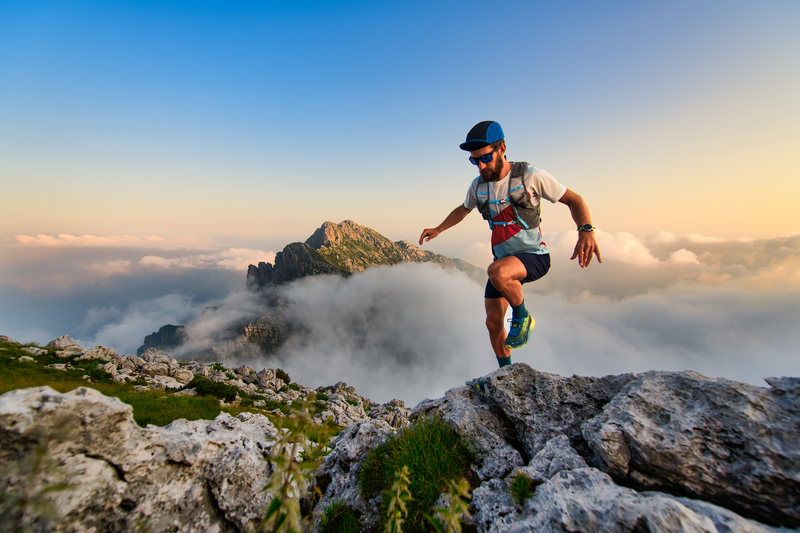
95% of researchers rate our articles as excellent or good
Learn more about the work of our research integrity team to safeguard the quality of each article we publish.
Find out more
ORIGINAL RESEARCH article
Front. Environ. Sci. , 24 April 2019
Sec. Freshwater Science
Volume 7 - 2019 | https://doi.org/10.3389/fenvs.2019.00050
This article is part of the Research Topic Achieving Water-Energy-Food Nexus Sustainability: A Science and Data Need or a Need for Integrated Public Policy? View all 26 articles
Optimizing water–food–energy (WFE) relations has been widely discussed in recent years as an effective approach for formulating pathways toward sustainable agricultural production and energy supply. However, knowledge regarding the WFE nexus is still largely lacking, particularly beyond the conceptual description. In this study, we combined a grid-based crop model (Python-based Environmental Policy Integrated Climate—PEPIC) with a hydropower scheme based on the Distributed Biosphere Hydrological (DBH) model to investigate the WFE interplays in China concerning irrigated agricultural production and hydropower potential. The PEPIC model was used to estimate crop yields and irrigation water requirements under various irrigated cropland scenarios, while the DBH model was applied to simulate hydrological processes and associated hydropower potential. Four major crops, i.e., maize, rice, soybean, and wheat, were included for the analyses. Results show that irrigation water requirements present high values (average about 400 mm yr−1) in many regions of northern China, where crop yields are much higher on irrigated land than on rainfed land. However, agricultural irrigation has largely reduced hydropower potential up to 50% in some regions due to the substantial withdrawal of water from streams. The Yellow River basin, the Hai River basin, and the Liao River basin were identified as the hotspot regions concerning the WFE interactions and tradeoffs. Further expansion the irrigated cropland would increase the tradeoffs between supporting sustainable food production and conserving hydropower potential in many parts of China. The results provide some insights into the WFE nexus and the information derived is useful for supporting sustainable water management, food production while conserving the potential for hydropower generation in China.
Water, food, and energy are the most important resources supporting the development of human society. Due to the highly intrinsic linkages between them, it is essential to manage the three sectors in an integrated way. The Water–Food–Energy (WFE) nexus was emerged as a concept to deal with the complex relations among the three sectors. The WFE nexus was firstly highlighted by the Bonn 2011 Nexus Conference through its background paper (Hoff, 2011). It is vital to optimize the WFE nexus for the purpose of achieving the ambitious Sustainable Development Goals (SDGs) ratified by the United Nations in September 2015, as 10 out of the 17 SDGs are related to the WFE nexus (Bieber et al., 2018). Our planet is facing great challenges to feed the growing and increasingly affluent population. Thinking and acting with a WFE concept is the key to improving overall resource use efficiency (Ringler et al., 2013). However, current research on the WFE nexus is still on the initial phase with a large number of review papers focusing on clarifying its definition and out looking the major research directions (Perrone and Hornberger George, 2014; Smajgl et al., 2016; Liu J. et al., 2017; Cai et al., 2018; D'odorico et al., 2018). Without detailed understanding of the WFE nexus and tradeoffs, it is difficult to use the WFE concept to facilitate the success of SDGs by 2030 (Galaitsi et al., 2018).
Water, especially that for irrigation, is recognized as the central position in framing the WFE nexus (Cai et al., 2018; D'odorico et al., 2018). As the largest water consumer, irrigation accounts for about 70% of global water withdrawal and is responsible for 40% of total grain production (Ringler et al., 2013). Hydropower is the most important renewable energy resources, which receive increasing attention worldwide (Stickler et al., 2013; Liu et al., 2016c). There is a conflict between irrigation water withdrawal and hydropower generation, especially in dry seasons. For instance, Zeng et al. (2017) found that 54% of global installed hydropower has competitive relationships with irrigation. On the other hand, irrigation pumping could be high energy consuming. Concerning resource use efficiency, optimizing the WFE nexus does not always correspond to maximum crop yields, with the potential to save water and energy (Zhang et al., 2017). Therefore, using the irrigation as a connection provides good case to demonstrate the WFE nexus and understand its complex interplays.
China is particularly facing great challenges associated with optimizing the WFE, as it has to use <10% of the global arable land to feed one fifth of the global population. Water resources distribute extremely unevenly in China, with very low water availability in the northern parts of the country. Furthermore, irrigation is important to increase crop yields there (Piao et al., 2010). The annual average of gross hydropower potential in mainland China was estimated as high as 650 GW (billion watt) over the period 1971–2000 (Liu et al., 2016c). However, it is still lacking in the literature that explores the extent to which the WFE nexus is interrelated and which regions are facing more challenges. In order to fill in this research gap, we used a unique approach by coupling a grid-based large-scale crop model (Python-based Environmental Policy Integrated Climate, PEPIC) with a hydrological model (Distributed Biosphere Hydrological, DBH) to estimate irrigation water requirements, crop yields, and hydropower potential in mainland China. The WFE interplays were investigated by considering various irrigation scenarios with respect to four major crops: maize, rice, soybean, and wheat. The study will identify the hotspot regions regarding the WFE nexus and provide a preliminary reference for the integrated resources management in China.
The PEPIC model (Liu et al., 2016a) was used to simulate irrigation water requirements and crop yields. PEPIC is a grid version of the EPIC (Environmental Policy Integrated Climate) model (Williams et al., 1984). The EPIC model was initially developed to estimate the effects of soil erosion on soil productivity in the mid-1980s. Since its inception, EPIC was continuously extended to simulate a wide range of complex processes related to crop growth, e.g., hydrology, soil erosion, soil temperature, carbon dynamics, and nutrient cycles (Williams, 1995; Izaurralde et al., 2006). EPIC has been widely used and validated around the world (Gassman et al., 2005). However, EPIC is a field level model. The grid-based PEPIC model facilitates the application of EPIC on large scales with high spatial resolutions. The PEPIC model has been used to simulate crop yields, crop water use, and irrigation water requirements (Liu et al., 2016a). It performed well on simulating national crop yields, which match the data reported by FAO around year 2000. The PEPIC model can also capture the interannual variability of crop yields caused by climate forcing (Müller et al., 2017). Beyond this, PEPIC was successfully used to simulate global nutrient cycling, e.g., nitrogen losses (Liu et al., 2016b), and phosphorus cycles (Liu et al., 2018) relating to production of major crops on a global scale. We used the calibrated PEPIC in this study.
PEPIC simulates crop growth at a daily scale. Daily potential biomass is simulated by considering an energy–biomass conversion approach. Potential biomass is reduced by a major plant stress (including temperature, water, nutrient, aeration, and salinity) to get the actual biomass. Crop yields are then estimated by multiplying a crop-specific harvest index and actual biomass accumulation when crop is mature. In this study, both rainfed and irrigated cultivations were simulated separately. For irrigated cropland, irrigation water requirements were estimated by using an automatic irrigation application approach. With this method, PEPIC applies water for irrigation automatically with sufficient amount of water when plant water stress limits potential biomass increases by a given threshold, e.g., 10% used in this study. This strategy can eliminate the plant water stress and is widely used in large scale crop modeling (Folberth et al., 2016; Liu et al., 2016b). The annual irrigation water requirements were calculated by summing up the applied water in each irrigation event during the whole growing season. An irrigation efficiency of 0.378 (Rost et al., 2008) was used for the whole of China in this study.
PEPIC requires digital elevation model (DEM), slope, climate, soil, fertilizer, and crop calendar as input data. Climate data, including precipitation, maximum and minimum daily temperature, wind speed, and relative humidity, were obtained from Weedon et al. (2014). Soil data were downloaded from the ISRIC-WISE dataset (Batjes, 2006). Fertilizer data, including nitrogen and phosphorus of mineral fertilizer and manure, were derived from the EarthStat dataset (Mueller et al., 2012; West et al., 2014). Crop calendar, including planting date and crop growth period, was based on the SAGE dataset (Sacks et al., 2010). The simulation period of this study is 1981–2010 with the first 20 years treating as spin-off period to phase out the impacts of unknown initial soil conditions. Four major crops, maize, rice, soybean and wheat, were simulated in the mainland of China with a spatial resolution of 0.5°.
A hydropower scheme (HPS) was developed based on the DBH model to estimate the gross hydropower potential (GHP) under different irrigation scenarios. The DBH model is a spatially distributed model integrating hydrological processes and soil-vegetation-atmosphere transfer processes (Tang et al., 2007, 2008). It incorporates a land surface model SiB2 (Sellers et al., 1996) and a distributed hydrological scheme. The hydrological scheme in the DBH model is based on geomorphological characteristics to estimate the surface and subsurface flow. Both saturated and unsaturated overland flows are considered in the model. The area-amount relationship for effective precipitation and the part of precipitation that becomes runoff, is used to estimate the overland flow. A linear reservoir routing model is used for large scale hydrological routing simulation (Liu et al., 2016d; Liu X. et al., 2017). The DBH model was initially developed and calibrated for large-scale hydrological simulations in the Yellow River basin (Tang et al., 2008) and showed fairly good performance. It was then improved for hydrological simulations taking human impact into account at a spatial resolution of half-degree and was verified in China (Liu et al., 2016c, 2019) and the globe (Liu et al., 2016d; Liu X. et al., 2017) with monthly and annual hydrological observations.
GHP is defined as the total energy of available runoff falling to the lowest level of a specific region. Based on the DBH model, flows for GHP estimation are considered from (1) cell-internal runoff (Q1) that falls from the mean to the minimum elevation (h1) of the considered cell and (2) inflow (Q2) that falls from the minimum elevation of the upstream cell to the minimum elevation (h2) of the considered cell (Liu et al., 2016c). In the HPS, GHP at each grid cell is estimated as:
where Q1 and Q2 are the cell-internal flow and the inflow (m3 s−1, cubic meter per second), respectively; h1 and h2 are the hydraulic head defined above (m); and g is gravitational acceleration (m s−2).
The HPS was coupled with the PEPIC model to represent the links between hydropower and agricultural irrigation at large scale (Figure 1). To do this, HPS was fed with irrigation water requirements at the monthly scale, which were estimated by the PEPIC model. HPS runs at a daily time step; therefore, the monthly irrigation requirements were evenly disaggregated into daily values. The estimated irrigation water requirements by the PEPIC model were provided for the flow routing process in the DBH model. Discharge was withdrawn from the considered cell and then its adjacent grid cells (up to four adjacent grid cells) if necessary to fulfill the irrigation requirements before the GHP estimation. GHP was calculated based on the remaining discharge and the natural hydraulic head along the river network. At each grid cell, 20% of daily streamflow was arbitrarily reserved for environmental flows (Hanasaki et al., 2008; Pastor et al., 2014). The withdrawn water in HPS will be lower than the estimated irrigation water requirements if streamflow is not sufficient. Annual GHP was aggregated based on daily values at each grid cell. In this study, we focused on GHP and no reservoir regulation was applied in the HPS. The feedback of irrigation on runoff generation was not considered, from which uncertainty in discharge simulations may arise in some regions (Liu et al., 2019).
In this study, the MIRCA-2000 land use data (Portmann et al., 2010) were used as the benchmark of cropland for wheat, rice, maize, and soybean. The MIRCA-2000 dataset provides crop-specific irrigated and rainfed land use data for 26 crops throughout the whole world around the period 1997–2003. We considered 12 irrigated cropland scenarios on the basis of the MIRCA-2000 dataset: the baseline scenario (represents the reality around year 2000, then the national average irrigated cropland was about 70% of the total cropland, with substantial regional variations); the zero scenario (no irrigated land, that is, the whole cropland as rainfed land), and 10 incremental irrigated cropland (i.e., 10, 20, 30, 40, 50, 60, 70, 80, 90, and 100%) scenarios, which take respective fractions of total cropland to irrigated land in each grid. It should be noted that the baseline scenario is different from the 70% incremental irrigated cropland scenario, as the later considers the equal percentage of cropland as irrigated land in all the river basins and grids. In the study, we kept the total cropland area unchanged, but adjusted the fraction of irrigated land to total cropland. These 12 irrigated cropland scenarios were used to examine the effects of varied irrigation water withdrawals on the hydropower potentials for mainland China and its 10 major river basins (Figure 2A).
Figure 2. Maps of irrigation water requirements over cropland (A) and total irrigation land area in each 0.5 degree grid cell (B) of the four crops. Irrigation water requirements were estimated by considering the whole cropland (the MIRCA-2000 dataset) as irrigated land for each crop and then aggregated by using area-weighted average of maize, rice, soybean, and wheat. Ten large river basins (presenting on the top panel) in the mainland of China were used to aggregated regional information, including the Hai River basin (HaiR), the Huai River basin (HuaiR), the Liao River basin (LiaoR), the Northwest River basins (NwR), the Pearl River basin (PeR), the Southeast River basins (SeR), the Songhua River basin (ShR), the Southwest River basins (SwR), the Yangtze River basin (YaR), and the Yellow River basin (YeR).
Figure 2A shows the spatial distribution of area-weighted average of irrigation water requirements of the four crops, under the 100% irrigated cropland scenario, representing the max irrigation water requirements. Irrigation water requirements of the four major crops present very high values (400 mm yr−1, millimeter per year) in the north parts of China, e.g., in the Hai River basin, the Liao River basin, the middle of the Yellow River basin, and the north part of the Huai River basin. The highest values are found in the northwest part of China with irrigation water requirements >500 mm yr−1. On the other hand, the irrigation water requirements are small in the southern parts of China, with values generally below 100 mm yr−1.
Irrigated cropland based on the MIRCA-2000 dataset (the baseline scenario) mainly located in the north parts of China, especially in the Hai River basin, the Huai River basin, and the Yellow River basin with high values over 200 kha (thousand hectares) in one grid (Figure 2B). Multiplying the irrigation water requirements with irrigated cropland of the four major crops, the Hai River basin required the largest amount of water up to 18 km3 yr−1 (cubic kilometer per year), followed by the Yellow River basin (13 km3 yr−1), the Northwest River basins (10 km3 yr−1), and the Huai River basin (10 km3 yr−1) (Table 1). The Yangtze River basin has the largest irrigated cropland area in total, while its irrigation water requirements are relatively small due to the relatively high rainfall (3.1 km3 yr−1).
The area-weighted average of rainfed yields (the zero scenario) of the four major crops shows high values in the east parts of China, over 5 ton ha−1 yr−1 (ton per hectare per year), while the rainfed yields are particularly low (< 1 ton ha−r yr−1) in the northwest parts of China (Figure 3A). Therefore, irrigation in these low rainfed yield regions can greatly improve crop yields, especially in the Northwest River basins and the middle of the Yellow River basin (Figure 3B). It is not surprising that the differences in crop yields (Figure 3B) between the full irrigated and full rainfed cultivation show similar spatial patterns to the irrigation water requirements under the 100% scenarios (Figure 2A). At the river basin level, irrigation under the baseline scenario can increase crop production by 5.5 times of that under the zero scenario in the Northwest River basins, followed by the Yellow River basin (by 40%), and the Hai River basin (by 38%) (Table 1). As for the whole mainland of China, current irrigation (the baseline scenario) increases its crop production by 13%.
Figure 3. Maps of rainfed crop yields (A) and differences between irrigated crop yields and rainfed crop yields (B) of maize, rice, soybean, and wheat. Rainfed crop yields were estimated by considering the whole cropland (the MIRCA-2000 dataset) as rainfed land for each crop and then aggregated by using area-weighted average of the four crops. Irrigated crop yields were estimated by using the same way as rainfed crop yields but considering the whole cropland as irrigated land.
Under the zero irrigation scenario, i.e., without irrigation water withdrawal, the hydropower potential is mainly concentrated in the southwest parts of China (Figure 4A), including the Southwest River basins and the head of the Yangtze River basin, with high values >1,000 MW (million watt). This is mainly because there are abundant water resources and large elevation differences in these areas. The hydropower potential is relatively small in the north parts of China, especially in the vast regions of the Northwest River basins, which is lower than 100 MW.
Figure 4. Maps of hydropower potential under the zero irrigation scenario (A) and percentage reduction in hydropower potential between the baseline and zero irrigation scenarios (B).
Water used for irrigation under the baseline scenario has largely reduced the hydropower potential in the north parts of China (Figure 4B). In some areas of the Northwest River basins and the Yellow River basin, the percentage reduction in hydropower potential is more than 50%. At the river basin level, the baseline irrigation results in the largest reduction of hydropower potential in the Yellow River basin by 10,354 MW (Table 1), which accounts for about 17% of its hydropower potential in the condition of zero irrigation. The percentage reductions in hydropower potential in the Hai River basin, the Liao River basin, and the Huai River basin are 11, 10, and 6%, respectively. At the national level, the percentage reduction is only 1.8%, mainly because the reduction in hydropower potential is very small in the four southern river basins of China, i.e., the Southwest River basins, the Yangtze River basin, the Pearl River basin, and the Southeast River basins, where the irrigation water requirements are very small relative to their water resources.
Agricultural irrigation has strong effects on the WFE nexus in the north parts of China, especially in the Yellow River basin, the Hai River basin, and Liao River basin, i.e., increases in crop production by more than 10% accompanied with reduction in hydropower potential by over 10%. Besides, the Northwest River basins and the Huai River basin have also relative strong WFE nexus. Crop production increases by 560% in the Northwest River basins due to irrigation, although the reduction in hydropower potential is relatively small (2.2%). In the Huai River basin, both the increase in crop production and the reduction in hydropower potential reach 6% (Table 1). Therefore, we focus on these five hotspot river basins and the mainland China to further investigate the impacts of irrigation under 10 different scenarios on the WFE nexus (Figure 5). Generally, the impacts of irrigation on the WFE nexus get more evident with increasing irrigated areas. The Yellow River basin demonstrates the most significant responses, that is, crop production could increase by 53% under the 100% irrigation scenario compared to crop production under the zero scenario, while hydropower potential would decrease by 25%. At the national level, crop production would have 16% increases and reduction in hydropower potential is about 2.8%.
Figure 5. The water-food-energy nexus under different irrigated cropland scenarios for Hai River basin (HaiR, A), Huai River basin (HuaiR, B), Liao River basin (LiaoR, C), Northwest River basins (NwR, D), Yellow River basin (YeR, E) and the mainland China (Nation, F). Increases in crop production: percentage increases in crop production between different irrigation scenarios and the zero scenario. Reduction in hydropower potential: percentage reduction in hydropower potential between different irrigation scenarios and the zero scenario. Color presents different irrigated cropland scenarios. Size presents irrigation water supply. Baseline fraction means the fraction of irrigated cropland area under the baseline scenario to the total cropland area. HaiR, Hai River basin; HuaiR, Huai River basin; LiaoR, Liao River basin; NwR, Northwest River basins; YeR, Yellow River basin; Nation: mainland of China. Location of each river basin is described in Figure 2A.
For the five presented basins and also the mainland China in Figure 5 except the Liao River basin, points for the baseline scenario locate at the upper and left side of the points for the other different irrigation fraction scenarios. This means that the distribution of current irrigation land (the baseline scenario) has advantages on the WFE nexus over the indiscriminate irrigation fraction scenarios. For example, about 86% of total cropland in the Hai River basin is irrigated land under the baseline scenario. If we consider 86% of total cropland in each grid as irrigated land, irrigation would result in the same increases (about 38%) in crop production compared to the baseline scenario, while it may cause about 2% more reduction in hydropower potential relative to the baseline condition.
The WFE nexus shows various tradeoffs in terms of increasing crop production with irrigation and conserving hydropower potential in China (Table 1). Generally, the northern parts of China have stronger WFE interactions than the southern parts of China. This is mainly because growing season precipitation in the south parts of China is much higher than that in the north parts of China (Liu et al., 2018), hence crop production only faces slight water deficiency and less irrigation water is required. Another reason is due to the high amount of hydropower potential in the south parts of China (Figure 4A). The reduction in hydropower potential caused by irrigation has therefore little effects on the overall hydropower potential in these regions. In contrast, the north parts of China demonstrate strong WFE tradeoffs. We identified the Yellow River basin, the Hai River basin, and the Liao River basin as the hotspot regions regarding the WFE nexus.
By considering high fractions of total cropland as irrigated land, e.g., the 70, 80, 90, and 100% scenarios, we found that the reductions in hydropower potential are less significant than that under the lower fraction scenarios, e.g., 10, 20, and 30%, in the Huai River basin and the Northwest River basins (Figure 5). For instance, the percentage reductions in hydropower potential are 1.2 and 0.3% between 10 and 20% irrigation scenarios for these two basins, respectively. But they decrease to only 0.5 and 0.2% between 90 and 100% irrigation scenarios. It indicates that streamflow is not sufficient to support irrigation water withdrawal under the high irrigation fraction levels in these regions and hence less reduction in hydropower potential is observed. In these cases, reservoir regulation or groundwater withdrawal is necessary to compensate surface water insufficiency for irrigation (Siebert et al., 2010). Reservoir regulation is not considered since we mainly focus on GHP in this study. However, a previous study (Liu et al., 2016c) showed that the changes in hydropower based on reservoirs were often consistent with the GHP changes. Therefore, here we can infer similar changes in hydropower potential based on existing reservoirs/hydropower facilities as those in the GHP. Nevertheless, further investigation is needed to address the important role of reservoirs in optimizing the WFE nexus. As a large amount of energy is consumed to pump groundwater for irrigation (Scott, 2013), groundwater consumption for irrigation provides another dimension of the WFE nexus in comparison to surface water consumption. Merging surface water and groundwater into an integrated research system can demonstrate a more comprehensive picture of the WFE nexus and deserves detailed investigation in future studies.
We found that the baseline irrigation pattern performs better in terms of effects on hydropower potential than the indiscriminate irrigation fraction of total cropland in the northern basins. However, in the Liao River basin, it is not the case. The baseline irrigation land accounts for 65% of total cropland. Although the baseline irrigation scenario has higher increases in crop production than that when 65% of cropland as irrigation land in each grid, interpolated from the trend line in Figure 5, the percentage reduction in hydropower potential under the baseline scenario is more than the percentage increase in crop production. It implies that there are spaces to optimize the current irrigation patterns for enhancing the WFE nexus there. Transforming more cropland into irrigated land in the regions with higher increase in crop yields and lower reduction in hydropower potential can be a possible pathway toward irrigated land optimization. As climate conditions can have impacts on WFE nexus (Conway et al., 2015; Berardy and Chester, 2017), especially due to the more frequent drought events (Zhang et al., 2017; Cai et al., 2018), such optimization is essential to improve the overall resource use efficiency in the framework of the WFE nexus (Ringler et al., 2013). However, a detailed analysis of optimizing irrigation patterns is beyond the scope of this study.
We acknowledge some limitations in this study. We only considered four major crops in the investigation. Excluding other crops would have some impacts on the analysis the WFE nexus. For example, irrigation water requirements for cotton production are much higher than that for maize and wheat cultivation in the northwest parts of China (Shen et al., 2013). The impact of irrigation on hydropower potential could be largely underestimated without considering cotton. Cotton was excluded from the analysis mainly because it is not a food crop and this study focuses on the tradeoffs between food production and hydropower potential. Besides, the irrigated areas of the four crops in the whole of China account for about 80% of the total irrigated areas of the 26 major crops in the country included in Portmann et al. (2010). Based on our estimation, for all the 26 crops, the total irrigation water requirements will be 25% higher. Consequently, the reduction of hydropower potential will be ~25% higher. Also, the estimated values by large-scale models are subject to high uncertainties due to model structure and parameters. For example, a previous study shows that the selection of different potential evapotranspiration methods built-in PEPIC can have large effect on the estimation of crop yields and irrigation water requirements (Liu et al., 2016a). Large uncertainty arisen from hydrological models including DBH were reported (Schewe et al., 2014; Liu X. et al., 2017), which indicates that improving model structure and parameters is needed. Although the uncertainty issue is out of scope of this preliminary analysis, it is in our agenda to address this issue in detail. Despite these limitations, this study provides the first attempt to illustrate the WFE nexus with respect to water, food, and hydropower potential relations. The information is of importance for understanding the WFE nexus in China and for formulating appropriate policies to tackle the nexus related challenges.
In this study, the PEPIC crop model was coupled with the DBH hydrological model to investigate the WFE nexus in mainland China under various irrigated cropland scenarios. The northern parts of China show strong WFE nexus and tradeoffs due to large amount of irrigation water requirements and relatively low water resources there, while irrigation had only little effects on the WFE nexus in the southern basins of China. The Yellow River basin, the Hai River basin, and the Liao River basin were identified as the hotspot regions regarding the tradeoffs in the WFE nexus, where more attention should be paid for detailed investigation. The current irrigated cropland generally presents good performance compared to the indiscriminate irrigation fraction of total cropland. Still, there are spaces for improving the distribution of irrigated cropland to maximize the WFE benefits. Complexity and uncertainties in studying the WFE nexus call for more comprehensive research to promote the usefulness of this concept as a robust tool for managing emerging challenges related to integrated and efficient management of water, food, and energy sectors.
This paper addressed the WFE nexus by specifying quantitatively the tradeoffs between food production increases through irrigation expansion (increased water withdrawal) and the loss of hydropower potential due to the reduction of streamflow. The information is useful for supporting integrated management of water resources for energy and food sustainability in China. However, the study did not go further to address the economic/social significance of the tradeoffs, as such analysis would be location/river basin specific. It is beyond the scope of this study to judge whether a specific river basin/region/country should choose to forego its hydropower potential in order to gain more food production. Such a decision would need much more information on socioeconomic conditions, regional development strategies, environmental status, etc. This would be the topic of our future study. Finally, we should like to mention that although this study focuses on China, the approaches developed can be used in other countries and basins in the world for addressing the WFE nexus quantitatively.
WL, HY, and XL designed the research. WL run the PEPIC model and analyzed the data and wrote the manuscript. XL run the DBH model. All authors participated in the interpretation of results and the writing and editing processes.
The authors declare that the research was conducted in the absence of any commercial or financial relationships that could be construed as a potential conflict of interest.
This study was supported by the funding from the Swiss Federal Institute of Aquatic Science and Technology (Eawag) and the World Food System Center at ETH Zürich. WL acknowledges the support received from the Early Postdoctoral Mobility Fellowship awarded by the Swiss National Science Foundation (P2EZP2_175096). XL was supported by funding from the National Natural Science Foundation of China (41877164). QT was supported by funding from the National Natural Science Foundation of China (41730645).
Batjes, N. H. (2006). ISRIC-WISE Derived Soil Properties on a 5 by 5 Arc-Minutes Global Grid (version 1.1). Wageningen: ISRIC–World Soil Infromation.
Berardy, A., and Chester, M. V. (2017). Climate change vulnerability in the food, energy, and water nexus: concerns for agricultural production in Arizona and its urban export supply. Environmental Research Letters 12, 035004. doi: 10.1088/1748-9326/aa5e6d
Bieber, N., Ker, J. H., Wang, X., Triantafyllidis, C., Van Dam, K. H., Koppelaar, R. H. E. M., et al. (2018). Sustainable planning of the energy-water-food nexus using decision making tools. Energy Policy 113, 584–607. doi: 10.1016/j.enpol.2017.11.037
Cai, X., Wallington, K., Shafiee-Jood, M., and Marston, L. (2018). Understanding and managing the food-energy-water nexus–opportunities for water resources research. Adv. Water Resour. 111, 259–273. doi: 10.1016/j.advwatres.2017.11.014
Conway, D., Van Garderen, E. A., Deryng, D., Dorling, S., Krueger, T., Landman, W., et al. (2015). Climate and southern Africa's water–energy–food nexus. Nat. Clim. Chang. 5, 837–846. doi: 10.1038/nclimate2735
D'odorico, P., Davis, K. F., Rosa, L., Carr, J. A., Chiarelli, D., Dell'angelo, J., et al. (2018). The global food-energy-water nexus. Rev. Geophys. 56, 456–531. doi: 10.1029/2017RG000591
Folberth, C., Skalsky, R., Moltchanova, E., Balkovic, J., Azevedo, L. B., Obersteiner, M., et al. (2016). Uncertainty in soil data can outweigh climate impact signals in global crop yield simulations. Nat. Commun. 7:11872. doi: 10.1038/ncomms11872
Galaitsi, S., Veysey, J., and Huber-Lee, A. (2018). “Where is the added value? A review of the water-energy-food nexus literature,” in: SEI Working Paper (Stockholm: Stockholm Environment Institute).
Gassman, P. W., Williams, J. R., Benson, V. W., Izaurralde, R. C., Hauck, L. M., Jones, C. A., et al. (2005). Historical development and applications of the EPIC and APEX Models. Ames: Working Paper 05-WP 397.
Hanasaki, N., Kanae, S., Oki, T., Masuda, K., Motoya, K., Shirakawa, N., et al. (2008). An integrated model for the assessment of global water resources–part 1: model description and input meteorological forcing. Hydrol. Earth Syst. Sci. 12, 1007–1025. doi: 10.5194/hess-12-1007-2008
Hoff, H. (2011). “Understanding the Nexus,” in Background Paper for the Bonn 2011 Nexus Conference: The Water, Energy and Food Security Nexus (Stockholm: Stockholm Environment Institute).
Izaurralde, R. C., Williams, J. R., Mcgill, W. B., Rosenberg, N. J., and Jakas, M. C. Q. (2006). Simulating soil C dynamics with EPIC: model description and testing against long-term data. Ecol. Modell. 192, 362–384. doi: 10.1016/j.ecolmodel.2005.07.010
Liu, J., Yang, H., Cudennec, C., Gain, A. K., Hoff, H., Lawford, R., et al. (2017). Challenges in operationalizing the water–energy–food nexus. Hydrol. Sci. J. 62, 1714–1720. doi: 10.1080/02626667.2017.1353695
Liu, W., Yang, H., Ciais, P., Stamm, C., Zhao, X., Williams, J. R., et al. (2018). Integrative crop-soil-management modeling to assess global phosphorus losses from major crop cultivations. Glob. Biogeochem. Cycles 32, 1074–1086. doi: 10.1029/2017GB005849
Liu, W., Yang, H., Folberth, C., Wang, X., Luo, Q., and Schulin, R. (2016a). Global investigation of impacts of PET methods on simulating crop-water relations for maize. Agric. For. Meteorol. 221, 164–175. doi: 10.1016/j.agrformet.2016.02.017
Liu, W., Yang, H., Liu, J., Azevedo, L. B., Wang, X., Xu, Z., et al. (2016b). Global assessment of nitrogen losses and trade-offs with yields from major crop cultivations. Sci. Total Environ. 572, 526–537. doi: 10.1016/j.scitotenv.2016.08.093
Liu, X., Liu, W., Yang, H., Tang, Q., Flörke, M., Masaki, Y., et al. (2019). Multimodel assessments of human and climate impacts on mean annual streamflow in China. Hydrol. Earth Syst. Sci. 23, 1245–1261. doi: 10.5194/hess-23-1245-2019
Liu, X., Tang, Q., Cui, H., Mu, M., Gerten, D., Gosling, S. N., et al. (2017). Multimodel uncertainty changes in simulated river flows induced by human impact parameterizations. Environ. Res. Lett. 12:025009. doi: 10.1088/1748-9326/aa5a3a
Liu, X., Tang, Q., Voisin, N., and Cui, H. (2016c). Projected impacts of climate change on hydropower potential in China. Hydrol. Earth Syst. Sci. 20, 3343–3359. doi: 10.5194/hess-20-3343-2016
Liu, X., Tang, Q., Zhang, X., and Leng, G. (2016d). Modeling the Role of Vegetation in Hydrological Responses to Climate Change. Hoboken, NJ: Wiley & Sons, Inc. doi: 10.1002/9781118971772.ch10
Mueller, N. D., Gerber, J. S., Johnston, M., Ray, D. K., Ramankutty, N., and Foley, J. A. (2012). Closing yield gaps through nutrient and water management. Nature 490, 254–257. doi: 10.1038/nature11420
Müller, C., Elliott, J., Chryssanthacopoulos, J., Arneth, A., Balkovic, J., Ciais, P., et al. (2017). Global gridded crop model evaluation: benchmarking, skills, deficiencies and implications. Geosci. Model Dev. 10, 1403–1422. doi: 10.5194/gmd-10-1403-2017
MWRC the Ministry of Water Resources of the People's Republic of China. (2007). The Bulletin of Water Resources of China 2006 [in Chinese].
Pastor, A. V., Ludwig, F., Biemans, H., Hoff, H., and Kabat, P. (2014). Accounting for environmental flow requirements in global water assessments. Hydrol. Earth Syst. Sci. 18, 5041–5059. doi: 10.5194/hess-18-5041-2014
Perrone, D., and Hornberger George, M. (2014). Water, food, and energy security: scrambling for resources or solutions? Wiley Interdiscipl. Rev. Water 1, 49–68. doi: 10.1002/wat2.1004
Piao, S. L., Ciais, P., Huang, Y., Shen, Z. H., Peng, S. S., Li, J. S., et al. (2010). The impacts of climate change on water resources and agriculture in China. Nature 467, 43–51. doi: 10.1038/nature09364
Portmann, F. T., Siebert, S., and Doll, P. (2010). MIRCA2000-global monthly irrigated and rainfed crop areas around the year 2000: a new high-resolution data set for agricultural and hydrological modeling. Global Biogeochem. Cycles 24:GB1011. doi: 10.1029/2008GB003435
Ringler, C., Bhaduri, A., and Lawford, R. (2013). The nexus across water, energy, land and food (WELF): potential for improved resource use efficiency? Curr. Opin. Environ. Sustain. 5, 617–624. doi: 10.1016/j.cosust.2013.11.002
Rost, S., Gerten, D., Bondeau, A., Lucht, W., Rohwer, J., and Schaphoff, S. (2008). Agricultural green and blue water consumption and its influence on the global water system. Water Resour. Res. 44:W09405. doi: 10.1029/2007WR006331
Sacks, W. J., Deryng, D., Foley, J. A., and Ramankutty, N. (2010). Crop planting dates: an analysis of global patterns. Global Ecol. Biogeogr. 19, 607–620. doi: 10.1111/j.1466-8238.2010.00551.x
Schewe, J., Heinke, J., Gerten, D., Haddeland, I., Arnell, N. W., Clark, D. B., et al. (2014). Multimodel assessment of water scarcity under climate change. Proc. Nat. Acad. Sci. U.S.A. 111, 3245–3250. doi: 10.1073/pnas.1222460110
Scott, C. A. (2013). Electricity for groundwater use: constraints and opportunities for adaptive response to climate change. Environ. Res. Lett. 8:035005. doi: 10.1088/1748-9326/8/3/035005
Sellers, P. J., Randall, D. A., Collatz, G. J., Berry, J. A., Field, C. B., Dazlich, D. A., et al. (1996). A revised land surface parameterization (SiB2) for atmospheric GCMs 0.1. Model formulation. J. Clim. 9, 676–705. doi: 10.1175/1520-0442(1996)009<0676:ARLSPF>2.0.CO;2
Shen, Y. J., Li, S., Chen, Y. N., Qi, Y. Q., and Zhang, S. W. (2013). Estimation of regional irrigation water requirement and water supply risk in the arid region of Northwestern China 1989–2010. Agric. Water Manag. 128, 55–64. doi: 10.1016/j.agwat.2013.06.014
Siebert, S., Burke, J., Faures, J. M., Frenken, K., Hoogeveen, J., Doll, P., et al. (2010). Groundwater use for irrigation–a global inventory. Hydrol. Earth Syst. Sci. 14, 1863–1880. doi: 10.5194/hess-14-1863-2010
Smajgl, A., Ward, J., and Pluschke, L. (2016). The water–food–energy nexus – realising a new paradigm. J. Hydrol. 533, 533–540. doi: 10.1016/j.jhydrol.2015.12.033
Stickler, C. M., Coe, M. T., Costa, M. H., Nepstad, D. C., McGrath, D. G., Dias, L. C. P., et al. (2013). Dependence of hydropower energy generation on forests in the Amazon Basin at local and regional scales. Proc. Natl. Acad. Sci. U.S.A. 110, 9601–9606. doi: 10.1073/pnas.1215331110
Tang, Q. H., Oki, T., Kanae, S., and Hu, H. P. (2007). The influence of precipitation variability and partial irrigation within grid cells on a hydrological simulation. J. Hydrometeorol. 8, 499–512. doi: 10.1175/JHM589.1
Tang, Q. H., Oki, T., Kanae, S., and Hu, H. P. (2008). Hydrological cycles change in the yellow river basin during the last half of the twentieth century. J. Clim. 21, 1790–1806. doi: 10.1175/2007JCLI1854.1
Weedon, G. P., Balsamo, G., Bellouin, N., Gomes, S., Best, M. J., and Viterbo, P. (2014). The WFDEI meteorological forcing data set: WATCH Forcing Data methodology applied to ERA-Interim reanalysis data. Water Resour. Res. 50, 7505–7514. doi: 10.1002/2014WR015638
West, P. C., Gerber, J. S., Engstrom, P. M., Mueller, N. D., Brauman, K. A., Carlson, K. M., et al. (2014). Leverage points for improving global food security and the environment. Science 345, 325–328. doi: 10.1126/science.1246067
Williams, J. R. (1995). “The EPIC model,” in Computer Models of Watershed hydrology, ed V. P. Singh (Highlands Ranch: Water Resources Publications), 909–1000.
Williams, J. R., Jones, C. A., and Dyke, P. T. (1984). A modeling approach to determining the relationship between erosion and soil productivity. Trans. Asae 27, 129–144. doi: 10.13031/2013.32748
Zeng, R., Cai, X., Ringler, C., and Zhu, T. (2017). Hydropower versus irrigation—an analysis of global patterns. Environ. Res. Lett. 12:034006. doi: 10.1088/1748-9326/aa5f3f
Keywords: water–food–energy nexus, irrigation water requirements, crop yields, hydropower potential, PEPIC, DBH
Citation: Liu W, Yang H, Tang Q and Liu X (2019) Understanding the Water–Food–Energy Nexus for Supporting Sustainable Food Production and Conserving Hydropower Potential in China. Front. Environ. Sci. 7:50. doi: 10.3389/fenvs.2019.00050
Received: 15 August 2018; Accepted: 01 April 2019;
Published: 24 April 2019.
Edited by:
Rabi Mohtar, Texas A&M University, United StatesReviewed by:
Fouad H. Jaber, Texas A&M University, United StatesCopyright © 2019 Liu, Yang, Tang and Liu. This is an open-access article distributed under the terms of the Creative Commons Attribution License (CC BY). The use, distribution or reproduction in other forums is permitted, provided the original author(s) and the copyright owner(s) are credited and that the original publication in this journal is cited, in accordance with accepted academic practice. No use, distribution or reproduction is permitted which does not comply with these terms.
*Correspondence: Xingcai Liu, eGluZ2NhaWxpdUBpZ3NucnIuYWMuY24=
Disclaimer: All claims expressed in this article are solely those of the authors and do not necessarily represent those of their affiliated organizations, or those of the publisher, the editors and the reviewers. Any product that may be evaluated in this article or claim that may be made by its manufacturer is not guaranteed or endorsed by the publisher.
Research integrity at Frontiers
Learn more about the work of our research integrity team to safeguard the quality of each article we publish.