- 1W. A. Franke College of Forestry and Conservation, University of Montana, Missoula, MT, United States
- 2Institute for Environmental Science, University of Geneva, Geneva, Switzerland
- 3Center for Large Landscape Conservation, Bozeman, MT, United States
- 4National Socio-Environmental Synthesis Center (SESYNC), University of Maryland, Annapolis, MD, United States
- 5Luc Hoffmann Institute, IUCN Conservation Centre, Gland, Switzerland
As the water-energy-food (WEF) nexus becomes an increasingly common framework for bridging science and policy, there is a growing need to unpack and make explicit many of the methods and assumptions being used to operationalize the nexus. In this paper, we focus on two common approaches to nexus research, quantitative modeling and futures thinking, and the ways that each set of methodological tools address uncertainty. We first review the underlying assumptions of each approach with a focus on sources of and ability to measure uncertainty, and potential complementarities. Quantitative modeling takes a probabilistic approach to predicting the likelihood of a specific outcome or future state based on estimates of current system dynamics. In contrast, futures thinking approaches, such as scenario processes, explore novel changes that cannot be fully predicted or even anticipated based on current understandings of the nexus. We then examine a set of applied nexus projects that bridge science and policy-making contexts to better understand practitioner experiences with different methodological tools and how they are utilized to navigate uncertainty. We explore one nexus case study, LIVES Cambodia, in-depth, to better understand the opportunities and challenges associated with participatory modeling and stakeholder engagement with uncertainty in a policy-making context. Across the cases, practitioners identify the complementarity between modeling and futures thinking approaches, and those projects that integrated both into the planning process experienced benefits from having multiple angles on uncertainty within the nexus. In particular, stakeholder engagement provided critical opportunities to address some types of uncertainties (e.g., data gaps) through the use of local knowledge. Explicit discussions of model uncertainty and use of scenario processes also enabled stakeholders to deepen their understandings of uncertainties and envision policy pathways that would be robust to uncertainty. In many senses, models became boundary objects that encouraged critical thinking and questioning of assumptions across diverse stakeholders. And, for some nexus projects, confronting uncertainty in explicit and transparent ways build capacity for policy flexibility and adaptiveness. We conclude with a discussion of when and how these benefits can be fully realized through the strategic use of appropriate approaches to characterizing and navigating nexus uncertainty.
Introduction
In the context of global environmental change and efforts to achieve the United Nations Sustainable Development Goals, the water-energy-food (WEF) nexus framework is increasingly promoted as a means to integrate across systems and solution, identify and address risks, and ultimately contribute to sustainability (Bazilian et al., 2011; Hoff, 2011; Hussey and Pittock, 2012; Boas et al., 2016; Benson et al., 2017; Weitz et al., 2017a; Simpson and Jewitt, 2019). Nexus research explicitly connects human and natural systems, in order to provide a more complete picture about the causes and consequences of change. Realizing the potential of the nexus framework requires making decisions with the future in mind. But future nexus interactions are uncertain, and both research and practice must effectively account for a range of uncertainties.
One of the core assumptions articulated in nexus literature is that improved integration across water, energy, and food will contribute to sustainability (Bazilian et al., 2011; Hoff, 2011; Weitz et al., 2017a). However, while the concept of “the nexus” helps identify cross-cutting research questions, truly integrated analytical approaches that can be readily translated into coherent cross-sectoral and scale policy have been lacking (for recent critiques of the nexus, see Bazilian et al., 2011; Allan et al., 2015; Nature, 2016; Endo et al., 2017; Weitz et al., 2017b). While nexus research is often framed by social-ecological systems questions, and therefore requiring interdisciplinary approaches, much of this research applies engineering approaches to specific technical problems, investing in additional data sets and modeling techniques relevant to those problems (Dai et al., 2018). Oftentimes, the narrow focus on technical problems and engineering solutions are inadequate for policy makers who need to assess risks, trade-offs, and synergies across a broader suite of nexus interactions (Benson et al., 2017) and address uncertainty about how systems will change in the future (Peronne and Hornberger, 2014).
While uncertainty may not always be at the forefront in nexus publications, nexus work is fundamentally concerned with future uncertainty, through its engagement with complex systems, non-linearity, and interlinkages, as well as its concern with climate change and the trajectories of human communities and the natural resources they depend on. There is inherent uncertainty in how we might maintain ecological integrity and water, energy and food securities given the complex interplay of global earth systems and localized drivers of change. In the context of unprecedented global environmental change, scholars, policy makers, and practitioners increasingly call for greater attention to uncertainty in social-ecological systems (Gallopin, 2006; Binder et al., 2013; D'Odorico et al., 2018). There is growing attention to the ways in which systems exhibit non-linear behavior, and the limits to which past conditions can inform our understanding of the future. Over the past decade, scholars have increasingly problematize the idea of “stationarity,” or the notion that natural systems function within a known envelope of variability (Milly et al., 2008; Gober, 2014; Poff et al., 2016). Problems that reflect cross-system dynamics are sometimes conceptualized as “wicked,” where the nature of the problem is novel, not fully understood, and solutions are neither obvious nor agreed upon (Head, 2018). At the nexus, the scale and complexity of wicked problems make management and planning difficult, as decision-makers cannot observe all the ways in which one sector impacts another and what ripple effects this causes across different populations, scales, geographies, and time periods.
The following three challenges need to be addressed for the nexus framework to effectively characterize and address the uncertainties inherent in real-world WEF problems: (1) Resolving the technical challenge of analyses across sectors (e.g., water, energy, food) in a way that is robust, transparent, and credible to diverse stakeholders (Shannak et al., 2018); (2) Recognizing the governance challenge in building the legitimacy and salience of these various analytic tools so that they enable inclusive decision making that acknowledges contested views and values (Weitz et al., 2017b); and (3) Recognizing and addressing deep uncertainty about the future to identify robust policy choices likely to perform as intended over multiple possible futures (Herman et al., 2014).
Although the nexus framework inherently engages with future uncertainty, we argue that nexus work would benefit from more deliberate and in-depth attention to how various analytic tools, from quantitative modeling to qualitative futures thinking, fit into the nexus “toolbox” and nexus policy-making. This paper builds upon recent work calling for a pragmatic and integrated approach to addressing uncertainty in complex systems research and adaptive management (Chaffin and Gunderson, 2016; Memarzadeh and Boettiger, 2018). We first review and synthesize the ways that uncertainty is conceptualized in different analytic tools commonly used in nexus projects. We then present an analysis of applied nexus projects and how they integrate future uncertainty into analyses and decision-making. We provide an in-depth review of the LIVES (Linked Indicators for Vital Ecosystem Services) Cambodia project. This project conducted both qualitative and quantitative nexus modeling through a participatory process that integrated stakeholder knowledge of situated uncertainties into system dynamics model building and scenario development in support of sustainable development planning in the Mekong river basin.
Based on analysis of these nexus projects, this paper examines the barriers and opportunities for integrating quantitative modeling and qualitative futures thinking, and what that means for nexus governance and policy-making.
Literature Review
With nexus efforts developing at pace, one of the frontiers for nexus research is assessing the value of the nexus to support policy and practice (Ringler et al., 2013; Howells and Rogner, 2014; Gain et al., 2015; Boas et al., 2016; de Strasser et al., 2016; Gallagher et al., 2016; Grafton et al., 2016; Benson et al., 2017; Hagemann and Kirschke, 2017; Pahl-Wostl, 2017; Scott, 2017; Weitz et al., 2017b). Recent reviews suggest that the majority of nexus research aims to understand or quantify nexus interactions as opposed to addressing the challenges of policy and governance (Endo et al., 2017; Albrecht et al., 2018; Dai et al., 2018; Shannak et al., 2018). Nexus research is often justified with neo-Malthusian statements and statistics about growing populations, imminent water shortages, and the need to increase energy and food production. For example, Endo et al. (2017:21) commence by stating that “demands for water, energy and food are estimated to increase by 40, 50, and 35%, respectively by 2030.” Similarly, in characterizing South Asia, Rasul (2016:15) states that “land, water and vital ecosystem resources are dwindling, but the population is growing.” Such precautions set the imperative for analyses that optimize resource systems and increase efficiency across sectors, but the underlying assumption is that improved knowledge of nexus interactions will improve policy-making.
Most nexus projects recognize the need to address future uncertainty in both analyses and decision-making. For example, some nexus projects discuss the ways in which climate change might alter model results (e.g., Khan et al., 2017; Warmink et al., 2017) or how uncertainty about the accuracy and precision of particular data leads to instability in parameterization (e.g., Herman et al., 2014; Al-Ansari et al., 2015). However, very few nexus projects have well-developed, transparent processes for dealing with uncertainty, and assumptions about uncertainty vary greatly between quantitative modeling and qualitative futures thinking (Zinn, 2016). The diversity of definitions of, and assumptions about, uncertainty in nexus research and practice reflect more general characterizations of uncertainty across different disciplinary and epistemological traditions. An oft quoted typology comes from Donald Rumsfeld: known knowns (what we know we know), known unknowns (what we know we don't know), and unknown unknowns (what we don't even know we don't know). Perhaps the most important distinction within the literature is between risk, the probability of a particular outcome from a known distribution of outcomes (Beck, 1996; Polasky et al., 2011), and true uncertainty, which is fundamentally unquantifiable (Stirling, 2010; Pielke, 2012). First distinguished by economist Frank Knight, the latter is often referred to as Knightian uncertainty (e.g., Stirling, 2010), deep uncertainty (e.g., Maier et al., 2016), or ignorance (e.g., Rayner, 2012). In contrast, risk is often characterized as a relatively known unknown, especially in quantitative analyses. Statistical techniques are increasingly able to include unknown error terms in models, and to test the impacts of those errors (known unknowns) on the outputs of complex models [examples of these techniques include sensitivity analyses and mixed modeling with random effects (Bolker et al., 2009; Albrecht et al., 2018)]. In other words, the likelihood (risk) of, and difference in, outcomes associated with any particular future value of a given variable can be explored mathematically. However, quantification of risk cannot capture deep uncertainty, since by definition the likelihood, magnitude, and direction of those uncertainties is not fully or discretely known in the current moment. Thus, qualitative futures thinking, in particular scenario processes, are seen as a promising method that enables the integration of deep uncertainty into analyses and policy-making.
Uncertainty in Quantitative Modeling at Nexus
Currently, most nexus research utilizes quantitative modeling techniques to build knowledge of the dynamics and mechanisms within water-energy-food systems, to identify potential inflection points arising from interconnections and dependencies, and to project the possible outcomes of specific interactions and decisions (Endo et al., 2017; Kaddoura and El Khatib, 2017; Albrecht et al., 2018; Dai et al., 2018; Shannak et al., 2018). For example, coupled systems approaches highlight uncertainty and change, and focus on leveraging feedback processes to achieve improvement or difference across multiple components of systems (Carr et al., 2013; Antle, 2015; Fader et al., 2016). Gallopín et al. (2001:222) explain the orientating assumption of coupled systems analyses: “fundamental uncertainty is introduced both by our limited understanding of human ecological processes, by the intrinsic indeterminism of complex dynamic systems, and by myriad human goals.” One key feature of the coupled systems approach is the need to incorporate spatial and temporal heterogeneity, but empirical analysis is often limited by data availability as well as by data quality. For example, until recently, the costs associated with gathering longitudinal data on natural systems precluded wide-scale coverage, and thus the historical record does not extend back very far at high temporal resolutions and broad spatial scales (Liu et al., 2007). Analyses therefore often use simulation approaches to model heterogeneity and probability of change in populations and systems, approaches that derive from both ecology (Holling, 2004) and theoretical economics (Antle et al., 2014).
Similarly, risk assessments characterizing relationships and potential feedbacks across system components often use simulations and statistical models that predict the likelihood of a specific risk or hazard and its impacts in a given place or on a given system (Healy et al., 2015; Roberts and Barton, 2015). Measuring risk often relies on financial valuation of the potential and actual negative impacts of natural and social phenomena to help make decisions about costs, benefits, and trade-offs associated with changes in a system (Daily et al., 2009; Poppy et al., 2014; Devineni et al., 2015). Another common quantitative approach in nexus research, life-cycle analysis (LCA) and associated flows research, incorporates uncertainty into macro or aggregate analyses of balance sheets and footprints (Ramaswami and Chavez, 2013; Blanchard and Fabrycky, 2014; Tilman and Clark, 2014). Heijungs and Huijbregts (2004) identify the statistical approaches used in LCA to address data gaps and data quality issues, which most commonly use frequentist statistics to determine confidence intervals and sensitivity analyses.
Advancements in various types of simulation and systems dynamics models have enabled uncertainty to be characterized in increasingly sophisticated ways. For example, agent-based modeling and Monte Carlo simulations can characterize impacts (or effects) of system dynamics even when the adequacy or accuracy of effect measurements are unknown. These techniques are able to communicate effect uncertainty not only as a single probability of occurrence, but also as a range of likelihoods (the potential risk) that an effect will occur. However, these models are more limited in their ability to integrate uncertainties that have not been measured with much precision or consistency, are inherently difficult to quantify, or are simply unknown. For example, if there are elements of the system that are qualitative in nature, such as future political will or technological innovation, attempts to quantify them in models may be meaningless. Further, if particular drivers, relationships, or outcomes are unknown, the very structure of the model produces uncertainty that cannot be dealt with mathematically. In short, contemporary and cutting-edge quantitative modeling approaches often used in nexus analyses are increasingly sophisticated at incorporating known unknowns, but by definition cannot capture parameters about which there is deep uncertainty.
Scholars and practitioners are increasingly calling for processes that engage stakeholders in thinking about the assumptions, strengths, and limitations of models and how conceptual and quantitative models fit into nexus governance and policy-making (see for example, Kumazawa et al., 2017; Pahl-Wostl, 2017; Bieber et al., 2018).
Uncertainty and Futures Thinking at the WEF Nexus
Futures thinking offers a distinctively different way of addressing uncertainty, as compared with the kinds of quantitative analyses typically used in nexus research. Futures thinking draws on a range of approaches that explicitly engage with deep uncertainty and attempt to integrate uncertainty into policy-making in ways that improve future outcomes. These approaches focus on current system dynamics and the multiple possible futures that could emerge from these. In preparing for multiple futures, futures thinking requires decision-makers to carefully consider the driving forces, key elements, assumptions, and even worldviews driving the system of interest, essentially, all the elements which could change in the future. This facilitates deep reflection on the current system structure, including transitions, thresholds, and tipping points, and the flexibility and capacity needed to deal with and manage (rather than mitigate and minimize) uncertainty. There is a growing call for adoption of future-oriented approaches to complex problems, such as those at the water-energy-food nexus (e.g., Kelly et al., 2004; Wyborn et al., 2016).
Futures thinking integrates a range of quantitative and qualitative approaches, including data mining (examining large data sets to identify trends), Delphi method (the questioning of expert panels), backcasting (where one future is envisioned and then traced backwards to the present (Boulding and Boulding, 1995), and visioning (imagining the ideal future). However, scenario planning is often regarded as the cornerstone of futures thinking due to its explicit focus on a range of plausible futures (Kelly et al., 2004). In scenario planning, a range of possible future situations are identified, highlighting the interactions between forces and elements in a system (Amer et al., 2013). In general, requirements for scenarios are that they are internally coherent, plausible, and fundamentally distinct from one another (Kelly et al., 2004). Typically the number of scenarios ranges from 2 to 6, and they can be normative (identifying a desired future) or descriptive (Bezold, 2009). A common approach is to consider archetypal scenarios, for example continued growth, collapse, steady state, and transformation (Dator, 2009).
A key theme of futures thinking is that the future is not deterministic; it takes as a central tenet that there is no one future that can be predicted, hence the focus on multiple futures (Kuosa, 2010). The goal of futures thinking is not to forecast the future, but to foster flexible and innovative thinking, or “stretch the strategist's imagination” (Bezold, 2009:86) by considering multiple possibilities. In this sense, it is more about stimulating a certain type of thinking and capacity than it is seeking concrete answers about what the future will look like. Thus, a key tenet of futures thinking is an engagement with and attention to uncertainty, including building mechanisms and capacities for adapting to change in the face of true surprises. For example, while scenario planning may integrate quantitative information, futures thinking does not ultimately quantify uncertainty or treated it as a risk or probability (Raskin et al., 2014). Instead, scenarios examine a range of plausible futures based on current science and on-the-ground knowledge, and then use those scenarios to develop actions that are robust to uncertainty. As Inayatullah (2008:6) says, “alternative futures thinking reminds us that while we cannot predict a particular future accurately, by focusing on a range of alternatives, we can better prepare for uncertainty, indeed to some extent, embrace uncertainty.”
A key strength of futures thinking is that it brings uncertainty to the forefront. In futures thinking approaches, uncertainty is not minimized or ignored, or necessarily quantified, but rather used to foster new types of decision making, as well as new criteria for evaluating decisions. For example, a decision may be judged based on how reversible, how flexible, how adaptive, how robust it is, rather than how optimal it is based on limited existing knowledge (Stirling, 2010). At the same time, purely qualitative approaches do not realize some of the key benefits of quantitative modeling. Models and modern computing power can perform calculations about system linkages and dynamics that human brains are not capable of. Furthermore, the probabilistic approach to uncertainty taken by quantitative models can provide some estimate of the likelihood of specific future scenarios, which can help to focus discussion and possible investments of scarce resources. In some cases, models results are surprising and point to future trends or risks that would not otherwise be anticipated.
Integrating Quantitative Modeling and Futures Thinking to Support Nexus Governance
If, as Stirling (Stirling (2015:5) argues, the nexus must “go beyond narrow risk-based methods of ‘sound scientific' ‘evidence based policy' to more fully address uncertainty, ambiguity, and ignorance,” then nexus research and practice must expand the frameworks and methods on which it draws. Stirling's (Stirling (2015) comparison of nexus studies analyzes how, within each study, the level of uncertainty was described as minimal, but comparing across studies, supposedly certain results differ dramatically. One way to address the limitations and partiality of most nexus analyses is to integrate the assumptions and approaches to dealing with uncertainty that are inherent in any single analytical approach, quantitative or qualitative. In Table 1 below, we present a typology summarizing the main sources of uncertainty identified in the nexus literature, as well as the analytical techniques typically used to address these uncertainties.
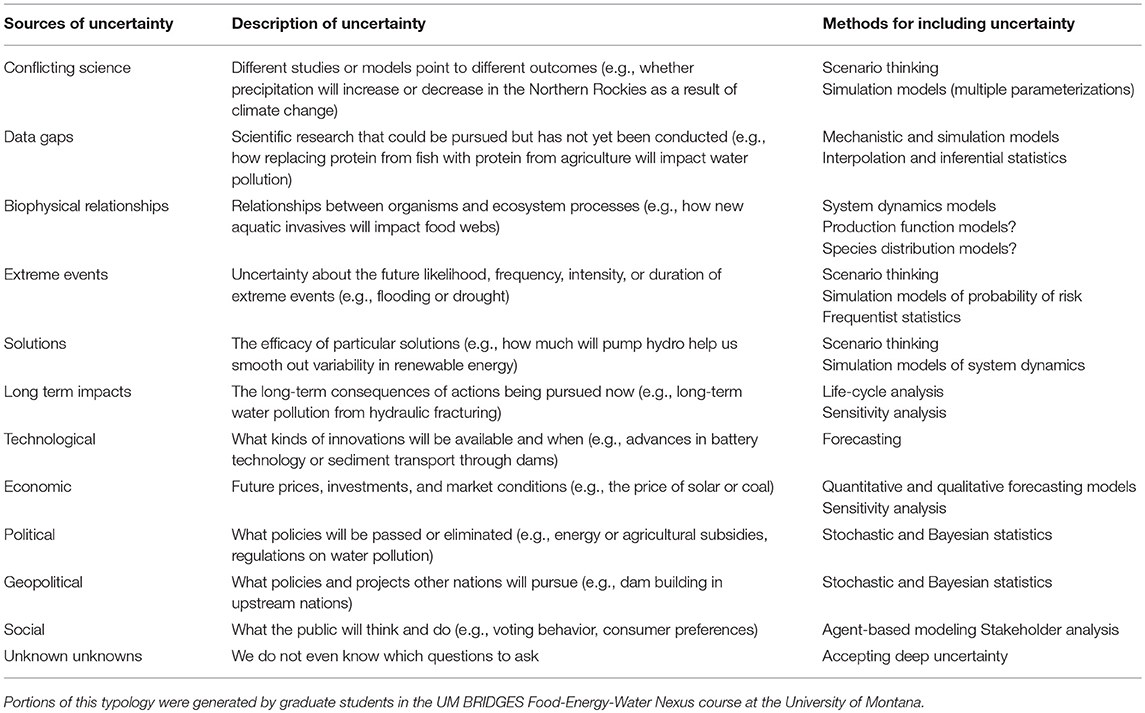
Table 1. A typology of sources of uncertainties and methods to include uncertainty in water-energy-food nexus research and application.
There are three reasons why we argue that integrating multiple analytic techniques, such as quantitative modeling and qualitative futures thinking, might produce results that are more policy-relevant as compared with using any single approach.
Firstly, mixed methods approaches are needed for analysis of complex and uncertain social-ecological systems because these systems are subject to constant, non-linear change. Interestingly, the two approaches described above, quantitative modeling and qualitative futures thinking, are rarely used together in nexus analyses. The divide between quantitative and qualitative approaches to uncertainty is problematic because it limits our ability to draw on the different strengths and potential synergies of these different methods, and ultimately contribute to the science-policy integration required for effective WEF governance [as identified by Doll and Romero-Lankao (2016); Weitz et al. (2017b)]. The role that uncertainty plays in different epistemological and disciplinary approaches has long been the subject of discussion by both philosophers of science and applied researchers (Samuelson, 1963; Schwandt, 1989). Contemporary theory and research focused on addressing complex sustainability challenges has begun to address head-on the underpinnings and manifestations of different conceptualizations of risk, uncertainty, and future possibility (e.g., Zinn, 2016; Warmink et al., 2017). An explicit engagement with futures thinking and with deep uncertainty, which requires acknowledging the unknowns beyond those that can be dealt with quantitatively in a model, could begin to address concerns raised by some quantitative researchers who worry that discussing uncertainty will undermine the perceived legitimacy of complex systems modeling (Doll and Romero-Lankao, 2016). Identifying the types of uncertainty that can be dealt with in robust ways within quantitative modeling provides both clarity about the strengths and clear delineation of the limitations of such modeling (Uusitalo et al., 2015).
Second, science-policy activities that do not recognize or address deep uncertainty work against flexible and robust decision making and introduce new risks. Flexibility and adaptability are believed to enhance resilience in the face of change (Armitage et al., 2009). Despite the distinction between uncertainty and risk made by many scholars, approaches to uncertainty often blur the line between these two concepts. Commonly, attempts to incorporate uncertainty involve reducing it to a probability through quantitative methods like Bayesian calculus (Maier et al., 2016). As Maier et al. (2016) explain, approaches to uncertainty often involve considerations of model inputs, parameters, and structure, all according to probability distributions: essentially, they engage only with the known unknowns. However, although the quantitative modeling frameworks described above have been primarily operationalized using techniques with limited ability to capture deep uncertainty, they can be integrated with qualitative approaches that account for processes that cannot be quantified and unknown unknowns. For example, coupled systems analyses has engaged with work on adaptive capacity (Gallopin, 2006; Carpenter and Brock, 2008; Chaffin and Gunderson, 2016), as well as institutional analyses of collective decision-making (Ostrom, 2009; Koontz et al., 2015), both of which emphasize mechanisms to address future uncertainty.
Thirdly, more fully embracing uncertainty can lead to more democratic and transparent policy processes. The tendency to quantify, reduce, or ignore uncertainty promotes expert-driven, top-down, and technocratic solutions (Stirling, 2010) because of the assumption that uncertainty can be reduced sufficiently to justify a one-size-fits-all policy solution. At the same time, scientific uncertainty can be politicized in ways that stymie policy-making, as is the case in climate policy, which can increase reluctance to explicitly acknowledge uncertainties. Thus, the use of science in policy-making is often influenced by historical, biophysical, social and political uncertainties—and attitudes toward those uncertainties—more so than by any objective contribution that science may make to determine a rational choice (Jamieson, 1996; Cash et al., 2003; Posner et al., 2016). By keeping uncertainty transparent, political processes remain just that: deliberative, based on human judgments, and subject to democratic processes, rather than dictated by unproblematic “scientific facts” that ignore and conceal uncertainties.
To examine these assumptions in more depth, we explored the following research questions:
1. How is uncertainty navigated in applied nexus projects that work across research and policy-making?
2. How have quantitative modeling and qualitative futures thinking been integrated?
3. What are some benefits and drawbacks to integrating quantitative modeling and qualitative futures thinking, specifically for a policy-making context?
Methods
We focused this research on applied nexus projects with an on-the-ground component or proposed policy intervention (and thus did not include projects that were purely research-oriented). This choice enabled us to explore how uncertainty and futures thinking are not just considered in nexus research, but how they are implemented and enacted (or not) in nexus policy-making.
To identify applied nexus projects, we conducted a Web of Science search for “water-energy-food nexus” and obtained ~500 results. We then examined each project to identify applied projects that clearly linked research to management or policy interventions. Not surprisingly, most nexus projects research, theorize, and critique the nexus. We also utilized online searches to identify additional projects that were not yet in the publication stage. We identified 12 applied nexus projects and collated basic information available from project websites in a database. We contacted all 12 projects and conducted in-depth, semi-structured interviews with project leads from nine projects in October 2017. One project consisted of multiple sub-projects, so two individuals from the organization were interviewed, resulting in 10 total interviews. We also reviewed relevant project documents obtained from interviewees.
An interview guide was utilized to ensure comparability across the interviews. The guide contained specific probes to obtain additional detail related to how uncertainty was conceptualized and operationalized. Interviewees were asked about the overall approach of the project, strengths and weaknesses of their approach, how the project dealt with uncertainty in current conditions, how it dealt with future uncertainty, and then how these concepts were communicated to stakeholders or participants in decision making contexts (see Appendix 1 for interview questions). Interviews were recorded and conducted with approval from the University of Montana Institutional Review Board. Interviews were then analyzed through an iterative constant comparative method to learn how different projects conceptualized and integrated uncertainty and futures thinking into their work. As part of this process, researchers compared across projects and engaged with relevant literature throughout the analysis to build a dialogue between theory and data, and to examine patterns across the dataset. To protect interviewee confidentiality, project names were removed and replaced with pseudonyms (P1–P9). All participants were then provided with the opportunity to review the results, make corrections, and identify their project by name if they desired. Thus, some projects are identified by name below while others remain anonymous. To provide a sense of the scope, scale, and nexus issues, a table of reviewed projects has been included (see Appendix 2).
We complement the nine projects examined through interviews and document analysis with an in-depth case study of LIVES Cambodia. LIVES Cambodia utilized participatory systems dynamics model building and scenario analysis to develop a nexus analysis of a transboundary landscape on the Mekong River. This case study contributes rich detail on beliefs and attitudes toward uncertainty within a project that blends quantitative modeling and qualitative futures thinking.
Similar to the nine projects describe above, the case study is examined from the perspective of the project lead (who is also an author on this paper), who served in the dual role of colleague-researcher on the project (Sandiford, 2015). The project lead draws on 15 interviews conducted in December 2017 with project partners using the Most Significant Change method (Dart and Davies, 2003). Interviewees were asked to reflect on critical outcomes—for themselves as individuals, for their organizations, and for the participants—and the barriers and enabling conditions related to achieving these outcomes. Using these interviews, project evaluations, and participant observation, the project lead reflects below on how local project partners and participants (1) changed their thinking on sources of uncertainty and how to address these in decision-making processes, and (2) the benefits and challenges of using both quantitative modeling and qualitative futures.
Results
Below we examine the results from our research on applied nexus projects and our analysis of the LIVES Cambodia case study. We describe how the nine applied nexus projects envision and operationalize uncertainty, synthesizing findings from in-depth interviews, as well as project documents and peer-reviewed articles describing the projects. We then explore the LIVES Cambodia case study, delving into the opportunities and challenges that this project faced relative to uncertainty. In both sections, stakeholder engagement plays a critical role in navigating uncertainty.
Overview of Applied Nexus Projects
The nine projects we initially investigated are extremely heterogeneous in scope, scale, and location. Some well-established, large scale research institutes like the Stockholm Environment Institute (SEI) and P1 carry out many projects at the nexus, from transnational and national to basin and sub-basin scales. Many projects were framed in terms of a basin or region, but carried out through stakeholder workshops on smaller scales. For example, the IUCN's Wise-Up to Climate project (henceforth Wise-Up) works in the Tana basin in Kenya and the Volta basin in Ghana/Burkina, but often focuses on sites at the community level. Other younger or more exploratory projects, for example P8, P4, and the Kitchen Nexus, work only on the regional scale or only in one location.
The majority of projects dealt with water, energy, and food as the primary nexus elements of concern, although many privilege one element over others or take one element as a starting point to see how the other elements will be impacted. For example, P8 works on redistributed manufacturing of food- they investigated how re-localizing production of bread and tomato paste would impact water and energy consumption in food systems.
Some initiatives were framed explicitly in terms of transdisciplinary research (Ring of Fire, Wise-Up, Northern Ireland Nexus), or citizen science (P4), and with only one exception (P2), all had participatory elements wherein stakeholders helped identify research questions to varying extents. The initiatives also had variable relationships to decision-making contexts. For example, in the projects by SEI and P1, researchers were specifically contracted to help policy-makers develop national-level plans, and thus were well-positioned to have an impact on specific decisions. Other projects were at an earlier stage of the research process, trying to create a basis for future engagement with decision-makers (Northern Ireland Nexus, Kitchen Nexus, and P8).
The initiatives also displayed a range of attitudes and approaches toward modeling. On one end, some projects had no modeling component (Kitchen Nexus, Northern Ireland Nexus, P4), and some project leads expressed doubts about the utility of combining quantitative modeling with narrative scenarios. On the other end of the spectrum, P2 was based largely around innovative modeling techniques and integration of different models in novel ways to support decision making. However, the majority of initiatives fell somewhere in the middle, using a quantitative model (or models) as part of a larger process of engagement. In general, project leads described models as tools, and not necessarily the main focus of the project. Exemplifying a typical approach, one project lead explained that, “the model becomes a boundary object- an object that people can discuss in more or less neutral terms in order to have discussions about hard trade-offs, but it's not the thing.” In general, project leads described the role of models in decision making in nuanced ways. As this person stated: “We know that extra information may not necessarily change decision making. It's not like decision-makers around the world have just been waiting for that one amazing model that's going to blow everyone's mind!” Throughout interviews, project leads described models as tools for creating dialogue, raising awareness, and bringing current challenges to the fore. For example, the project lead from SEI explained that, “They know that water scarcity will be an issue in the future, but the model shows that one million people will be without water under X development plan.” In summary, interviewees argued that models can make general areas of concern more concrete and tangible.
How Applied Nexus Projects Integrated Uncertainty
These projects dealt with uncertainty in a variety of ways. For the projects using quantitative models, project leads spoke in detail about the different kinds of uncertainties in the model. One recurring challenge was finding sufficient data. For example, for projects working in the Mekong, Eastern Africa, and the Nile basin obtaining data was a continual struggle. Project leads repeatedly referred to the challenges of working in a “data scarce environment.” For some, lack of data seemed to be the only type of uncertainty. However, others suggested that lack of data was just one of the uncertainties at play. In other words, some projects engage primarily with the known unknowns (data gaps, etc.), whereas others described multiple sources of uncertainty, including unknown unknowns. For example, a P1 project lead explained that he had worked with Bayesian analysis, Monte Carlo simulations, and other methods for dealing with uncertainty probabilistically, but that these methods were insufficient. As he put it:
One of the biggest mistakes people make, in every type of modelling too, is to assume that the past will continue under the same conditions. This is what you can call structural uncertainty…the relationship between different drivers is changing, their weight is changing, or there will be new drivers. So that's something you have to open up in people's brains.
P1 uses various types of quantitative models which are incorporated into “visioning” processes carried out with stakeholders. In the P1 approach, stakeholders develop detailed visions, or pictures of an ideal future. P1 researchers then sit in on stakeholder discussions, and listen to causal statements- assumptions about what causes what that may not be directly related to the vision, yet underpin it. The model is then used to test these causal assumptions, or “rattle the mental models,” as project lead explained. For example, if researchers hear stakeholders make statements like, “irrigation schemes will result in less poverty,” the model will then be used to test this assumption. Once these causal assumptions have been tested, they are presented back to stakeholders who then alter their development plans to achieve their shared vision. The P1 project lead explained that, before presenting the model, researchers worked to set the stage for stakeholders that “what you are about to see is not the truth.” In this project, the model is used as a tool to help stakeholders reflect on the assumptions that underpin their strategies. The project lead said that it was often challenging for the researchers who produced the model to be this explicit about the uncertainty.
The most common approach to dealing with uncertainty was through the use of scenarios. For some projects, the scenario approach was adopted specifically to highlight uncertainties. For example, the Northern Ireland Nexus project lead explained, “I was deliberately trying to create a sense of uncertainty… to be subversive of anyone's sense of security.” Many projects work explicitly to help stakeholders think in terms of multiple futures. For example, the SEI project lead discussed how their approach deliberately disturbs the conventional notion that there is one impending future: “The national plans suggest a single future world of wonderfulness, but what we're trying to argue is that that may get derailed. Your wonderful agricultural world may derail your wonderful energy world.”
One surprising finding from the interviews was that some projects take distinct approaches to uncertainty in different work streams. In Ring of Fire, an interviewee explained that uncertainty is dealt with by creating scenarios; however the project takes two distinct approaches to creating them. One is quantitative, based on integrated modeling and deals with uncertainty through probabilities. In a separate work stream, they create qualitative scenarios with local stakeholders, which grapple with all sorts of uncertainties that are difficult to quantify, for example uncertainties related to changing population demographics and future legislation. While the two types of scenarios had not been integrated at the time of the interview, Ring of Fire planned to incorporate the quantitative indicators into the qualitative scenarios and then use these integrated scenarios to engage local government.
Similarly, P8 and Wise-Up have distinct biophysical/economic modeling and socio-political work streams with distinct methods and treatments of uncertainty. For example, the project lead of P8 who is a modeler was able to speak to the ways that the model deals with uncertainty, while acknowledging all of the types of uncertainty that are unquantifiable, and dealt with more by his colleagues in the other work streams. Interviewees from P8, Wise-Up, and Ring of Fire all expressed that integration between work streams was sometimes constrained by time and finances, as well as differing visions and epistemological assumptions between research teams.
Two of the projects that did not use models utilized different understandings of uncertainty. In P4 where citizen science is the focus, the project lead was able to explain how uncertainty is understood in the community where he works. Community members talk about variable rainfall as a symptom of political corruption and changes in the social order in the community: it is a reflection of the health of the world which is out of balance. For these community members, uncertainty is a symptom of what is wrong with society. In this project, uncertainty is addressed through ethnographic methods- examining how it is understood by local residents.
How Applied Nexus Projects Engaged Stakeholders in Thinking About Uncertainty
Since these projects dealt with uncertainty to varying extents (both explicitly and implicitly), insights on how to communicate these ideas to stakeholders or use them to facilitate decision making were also uneven. However, when asked how projects communicate about uncertainty to stakeholders, a resounding answer from almost all projects was that this was a two-way process. Nearly all project leads emphasized that they learned from participants as much as the opposite. For example, in Northern Ireland Nexus, stakeholders brought up uncertainties precipitated by Brexit that had not been previously considered. P8 project staff learned that exorbitant rent prices in their study site were a major uncertainty for future local food manufacturing. In both cases, the project had not considered these sources of uncertainty before engaging with stakeholders.
Along this line, many project leads emphasized that working with stakeholders was a sort of ground-truthing for data, particularly in data-scarce, highly uncertain contexts. As a P1 lead explained, “We ask, how valid do you think these findings are?” Similarly, the project lead from P4 emphasized that their participatory iterative approach helps “break down the assumption that you as an outside researcher make about both the way the landscape works, and also the aspirations the community has for it.” Project leads repeatedly emphasized that they learned from stakeholders with extensive experience in a given context or sector.
Conversations with project leads also revealed specific, practical tips for getting stakeholders to think about uncertainty and communicating the results of complex models. In getting people to engage with multiple possible futures, a consistent concept (expressed in many different ways) was anchoring the future to something concrete that the participants can relate to. According to project leaders, anchoring is used to help stakeholders imagine the future by connecting it to something that has already happened (a past event) or already exists (a current policy). This helps futures become more relatable and tangible for stakeholders. For example, in Northern Ireland Nexus, the project lead began the scenarios with actual events that had occurred in the past.
Similarly, SEI sometimes reminds participants of an unexpected event from the past:
If [participants] are getting stuck on assuming something will happen that is in fact uncertain- remind about what has happened in the past there or elsewhere. Wherever they're stuck, try to give them an anchor in their own experience that gets them out of that stuck place.
The project lead illustrated this process using the example of the fall of the Berlin wall, saying that the day before it happened, the world was certain it wouldn't. In other SEI nexus projects, they embed their scenarios within existing national plans. The concept is the same- begin with concrete ideas that are in the participants' realm of experience, and then gradually become more imaginative to illustrate multiple plausible futures through the scenarios.
One consistent recommendation was to be transparent about assumptions, uncertainties, and sources of data. However, accompanying this was an emphasis on being judicious and strategic with the amount of information shared with stakeholders. As one person explained,
You don't have to go into the detail. There are just some key things people need to know to feel comfortable with the model….they don't necessarily need to know how it works, but they really want to know what's gone into it, and the key assumptions of that data going into it.
As another project lead explained, “the modeler gets up there, with scatter plots and everything, and people's eyes glaze over. Even within the research team!” She accompanied this by emphasizing the importance of participation in workshops and having stakeholders “present back” to researchers.
Multiple project leads explained that representing uncertainty numerically or probabilistically was insufficient for most policy audiences, that those numbers would “lose their nuance in policy circles.” As one person explained, a probabilistic representation of uncertainty (i.e., risk) may be well-understood by technical audiences, but amongst less technical audiences, the high levels of uncertainty represented in one number may be lost in translation. Said differently, a single, definitive representation of uncertainty is more vulnerable to political pressure than multiple, qualitative forms of uncertainty. This is not to say that uncertainties should never be represented quantitatively, but rather that the way uncertainty is communicated should be carefully tailored to the particular audience.
Conversations on communicating about future uncertainty also surfaced challenges. The most consistent challenge in getting stakeholders to engage with multiple futures was the mismatch between scales. Often, politicians think in terms of 5–10 years, whereas nexus research may be concerned with larger timescales. A project lead from Ring of Fire illustrated this challenge with a typical example. The researchers involved in the project wanted to discuss projections for the year 2100, stakeholders wanted to focus on 2040, and it was such a conflict, they ended up doing both.
Another consistent challenge in getting stakeholders to discuss the future and uncertainty was working in contexts with immediate, pressing issues. For example, for P2, trying to engage policymakers in turbulent, war-torn countries in conversations about the future was a challenge while immediate political unrest was so prevalent. Similarly, in the P4 project, electricity and population growth present immediate challenges to decision-makers: having conversations about long term future is not always a priority.
Case Study of LIVES Cambodia
The case study location is the Mekong Flooded Forest Landscape which covers two provinces in northeastern Cambodia, Kratie and Stung Treng, and Champasak province in Lao PDR (see Figure 1). Two major mainstem hydropower projects are underway in the landscape. Hydropower is one of few alternatives to improve national energy security (RGC, 2016) but the resulting changes in the Mekong river's flow, flood regime, fish migration patterns, and biodiversity from these developments is a risk to rural livelihoods and food security (MRC, 2017). These risks are compounded by anticipated changes to monsoon seasons, as well as changes to overall precipitation and temperature, as a result of climate change (Loo et al., 2015). Equitable solutions have been elusive due to differences in local and national priorities (Siciliano et al., 2015) and complex cultural, political, and historical factors (Milne and Mahanty, 2015). Most development planning to date has been sector-specific.
LIVES Cambodia was a 3 years transdisciplinary project collaboratively designed by a project team that included individuals from Cambodia's Ministry of Environment's National Council on Sustainable Development; World Wide Fund for Nature; KnowlEdge, a consultancy firm for participatory system dynamics modeling; the Royal University of Agriculture Cambodia, the Royal University of Phnom Penh, the University of Bergen, and the University of Maryland. The project sought to demonstrate the value of integrated, cross-sectoral, participatory nexus analyses and to co-produce new knowledge (Cash et al., 2006; Berkes, 2009) to support sustainable development decision making in this landscape using participatory system dynamics modeling (Antunes et al., 2015). Participants, including provincial departments of national ministries, civil society representatives, and members of local farming and fishing communities, were involved in participatory model building exercises as well as facilitated dialogue on qualitative and computer simulation scenario outputs (as per Vennix et al., 1996; Lane, 2008; Antunes et al., 2015) (see Figure 2).
Navigating Uncertainty in a Data-Poor Environment
The LIVES Cambodia model was designed explicitly to produce descriptions of possible futures (scenarios) potentially stemming from the convergence of local water-energy-food conflict, global environmental change, and socioeconomic change in the Mekong Flooded Forest landscape. System dynamics modeling creates explanatory models of system structures and simulates the dynamic interplay between key variables to explore system behavior over time (Forrester, 1961). Systems dynamics models have been used extensively for planning under complexity and uncertainty and look promising for nexus assessments given their ability to integrate social, economic, and environmental dimensions into scenarios (Bassi and Gallagher, 2016). The process entails iterative problem identification, system conceptualization, computer model formulation and validation, and both qualitative and quantitative scenario analysis.
At the start of the process, team members and participants assumed the LIVES Cambodia model would fill current data gaps on mainstem hydropower development risks for national and local energy security, local fishery sustainability, local food security and agricultural livelihoods sustainability to predict future outcomes for policy solutions accurately. One civil society partner argued that reducing uncertainties through scientific evidence was “required for policy change.” As the group built the model, many project team members and participants started “to realize we don't have that data” and that “without the data, it's hard to make choices.” LIVES Cambodia aimed to missing long-term data on such variables as fisheries and land productivity, dolphin birth rates and death rates, local market prices for rice and fish in part through the participatory stakeholder process. Dialogue processes helped to deepen participants understanding of uncertainties and key data gaps. Key uncertainties revolved around the relationships between developments (e.g., hydropower) and impacts (e.g., the availability of fish resources), which future changes to anticipate (e.g., how climate change might impact the monsoon), and the efficacy of specific mitigation and adaptation actions. As one research partner reflected: “We don't know exactly yet what we will change…We don't know exactly what is the policy that will work.”
Initially, uncertainty was not explicitly discussed in the stakeholder workshops beyond acknowledging these data gaps and issues. The main objective communicated to participants was to identify potential future risks in Kratie and Stung Treng provinces that could be monitored through 10–12 key indicators. These indicators would inform policy actions and investments in formal commune and national development planning processes. Project team members were reluctant to raise the question of uncertainty directly with provincial and community stakeholders. Some team members worried that an open discussion of uncertainty would further complicate an already complex project and method that was new to stakeholders. Others were concerned that final results would be appear less credible to participants if uncertainty was made explicit, potentially affecting the policy impact of the project. Some team members also had concerns about model robustness. However, uncertainty implicitly played a key role in the project design through the focus on building and questioning causal assumptions with the intention of identifying unforeseen risks due to interplay between WEF nexus elements.
The basic premise, based on previous applications in similar contexts (Voinov and Brown Gaddis, 2008; Videira et al., 2010; Kopainsky et al., 2017), was that (1) participatory system dynamics modeling could identify major structural elements in the social-ecological system and how these elements might interact and evolve over time, despite local data scarcity (Sterman, 2000); and (2) this would be useful to decision making under uncertainty because of the contribution of “crowdsourcing” (Backstrand, 2003; Bott and Young, 2012) accomplished through participatory processes that encouraged critical thinking about system linkages (Leys and Vanclay, 2011; Bodin, 2017).
The project focused on scenarios rather than specific policies because, in this context of multiple and heterogeneous actors, scenarios provide an aggregated analysis of the different governance responses that first must be negotiated and agreed upon before specific policies could be defined and implemented. Further, training and capacity building was provided to the project team to be transparent about the meaning of the model outcomes, with a specific focus on how the model did not predict future outcomes but rather produced results that represented one possible future. As one of the project modelers reflected:
People believe if they invest a lot of money in a model, they believe that it should accurately predict the future…The [LIVES] project raised awareness about the how hard it is to predict the future. I get frustrated with tools that say that they can predict the future and help policy makers…Donors and policy people want research to give certainty to policy making but in reality, it is very hard to prove that a particular intervention is going to work…it seems like anybody who suggests their data and models can give absolute certainty is lying.
Thus, a key strategy to help participants engage with the model was understanding its strengths and limitations, and predictive abilities given that multiple futures were possible for the landscape.
Integrating Futures Thinking Into the Deliberative Process
A notable challenge was developing the scenarios in futures thinking procedures with provincial administration stakeholders. For some, the computer software was confusing. Others reported to local researchers that they felt they were not in a position to speculate about the future given “the decision [about hydropower infrastructure placement and design] is not made by them.”
This almost certainly reflects the politically sensitive nature of the forthcoming hydropower developments approved at central government level, rather than a lack of capacity to imagine the future. The project team implemented two strategies to overcome this barrier. A more intensive engagement with representatives from fishing and farming communities—both mixing these participants with provincial administrators and conducting separate sessions just for community-level actors. The latter enabled a more grounded, less overtly political discussion of future trends and potential responses as seen from the local-level perspective. Secondly, a more “hands on” group modeling protocol that allowed participants to engage in deeper ways with scenario development. Finally, the project team synthesized discussions across both the provincial and community sessions to develop draft scenarios which were then validated with stakeholders in final workshops. The final scenarios explored the ways that different development scenarios would impact food, water and livelihoods security. The four scenarios are summarized here:
1) Maintaining the status quo development pathway (no dams);
2) Introducing the two planned hydropower developments, modeling the impact of the Stung Treng dam and then subsequently adding the Sambor dam (in order of their likely completion and operation);
3) Implementing one adaptation strategy in local agricultural systems focused on intensification of rice production (based on Cambodia-specific data: Ly et al., 2012, 2016);
4) Implementing an environmental flows mitigation policy in the planned hydropower projects with the goal of maintaining flows on the mainstream of the Mekong river [as per specifications in Babel et al. (2012)].
A common reflection across project team members and participants points to one factor enabling this outcome. The participatory system dynamics modeling methodology used often gave voice and legitimacy to a range of interests, opening up space for deliberation and engendering a new sharing of information and perspectives. As one civil society team member claimed: “participants dared to say anything, their voice is included.” Local participants initially expressed that they did not have the education or credentials of national level government and university participants. But as they engaged in the process, they “challenged” the assumptions of the model “saying this variable shouldn't connect to that one.” As a result, some national government team members realized that they “lack the knowledge of the reality” of local communities and that local stakeholders “know more about the landscape, they know with their own eyes.” The participatory process enabled the group to recognize the value of local knowledge and integrate that knowledge into the model to fill data gaps regarding the relationships between key variables. As one participant commented about the model building process, “It's done with people. I think as a planner, this is really important.”
By the end of the 3 years, there were signs that the project team and participants were shifting away from a focus on filling data gaps and waiting for model results toward asking how the LIVES Cambodia process of participatory model building and futures thinking itself might contribute to a “better plan, better future.” Project participants with responsibilities for local planning and expressed an interest in utilizing the participatory model building and scenario tools in Commune Investment Planning, a formal part of developing the next National Strategic Development Plan (2019–2023). Further, some project team members came to recognize that uncertainty is not always a result of lack of information or data gaps. Because data is politicized in Cambodia, some participants expressed concerns that others were deliberately withholding information during the model building process to create uncertainty about the impacts of hydropower developments on local communities: “Data holders do not want to share data,” one civil society project partner claimed. For other team members, the interest that government partners had displayed throughout the process provided evidence that policy influence was possible even in the face of ongoing uncertainties. For example, one civil society team member who initially expressed frustration at not having “evidence for advocacy” on hydropower from the model, reflected later that uncertainty about future actions and solutions could potentially be tackled in through stakeholder deliberation, saying “If we introduce integrated planning based on the causal loop diagrams, all the people sit in the same room and discuss from one issue to another issue and get consensus for policy implementation. This way is very effective.”
Ultimately, the deliberative process that drove the model building and scenario development enabled stakeholder recommendations to be crafted in the face of uncertainty. Looking back, some project team members who had initially opposed addressing uncertainty explicitly reflected that one of the key insights from LIVES Cambodia was that planning for the future often requires thinking about complexity and uncertainty. One civil society partner compared SWOT (strengths, weaknesses, opportunities, and threats) analysis (commonly used in planning processes in the case study location) to the group systems model building (Causal Loop Diagrams), saying: “We have been trying to cope with limited local knowledge by using simple tools—but the CLD shows that even if it is complicated, it is better. People accepted that SWOT was simpler, but giving the wrong analysis. CLD is more complex but reflects the situation better.” In effect, though the original plan had been to minimize discussions of uncertainty in stakeholder workshops, the choice of the system dynamics modeling methodology may have introduced uncertainty by default. New capacities for dealing with uncertainties were developed through the engaging with the modeling process, specifically critical analysis of feedback loops, the possibility of non-linear change, and the potential for delayed effects in a system's structure and evolution. The participatory process created conditions whereby deep uncertainty could be explored by stakeholders. Discussions about cause-effect linkages and future scenarios led to more agreement on where and how to intervene—including clarity on who has the capacity to intervene. This process also pointed to a range of possible solutions or development pathways. As this participant pointed out: “That's the kind of planning you need. […] Not just filling in the template. It gives you flexibility. Though you know your goal is sustainable development, there are many ways there. We can identify diverse ways to get there.”
Summary of Results
The nexus case studies demonstrated a range of levels and a diversity of types of engagement with uncertainty and futures thinking. For some, uncertainty played a key role in the project and was intentionally brought into focus for stakeholders. In these projects, uncertainty was invoked to foster robust decision making, question causal assumptions, and surface unforeseen conflicts between nexus elements. In other projects, uncertainty was more incidental, implied in the research question or encountered as a challenge to the project as planned. LIVES Cambodia, a project in the latter category, demonstrates how projects mixing participatory research, quantitative modeling, and qualitative futures thinking will often be called to explicitly address uncertainty in some way. This kind of flexibility and reflection on development pathways builds the adaptive capacity needed in the face of deep uncertainty and potential surprises. We suggest that mixing participatory research, quantitative modeling, and qualitative futures thinking methods shows promise in developing such capacity.
Discussion
We set out to understand how nexus projects envision, operationalize, and navigate uncertainty, specifically in those cases where nexus research is linked to policy-making contexts. Through in-depth interviews with project leads from nine applied nexus projects and a detailed examination of LIVES Cambodia, we discovered complex, nuanced understandings of the types of uncertainties that are important, as well as detailed insights into the opportunities and challenges of engaging stakeholders in thinking about uncertainty.
Interestingly, interviews with project leads led to more nuanced discussions of uncertainty than are currently present in the nexus literature. This may be a result of the focused nature of academic publishing and the barriers to reflecting in-depth on the limitations of a particular analyses (especially the analysis that was employed for the publication). This finding may also be an artifact of our sampling process, which focused specifically on nexus projects that bridge science and policy. For example, while LIVES Cambodia did not set out to explicitly integrate uncertainty into their project, workshops with stakeholders raised critical questions about uncertainty that became an important part of the process. Thus, it is possible that moving nexus science into policy-making requires confronting uncertainty in different ways as compared with nexus research that is more detached from applied contexts.
That said, engaging stakeholders in explicit conversations about uncertainty is challenging. This is due to the technical nature of how uncertainty is dealt with and represented in modeling computations and the challenge of communicating about that process to a broad audience. But conversations about uncertainty are also challenging because of a fear that acknowledging uncertainty will undermine the credibility and utility of a particular model.
One way out of this conundrum is to utilize qualitative futures thinking processes, such as scenario planning, to engage stakeholders in more deliberative, explicit discussions of uncertainty (either alongside quantitative modeling or as a separate exercise). Futures thinking processes require participants to confront uncertainty through a focus on a specific range of futures, and as a result they question assumptions, foster flexibility, gain insight into the present, and craft robust solutions. Of the nexus projects we examined, those with a clear focus on multiple possible futures discussed various types of uncertainty more explicitly and in more detail, and emphasized uncertainties with their stakeholders.
However, models were also utilized in stakeholder processes to enable critical thinking about uncertainty, system dynamics, and proposed policies. As models moved into stakeholder processes, they often played the role of boundary objects (Midgley, 1992), fostering dialogue about the assumptions about the system that underlie computations, as well as on the strengths and weaknesses of specific computational techniques. As was demonstrated by LIVES Cambodia, engaging stakeholders in the model building process can also help improve the model to the extent that local knowledge can be used to address data gaps and critically evaluate model assumptions. Further, many projects were using models in participatory processes in which hypotheses could be tested, evaluated, and revised, through a transparent and collaborative process. This process required modelers to develop the skills to engage in critical reflection with the models that they built (Senge and Sterman, 1992). Project leads found that while these conversations were challenging, they led to deeper discussions, more holistic framings of problems, and an acceptance of the different types of uncertainties, beyond simple data gaps, that plague nexus research and governance.
This is the process of knowledge co-production, where experts and non-experts collaborate to create new knowledge and catalyze change (Cash et al., 2006; Berkes, 2009; Miller and Wyborn, 2018). The negotiation, mediation, and deliberation that comprise co-production can play an important role in achieving new governance outcomes for collective action problems characterized by deep uncertainty (Innes and Booher, 2010; Wyborn, 2015). Howarth and Monasterolo (2017) justify a co-production approach to nexus research precisely because of complexity, non-linearity, and uncertainty inherent in such analysis.
Critically, when situated within a participatory process, quantitative models present a “jumping off” point from which to initiate discussions about the future. Breaking out of a focus on present day politics and challenges is one of the difficulties that needs to be addressed when facilitating conversations about the future. In such circumstances, a quantitative model that stretches out to 2030–2050 or beyond can present participants with a future that “destabilizes” their connection to the present, and confronts them with medium to long term outcomes that can be traced back to choices made historically. The model then provides a tangible boundary object around which to have a conversation about human agency within a system, and the various pathways that are available. In these circumstances, simulation models can be used to test the outcomes of proposed pathways, or a set of assumptions about how a given set of actions might lead to particular changes. When the simulations expose a set of unanticipated consequences or behaviors, the model does not need to be “right” to be useful, as it shows the possibility for unforeseen impacts, thereby explicitly demonstrating the nature of the uncertainties present within nexus governance.
Creating actionable knowledge in this way is an iterative process that requires diverse points of view at all stages of research (Pohl, 2005, 2010; Lang et al., 2012). If the goal of nexus work is to link science and policy, then producing science that engages in meaningful ways with policy-making requires more than integrating across quantitative modeling and qualitative futures thinking. It requires thinking carefully about how science and policy come together when projects engage stakeholders in model building and in scenario development, and through both processes build capacity for making decisions in the context of uncertainty.
Recommendations
Based on the research described above, we provide the following recommendations for applied nexus projects:
Embrace transparency—Be sure that all assumptions, key uncertainties, and data sources in a model or any kind of analyses are communicated clearly to stakeholders.
Be participatory—Create a process for mutual learning that values different kinds of knowledge, including expert and local knowledge. Stakeholders can help validate research findings, critique and support assumptions made by researchers, and present new uncertainties that hadn't been considered.
Envision models as a tool- Encourage participants to value the model as a boundary object that facilitates discussion about future possibilities, based on available data and current assumptions. Models are powerful tools to spark discussion and raise areas of concern. Discussions about model uncertainty and limitations can be balanced with an emphasis on the critical role that models can play in facilitating discussions about the future.
Use scenarios to raise awareness of uncertainty—Craft scenarios so as to highlight areas of uncertainty that stakeholders may not have fully considered. Techniques like wind-tunneling (seeing how a given policy will perform under various scenarios) can help to fortify a given policy or management plan against multiple uncertainties. Scenarios also help participants consider how to build adaptive capacity in the context of deep uncertainty.
Represent uncertainties in ways that work for your audience—With technical audiences, probabilistic treatments of uncertainty may be sufficient to communicate uncertainty within a model. However, with many policy-makers and practitioners, a probability, or risk, may not be adequate. Simplified, numeric representations of uncertainty may be more vulnerable to political pressure and may neglect deeper uncertainty. Instead, help stakeholders understand the sources and nature of uncertainty, and encourage critical thinking about the role of uncertainty in decision-making.
Author Contributions
LY, EL, LG, KJ, and CW jointly contributed to manuscript development. LY, CW, LG, and EL designed the study of applied nexus projects. EL conducted interviews and analyzed interview data. LG conducted the case study analyses. KJ provided the examination of quantitative nexus analyses. CW and LY synthesized across project elements.
Funding
The research presented here is based on work supported by the Nomis Foundation, Luc Hoffmann Institute, the National Science Foundation (Grant No. 1633831), and the National Socio-Environmental Synthesis Center (under funding received from the National Science Foundation DBI-1639145).
Conflict of Interest Statement
The authors declare that the research was conducted in the absence of any commercial or financial relationships that could be construed as a potential conflict of interest.
Acknowledgments
We also wish to thank the project leads from applied nexus projects who participated in interviews as well as the team members and participants from the LIVES Cambodia project.
Supplementary Material
The Supplementary Material for this article can be found online at: https://www.frontiersin.org/articles/10.3389/fenvs.2019.00037/full#supplementary-material
References
Al-Ansari, T., Korre, A., Nie, Z., and Shah, N. (2015). Development of a life cycle assessment tool for the assessment of food production systems within the energy, water and food nexus. Sustain. Prod. Consum. 2, 52–66. doi: 10.1016/j.spc.2015.07.005
Albrecht, T. R., Crootof, A., and Scott, C. A. (2018). The water-energy-food nexus: a systematic review of methods for nexus assessment. Environ. Res. Lett. 13:043002. doi: 10.1088/1748-9326/aaa9c6
Allan, T., Keulertz, M., and Woertz, E. (2015). The water–food–energy nexus: an introduction to nexus concepts and some conceptual and operational problems. Int. J. Water Resour. D. 31, 301–311. doi: 10.1080/07900627.2015.1029118
Amer, M., Daim, T. U., and Jetter, A. (2013). A review of scenario planning. Futures. 46, 23–40. doi: 10.1016/j.futures.2012.10.003
Antle, J. M. (2015). “Climate vulnerability of farm households: new methods and evidence,” in Conference paper for Resources for the Future (Washington, DC).
Antle, J. M., Stoorvogel, J. J., and Valdivia, R. O. (2014). New parsimonious simulation methods and tools to assess future food and environmental security of farm populations. Philos. Trans. R. Soc. Brit. 369:20120280. doi: 10.1098/rstb.2012.0280
Antunes, M. P., Stave, K. A., Videira, N., and Santos, R. (2015). “Using participatory system dynamics in environmental and sustainability dialogues,” in Handbook of Research Methods and Applications in Environmental Studies, ed. M. Ruth (Cheltenham: Edward Elgar Publishing), 346–374.
Armitage, D. R., Plummer, R., Berkes, F., Arthur, R. I., Charles, A. T., Davidson-Hunt, I. J., et al. (2009). Adaptive co-management for social–ecological complexity. Front. Ecol. Environ. 7:89. doi: 10.1890/070089
Babel, M. S., Nguyen, Dinh. C, Reaz, M. A., and Umamahesh, N. V. (2012). Operation of a hydropower system considering environmental flow requirements: a case study in La Nga River Basin, Vietnam. J. Hydroenviron. Res. 6, 63–73. doi: 10.1016/j.jher.2011.05.006
Backstrand, K. (2003). Civic science for sustainability: reframing the role of experts, policy-makers and citizens in environmental governance. Glob. Environ. Polit. 3, 24–41. doi: 10.1162/152638003322757916
Bassi, A. M., and Gallagher, L. A. (2016). “Integrated economic and spatial planning for the food-energy-water nexus” in Economic Modeling, Analysis, and Policy for Sustainability Goswami, ed A. Mishra (Hershey, PA: IGI Global), 1–323.
Bazilian, M., Rogner, H., Howells, M., Hermann, S., Arent, D., Gielen, D., et al. (2011). Considering the energy, water and food nexus: towards an integrated modelling approach. Energy Policy. 39, 7896–7906. doi: 10.1016/j.enpol.2011.09.039
Beck, U. (1996). World risk society as cosmopolitan society? Ecological questions in a framework of manufactured uncertainties. Theory Cult. Soc. 13, 1–32. doi: 10.1177/0263276496013004001
Benson, D., Gain, A. K., Rouillard, J., and Giupponi, C. (2017). “Chapter 8: Governing for the nexus” in Water-Energy-Food Nexus: Principles and Practices, eds P. Abdul Salam, S. Shrestha, V. P. Pandey, and A. K. Anal (John Wiley & Sons, Inc.), 77–88. doi: 10.1002/9781119243175.ch8
Berkes, F. (2009). Evolution of co-management: role of knowledge generation, bridging organizations and social learning. J. Environ. Manage. 90, 1692–1702. doi: 10.1016/j.jenvman.2008.12.001
Bieber, N., Ker, J. H., Wang, X., Triantafyllidis, C., van Dam, K. H., Koppelaar, R. H. E. M., et al. (2018). Sustainable planning of the energy-water-food nexus using decision making tools. Energy Policy. 113, 584–607. doi: 10.1016/j.enpol.2017.11.037
Binder, C. R., Hinkel, J., Bots, P. W., and Pahl-Wostl, C. (2013). Comparison of frameworks for analyzing social-ecological systems. Ecol. Soc. 18:26. doi: 10.5751/ES-05551-180426
Boas, I., Biermann, F., and Kanie, N. (2016). Cross-sectoral strategies in global sustainability governance: towards a nexus approach. Int. Environ. Agreem. Polit. Law Econ. 16, 449–464. doi: 10.1007/s10784-016-9321-1
Bodin, Ö. (2017). Collaborative environmental governance: achieving collective action in social-ecological systems. Science. 357:eaan1114. doi: 10.1126/science.aan1114
Bolker, B. M., Brooks, M. E., Clark, C. J., Geange, S. W., Poulsen, J. R., Stevens, M. H., et al. (2009). Generalized linear mixed models: a practical guide for ecology and evolution. Trends Ecol. Evol. 24, 127–135. doi: 10.1016/j.tree.2008.10.008
Bott, M., and Young, G. (2012). The role of crowdsourcing for better governance in international development. Praxis. 27, 47–70.
Carpenter, S. R., and Brock, W. A. (2008). Adaptive capacity traps. Ecol. Soc. 13. Available online at: http://www.jstor.org/stable/26267995
Carr, J. A., D'Odorico, P., Laio, F., and Ridolfi, L. (2013). Recent history and geography of virtual water trade. PLoS ONE. 8:e0055825. doi: 10.1371/journal.pone.0055825
Cash, D. W., Adger, W., Berkes, F., Garden, P., Lebel, L., Olsson, P., et al. (2006). Scale and cross-scale dynamics: governance and information in a multilevel world. Ecol. Soc. 11:8. doi: 10.5751/ES-01759-110208
Cash, D. W., Clark, W. C., Alcock, F., Dickson, N. M., Eckley, N., Guston, D. H., et al. (2003). Knowledge systems for sustainable development. Proc. Natl. Acad. Sci. U.S.A. 100, 8086–8091. doi: 10.1073/pnas.1231332100
Chaffin, B. C., and Gunderson, L. H. (2016). Emergence, institutionalization and renewal: rhythms of adaptive governance in complex social-ecological systems. J. Environ. Manag. 165, 81–87. doi: 10.1016/j.jenvman.2015.09.003
Dai, J., Wu, S., Han, G., Weinberg, J., Xie, X., Wu, X., et al. (2018). Water-energy nexus: a review of methods and tools for macro-assessment. Appl. Energy. 210, 393–408. doi: 10.1016/j.apenergy.2017.08.243
Daily, G. C., Polasky, S., Goldstein, J., Kareiva, P. M., Mooney, H. A., Pehjchar, L., et al. (2009). Ecosystem services in decision making: time to deliver. Front. Ecol. Environ. 7:25. doi: 10.1890/080025
Dart, J., and Davies, R. (2003). A dialogical, story-based evaluation tool: the most significant change technique. Am. J. Eval. 24, 137–155. doi: 10.1177/109821400302400202
de Strasser, L., Lipponen, A., Howells, M., Stec, S., and Bréthaut, C. (2016). A methodology to assess the water energy food ecosystems nexus in transboundary river basins. Water. 8:59. doi: 10.3390/w8020059
Devineni, N., Lall, U., Etienne, E., Shi, D., and Xi, C. (2015). America's water risk: current demand and climate variability. Geophys. Res. Lett. 42, 2285–2293. doi: 10.1002/2015GL063487
D'Odorico, P., Davis, K. F., Rosa, L., Carr, J. A., Chiarelli, D., Dell'Angelo, J., et al. (2018). The global food-water-energy nexus. Rev. Geophys. 56, 456–531. doi: 10.1029/2017RG000591
Doll, P., and Romero-Lankao, P. (2016). How to embrace uncertainty in participatory climate change risk management: a roadmap. Earths Fut. 5, 18–36. doi: 10.1002/2016EF000411
Endo, A., Tsurita, I., Burnett, K., and Orencio, P. M. (2017). A review of the current state of research on the water, energy, and food nexus. J. Hydrol. Reg. Stud. 11, 20–30. doi: 10.1016/j.ejrh.2015.11.010
Fader, M., Rulli, M. C., Carr, J., Dell'Angelo, J., D'Odorico, P., Gephart, J. A., et al. (2016). Past and present biophysical redundancy of countries as a buffer to changes in food supply. Environ. Res. Lett. 11:055008. doi: 10.1088/1748-9326/11/5/055008
Gain, A. K., Giupponi, C., and Benson, D. (2015). The water–energy–food (WEF) security nexus: the policy perspective of Bangladesh. Water Int. 40, 895–910. doi: 10.1080/02508060.2015.1087616
Gallagher, L., Dalton, J., Brethaut, C., Allan, T., Bellfield, H., Crilly, D., et al. (2016). The critical role of risk in setting directions for water, food and energy policy and research. Curr. Opin. Env. Sust. 23, 12–16. doi: 10.1016/j.cosust.2016.10.002
Gallopin, G. C. (2006). Linkages between vulnerability, resilience, and adaptive capacity. Glob. Environ. Change 16, 293–303. doi: 10.1016/j.gloenvcha.2006.02.004
Gallopín, G. C., Funtowicz, S., O'connor, M., and Ravetz, J. (2001). Science for the twenty-first century: From social contract to the scientific core. Int. Soc. Sci. J. 53, 219–229. doi: 10.1111/1468-2451.00311
Gober, P. (2014). “Decision making under uncertainty: a new paradigm for water resource planning and management,” in Modern Water Resources Engineering, eds L. K. Wang, and C. T. Yang (New York, NY: Springer), 411–436.
Grafton, R. Q., McLindin, M., Hussey, K., Wyrwoll, P., Wichelns, D., Ringler, C., et al. (2016). Responding to global challenges in food, energy, environment and water: risks and options assessment for decision-making. Asia Pac. Policy Stud. 3, 275–299. doi: 10.1002/app5.128
Hagemann, N., and Kirschke, S. (2017). Key issues of interdisciplinary NEXUS governance analyses: lessons learned from research on integrated water resources management. Resources 6:9. doi: 10.3390/resources6010009
Head, B. W. (2018). Forty years of wicked problems literature: forging closer links to policy studies. Policy Soc. doi: 10.1080/14494035.2018.1488797. [Epub ahead of print].
Healy, R. W., Alley, W. M., Engle, M. A., McMahon, P. B., and Bales, J. D. (2015). The water-energy nexus: an earth science perspective. US Geol. Survey Circ. 1407:107. doi: 10.3133/cir1407
Heijungs, R., and Huijbregts, M. A. J. (2004). A review of approaches to treat uncertainty in LCA. Int. Congr. Environ. Model. Softw. 197. Available online at: https://scholarsarchive.byu.edu/iemssconference/2004/all/197
Herman, J. D., Zeff, H. B., Reed, P. M., and Characklis, G. W. (2014). Beyond optimality: multistakeholder robustness tradeoffs for regional water portfolio planning under deep uncertainty. Water Resour. Res. 50, 7692–7713. doi: 10.1002/2014WR015338
Hoff, H. (2011). “Understanding the nexus,” in Background Paper for the Bonn2011 Conference: The Water, Energy and Food Security Nexus (Stockholm: Stockholm Environment Institute).
Holling, C. S. (2004). From complex regions to complex worlds. Ecol. Soc. 9:11. doi: 10.5751/ES-00612-090111
Howarth, C., and Monasterolo, I. (2017). Opportunities for knowledge co-production across the energy-food-water nexus: making interdisciplinary approaches work for better climate decision making. Environ. Sci. Policy. 75, 103–110. doi: 10.1016/j.envsci.2017.05.019
Howells, M., and Rogner, H. H. (2014). Assessing integrated systems. Nat. Clim. Change. 4:246. doi: 10.1038/nclimate2180
Hussey, K., and Pittock, J. (2012). The energy–water nexus: managing the links between energy and water for a sustainable future. Ecol. Soc. 17:31. doi: 10.5751/ES-04641-170131
Inayatullah, S. (2008). Six pillars: futures thinking for transforming. Foresight. 10, 4–21. doi: 10.1108/14636680810855991
Innes, J., and Booher, D. E. (2010). Planning With Complexity: An Introduction to Collaborative Rationality for Public Policy. Oxford: Routledge.
Jamieson, D. (1996). Scientific uncertainty and the political process. Ann. Am. Acad. Polit. Soc. Sci. 545, 35–43. doi: 10.1177/0002716296545001004
Kaddoura, A., and El Khatib, A. (2017). Review of water-energy-food nexus tools to improve the nexus modelling approach for integrated policy making. Environ. Sci. Policy. 77, 114–121. doi: 10.1016/j.envsci.2017.07.007
Kelly, R., Sirr, L., and Ratcliffe, L. (2004). Futures thinking to achieve sustainable development at local levels in Ireland. Foresight. 6, 80–90. doi: 10.1108/14636680410537547
Khan, H., Yang, Y. C. E., Xie, H., and Ringler, C. (2017). A coupled modeling framework for sustainable watershed management in transboundary river basins. Hydrol. Earth Syst. Sci. 21, 6275–6288. doi: 10.5194/hess-21-6275-2017
Koontz, T. M., Gupta, D., Mudliar, P., and Ranjan, O. (2015). Adaptive institutions in social-ecological systems governance: a synthesis frameworks. Environ. Sci. Policy. 53(Part B), 139–151. doi: 10.1016/j.envsci.2015.01.003
Kopainsky, B. G., Hager, H., and Herrera, Nyanga, P. H. (2017). Transforming food systems at local levels: using participatory system dynamics in an interactive manner to refine small-scale farmers' mental models. Ecol. Model. 362, 101–110. doi: 10.1016/j.ecolmodel.2017.08.010
Kumazawa, T., Hara, K., Endo, A., and Taniguchi, M. (2017). Supporting collaboration in interdisciplinary research of water–energy–food nexus by means of ontology engineering. J. Hydrol. Reg. Stud. 11, 31–43. doi: 10.1016/j.ejrh.2015.11.021
Kuosa, T. (2010). Evolution of futures studies. Futures. 43, 327–336. doi: 10.1016/j.futures.2010.04.001
Lane, D. C. (2008). The emergence and use of diagramming in system dynamics: a critical account. Syst. Res. 25, 3–23. doi: 10.1002/sres.826
Lang, D. J., Wiek, A., Bergmann, M., Stauffacher, M., Martens, P., Moll, P., et al. (2012). Transdisciplinary research in sustainability science: practice, principles, and challenges. Sustain. Sci. 7, 25–43. doi: 10.1007/s11625-011-0149-x
Leys, A. J., and Vanclay, J. K. (2011). Social learning: a knowledge and capacity building approach for adaptive co-management of contested landscapes. Land Use Policy. 28, 574–584. doi: 10.1016/j.landusepol.2010.11.006
Liu, J., Dietz, T., Carpenter, S. R., Alberti, M., Folke, C., Moran, E., et al. (2007). Complexity of coupled human and natural systems. Science. 317, 1513–1516. doi: 10.1126/science.1144004
Loo, Y. Y., Billa, L., and Singh, A. (2015). Effect of climate change on seasonal monsoon in Asia and its impact on the variability of monsoon rainfall in southeast Asia. Geosci. Front. 6, 817–823. doi: 10.1016/j.gsf.2014.02.009
Ly, P., Jensen, L.S., Bruun, T.B., Rutz, D., and de Neergaard, A. (2012). The system of rice intensification: adapted practices, reported outcomes and their relevance in Cambodia. Agr. Syst. 113, 16–27. doi: 10.1016/j.agsy.2012.07.005
Ly, P., Jensen, L. S., Bruun, T. B., and de Neergaard, A. (2016). Factors explaining variability in rice yields in a rain-fed lowland rice ecosystem in southern cambodia. NJAS-Wageningen J. Life Sci. 78, 129–37. doi: 10.1016/j.njas.2016.05.003
Maier, H. R., Guillaume, J. H. A., van Delden, H., Riddell, G. A., Haasnoot, M., and Kwakkel, J. H. (2016). An uncertain future, deep uncertainty, scenarios, robustness and adaptation: how do they fit together? Environ. Model. Softw. 81, 154–164. doi: 10.1016/j.envsoft.2016.03.014
Memarzadeh, M., and Boettiger, C. (2018). Adaptive management of ecological systems under partial observability. Biol. Conserv. 224, 9–15. doi: 10.1016/j.biocon.2018.05.009
Midgley, G. (1992). The sacred and profane in critical systems thinking. Syst. Pract. 5, 5–16. doi: 10.1007/BF01060044
Miller, C. A., and Wyborn, C. (2018). Co-production in global sustainability: histories and theories. Environ. Sci. Policy. doi: 10.1016/j.envsci.2018.01.016. [Epub ahead of print].
Milly, P. C., Betancourt, J., Falkenmark, M., Hirsch, R. M., Kundzewicz, Z. W., Lettenmaier, D. P., et al. (2008). Stationarity is dead: whither water management? Science. 319, 573–574. doi: 10.1126/science.1151915
Milne, S., and Mahanty, S. (2015). Conservation and Development in Cambodia: Exploring Frontiers of Change in Nature, State and Society. London; New York, NY: Routledge.
MRC (2017). The Study on Sustainable Development and Management of the Mekong River, including the Impacts of Mainstream Hydropower Projects. Veintiane: Mekong River Commission, Lao PDR. Available online at: http://www.mrcmekong.org/highlights/the-study-on-sustainable-management-and-development-of-the-mekong-river-including-impacts-of-mainstream-hydropower-projects/ (accessed January 18, 2019).
Ostrom, E. (2009). A general framework for analyzing sustainability of social-ecological systems. Science. 325, 419–422. doi: 10.1126/science.1172133
Pahl-Wostl, C. (2017). Governance of the water-energy-food security nexus: a multi-level coordination challenge. Environ. Sci. Policy. 92, 356–367. doi: 10.1016/j.envsci.2017.07.017
Peronne, D., and Hornberger, G. M. (2014). Water, food, and energy security: scrambling for resources or solutions? WIREsWater. 1, 49–68. doi: 10.1002/wat2.1004
Pielke, R. (2012). Post-normal science in a German landscape. Nat. Cult. 7, 196–212. doi: 10.3167/nc.2012.070205
Poff, N. L., Brown, C. M., Grantham, T. E., Matthews, J. H., Palmer, M. A., Spence, C. M., et al. (2016). Sustainable water management under future uncertainty with eco-engineering decision scaling. Nat. Clim. Change 6, 25–34. doi: 10.1038/nclimate2765
Pohl, C. (2005). Transdisciplinary collaboration in environmental research. Futures. 37, 1159–1178. doi: 10.1016/j.futures.2005.02.009
Pohl, C. (2010). From transdisciplinarity to transdisciplinary research. J. Eng. Sci. 1, 74–83. doi: 10.22545/2010/0006
Polasky, S., Carpenter, S. R., Folke, C., and Keeler, B. (2011). Decision-making under great uncertainty: environmental management in an era of global change. Trends Ecol. Evol. 26, 398–404. doi: 10.1016/j.tree.2011.04.007
Poppy, G. M., Chiotha, S., Eigenbrod, F., Harvey, C. A., Honzák, M., Hudson, M. D., et al. (2014). Food security in a perfect storm: using the ecosystem services framework to increase understanding. Philos. Trans. R. Soc. Brit. 369, 1–13. doi: 10.1098/rstb.2012.0288
Posner, S. M., McKenzie, E., and Ricketts, T. H. (2016). Policy impacts of ecosystem services knowledge. Proc. Natl. Acad. Sci. U.S.A. 113, 1760–1765. doi: 10.1073/pnas.1502452113
Ramaswami, A., and Chavez, A. (2013). What metrics best reflect the energy and carbon intensity of cities? Insights from theory and modeling of 20 US cities. Environ. Res. Lett. 8:035011. doi: 10.1088/1748-9326/8/3/035011
Raskin, P., Monks, F., Ribeiro, T., van Vuuren, D., and Zurek, M. (2014). “Global scenarios in historical perspective,” in Ecosystems and Human Well-Being, eds S. R. Carpenter, P. L. Pingali, E. M. Bennett, and M. B. Zurek (Washington, DC: Island Press), 35–44.
Rasul, G. (2016). Managing the food, water, and energy nexus for achieving the sustainable development goals in South Asia. Environ. Dev. 18, 14–12. doi: 10.1016/j.envdev.2015.12.001
Rayner, S. (2012). Uncomfortable knowledge: the social construction of ignorance in science and environmental policy discourses. Econ. Soc. 41,107–125. doi: 10.1080/03085147.2011.637335
RGC (2016). DRAFT Cambodia Power Development Plan. Ministry of Industry, Mines and Energy. Phnom Penh: Royal Government of Cambodia.
Ringler, C., Bhaduri, A., and Lawford, R. (2013). The nexus across water, energy, land and food (WELF): potential for improved resource use efficiency? Curr. Opin. Environ. Sustain. 5, 617–624. doi: 10.1016/j.cosust.2013.11.002
Roberts, E., and Barton, B. (2015). Feeding Ourselves Thirsty: How the Food Sector is Managing Global Water Risks. Boston, MA: Ceres.
Sandiford, P. J. (2015). “Participant observation as ethnography or ethnography as participant observation in organizational research,” in The Palgrave Handbook of Research Design in Business and Management, ed K. D. Strang (New York, NY: Palgrave Macmillan), 411–446.
Schwandt, T. A. (1989). Solutions to the paradigm conflict: coping with uncertainty. J. Contemp. Ethnogr. 17, 379–407. doi: 10.1177/089124189017004001
Scott, A. (2017). Making Governance Work for Water–Energy–Food Nexus Approaches. Climate and Development Knowledge Network (CDKN) Working Paper Series. London: Overseas Development Institute.
Senge, P. M., and Sterman, J. D. (1992). Systems thinking and organizational learning: acting locally and thinking globally in the organization of the future. Eur. J. Oper. Res. 59, 137–150. doi: 10.1016/0377-2217(92)90011-W
Shannak, S., Mabrey, D., and Vittorio, M. (2018). Moving from theory to practice in the water–energy–food nexus: an evaluation of existing models and frameworks. Water Energy Nexus. 1, 17–25. doi: 10.1016/j.wen.2018.04.001
Siciliano, G., Urban, F., Kim, S., and Lonn, P. D. (2015). Hydropower, social priorities and the rural–urban development divide: the case of large dams in Cambodia. Energy Policy. 86, 273–285. doi: 10.1016/j.enpol.2015.07.009
Simpson, G. B., and Jewitt, G. (2019). The development of the water-energy-food nexus as a framework for achieving resource security: a review. Front. Environ. Sci. 8. doi: 10.3389/fenvs.2019.00008
Sterman, J. (2000). Business Dynamics: Systems Thinking and Modeling for a Complex World. New York, NY, Irwin/McGraw-Hill.
Stirling, A. (2015). Developing ‘Nexus Capabilities': Towards Transdisciplinary Methodologies. Technical Paper. Sussex: The Nexus Network.
Tilman, D., and Clark, M. (2014). Global diets link environmental sustainability and human health. Nature. 515, 518–522. doi: 10.1038/nature13959
Uusitalo, L., Lehikoinen, A., Helle, I., and Myrberg, K. (2015). An overview of methods to evaluate uncertainty of deterministic models in decision support. Environ. Model. Softw. 63, 24–31. doi: 10.1016/j.envsoft.2014.09.017
Vennix, J. A. M., Akkermans, H. A., and Rouwette, E. A. J. A. (1996). Group model building to facilitate organizational change: an exploratory study. Syst. Dyn. Rev. 12, 39–58. doi: 10.1002/(SICI)1099-1727(199621)12:1<39::AID-SDR94>3.0.CO;2-K
Videira, N., Antunes, P., Santos, R., and Lopes, R. (2010). A participatory modelling approach to support integrated sustainability assessment processes. Syst. Res. Behav. Sci. 27, 446–460. doi: 10.1002/sres.1041
Voinov, A., and Brown Gaddis, E. J. (2008). Lessons for successful participatory watershed modeling: a perspective from modeling practitioners. Ecol. Model. 216, 197–207. doi: 10.1016/j.ecolmodel.2008.03.010
Warmink, J. J., Brugnach, M., Vinke-de Kruijf, J., Schielen, R. M. J., and Augustijn, D. C. M. (2017). Coping with uncertainty in river management: challenges and ways forward. Water Res. Manag. 31, 4587–4600. doi: 10.1007/s11269-017-1767-6
Weitz, N., Carlsen, H., Nilsson, M., and Skånberg, K. (2017a). Towards systemic and contextual priority setting for implementing the 2030 agenda. Sustain. Sci. 13, 531–548. doi: 10.1007/s11625-017-0470-0
Weitz, N., Strambo, C., Kemp-Benedict, E., and Nilsson, M. (2017b). Closing the governance gaps in the water-energy-food nexus: insights from integrative governance. Glob. Environ. Change 45, 165–73. doi: 10.1016/j.gloenvcha.2017.06.006
Wyborn, C., van Kerkhoff, L., Dunlop, M., Dudley, N., and Guevara, O. (2016). Future oriented conservation: knowledge governance, uncertainty and learning. Biodiv. Conserv. 25, 1401–1408. doi: 10.1007/s10531-016-1130-x
Wyborn, C. A. (2015). Connecting knowledge with action through coproductive capacities: adaptive governance and connectivity conservation. Ecol. Soc. 20:11. doi: 10.5751/ES-06510-200111
Keywords: water-energy-food, nexus, governance, modeling, futures thinking, scenario planning, stakeholder engagement
Citation: Yung L, Louder E, Gallagher LA, Jones K and Wyborn C (2019) How Methods for Navigating Uncertainty Connect Science and Policy at the Water-Energy-Food Nexus. Front. Environ. Sci. 7:37. doi: 10.3389/fenvs.2019.00037
Received: 11 September 2018; Accepted: 01 March 2019;
Published: 12 April 2019.
Edited by:
Rabi Mohtar, Texas A&M University, United StatesReviewed by:
Richard Meissner, Council for Scientific and Industrial Research (CSIR), South AfricaNidhi Nagabhatla, United Nations University Institute for Water Environment and Health, Canada
Copyright © 2019 Yung, Louder, Gallagher, Jones and Wyborn. This is an open-access article distributed under the terms of the Creative Commons Attribution License (CC BY). The use, distribution or reproduction in other forums is permitted, provided the original author(s) and the copyright owner(s) are credited and that the original publication in this journal is cited, in accordance with accepted academic practice. No use, distribution or reproduction is permitted which does not comply with these terms.
*Correspondence: Laurie Yung, laurie.yung@umontana.edu