- 1Science Systems and Applications, Inc., Consultant, Diamondhead, MS, United States
- 2NASA GSFC Hydrological Sciences Branch, Greenbelt, MD, United States
- 3Science Applications International Corporation, Hydrological Sciences Laboratory, NASA Goddard Space Flight Center, Greenbelt, MD, United States
- 4Spatial Sciences Laboratory, Texas A&M University, College Station, TX, United States
- 5Department of Engineering Systems and Environment, University of Virginia, Charlottesville, VA, United States
The Lower Mekong Basin (LMB) is biologically diverse, economically important, and home to about 65 million people. The region has undergone extensive environmental changes since the 1990s due to such factors as agricultural expansion and intensification, deforestation, more river damming, increased urbanization, growing human populations, expansion of industrial forest plantations, plus frequent natural disasters from flooding and drought. The Mekong river is also heavily used for human transportation, fishing, drinking water, and irrigation. This paper discusses use of pre-existing LULC maps from 1997 and 2010 to derive a LMB regional LULC change map for 9 classes per date using GIS overlay techniques. The change map was derived to aid SWAT hydrologic modeling applications in the LMB, given the 2010 map is currently used in multiple LMB SWAT models, whereas the 1997 map was previously used. The 2010 LULC map was constructed from Landsat and MODIS satellite data, while the 1997 map was from before the MODIS era and therefore based on available Landsat data. The 1997–2010 LULC change map showed multiple trends. Permanent agriculture had expanded in certain sub-basins into previously forested areas. Some agricultural areas were converted to industrial forest plantations. Extensive forest changes also occurred in some locations, such as areas changed to shifting cultivation or permanent crops. Also, the 1997 map under classified some urban areas, whereas the 2010 LULC map showed improved identification of such areas. LULC map accuracy were assessed for 213 randomly sampled locations. The 1997 and 2010 LULC maps showed high overall agreements with reference data exceeding 87%. The LULC change map yielded a moderately high level of overall agreement (78%) that improved to ∼83% once LULC classification scheme specificity was reduced (forests and agriculture were each mapped as singular classes). The change map regionally showed a 4% decrease in agriculture and a 4% increase in deciduous and evergreen forests combined, though deforestation hot spot areas also were evident. The project yielded LULC map data sets that are now available for aiding additional studies that assess LMB LULC change and the impacts such change may pose to water, agriculture, forestry, and disaster management efforts. More work is needed to map, quantify and assess LULC change since 2010 and to further update the 2010 LULC map currently used in the LMB SWAT models.
Introduction
The Lower Mekong Basin (LMB) is a region in the southeast Asia known for its agriculture, forests, fisheries, wildlife, and diverse, natural ecosystems [Mekong River Commission MRC (2010)]. About 65 million people live in this region, depending on the Mekong River and its tributaries for transportation, water for drinking and bathing, fishing, and agricultural irrigation. The MRC (2014) reports that agriculture is the most important economic activity in the LMB with rice cultivation occurring on millions of hectares.
The LMB human inhabitants are experiencing multiple environmental threats to their ways of life that include land use land cover (LULC) change (e.g., from river damming, agricultural expansion, and deforestation) and frequent natural disasters (e.g., flooding from severe storms, and drought). The drivers of LULC change in the region include anthropogenic (e.g., economic and demographic) and environmental factors (Tran et al., 2015). Both negative and positive forest change drivers occur, which can collectively affect the amount of agricultural expansion and contraction, well as the amount of deforestation and afforestation (Costenbader et al., 2015a, b; Imai et al., 2018). The causes (i.e., drivers) of LULC change in the Mekong region are multifaceted with the prices of agricultural and forest products, road accessibility, and changes in land titles identified as important drivers of LULC change (Rowcroft, 2008; Xing, 2013). Other drivers of LULC change in the Mekong include anthropogenic drivers such as the construction of large-scale hydropower dams and other abiotic drivers such as climate change (e.g., sea level rise) (Keskinen et al., 2010; Evers and Pathirana, 2018). Subsidence in conjunction with ground water extraction and sea level rise is another driver negatively affecting coastal LMB agriculture (Minderhoud et al., 2018). Climate change in the LMB is regarded as a threat to agriculture (MRC, 2010; MRC, 2014; Pokhrel et al., 2018a) and forests (Estoque et al., 2019).
Such LULC change drivers can affect human settlements and livelihoods, as well as the natural resources that are depended upon by the inhabiting humanity, wildlife, fisheries and other valued wild and domestic life forms (MRC, 2010). The management and sustainability of water resources in the region is particularly of great concern to people in the LMB, given a growing number of hydrologic modifications along the main waterways (e.g., river damming), increased demand on water for irrigation from agricultural intensification, expansion of agriculture into areas formerly occupied by forest, and a growing human population (MRC, 2010; Pokhrel et al., 2018a). In addition, meteorological disasters such as severe droughts and flooding events further threaten regional inhabitants and their economic activities, such as agriculture, forestry, commercial fishing, and transportation (Ribbe et al., 2013).
The LMB and the Mekong region at large has reportedly undergone substantial LULC change in recent decades (Lyon et al., 2017; Yasmi et al., 2017; Pokhrel et al., 2018b), though questions and uncertainties remain as to the location and geospatial extent for specific kinds of LULC change. An assortment of satellite data has been used to map and quantify LULC change in the region, including AVHRR (Giri et al., 2003), Landsat data (Heinimann et al., 2007), SPOT-4 Vegetation (Stibig et al., 2004), and MODIS data (Leinenkugel et al., 2015). Unfortunately, it is difficult to effectively compare results from such change maps given that they can differ in terms of the kind of satellite data used, the observed time span, the methods for generating and validating such products, the LULC change classification scheme employed, spatial resolution of the map, the geographic domain covered by the map, the objectives of the mapping project, and the organizations responsible for making LULC maps. Some of these issues are discussed by Patil and Gumma (2018) with respect to updating south Asia cropland and other land cover types. The challenges arising from the differences in LULC mapping methods may be addressed in part by comparing provenance of geospatial workflows (Tullis et al., 2015). Note that the LULC maps referred to in this paper are digital geospatial data sets as opposed to cartographic hardcopy mapping products.
To help address water, disaster management, and crop security concerns, the Mekong River Commission has been using the Soil and Water Assessment Tool (SWAT) framework (Gassman et al., 2007) for hydrologic modeling in the non-coastal portion of the LMB, including sub-basins 1–8, as discussed by Rossi et al. (2009). The LULC map is a key input parameter to the SWAT framework, given the effect that an area’s LULC characteristics can have on surface water flow and runoff. The initial MRC SWAT model employed a LULC map that was based on 1997 Landsat data (Dat, 2013). More recently, MRC SWAT models were updated with a 2010 LULC map for the LMB that was developed using a combination of monthly MODIS NDVI and Landsat data (Spruce et al., 2018). The updated map included more detailed agricultural LULC classes in order to revise and improve SWAT hydrologic modeling products and applications (Mohammed et al., 2018a, b).
The purpose of the project discussed in this paper was to map, quantify and assess spatio-temporal changes in non-coastal LMB LULC that occurred from 1997 to 2010, using pre-existing LULC map data sets as inputs. This work aimed to assess apparent LULC changes during the observed time frame mainly with respect to forest and agricultural areas. Such information was needed to augment understanding and interpretation of LULC effects on SWAT modeling of runoff using either the 2010 or 1997 data. The LULC patch mosaic can either accelerate or impede the runoff depending on the characteristics of LULC, including the location and extensiveness of LULC types. Although the runoff characteristics of common LULC types are generally known (USDA, 1986; Hong and Adler, 2008), an assessment of LULC change for the updated versus previous LULC map datasets was needed to help resource management organizations (e.g., the MRC and the Asian Disaster Preparedness Center or ADPC) assess SWAT modeled hydrologic response of surface runoff in the LMB, especially in watershed reaches with extensive LULC change. In doing so, such an assessment could help further document and understand the provenance of the LMB SWAT models.
There are several published reviews of raster imagery-based two date change detection methods on the use of remotely sensed data or pre-existing map datasets from remotely sensed data (Mas, 1999; Coppin et al., 2004; Jensen et al., 2012; Hussain et al., 2013). These methods include: (1) image ratioing; (2) image differencing; (3) Principal Components Analysis (PCA); (4) multi-date classification; and (5) Change Vector Analysis (CVA); and (6) post classification comparison. The choice of method can depend on such factors as the input data used for deriving the change map dataset and the kind of change map (e.g., general forest change or more specific LULC change). Excluding the post-classification method, most change detection mapping methods require that remotely sensed imagery from two or more dates as input to a data processing workflow in order to derive a change map.
The post-classification method is the main method used to derive LULC change maps from pre-existing LULC map digital datasets. In doing so, Geographic Information System (GIS) methods (e.g., additive overlays or indexing techniques) are employed to integrate the two LULC map datasets into a digital change map. Jensen et al. (2012) reported that post classification comparison is one of the more commonly used change detection methods. Also termed the delta classification technique, the post classification comparison method has the advantage of not requiring that remote sensed data be acquired and then processed into a digital change map. Post classification change map datasets are also relatively easy to calculate and yield “from to” change information that resource managers can usually understand (Wang et al., 2009). However, the accuracy of this method depends heavily on the accuracy of the two input LULC map datasets. The accuracy of a post classification comparison-derived change map dataset can approximate the product of the accuracies of the 2 input LULC digital maps (e.g., each map scaled on a 0 to 1 floating point scale) (Coppin et al., 2004). This can but not necessarily result in a low accuracy change map dataset. However, the same kind of change detection errors can also occur with change maps derived with methods other than the post classification comparison technique. For example, change maps regardless of method can include thematic mapping errors due to misregistration (i.e., geo-positional or locational) errors in the input data sets used to derive the digital change map. Also, the post classification method can produce superior change map datasets. For example, Mas (1999) conducted a study comparing different change mapping methods, observing that the post classification method yielded the best results. The post classification method is also employed as a method used in part to compute operational, digital LULC mapping products, including the USGS National Land Cover Database (NLCD) (Yang et al., 2018) and NOAA Coastal Change Analysis Program (C-CAP) (McCombs et al., 2016) LULC datasets.
All maps of LULC and LULC change are imperfect and have biases (e.g., classification and positional errors) and uncertainties about the quality of such products (Verberg et al., 2011). The biases (i.e., errors) that occur for a given LULC change map data product can depend on kind and quality of remotely sensed data, how it is processed, minimum mapping unit, classification scheme, characteristics of the study area, and other factors (Jensen et al., 2012; Congalton, 2015). Such imperfections can limit and pose challenges on how digital LULC maps should be used, depending on the application. LULC and LULC change map accuracy assessments can provide information on the quality of such map data set and how to effectively use these products.
Materials and Methods
Study Area Description
The LMB study area is in southeast Asia that includes portions of Laos, Myanmar, Thailand, and Vietnam, and Cambodia (Figure 1). The area includes 8 sub-basins (SBs) discussed by Rossi et al. (2009) that are used in the MRC SWAT modeling applications. This location includes extensive areas agricultural and forested land uses in addition to locally present land uses (e.g., urban). The study area includes most of the interior LMB, excluding the most coastal portion (e.g., Tonle Sap Basin and Mekong Delta). The study area has a tropical climate with dry and wet seasons, an average maximum temperature of 20.4 to 33.6°C, and a mean annual precipitation of ∼900 to 3300 mm, based on data from International Centre for Environmental Management (ICEM, 2013) study that is hosted on a Greater Mekong Subregion Environment Operations Center (GMS-EOC) Information Portal (GMS-EOC, 2019). The study area mostly has a moderately high or high hydrologic runoff potential, according to a 250 m hydrologic soil group (HSG) map by Ross et al. (2018). Elevations in the study area varies from ∼9 to 2,433 m, based on a 90-m Digital Elevation Model (DEM) data from the Shuttle Radar Topography Mission (SRTM) (NASA and JPL, 2013).
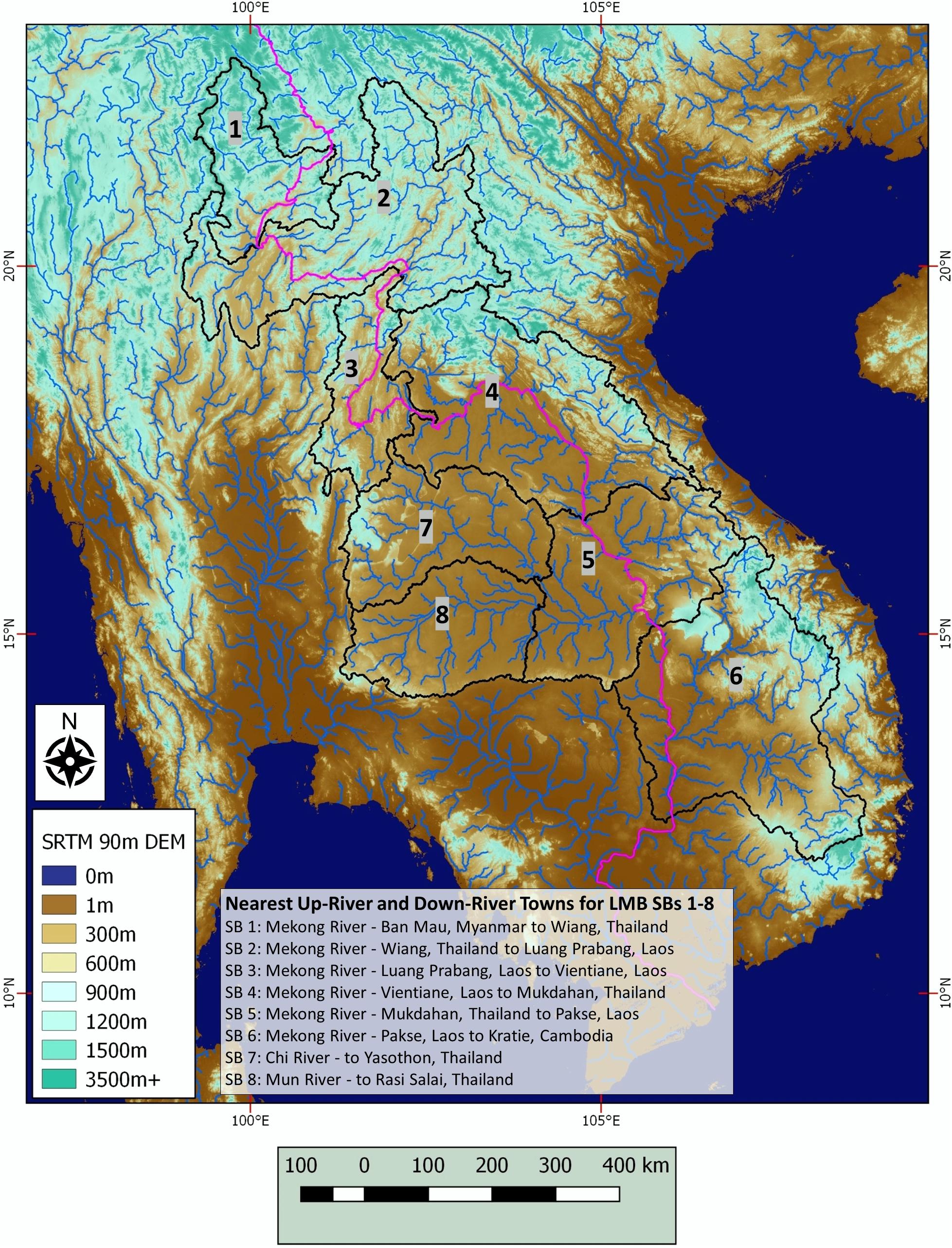
Figure 1. Study area location in Lower Mekong Basin of southeast Asia with Sub-basins (SBs) 1–8 (in black) along with river boundaries (blue) overlain onto a colorized 90 m digital elevation model (DEM) from the NASA Shuttle Radar Topography Mission (SRTM). The main branch of the Mekong River is shown in a magenta tone and the SB #s are included as black text.
Data Acquisition
The project used pre-existing LULC GIS data layers for the 1997 and 2010 time periods to generate the needed LULC change map. Acquired from the MRC as a vector data layer, the 1997 LULC map was derived from image interpretation, delineation, and digitization of LULC patches from 1:250,000 scale hard copies of Landsat Thematic Mapper imagery (Malyvanh and Feldkotter, 1999). The 2010 LULC map dataset was obtained from a previous project discussed by Spruce et al. (2018) that used a combination of ∼quarter kilometer resolution 2010 MODIS 8-day reflectance data from the LP-DAAC web site1 and single date circa 2010 dry season Landsat Thematic Mapper reflectance data at 30 m resolution from the USGS GLOVIS web site2. For this project, the 1997 and 2010 LULC maps were nominally gridded to a ∼231.66-m resolution.
To aid the project’s change detection product validation, additional Level-1 Landsat data for 1997 and 2010 was acquired from the USGS Earth Explorer3 and GLOVIS web sites. The LULC change map derivation and assessment also made use of other LMB LULC data acquired from the MRC, including circa 2010 field survey data (Nam et al., 2015), an alternative circa 2010 LULC map from Landsat data (Kityuttachai et al., 2016), and crop calendars (Halcrow Group Limited, 2004).
Data Processing and Analysis
To compute the needed LULC change map, the LULC maps for the 1997 and 2010 time periods were recoded into 9 LULC classes per date (Table 1). The 1997 map (Figure 2) from the MRC were coded into intervals of 10 and the 2010 map (Figure 3) were coded into the intervals of 1. This recoding strategy enabled the two input maps to be effectively combined into a change map via the QGIS Raster Calculator (by adding the two LULC maps together). The recoded LULC maps were first aligned with each other in QGIS and then added together to derive the LULC change map (Figure 4). In doing so, change was calculated only for pixels that were classified as having terrestrial (non-water) LULC classes on both input dates.
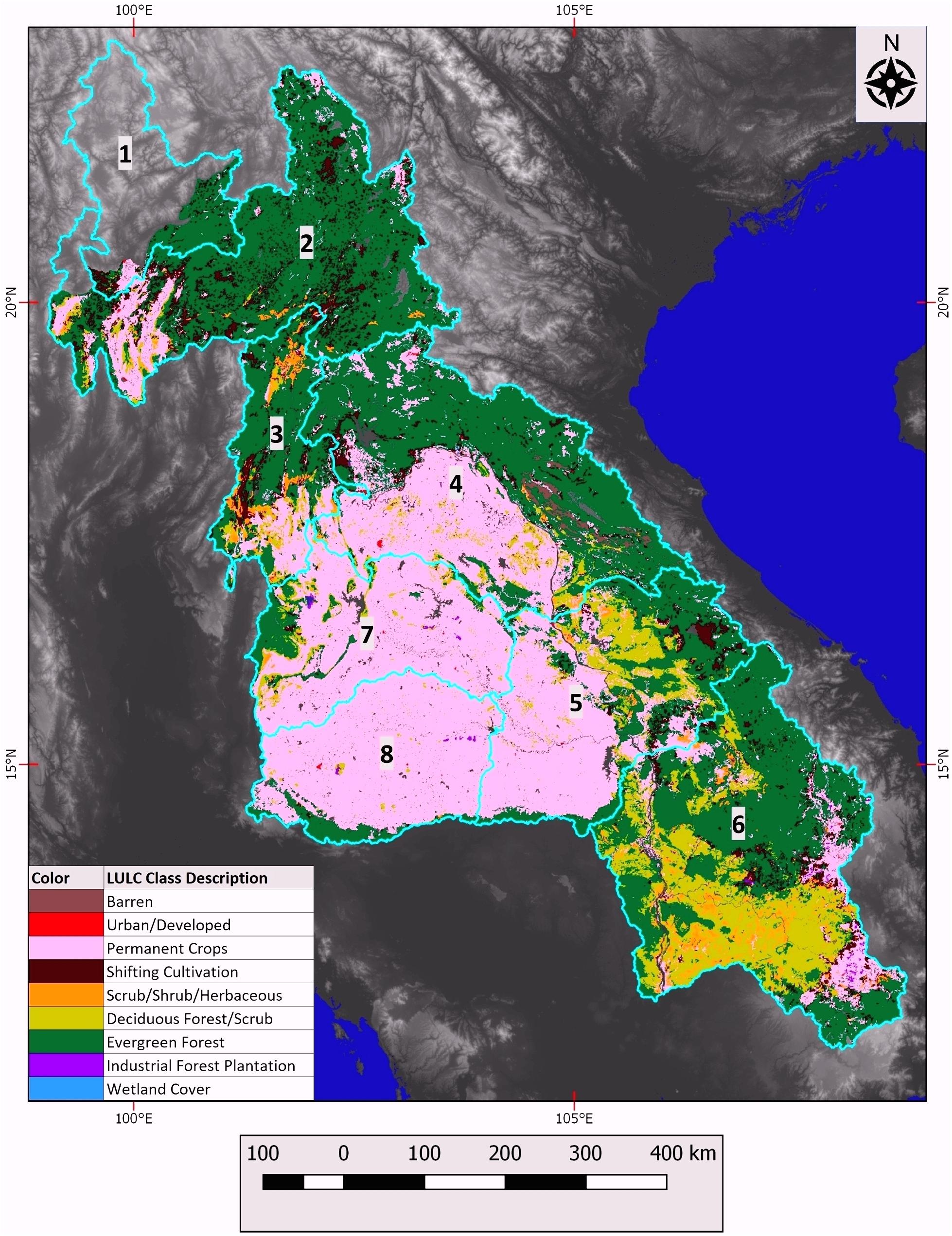
Figure 2. Study area 1997 LULC map with SB boundaries overlain in cyan for SBs 1–8. The SB #s are included as black text. The backdrop image adjacent to the LULC map is a 90 m DEM from SRTM data.
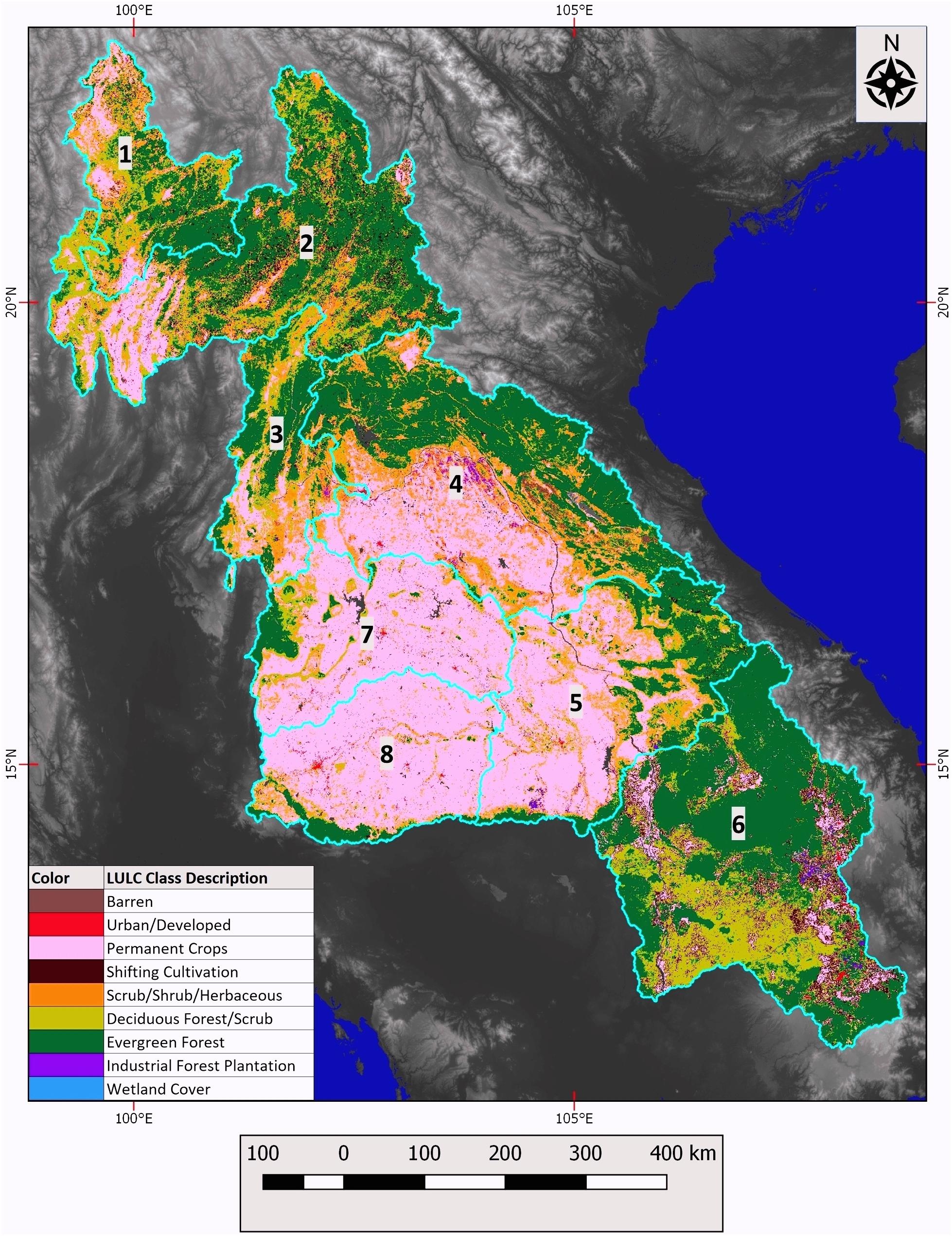
Figure 3. Study area 2010 LULC map with SB boundaries overlain in cyan for SBs 1–8. The SB #s are included as black text. The backdrop image adjacent to the LULC map is a 90 m DEM from SRTM data.
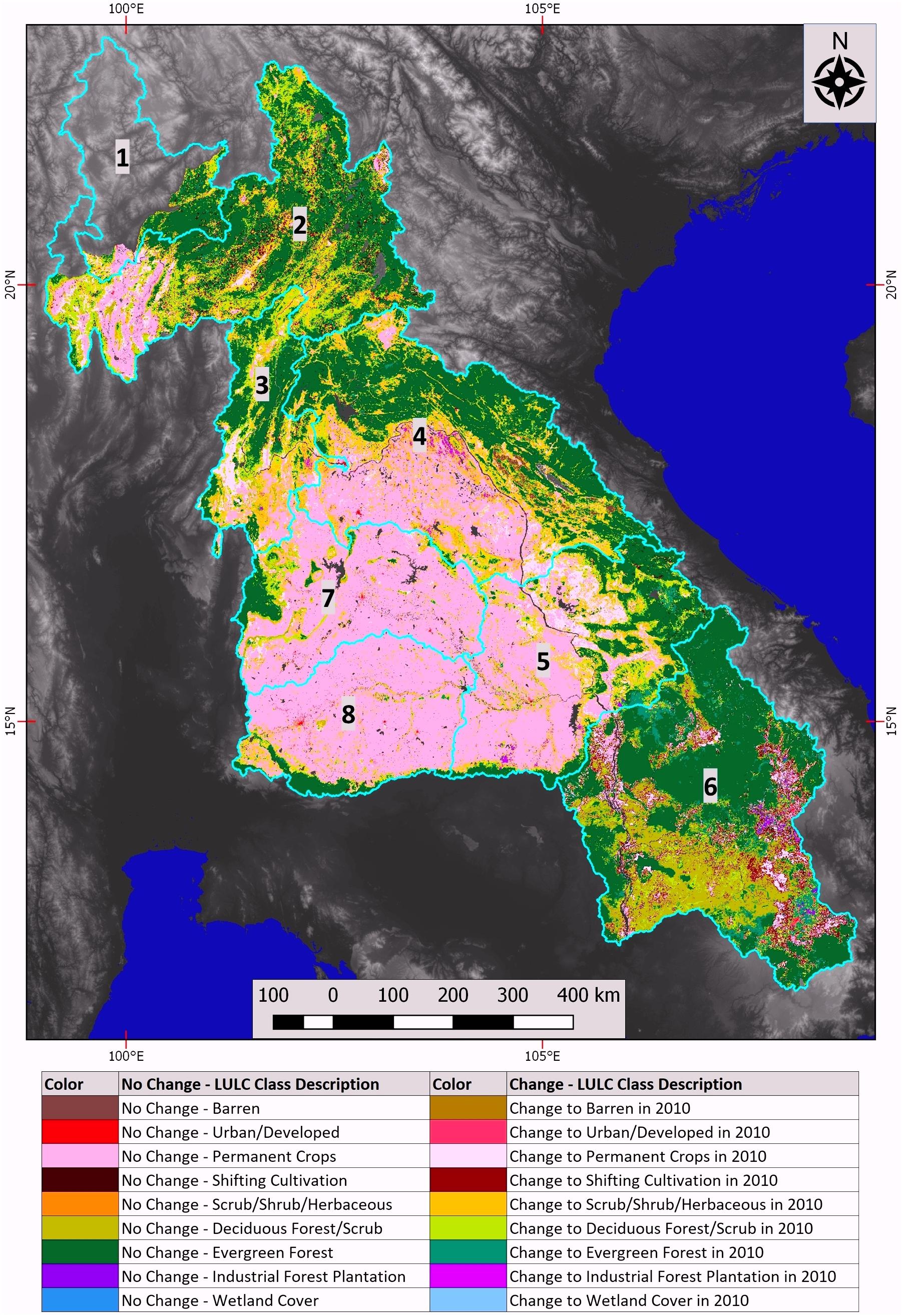
Figure 4. 1997–2010 LULC change map for study area with SB boundaries overlain in cyan for SBs 1–8. All LULC change classes are colorized to show the LULC class for the end date of 2010, as well as whether the class was of a “change” or “no-change” type, given the change map consists of both change and “no change” classes. For a given LULC category, the “change” class color was assigned a lighter color than the “no change” class (e.g., barren no change was set to dark brown, while change to barren was set to light brown). The SB #s are included as black text. The backdrop image adjacent to the LULC map is a 90 m DEM from SRTM data.
The LULC change map was then assessed for classification accuracy, considering guidance from Congalton (1991, 2015), Congalton and Green (2009), and Olofsson et al. (2013, 2014). The goal of the map validation was to assess the overall accuracy of the LULC change map compared to available reference data. In doing so, the LULC change map dataset was reprojected from geographic coordinates to the UTM map projection and then summarized in terms of pixel frequency for each LULC change class. For this analysis, a stratified random sampling of pixels was then drawn from the LULC change map using the QGIS (QGIS, 2017) and its plugin “AcATaMa” with the number of pixels drawn per class based on the proportion of the LULC class pixel frequency relative to the total number of mapped pixels. The adjacent pixels around each candidate sample pixel were considered in the random selection process. Candidate pixels were selected when they were the center pixel of a 3 by 3 pixel window and that most of the pixels in that window (5 or more) were of the same LULC class as that in the center pixel. In all, a total of 213 pixels were selected for map accuracy assessment. The frequency of occurrence and the number of samples selected per LULC change class is given for the 25 most common classes in Table 2.
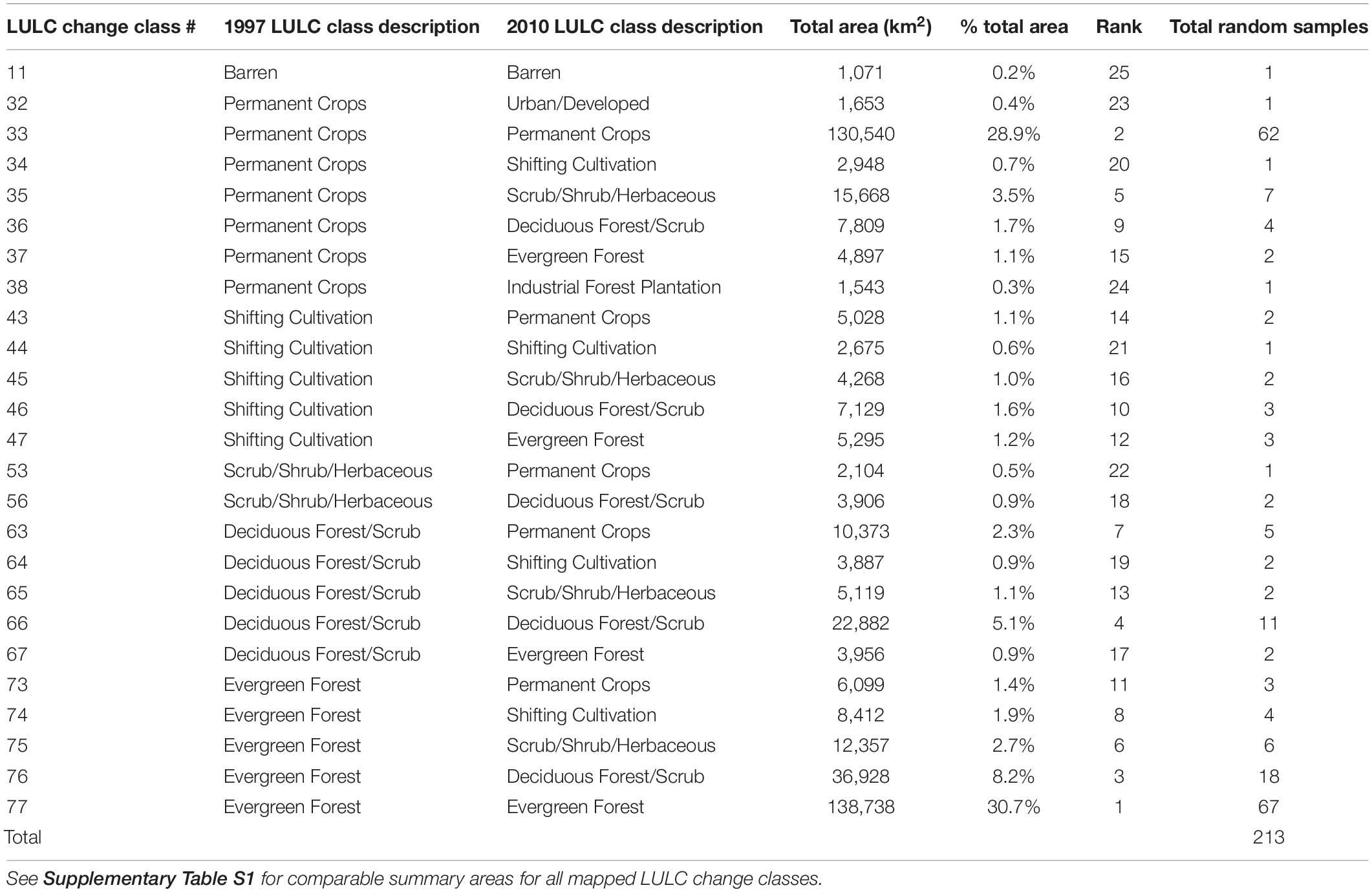
Table 2. Total area for the 25 most common change classes of the 1997–2010 LULC change map, along with # samples drawn per class.
Each selected sample location was then assessed by a trained, experienced image analyst to identify the LULC class for 1997 and 2010 time periods using assorted reference data that included Landsat false color RGBs and higher spatial resolution imagery from Bing and Google via the QGIS OpenLayers plugin. We considered using the Collect Earth tool (Bey et al., 2016; Saah et al., 2019a) for accuracy assessment, though selected QGIS for this task mainly due to its image enhancement, vector grid overlay, vector editing, and the “AcATaMa” accuracy assessment plugin capabilities. Within QGIS, some of the random sample areas were also reviewed on various dates of high-resolution true color imagery resident to the Google Earth Pro software. The sample locations were also compared and assessed with respect to other digital LULC mapping products primarily from the MRC, along with descriptive information on LULC classes from the MRC. In addition, a vector-based grid map of each pixel’s boundaries was superimposed on remote sensing imagery products. Each sample was image interpreted at a 1:6,000 scale which enables viewing individual applicable Landsat pixels of 30 m resolution (∼8 × 8 or 64 pixels) located within a given LULC change map pixel of a nominally 231.66 m spatial resolution. The apparent LULC class was assessed separately for the 1997 and 2010 time periods. For each sampled location, the reference LULC for each date was then additively combined using MS Excel into a change map score that was then compared to the test LULC change map to determine agreement or disagreement.
The assessment results were then used to summarize the percent overall agreement between the 1997–2010 LULC change map and reference datasets. An error matrix was constructed (Supplementary Table S2) to compute the percent overall accuracy for the LULC change map and the two LULC maps. This matrix given in basic form differs from guidelines of Olofsson et al. (2014), who suggested also including information on the percent area that a given LULC class represents in relation to the entire mapped area. This information on the percent of total area per LULC class is alternatively reported in Supplementary Table S1.
Percent overall agreement with reference data was computed for the LULC change map as well as the 1997 and 2010 LULC maps. In addition, individual class accuracies were computed in terms of user and producer agreement for the permanent crops and evergreen forest LULC classes, given that each of these two classes each were allotted 50 or more randomly sampled locations in the stratified random sample. The results of mentioned overall and individual classes agreement statistics are shown in Figure 5. In addition, percent overall agreement of the LULC change map was also computed for more simplified classification schemes in which included mature forest (grouping evergreen and deciduous forest types) and agriculture (grouping permanent crops and shifting cultivation classes).
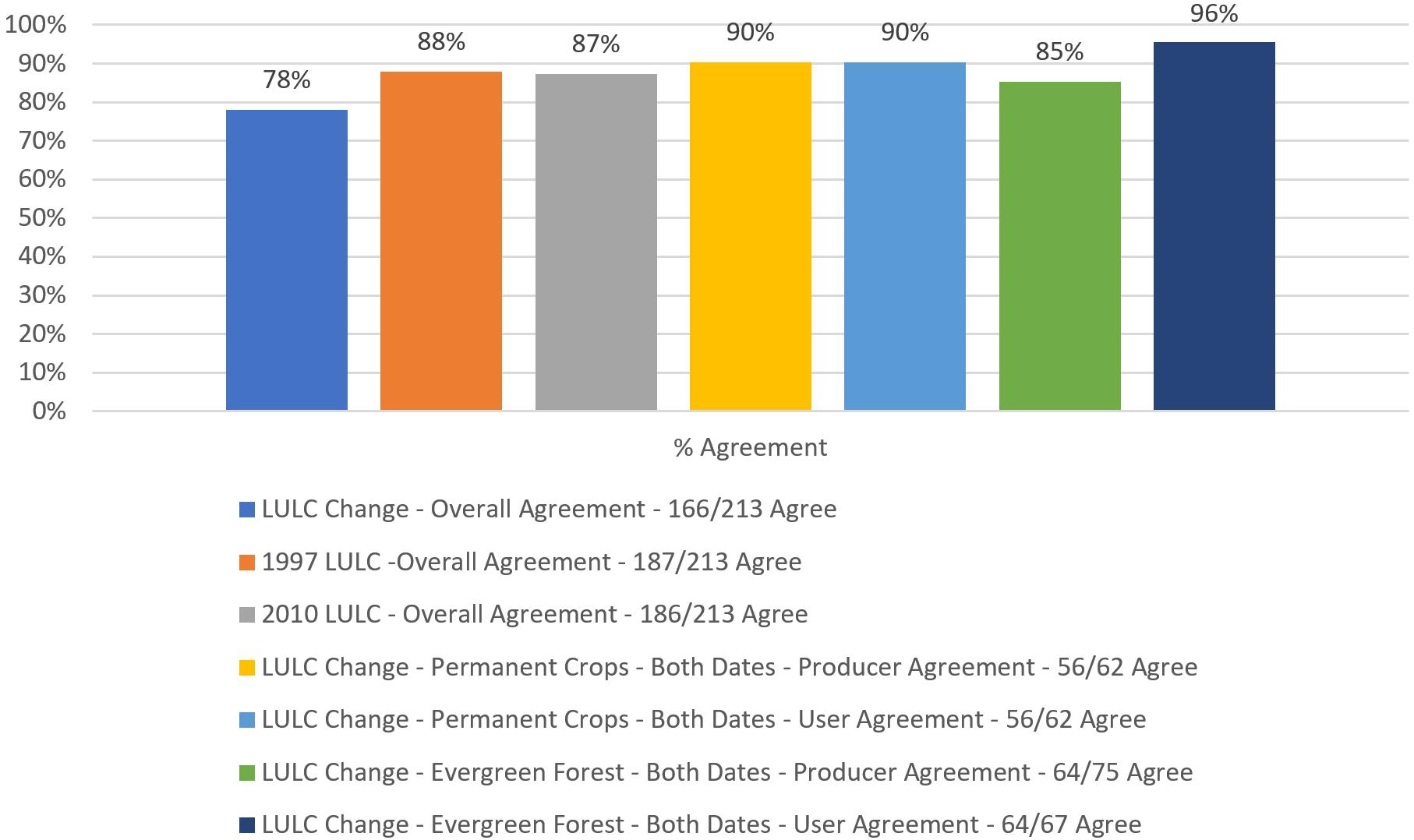
Figure 5. Results of LULC change map accuracy assessment, including percent overall agreement of LULC change map, 1997 LULC map, and 2010 LULC map was well as percent user and producer agreement for the no change permanent crops and evergreen forest LULC change classes.
The LULC class frequencies for the 1997 and 2010 maps were summarized in terms percent of total area for each mapped LULC class (Figure 6) with additional information provided in Supplementary Table S3. In addition, a Sankey diagram was computed to illustrate and further assess LULC change transitions between 1997 and 2010 (Figure 7). Summary areas for select LULC change classes were then merged into more general categories to further assess the main LULC changes across the study area (Figure 8 and Supplementary Table S4).
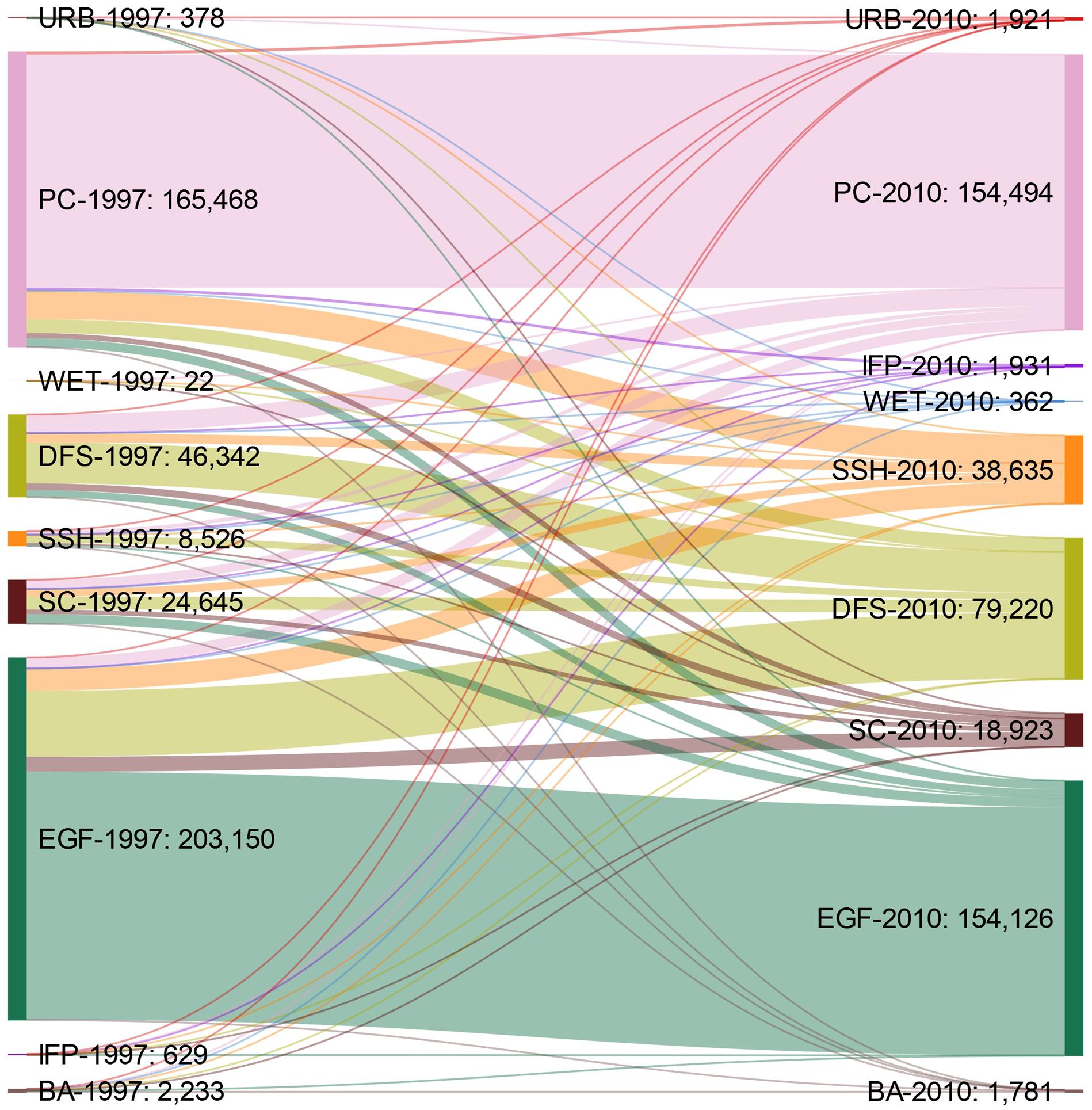
Figure 7. Sankey diagram showing LULC change transitions for 1997–2010. LULC class frequency is reported in terms of km2. LULC classes include: (1) BA (Barren); (2) URB (Urban/Developed); (3) PC (Permanent Crops); (4) SC (Shifting Cultivation); (5) SSH (Scrub/Shrub/Herbaceous); (6) DFS (Deciduous Forest/Scrub); (7) EGF (Evergreen Forest); (8) IFP (Industrial Forest Plantation); and (9) WET (Wetland Cover). For additional descriptions of these LULC classes, see Table 1.
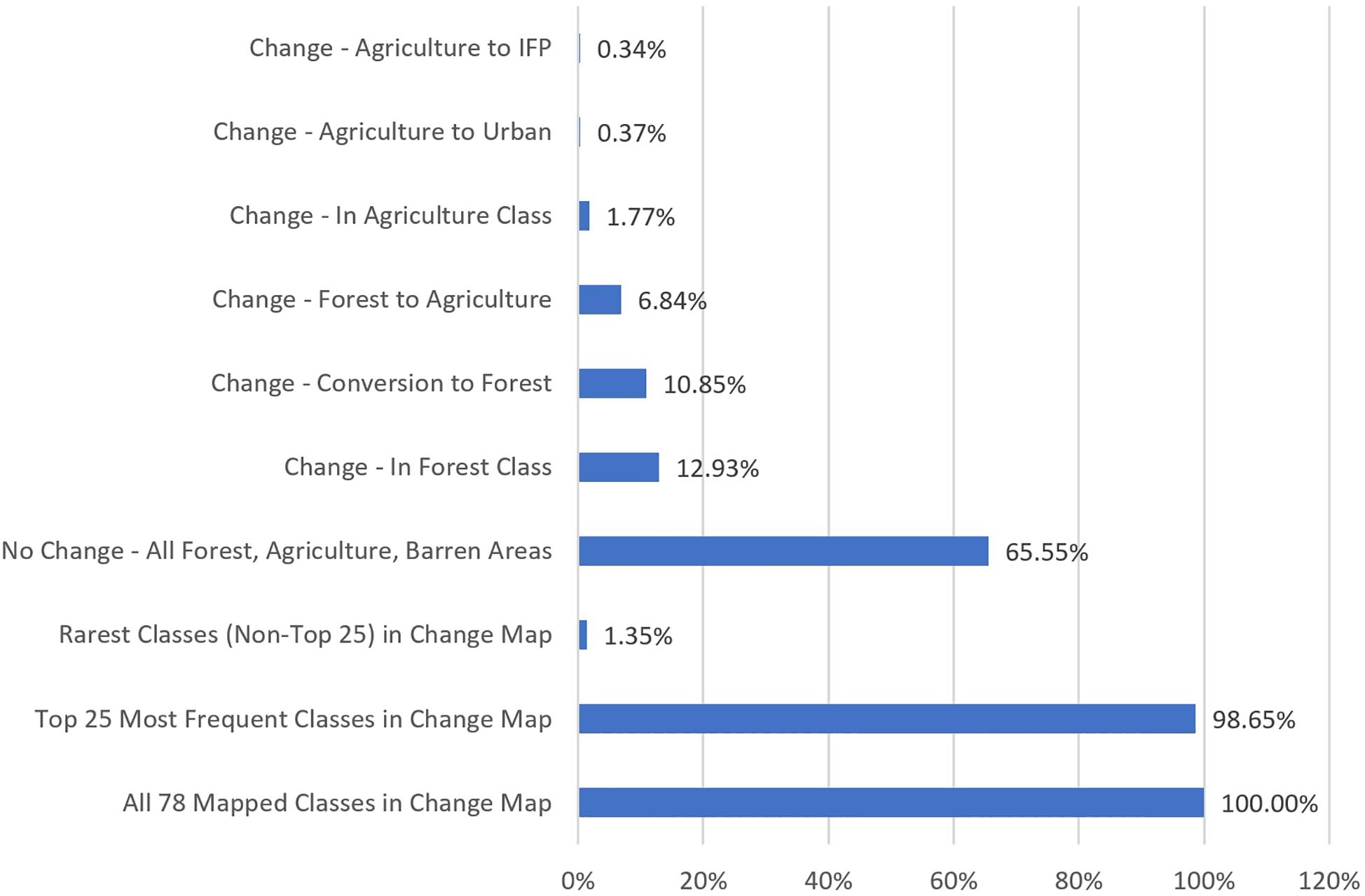
Figure 8. Percent frequency of grouped LULC change categories compared to total mapped area. For this figure: (1) forests pertain to semi-natural deciduous forests, evergreen broadleaved forests, and scrub/shrub/herbaceous areas; (2) agriculture includes permanent crops and shifting cultivation areas; (3) IFP = Industrial Forest Plantations. For additional information, see Supplementary Table S4.
Results and Discussion
LULC Change Map Validation
Compared to available reference data, the LULC change map dataset produced an estimated overall agreement of 78% with 6.7% margin of error at the 95% confidence interval, based on 166 sample locations in agreement out of a total of 213 sample locations (Figure 5). However, the overall agreement level for the LULC change map with reference data increases to ∼82% (174/213 sample locations agreed) if one groups the evergreen and deciduous forest into 1 class. The overall agreement further improved to ∼83% (177/213 total) by grouping the forest classes into a mature forest category and grouping the agricultural classes into a single agriculture class. The level of overall agreement for the LULC change map with reference data was influenced in part by the complexity of the map in terms of total mapped 78 change classes out of possible maximum of 81 (Supplementary Table S1).
The validation of the change map also enabled estimates of percent overall agreement for each input LULC map compared to reference data (Figure 5). Both the 1997 and 2010 LULC maps (each with 9 total classes) showed similar levels of overall agreement to available reference data. The 1997 map produced an 87.8% overall agreement and the 2010 map yielded an 87.3% overall agreement. The observed disagreement between the change map and reference data (i.e., classification errors) were caused by disagreement on either the 1997 and/or 2010 LULC map compared to reference data, including 6 sample locations with errors disagreement on both dates of LULC maps, 20 locations with apparent errors only on the 1997 LULC map, and 21 locations with apparent errors only on the 2010 LULC map. The observed overall agreement for the 1997 and 2010 LULC maps to reference data is regarded as good for general LULC classification schemes and single dates of LULC maps, given that the overall agreement for both dates equaled or exceeded 85% (Anderson et al., 1976). These accuracy assessment results for the individual dates of LULC maps are also comparable to what was produced in another multi-date LULC mapping and change detection study discussed by Ellis et al. (2011) and Spruce et al. (2014).
In addition, the two most frequent LULC change classes, no change classes for permanent crops and evergreen forest, both yielded user and producer class agreements that were high, ranging from 85 to 96% (Figure 5). The frequency of occurrence for other mapped LULC change classes were much lower (Table 2) and as a result the number of samples for other LULC change classes were much less than 50 sample locations per class (e.g., the third most frequent LULC change class was allotted 18 samples), which is too small to viably estimate individual class producer and user agreement statistics. Given available resources, the main goal of the LULC map validation was to estimate the overall accuracy of the LULC maps. More analysis is needed to further assess accuracy of the low frequency, regionally scarce to rare LULC change classes.
The LMB SWAT models use single dates of LULC map data as an input and not the LULC change map dataset. Although the LULC change map is not to be directly utilized by LMB SWAT models, it provides information that is complementary to the 1997 and 2010 LULC maps.
LMB LULC Change Trends
The LULC maps for 1997 and 2010 are shown in Figures 2, 3 and the LULC change map for 1997–2010 is given in Figure 4. These maps collectively show areas with both LULC stability and change with the main LULC changes occurring in LMB sub-basins that have more extensive forests and are less agriculturally dominated. For this region, some conversion of forest to agriculture (i.e., deforestation) was observed on the LULC change map. Increases in permanent crops were apparent in multiple SBs, including SBs 3, 5, and 6. Some of such new permanent agriculture areas for 2010 were mapped as forest in 1997. In addition, some of the new permanent crops areas in 2010 were mapped as shifting cultivation in 1997. Agriculture dominated SBs (SBs 7 and 8) on the 1997 LULC map showed less overall change than other SBs that were not agriculturally dominated as of 1997. The SBs that were mixed agriculture and forest in 1997 tended to have more frequent, visibly apparent forest to agriculture conversion by 2010. Some areas adjacent to contiguous forests in 1997 appeared to convert from evergreen (broadleaved) forest or shifting cultivation to deciduous forest/scrub. SBs with extensive forest in 1997 tended to retain extensive forests as of 2010, especially with respect to more remote, higher elevations. As of 1997, much of the lowland forests (e.g., in the Khorat Plateau) were already largely converted to agriculture, including areas along waterways that were presumably woody wetlands. An expansion of industrial forest plantations (IFPs) was observed primarily in SBs 4 and 6. Some expansion of urban/developed areas was also observed from 1997 to 2010, though some of the increase in urban areas were due to commission errors on the 1997 map (compared to Landsat false color imagery) in addition to urban expansion. In SB 6, some increases in evergreen forests were observed on the change map, along with increases in permanent and shifting cultivation that occurred mostly adjacent to extensive deciduous forests.
Of the 25 most frequent LULC change classes, only 5 were for “no change” categories where the LULC class stayed the same for both dates (Table 2). These 5 most frequent non-change classes represented 65.6% of the total mapped area. In addition, 3 of the top 5 most frequent LULC change classes were for non-change categories. The latter categories regarded evergreen forest, permanent crops and deciduous forest/scrub and collectively represented ∼64.7% of the total mapped area. The no change classes for evergreen forest and permanent crops covered 30.7 and 28.9% of the total area, respectively. Collectively these 2 classes occurred on ∼59.7% of the total mapped area. Even for the more stable LULC no change classes (e.g., evergreen forest and permanent crops) we also observed losses (to other LULC classes) and gains from other LULC class changes to a given LULC type. The more transitional LULC classes were as expected particularly dynamic, including the shifting cultivation, scrub/shrub/herbaceous, and deciduous forest/scrub classes that can be related to swidden cultivation practices. Of the 25 most frequent LULC change classes, 7 of these regarded permanent crops (including 1 no change class). In addition, there was a 5 total of LULC change classes each for shifting cultivation, deciduous forest/scrub, and evergreen forest LULC classes (including 1 no change class per mentioned LULC category). The scrub/shrub/herbaceous class mapped in 1997 was not one of the top 25 most frequent LULC change classes, which may be in part due to it being a highly transitional or forest successional LULC class as opposed to a more permanent (i.e., stable) LULC class. The 25 most frequent LULC change classes all together comprised 98.65% of the total mapped area. In contrast, the highly rare LULC change classes collectively represented 1.35% of the total area and consisted of 53 total classes with each class ranging from 0.00 to less than 0.24% of the total area. Supplementary Table S1 includes summary area estimates for all the LULC change classes, including these rarer classes not reported in Table 2.
Figure 6 reports percent of total area of individual LULC classes recorded on the 1997 and 2010 maps and it, along with Supplementary Table S3, were used to identify multiple trends: Barren areas were reduced in 2010 slightly from 0.49 to 0.40% of the total mapped area. Although infrequent in occurrence on both dates, urban areas increased by ∼5 times, which was due to part to increased urbanization and the apparent under classification of urban areas on the 1997 LULC map. The permanent agriculture class decreased by ∼−2.5% of the total mapped area, which could be due to multiple factors such as abandonment of permanent crops or the conversion of permanent crops to forests and other LULC classes. The shifting cultivation class decreased as well by ∼−1.3%, which was expected due to a reported decreased use of this agricultural practice in the region (FAO/IWGIA/AIPP, 2015). In contrast, the scrub/shrub/herbaceous category showed a ∼6.7% increase in total mapped area that could be due to abandoned agricultural areas reverting to forest. The latter class also commonly occurs amongst shifting cultivation and permanent crops areas. The deciduous forest class also appeared to increase by ∼7.3% with some of this occurring in areas that were mapped as evergreen broadleaved forest in 1997. This trend could be due in part to the methods and data used to classify forest deciduousness in 1997 compared to 2010. In addition, some agricultural areas in 1997 had changed to deciduous forest/scrub in 2010.
The evergreen broadleaved forests showed the largest change in area estimated of any LULC class with a 10.8% decrease in the total mapped area from 2010 versus 1997 (Figure 6). The decrease in evergreen forest is probably due to multiple factors. We think it is in part related to the remotely sensed data and mapping method used to derive the 1997 LULC map. The forest deciduousness levels for the 1997 LULC map did not include Landsat image data collected at monthly intervals across the calendar year, while the 2010 LULC map used the monthly MODIS NDVI data to help classify forest deciduousness according to vegetation greenness phenology (Spruce et al., 2018). Given that the 2010 map used higher temporal resolution MODIS data to classify forest deciduousness, we think that the 2010 LULC map probably shows forest deciduousness classes more realistically compared to the 1997 map dataset. The production of the 2010 LULC map leveraged the vegetation greenness phenology to classify forest types based on deciduousness and multiple agricultural types with comparatively unique phenology (Spruce et al., 2018). Other potential factors include conversion to agriculture or transition to deciduous forest as a result of timber harvesting according to Rundel (2009). In addition, the drought of 2010 (Zhang et al., 2013; Guo et al., 2017) may be a factor why there was less evergreen forest in 2010, given that drought can cause deciduousness in otherwise evergreen forest (Rundel, 1999).
In addition, the IFP was mapped in 2010 was ∼3 times more than in 1997 but still a very small percentage of the total mapped area (0.3%) (Figure 6). Although rare in occurrence (0.1% of total area), about 15 times more wetlands were mapped in 2010, perhaps due to: (1) certain permanent agricultural areas on wetland sites in 1997 had apparently been abandoned and reverted to wetland vegetation in 2010; and (2) occasional commission errors observed on the 2010 LULC map.
The Sankey diagram (Figure 7) shows LULC class change transitions occurring from 1997 to 2010. The transitions are colorized on this diagram so that one can trace back from 2010 to 1997 to assess how LULC changed. Most of the permanent crops and evergreen forest areas in 2010 were also the same class in 1997. Some of the permanent crops in 2010 was previously either a forest class or shifting cultivation in 1997. Also, certain areas mapped as agriculture in 1997 transitioned to the evergreen forest class by 2010. The deciduous forest class in 2010 was previously mapped as a mixture of forest and agricultural classes in 1997. Also, some areas with forest and agricultural classes in 1997 had transitioned to the transient scrub/shrub/herbaceous class in 2010. Only a few scrub/shrub/herbaceous areas in 1997 were retained as the same class in 2010, which is related to the transitional nature of this LULC class. Most of the shifting cultivation areas in 2010 were previously mapped as evergreen forest in 1997. Some deciduous forest and agricultural areas in 1997 also transitioned to shifting cultivation in 2010. The remaining 4 of 9 mapped LULC classes in 2010 were comparatively rare and tended to be mapped as a wide variety of LULC classes in 1997. Taken as a whole, the Sankey diagram indicates that extensive forest and agricultural areas of the LMB region had the same LULC class for both dates, though some of the forest changed from evergreen forest to some other forest class or agriculture. Also, conversely, certain agricultural areas had transitioned to various forest classes. The mapped area at large includes mixture of forest and agriculture areas for both dates with occasional area specific fluctuations between forest and agricultural types occurring between the two dates. Also, there appears to be a diminished frequency of shifting cultivation by 2010, which is possibly related to afforestation or else conversion to permanent agriculture. The shifting cultivation type is visibly dynamic for 1997 versus 2010 as well.
More generalized groupings of the 25 most frequent LULC change classes are summarized as a percent of total mapped in Figure 8 (see Supplementary Table S4 for additional information). The most frequent group was for no change LULC classes, representing 65.55% of the total mapped area. Changes in forest class was 2nd most frequent group estimated at 12.93% of the total mapped area. In this analysis, forest classes are regarded as being either evergreen forest, deciduous forest, or scrub/shrub/herbaceous (i.e., forest regeneration). The 3rd most frequent LULC change group was for non-forest (mostly agricultural classes) changing to some form of forest. The latter change group represented 10.85% of the total area mapped. Forest to agriculture conversion occurred on ∼6.84% of the total area. The remaining 3 LULC class groups shown in Figure 8 were much less frequent in occurrence, including 0.34% (of total area) for changes from agriculture to IFP, 0.37% for change from agriculture to urban, and 1.77% for changes in agricultural class (e.g., from shifting cultivation to permanent crops).
Some of the observed 1997–2010 LULC change areas (Figure 4) were for locally common yet regionally scarce to rare classes, including LULC classes of concern such as those related to the expansion of IFPs and urban areas (Supplementary Table S1). Such infrequent yet important LULC change classes were not sampled in the accuracy assessment, due in part to the comparatively low class frequency, the adopted stratified random sampling approach, plus the time and resources available to do the accuracy assessment. In addition, other scarce classes (that were more frequent than rare but less frequent than common classes) were only minimally sampled in the accuracy assessment. The utilized stratified random sampling approach did provide a means to assess the overall agreement between the LULC change map and reference data. It also yielded some insight into the ability of the two most predominant LULC change classes (evergreen forest and permanent crops no change categories) to be mapped. However, more work is needed to further assess map accuracy of other individual LULC change classes, such as the comparatively scarce to rare LULC change classes.
More effort is also be needed to map and assess LULC change according to a more specific LULC scheme. To construct the change map, the adopted LULC classification scheme for agriculture was more general than desired, given that the 1997 LULC only included one general permanent crops class and that it did not distinguish the number of crops per year and between row crops and rice paddies (Spruce et al., 2018). As a result, the LULC scheme used for both 1997 and 2010 in this study included only 1 all-encompassing permanent crops class, due to the 1997 LULC map only containing 1 permanent crops class. It would be useful from a SWAT modeling perspective to know more about the changes in irrigated rice cultivation from 1997 to 2010. Although 1 permanent crops class in the overall LULC scheme is sub-optimal desired for MRC SWAT modeling applications, this general category was still useful in our study for assessing the change status of permanent cropped areas within the study area and observation period.
The assessment of the LULC change map also revealed other certain limitations. For example, the 1997 LULC map did not have complete coverage for all 8 SBs that were used in the SWAT modeling framework, perhaps due to clouds on the utilized Landsat data and much less area mapped for SB 1. In contrast, the 2010 LULC map included full coverage for SB 1 and did not have no-data areas due to clouds.
Implications of Results
The 1997–2010 LULC change map indicates a mix of forest and agricultural LULC change classes occurred, including about 2/3 of the total area staying the same LULC class for both dates. Conversely, about 1/3 of the mapped area underwent some form of LULC change, according to the LULC change map. Although some of the study area has undergone noteworthy LULC change over the 13-year interval from 1997 to 2010, extensive areas of evergreen forest and permanent crops were not converted to some other land use. Some of the apparent LULC change during the observation period appeared to be of an ephemeral or transitional nature (e.g., change related to agricultural and forestry practices). Other observed LULC change was apparently more permanent in nature (e.g., forest cover to permanent crops). In addition, the LULC change map included areas with deforestation and afforestation, which help to illustrate the dynamic nature of LULC in the region during the observed 13-year time frame.
Some new IFP areas were observed with ∼3 times more IFP mapped on the 2010 vs. 1997 LULC map (Figure 6). The amount of mapped IFP in 2010 is a very low percentage of the total mapped area. However, the amount of IFP mapped on at least the 2010 date is somewhat conservatively estimated, given IFP mapping for 2010 was based on 1 date of dry season Landsat data in which some of the IFPs were highly green and other IFP patches were senesced. Improved classifications of IFP may be produced using multiple dates of Landsat data from different times of year when such is available in cloud-free form (Fan et al., 2015; Hurni et al., 2017). Based on spot checks on more recent high-resolution aerial and satellite data on Google Earth Pro and recent publications (Fox and Castella, 2013; Fox et al., 2014), it seems likely that there has been some expansion of IFP areas since 2010 in the SBs 1–8 of the LMB. More work is needed to produce a more up to date LULC map for assessing expansion of IFP areas as well as other LULC changes in the LMB since 2010. When doing so, it might be possible to improve maps of IFP areas using a combination of harmonized Landsat and Sentinel-2 data so that classification of phenology of rubber trees can be better leveraged in deriving more effective IFP maps. Such a strategy could increase the availably of cloud free data in the cloud prone tropical portion of the LMB for both the leaf-on and leaf-off states of IFP, though additional data processing may be needed to effectively combine use of the Landsat and Sentinel-2 data (Flood, 2017; Claverie et al., 2018). Use of time series data processing methods (Robinson et al., 2017; Jönsson et al., 2018) could further improve the Landsat/Sentinel-2 reflectance data products needed to map IFP areas across a broad region like the LMB.
We think there are multiple drivers affecting the patterns evident on the LULC change map with the importance of specific drivers somewhat dependent on the SB. LULC change related to permanent agricultural expansion and intensification, reduced shifting cultivation patch sizes, and forest practices could be related to economic and policy drivers. Part of the observed agricultural intensification may be related to the increased cultivation of cash crops. Also, the observed increase in industrial forest plantations mostly pertains to a woody cash crop (i.e., rubber plantations). We observed increased urbanization which in part could be due to demographic drivers (i.e., human population growth). Based on reviews of 1997 and 2010 Landsat imagery, we could see some forest change (e.g., deforestation and conversion to permanent crops) in areas where roads had expanded into forested areas. Road access was also identified as a LULC change driver by Rowcroft (2008) and Xing (2013). Based on summary areas per class for the 1997 and 2010 LULC maps (Figure 6 and Supplementary Table S3), mature broadleaved forests (deciduous and evergreen forest combined) increased by ∼4% of the entire mapped area, while all agriculture (permanent and shifting cultivation) decreased by ∼4% of the total mapped area. The decreased extent of agricultural areas could possibly be explained by a combination of agricultural intensification, technical advances in agriculture that required less but more intensively cropped land, and agricultural conversion to other LULC types, such as semi-natural forest, industrial forest, and urbanized areas. We further believe that the water and land use planning and policy making in the region may have influenced forest and agricultural change occurring during the observed time frame. Although afforestation appears to have occurred in the LMB at large from 1997 to 2010, the change map also showed a decrease in evergreen broadleaved forest that is in part related to recovery from forest disturbances. Also, on the LULC change map, there are hot spot areas in certain SBs where deforestation is quite prevalent. Afforestation at the country scale was also recently noted in a 1990 to 2015 forest change map produced for the FAO 2015 Global Forest Resources Assessment for Laos, Thailand, and Vietnam (FAO, 2015). The same study noted that forest loss occurred in Myanmar and Cambodia, though very little area in Myanmar is mapped in LMB area covered by our maps. Also, our study area only partially covers Cambodia. Netzer et al. (2019) noted that considerable deforestation occurs is in the Tonle Sap sub-basin of Cambodia, which is outside the study area for our LULC change map.
The LULC change map showed a moderate level of overall agreement (∼78%) with reference data, which improved to ∼83% by reducing the total number of LULC classes per date from 9 to 5 as follows: (1) merging the deciduous and evergreen broadleaved forest classes into 1 category; and (2) merging the permanent crops and shifting cultivation types into 1 class. At 9 classes per date, the 1997 and 2010 LULC maps used to make the change map each showed ∼87% level of overall agreement. Although imperfect, the LULC mapping products provided previously unavailable information on LULC change from 1997 to 2010. We acknowledge that the sample intensity used in the accuracy assessment of LULC mapping products in this study is lower than some studies, such as those by McCombs et al. (2016) and Yang et al. (2018). However, our study, as is, enabled quantification of the percent overall accuracy of the LULC change map and the individual LULC maps used to compute the change map. Such information, along with corresponding LULC maps is now available to aid SWAT modeling efforts in the non-coastal LMB, which helps given the lack of comparable LULC mapping studies in the region for the same time frame and purpose. The total sampling size used in the LULC change map accuracy assessment was constrained by available resources and time, though was greater than or comparable to the sample intensities used in LULC mapping studies by Ellis et al. (2011) and Spruce et al. (2014). However, the margin of error for our LMB LULC change map accuracy estimate could be reduced by increasing the sampling size.
We think it is also possible to improve LULC change map accuracy for the region and time period, though doing so would likely require different methods and data than was used in this project. The 1997 LULC map dataset that we used was based on Landsat data hard copies that were photo interpreted, delineated, labeled, and digitized. There also isn’t any MODIS data available for 1997, given that the first MODIS sensor wasn’t deployed until 2000. In contrast, the 2010 LULC map was based on both MODIS and Landsat data in conjunction with image classification and GIS techniques (Spruce et al., 2018). While it is unclear if the 2010 versus 1997 LULC change map data from our project can be improved with additional processing, a more viable 2010 versus 2003 LULC change map dataset could be derived using MODIS Terra/Aqua and Landsat data for both dates of LULC. In doing so, the begin date could be processed at a higher classification scheme specificity than what was feasible with the 1997 LULC map data set. In particular, the 1997 LULC map data only included one type of permanent crops, which is less desirable for SWAT modeling. The LULC change map for this project was generated to aid interpretation of SWAT modeling output using either the 2010 or 1997 LULC map data as inputs. However, the SWAT model requires a single date of LULC data as opposed to a LULC change map. Given this fact, the overall accuracy of each date of LULC map used in LMB SWAT models is probably a more important consideration to SWAT modelers than the overall accuracy of the LULC change map for application at hand (e.g., SWAT hydrologic modeling).
LULC map data is an important input to the SWAT hydrologic model in addition to data on soils, terrain, and precipitation (Gassman et al., 2007; Rossi et al., 2009). Based on the soils data alone (e.g., on hydrologic soil group), most of the LMB is regarded as having either moderately high or high runoff potential (Ross et al., 2018). The runoff risk is further acerbated at least seasonally by the high amounts of rainfall that can occur in this tropical region during the rainy season (MRC, 2010). LULC classes can have unique runoff potential which are described in part via Soil Conservation Service (SCS) curve numbers (USDA, 1986; Hong and Adler, 2008). For example, evergreen broadleaved forests have a lower runoff potential (i.e., SCS curve number) than agricultural cropland for given hydrologic soil group and hydrologic condition class (Hong and Adler, 2008). Extensive conversion of forests to permanent crops or other non-forests can change the runoff characteristics within a sub-basin (Netzer et al., 2019). Consequently, forests are a preferred LULC type by the MRC for catchments being used for water supplies (MRC, 2010).
The LULC change map data product from our study enabled more insight into the location, extent and kind of LMB LULC change in the region and within SBs (#1–8) used in SWAT hydrologic modeling applications, such as SWAT models of runoff for watersheds within a given SB. The project results increased understanding of LULC change in the region during the observed time frame, which could help those using and further developing LMB SWAT modeling products. For example, we think the change map from this project can be employed to aid use and development of LMB SWAT modeling products to support NASA/USAID SERVIR water and disaster management applications in the LMB (Mohammed et al., 2018a,b,c; McDonald et al., 2019). The LULC change map produced through this project (Supplementary Data File 1) can be used to view and assess potential 1997–2010 LULC change impacts for watersheds within LMB SBs 1–8. The data products from this project provide a baseline on LULC change for 1997–2010 that could be used to aid management of water, agriculture, and natural disasters in the region, as well as more in-depth follow-on studies on the LULC change occurring in the LMB since 2010. Such LULC change data sets could also possibly benefit the SERVIR Mekong Hub’s Regional Land Cover Monitoring System (Saah et al., 2019b), which was recently applied by Ingalls et al. (2018) in LULC change assessment and land resource planning.
Conclusion
A LULC change mapping study for the LMB was conducted using GIS techniques along with 1997 and 2010 LULC map data sets to produce a LULC change map. Both dates of LULC maps have been used as inputs to the MRC SWAT hydrologic model framework for the SBs 1–8 of the LMB. The change map was derived to: (1) map, quantify, and assess 1997–2010 LULC change to aid assessment of the LMB SWAT modeling results; and (2) to provide previously unavailable information on LMB LULC change for 1997–2010 that could be used for supporting water and land resource management and planning. While much (almost 2/3rds) of the LULC change map showed no change, there were other observed areas with apparent LULC change. This was as expected given that the area includes a dynamic mix of semi-natural and human-dominated LULC. In addition, not all of the observed change appeared to be of a permanent nature, but instead some form of ephemeral or transitional change associated with agricultural and/or forestry practices.
The study provided needed supplemental geospatial information on 1997–2010 LULC change to modelers for assessing LMB SWAT modeling results that are based on either the currently used 2010 or the previously used 1997 LULC map. The study also helped to identify LULC change hotspot locations within specific SBs where LULC change may be affecting hydrologic parameters of sub-watersheds that are modeled with the SWAT modeling framework. The project’s LULC change map dataset (Supplementary Data File 1) and corresponding analysis discussed in this paper enabled a baseline record of the LULC change for 1997–2010 that is available for aiding follow-on studies in support of water, disaster, forest, and agricultural management efforts in the LMB. The 1997–2010 LULC change map from the project could possibly be refined with additional data processing techniques. More work is also needed to further update the 2010 LULC map now used in LMB SWAT modeling for the current time frame and to map LMB LULC change since 2010.
Data Availability Statement
All datasets generated for this study are included in the article/Supplementary Material.
Author Contributions
The project design was developed by JS and JB. The project was implemented by JS with input from JB. The article was drafted by JS with input from JB. The article was reviewed by JB, IM, RS, and VL, revised and submitted by JS.
Funding
This work was funded from a 2011 NASA ROSES A.33 Earth Science Applications: Disasters grant (NNH11ZDA001N-DISASTER) in conjunction with a NASA Goddard Space Flight Center (GSFC) Applied Sciences grant (NNG15HQ01C) to Science Systems and Applications, Inc. (SSAI) for supporting work by the Hydrospheric and Biospheric Sciences (HBS) Laboratory at NASA GSFC.
Conflict of Interest
The authors declare that the research was conducted in the absence of any commercial or financial relationships that could be construed as a potential conflict of interest.
Acknowledgments
The project was benefitted by collaboration, documentation, and data from the Mekong River Commission provided by Dr. Sopheap Lim (who works with LMB SWAT models). The 2010 LULC map from the project employed MODIS monthly NDVI data products that were originally compiled for a project conducted at NASA Stennis Space Center to aid the USGS Forecast Mekong project. This study also aided by cooperation with the SERVIR Mekong Hub and the Asian Disaster Preparedness Center. Opinions, findings, and conclusions or recommendations found in this work are those of the author(s) and do not necessarily represent the views of NASA, SSAI, and or other participating organizations.
Supplementary Material
The Supplementary Material for this article can be found online at: https://www.frontiersin.org/articles/10.3389/fenvs.2020.00021/full#supplementary-material
Footnotes
References
Anderson, J. R., Hardy, E. E., Roach, J. T., and Witmer, R. E. (1976). A Land Use and Land Cover Classification System for Use with Remote Sensor Data. Geological Survey Professional Paper 964. Reston, VA: U.S. Geological Survey.
Bey, A., Sanchez-Paus Diaz, A., Maniatis, D., Marchi, G., Mollicone, D., Ricci, S., et al. (2016). Collect earth: land use and land cover assessment through augmented visual interpretation. Rem. Sens. 8:807. doi: 10.3390/rs8100807
Claverie, M., Ju, J., Masek, J. G., Dungan, J. L., Vermote, E. F., Roger, J. C., et al. (2018). The Harmonized Landsat and Sentinel-2 surface reflectance data set. Remote Sens. Environ. 219, 145–161. doi: 10.1016/j.rse.2018.09.002
Congalton, R., and Green, K. (2009). Assessing the Accuracy of Remotely Sensed Data: Principles and Practices, 2nd Edn. Boca Raton, FL: CRC/Taylor & Francis, 183.
Congalton, R. G. (1991). A review of assessing the accuracy of classifications of remotely sensed data. Remote Sens. Environ. 37, 35–46. doi: 10.1016/0034-4257(91)90048-b
Congalton, R. G. (2015). “Assessing positional and thematic accuracies of maps generated from remotely sensed data,” in Remotely Sensed Data Characterization, Classification, and Accuracies, ed. P. Thenkabail, (Boca Raton, FL: CRC Press), 583–601.
Coppin, P., Jonckheere, I., Nackaerts, K., Muys, B., and Lambin, E. (2004). Review article digital change detection methods in ecosystem monitoring: a review. Int. J. Remote Sens. 25, 1565–1596. doi: 10.1080/0143116031000101675
Costenbader, J., Broadhead, J. S., Yasmi, Y., and Durst, P. B. (2015a). Drivers Affecting Forest Change in the GMS: an Overview. Rome: FAO/USAID LEAF. doi: 10.1080/0143116031000101675
Costenbader, J., Varns, T., Vidal, A., Stanley, L., and Broadhead, J. S. (2015b). Drivers of Forest Change in the Greater Mekong Subregion. Regional Report. Rome: FAO/USAID LEAF.
Dat, N. D. (2013). “Model setup in Mekong Basin,” in Presentation given by MRC Modeling Team From the Information and Knowledge Management Programme, (Washington, DC: NASA Headquarters).
Ellis, J. T., Spruce, J. P., Swann, R. A., Smoot, J. C., and Hilbert, K. W. (2011). An assessment of coastal land-use and land-cover change from 1974–2008 in the vicinity of Mobile Bay. Alabama. J. of Coastal Conservation 15, 139–149. doi: 10.1007/s11852-010-0127-y
Estoque, R. C., Ooba, M., Avitabile, V., Hijioka, Y., DasGupta, R., Togawa, T., et al. (2019). The future of Southeast Asia’s forests. Nat. Commun. 10:1829. doi: 10.1038/s41467-019-09646-4
Evers, J., and Pathirana, P. (2018). Adaptation to climate change in the Mekong River Basin: introduction to the special issue. Climate Change 149, 1–11. doi: 10.1007/s10584-018-2242-y
Fan, H., Fu, X., Zhang, Z., and Wu, Q. (2015). Phenology-Based Vegetation Index Differencing for Mapping of Rubber Plantations Using Landsat OLI Data. Remote Sens. 7, 6041–6058. doi: 10.3390/rs70506041
FAO (2015). Global Forest Resources Assessment 2015 - Desk Reference, Report, Food and Agriculture Organization of the United Nation. Rome: FAO, 245.
FAO/IWGIA/AIPP (2015). “Shifting cultivation, livelihood and food security - new and old challenges for indigenous peoples in Asia,” in Report Published Jointly by the Food and Agriculture Organization of the United Nations, International Work Group for Indigenous Affairs (IWGIA), and Asia Indigenous Peoples Pact (AIPP), Christian Erni Editor, (Rome: FAO/USAID LEAF), 434.
Flood, N. (2017). Comparing Sentinel-2A and Landsat 7 and 8 using surface reflectance over Australia. Remote Sens 9, 659. doi: 10.3390/rs9070659
Fox, J., and Castella, J. C. (2013). Expansion of rubber (Hevea brasiliensis) in mainland Southeast Asia: what are the prospects for smallholders? J. Peasant Stud. 40, 155–170. doi: 10.1080/03066150.2012.750605
Fox, J., Castella, J. C., Ziegler, A., and Westley, S. (2014). Rubber plantations expand in mountainous Southeast Asia: What are the consequences for the environment? Asia Pacific Issues 114, 1–8.
Gassman, P. W., Reyes, M. R., Green, C. H., and Arnold, J. G. (2007). The soil and water assessment tool: Historical development, applications, and future research directions. Trans. of the ASABE 50, 1211–1250. doi: 10.13031/2013.23637
Giri, C. P., Defourny, P., and Shrestha, S. (2003). Land cover characterization and mapping of continental Southeast Asia using multi-resolution satellite sensor data. Int. J. Remote Sens. 24, 4181–4196. doi: 10.1080/0143116031000139827
GMS-EOC (2019). Climate Change in Lower Mekong Basin, Web Site for GIS Data Layers Used in USAID Mekong Adaptation and Resilience to Climate Change (ARCC) Project Conducted by ICEM. Avaliable online at: http://portal.gms-eoc. org/maps?cmbIndicatorMapType=data&cmbIndicatorTheme=9&cmb IndicatorMap=30 (last accessed 5/10/19).
Guo, H., Bao, A., Liu, T., Ndayisaba, F., He, D., Kurban, A., et al. (2017). Meteorological drought analysis in the Lower Mekong Basin using satellite-based long-term CHIRPS product. Sustainability 9:901. doi: 10.3390/su9060901
Halcrow Group Limited (2004). “Tables of crop calendars, crop factors, and irrigation efficiency and return factors,” in Annex B.2 to: Decision Support Framework. Water Utilization Project Component A: Final Report, (Phnom Penh: MRC Technical Reference Report, DSF 620 SWAT and IQQM Models), 11.
Heinimann, A., Messerli, P., Schmidt-Vogt, D., and Wiesmann, U. (2007). The dynamics of secondary forest landscapes in the Lower Mekong Basin. Mt. Res. Dev. 27, 232–241. doi: 10.1659/mrd.0875
Hong, Y., and Adler, R. F. (2008). Estimation of global SCS curve numbers using satellite remote sensing and geospatial data. Int. J. Remote Sens. 29, 471–477. doi: 10.1080/01431160701264292
Hurni, K., Schneider, A., Heinimann, A., Nong, D. H., and Fox, J. (2017). Mapping the expansion of boom crops in mainland Southeast Asia using dense time stacks of Landsat data. Remote Sens. 9:320. doi: 10.3390/rs9040320
Hussain, M., Chen, D., Cheng, A., Wei, H., and Stanley, D. (2013). Change detection from remotely sensed images: from pixel-based to object-based approaches. ISPRS J. Photogramm. Remote Sens. 80, 91–106. doi: 10.1016/j.isprsjprs.2013.03.006
ICEM (2013). USAID Mekong ARCC Climate Change Impact and Adaptation Study for the Lower Mekong Basin: Main Report. Bangkok: USAID Mekong ARCC Project.
Imai, N., Furukawa, T., Tsujino, R., Kitamura, S., and Yumoto, T. (2018). Factors affecting forest area change in Southeast Asia during 1980-2010. PLoS One 13:e0197391.
Ingalls, M. L., Diepart, J. C., Truong, N., Hayward, D., Neil, T., Phomphakdy, C., et al. (2018). “State of Land in the Mekong Region,” in Centre for Development and Environment, University of Bern and Mekong Region Land Governance, (Bern: With Bern Open Publishing).
Jensen, J., Guptil, S., and Cowen, D. (2012). “Change Detection Technology Evaluation”: U.S. Census Bureau Geography Division, Deliverable #9 from Syneren Technologies Contract Task T007, Report No. 240. Suitland, MA: Census Bureau Geography Division.
Jönsson, P., Cai, Z., Melaas, E., Friedl, M. A., and Eklundh, L. (2018). A method for robust estimation of vegetation seasonality from Landsat and Sentinel-2 time series data. Remote Sens 10:635. doi: 10.3390/rs10040635
Keskinen, M., Chinvanno, S., Kummu, M., Nuorteva, P., Snidvongs, A., Varies, O., et al. (2010). Climate change and water resources in the Lower Mekong River Basin: putting adaptation into the context. J. Water Clim. Chang. 1, 103–117. doi: 10.2166/wcc.2010.009
Kityuttachai, K., Heng, S., and Sou, V. (2016). “Land cover map of the Lower Mekong Basin,” in MRC, Technical Paper No. 59, Information and Knowledge Management Programme, Mekong River Commission, Vol. 82, (Phnom Penh), 1683–1489.
Leinenkugel, P., Wolters, M. L., Oppelt, N., and Kuenzer, C. (2015). Tree cover and forest cover dynamics in the Mekong Basin from 2001 to 2011. Remote Sens. Environ 158, 376–392. doi: 10.1016/j.rse.2014.10.021
Lyon, S. W., King, K., and Polpanich, O. U. (2017). Lacombe G. assessing hydrologic changes across the Lower Mekong Basin. J. Hydrol. Reg. Stud. 12, 303–314. doi: 10.1016/j.ejrh.2017.06.007
Malyvanh, M., and Feldkotter, C. (1999). “Application of remote sensing and GIS for forest cover monitoring in LAO P.D.R,” in Conference Paper, Proceedings of Application of Resource Information Technologies (GIS/GPS/RS) in Forest Land & Resources Management, October 18 - 20, 1999, Hanoi, 48–61.
Mas, J. F. (1999). Monitoring land-cover changes: a comparison of change detection techniques. Int. J. Remote Sens. 20, 139–152. doi: 10.1080/014311699213659
McCombs, J. W., Herold, N. D., Burkhalter, S. G., Christopher, J., and Robinson, C. J. (2016). Accuracy assessment of NOAA coastal change analysis program 2006-2010 land cover and land cover change data. Photogramm. Eng. Remote Sens. 82, 711–718. doi: 10.14358/pers.82.9.711
McDonald, S., Mohammed, I. N., Bolten, J. D., Pulla, S., Meechaiya, C., Markert, A., et al. (2019). Web-based decision support system tools: the soil and water assessment tool online visualization and analyses (SWATOnline) and NASA earth observation data downloading and reformatting tool (NASAaccess). Environ. Modell. Software 120, doi: 10.1016/j.envsoft.2019.104499
Minderhoud, P., Coumou, L., Erban, L., Middelkoop, H., Stouthamer, E., and Addink, E. (2018). The relation between land use and subsidence in the Vietnamese Mekong Delta. Sci. Total Environ. 634, 715–726. doi: 10.1016/j.scitotenv.2018.03.372
Mohammed, I. N., Bolten, J. D., Srinivasan, R., and Lakshmi, V. (2018a). Improved hydrological decision support system for the Lower Mekong River Basin using satellite-based earth observations. Remote Sens. 10:885. doi: 10.3390/rs10060885
Mohammed, I. N., Bolten, J. D., Srinivasan, R., and Lakshmi, V. (2018b). Satellite observations and modeling to understand the Lower Mekong River Basin streamflow variability. J. Hydrol. 564, 559–573. doi: 10.1016/j.jhydrol.2018.07.030
Mohammed, I. N., Bolten, J. D., Srinivasan, R., Meechaiya, C., Spruce, J. P., and Lakshmi, V. (2018c). Ground and satellite based observation datasets for the Lower Mekong River Basin. Data in Brief 21, 2020–2027. doi: 10.1016/j.dib.2018.11.038
MRC (2014). Crop Production for Food Security and Rural Poverty - Baseline and Pilot Modeling. Vientiane: Mekong River Commission.
Nam, V. T. B., Haase, M., Kityuttachai, K., and Virak, S. (2015). “Land cover information catalogue of the Lower Mekong Basin,” in MRC Technical Paper, Information and Knowledge Management Programme, (Phnom Penh: Mekong River Commission), 18.
NASA and JPL (2013). NASA Shuttle Radar Topography Mission Global 1 arc second, distributed by NASA EOSDIS Land Processes DAAC. Avaliable online at: doi: 10.5067/MEaSUREs/SRTM/SRTMGL1.003 (last accessed 5/11/19).
Netzer, M. S., Sidman, G., Pearson, T. R. H., Walker, S. M. W., and Srinivasan, R. (2019). Combining global remote sensing products with hydrological modeling to measure the impact of tropical forest loss on water-based ecosystem services. Forests 10, 413. doi: 10.3390/f10050413
Olofsson, P., Foody, G. M., Herold, M., Stehman, S. V., Woodcock, C. E., and Wulder, M. A. (2014). Good practices for estimating area and assessing accuracy of land change. Remote Sens. Environ. 148, 42–57. doi: 10.1016/j.rse.2014.02.015
Olofsson, P., Foody, G. M., Stehman, S. V., and Woodcock, C. E. (2013). Making better use of accuracy data in land change studies: estimating accuracy and area and quantifying uncertainty using stratified estimation. Remote Sens. Environ. 129, 122–131. doi: 10.1016/j.rse.2012.10.031
Patil, P., and Gumma, M. K. (2018). A review of the available land cover and cropland maps for South Asia. Agriculture 8, 111. doi: 10.3390/agriculture8070111
Pokhrel, Y., Burbano, M., Roush, J., Kang, H., Sridhar, V., and Hyndman, D. W. (2018a). A review of the integrated effects of changing climate, land use, and dams on Mekong River. Hydrology 10:266. doi: 10.3390/w10030266
Pokhrel, Y., Shin, S., Lin, Z., Yamazaki, D., and Qi, J. (2018b). Potential disruption of flood dynamics in the Lower Mekong River Basin due to upstream flow regulation. Sci. Rep. 8:17767. doi: 10.1038/s41598-018-35823-4
QGIS (2017). QGIS Training Manual, Release 2.14, 418. Available online at: https://docs.qgis.org/2.14/pdf/en/QGIS-2.14-UserGuide-en.pdf (accessed September 03, 2020).
Ribbe, L., and Nauditt, A., Meinardi, D., Morbach, M., Becker, R., and Deutsche Gesellschaft f r Internationale Zusammenarbeit (GIZ) (2013). Comparison of Key Drivers Regarding their Significance for Hydro-meteorological Extremes and their Impacts on Selected Hotspots Within the Mekong River Basin. GIZ Report, No. 128. Cologne: University of Applied Sciences.
Robinson, N. P., Allred, B. W., Jones, M. O., Moreno, A., Kimball, J. S., Naugle, D. E., et al. (2017). A dynamic Landsat derived Normalized Difference Vegetation Index (NDVI) product for the conterminous United States. Remote Sens. 9, 863. doi: 10.3390/rs9080863
Ross, C. W., Prihodko, L., Anchang, J., Kumar, S., Ji, W., and Hanan, N. P. (2018). HYSOGs250m, global gridded hydrologic soil groups for curve-number-based runoff modeling. Sci. Data 5:180091. doi: 10.1038/sdata.2018.91
Rossi, C. G., Srinivasan, R., Jirayoot, K., Le Duc, T., Souvannabouth, P., Binh, N., et al. (2009). Hydrologic evaluation of the Lower Mekong River Basin with the soil and water assessment tool model. Int. Agric. Eng. J. 18, 1–13.
Rowcroft, P. (2008). Frontiers of change: the reasons behind land-use change in the Mekong Basin. Ambio 37, 213–218. doi: 10.1579/0044-7447(2008)37[213:foctrb]2.0.co;2
Rundel, P. W. (1999). “Forest habitats and flora in Lao PDR,” in Cambodia, and Vietnam. World Wildlife Fund Indochina Programme, Hanoi, 171.
Rundel, P. W. (2009). “Vegetation of the Mekong Basin,” in The Mekong: Biophysical Environment of an International River Basin, ed. I. C. Campbell, (Amsterdam: Academic Press), 143–160. doi: 10.1016/b978-0-12-374026-7.00007-3
Saah, D., Johnson, G., Ashmall, B., Tondapu, G., Tenneson, K., Patterson, M., et al. (2019a). Collect earth: an online tool for systematic reference data collection in land cover and use applications. Environ. Modell. Software 118, 166–171. doi: 10.1016/j.envsoft.2019.05.004
Saah, D., Tenneson, K., Matin, M., Uddin, K., Cutter, P., Poortinga, A., et al. (2019b). Land cover mapping in data scarce environments: challenges and opportunities. Front. Environ. Sci. 7:150. doi: 10.3389/fenvs.2019.00150
Spruce, J., Bolten, J., Srinivasan, R., and Lakshmi, V. (2018). Developing land use land cover maps for the Lower Mekong Basin to aid hydrologic modeling and basin planning. Remote Sens. 10, 1910. doi: 10.3390/rs10121910
Spruce, J. P., Smoot, J. C., Ellis, J. T., Hilbert, H., and Swann, R. (2014). Geospatial method for computing supplemental multi-decadal US coastal land use and land cover classification products, using Landsat data and C-CAP products. Geocarto Int. 29, 470–485. doi: 10.1080/10106049.2013.798357
Stibig, H. J., Achard, F., and Fritz, S. (2004). A new forest cover map of continental Southeast Asia derived from satellite imagery of coarse spatial resolution. Appl. Vegetation Sci. 7, 153–162. doi: 10.1111/j.1654-109x.2004.tb00606.x
Tran, H., Tran, T., and Kervyn, M. (2015). Dynamics of Land Cover/Land Use Changes in the Mekong Delta, 1973–2011: A Remote Sensing Analysis of the Tran Van Thoi District, Ca Mau Province, Vietnam. Remote Sens. 7, 2899–2925. doi: 10.3390/rs70302899
Tullis, J. A., Cothren, J. D., Lanter, D. P., Shi, X., Limp, W. F., Linck, R. F., et al. (2015). “Geoprocessing, workflows, and provenance,” in Remotely Sensed Data Characterization, Classification, and Accuracies, ed. P. Thenkabail, (Boca Raton, FL: CRC Press), 401–421.
USDA (1986). Urban Hydrology for Small Watersheds; Technical Release 55, Version 2.31. Washington, DC: USDA Natural Resource Conservation Service, Engineering Division, 164.
Verberg, P. H., Neumann, K., and Nol, L. (2011). Challenges in using land use and land cover data for global change studies. Global Change Biol. 17, 974–989. doi: 10.1111/j.1365-2486.2010.02307.x
Wang, Y., Mitchell, B., Nugranad-Marzilli, J., and Bonynge, G. (2009). Remote sensing of land-cover change and landscape context of the National Parks: a case study of the Northeast Temperate Network. Remote Sens. Environ. 113, 1453–1461. doi: 10.1016/j.rse.2008.09.017
Xing, L. (2013). “‘Land-Use change in the Mekong region’,” in The Water–Food–Energy Nexus in the Mekong Region: Assessing Development Strategies Considering Cross-Sectoral and Transboundary Impacts, eds A. Smajgl and J. Ward, (New York: Springer), 179–190.
Yang, L., Jin, S., Danielson, P., Homer, C., Gass, L., Case, A., et al. (2018). A new generation of the United States national land cover database: requirements, research priorities, design, and implementation Strategies. ISPRS J. Photogramm. Remote Sens. 146, 108–123. doi: 10.1016/j.isprsjprs.2018.09.006
Yasmi, Y., Durst, P., Haq, R. U., and Broadhead, J. (2017). Forest Change in the Greater Mekong Subregion (GMS): An overview of Negative and Positive drivers. FAO Report. Bangkok.
Keywords: Lower Mekong Basin, land use land cover change, SWAT hydrologic model, agricultural monitoring, deforestation
Citation: Spruce J, Bolten J, Mohammed IN, Srinivasan R and Lakshmi V (2020) Mapping Land Use Land Cover Change in the Lower Mekong Basin From 1997 to 2010. Front. Environ. Sci. 8:21. doi: 10.3389/fenvs.2020.00021
Received: 29 May 2019; Accepted: 17 February 2020;
Published: 19 March 2020.
Edited by:
Daniel Eric Irwin, Marshall Space Flight Center (NASA), United StatesReviewed by:
Jason A. Tullis, University of Arkansas, United StatesPablo Pacheco, World Wildlife Fund, United States
Copyright © 2020 Spruce, Bolten, Mohammed, Srinivasan and Lakshmi. This is an open-access article distributed under the terms of the Creative Commons Attribution License (CC BY). The use, distribution or reproduction in other forums is permitted, provided the original author(s) and the copyright owner(s) are credited and that the original publication in this journal is cited, in accordance with accepted academic practice. No use, distribution or reproduction is permitted which does not comply with these terms.
*Correspondence: Joseph Spruce, j_spruce@outlook.com