli and Bechter. This is an open-access article distributed under the terms of the Creative Commons Attribution License (CC BY). The use, distribution or reproduction in other forums is permitted, provided the original author(s) and the copyright owner(s) are credited and that the original publication in this journal is cited, in accordance with accepted academic practice. No use, distribution or reproduction is permitted which does not comply with these terms.
*Correspondence: Florian Borgwardt, florian.borgwardt@boku.ac.at