- 1School of Geography, University of Leeds, Leeds, United Kingdom
- 2Center for Climate and Resilience Research, Universidad de Chile, Santiago, Chile
- 3Department of Geophysics, Universidad de Chile, Santiago, Chile
- 4Instituto de Ciencias de la Tierra, Facultad de Ciencias, Universidad Austral de Chile, Valdivia, Chile
- 5School of Earth and Environment, University of Leeds, Leeds, United Kingdom
- 6Institut für Geographie, Friedrich-Alexander-Universität Erlangen-Nürnberg, Erlangen, Germany
Recent evidence shows that most Patagonian glaciers are receding rapidly. Due to the lack of in situ long-term meteorological observations, the understanding of how glaciers are responding to changes in climate over this region is extremely limited, and uncertainties exist in the glacier surface mass balance model parameterizations. This precludes a robust assessment of glacier response to current and projected climate change. An issue of central concern is the accurate estimation of precipitation phase. In this work, we have assessed spatial and temporal patterns in snow accumulation in both the North Patagonia Icefield (NPI) and South Patagonia Icefield (SPI). We used a regional climate model, RegCM4.6 and four Phase Partitioning Methods (PPM) in addition to short-term snow accumulation observations using ultrasonic depth gauges (UDG). Snow accumulation shows that rates are higher on the west side relative to the east side for both icefields. The values depend on the PPM used and reach a mean difference of 1,500 mm w.e., with some areas reaching differences higher than 3,500 mm w.e. These differences could lead to divergent mass balance estimations depending on the scheme used to define the snow accumulation. Good agreement is found in comparing UDG observations with modeled data on the plateau area of the SPI during a short time period; however, there are important differences between rates of snow accumulation determined in this work and previous estimations using ice core data at annual scale. Significant positive trends are mainly present in the autumn season on the west side of the SPI, while on the east side, significant negative trends in autumn were observed. Overall, for the rest of the area and during other seasons, no significant changes can be determined. In addition, glaciers with positive and stable elevation and frontal changes determined by previous works are related to areas where snow accumulation has increased during the period 2000–2015. This suggests that increases in snow accumulation are attenuating the response of some Patagonian glaciers to warming in a regional context of overall glacier retreat.
Introduction
Patagonia is the largest glaciated area in the Southern Hemisphere outside Antarctica. Patagonian glaciers show a strong sensitivity to climate variations and recent evidence shows that most of these glaciers are receding rapidly (Davies and Glasser, 2012; White and Copland, 2015; Meier et al., 2018). This deglaciation process is a matter of concern due to their observed and potential contribution to sea level rise (Rignot et al., 2003; Willis et al., 2012; Gardner et al., 2013). Despite this, only a few studies have focused on understanding how glaciers are responding to changes in climate over this important region of the Southern Hemisphere (Pellicciotti et al., 2014; Malz et al., 2018). The main reason for the lack of research studies over this region is associated with the scarcity of in situ long-term meteorological observations, especially on the plateau of both icefields, where extreme weather conditions prevail throughout the year.
Previous studies have focused on comparing surface elevation changes during recent decades derived from digital elevations model (DEMs) obtained from topographic and satellite data in both the North Patagonia Icefield (NPI) and the South Patagonia Icefield (SPI). Overall, these analyses show surface lowering in almost all the NPI, with some exceptions in the accumulation zones (Rivera et al., 2007; Dussaillant et al., 2018; Foresta et al., 2018). Meanwhile, negative changes are concentrated in the northern sector of the SPI (Foresta et al., 2018; Malz et al., 2018), whereas the south-central sector shows near balance conditions and even positive changes in elevation (e.g., Pío XI Glacier). As might be expected, the highest negative trends are concentrated in the ablation zone of the glaciers. The heterogeneity in glacial elevation changes reveals a complex spatial structure of the glacier responses in this region. The overall observed negative changes in surface elevation contrast with surface mass balance models studies that largely illustrate positive trends on both the NPI and SPI (Schaefer et al., 2013, 2015; Mernild et al., 2016b; Weidemann et al., 2018). One explanation suggested for this discrepancy is increasing ice flow velocities associated with ice loss due to calving. Unfortunately, to date there are no empirically-based studies of the meteorological conditions over both sides of the Patagonian Icefields that can constrain the mass balance modeling studies. Due to the lack of observation-based analyses, high uncertainties exist in the glacier surface mass balance model parameterizations precluding a complete validation of the simulated accumulation (Villarroel et al., 2013) as well as a robust assessment of the response of the glaciers to projected climate change.
Despite recent and past efforts, one of the main components of the glacier mass balance, the snowfall and hence the snow accumulation in Patagonia, remains poorly understood. Snow accumulation has been estimated at different scales from Global and Regional Climate Models (Schaefer et al., 2013, 2015; Lenaerts et al., 2014; Mernild et al., 2016a; Weidemann et al., 2018) to point scale estimates from stake observations (Rivera, 2004) and ice core data (Yamada, 1987; Matsuoka and Naruse, 1999; Shiraiwa et al., 2002; Schwikowski et al., 2013). Considering the spatial extent of both Patagonian Icefields, ice cores and stake estimates are only representative of local conditions. Furthermore, the lack of observed accumulation data causes high uncertainties in the simulations regarding the glacier-wide amount of solid precipitation. For example, using a mass balance model for Tyndall and Gray glaciers on the SPI, Weidemann et al. (2018) showed that the accumulation values are significantly lower than those estimated by Mernild et al. (2016b). These discrepancies in the estimations are partially associated with atmospheric forcing fields and different precipitation schemes used in the mass balance models.
The accurate estimation of precipitation phase is an issue of central concern regarding the uncertainties of mass balance models. To calculate accumulation in Patagonia, the precipitation phase has been parameterized using air temperature as the input for typical Phase Partitioning Methods (PPMs) such as static threshold (e.g., Rivera, 2004; Koppes et al., 2011) and linear transition (e.g., Schaefer et al., 2013, 2015; Weidemann et al., 2018) methods. However, recent studies have shown that precipitation phase is not only a function of surface air temperature, but is also influenced by relative humidity, wind speed, stability of the atmosphere, and interaction between hydrometeors (Behrangi et al., 2018 and references therein). According to Harpold et al. (2017), the first step toward improved hydrological (and glaciological) modeling in areas with a mixed precipitation phase such as the NPI and SPI, is to educate the scientific community about current techniques and their limitations and to highlight the areas where research is most needed.
Given the general lack of data and analysis on accumulation estimates and thus associated uncertainties, as well as its relevance to understanding snow accumulation processes on the Patagonian Icefields, the main goal of this work is to analyze snow accumulation over the period 1980–2015 using a regional climate model simulation (RegCM4.6, at ~10 km spatial resolution) and short-term on-glacier accumulation observations. In the first section, we compare the results of four PPMs, three of them previously used in the region. In the second section, we analyze the seasonal trends in snow accumulation over the period 1980–2015. In the third section, we compare the datasets with the in situ observations and previous estimates made in the scientific literature. Finally, the results are discussed in terms of the implications for glacier surface mass balance modeling and the observed glacier response.
Materials and Methods
Study Area
The study area includes the two largest temperate ice masses located in the Southern Hemisphere: the North Patagonia Icefield (NPI) and the South Patagonia Icefield (SPI) (Figure 1). The NPI extends from 46°30′S to 47°30′S (Figure 1B), stretching almost 125 km north–south. It covered an area of ~3,675 km2 in 2016 (Meier et al., 2018) extending from sea level to elevations in excess of 4,000 m a.s.l. at the summit of Mount San Valentin. It is composed of 38 glaciers larger than 0.5 km2 (Dussaillant et al., 2018).
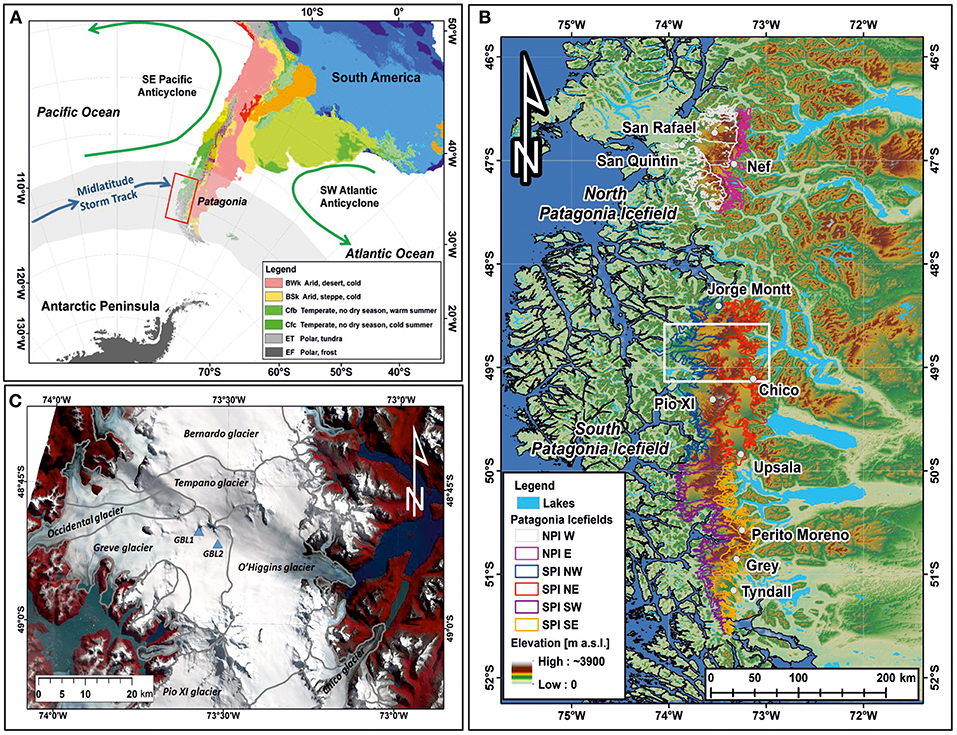
Figure 1. (A) Regional climatic setting over the Patagonian region. Colors correspond to the predominant Köppen-Geiger climate classification for Patagonia (Beck et al., 2018) and the red rectangle corresponds to the study area. (B) SRTM topography at 1 km resolution of the study area and locations of some glaciers mentioned in the text, and (C) localization of the observations (Ultrasonic Depth Gauges) used in this work. The satellite image was acquired by Landsat 8 (OLI) on the 8 April 2014.
The SPI spreads over 350 km between the latitudes 48°20′S and 51°30′S (Figure 1B), and is the most glaciated zone of the Andes, covering an area of 12,232 km2 in 2016 (Meier et al., 2018). The SPI includes a total of 48 main glacier basins, ending mainly in fjords on the western side and in lakes on the eastern side (Aniya et al., 1996). These glaciers are joined in the accumulation zone (“plateau”), with an average altitude of 1,600 m a.s.l., and a measured thickness of more than 700 m (Rivera and Casassa, 2002) with a maximum estimate of ice thickness of ~1,250 m (Gourlet et al., 2016).
This zone is strongly influenced by frontal systems associated with mid-latitude cyclones forming over the South Pacific Ocean (Figure 1A). Given the complex terrain and extreme environment, there are a small number of meteorological stations (Garreaud et al., 2013) that only partially capture the climatology and meteorological conditions of this zone, especially at higher elevations. Data from the Climate Research Unit (CRU; Sagredo and Lowell, 2012) and from Puerto Eden weather station located in the fjord zone (49° 08′ S, 74° 25′ W, Carrasco et al., 2002), show that this zone is characterized by a seasonal variation in temperature with minimum values in the winter months (~3°C) and maximum values in summer (~11°C). South of 49°S, the precipitation is equally distributed throughout the year, with slightly maximum values in March and April (Sagredo and Lowell, 2012). However, given that Patagonian Icefields are in a transition zone, immediately to the north there is a marked annual cycle in the precipitation, with a winter maximum. At inter-annual scales, precipitation variability responds to hemispheric scale patterns associated with the Southern Annular Mode (SAM) and El Nino Southern Oscillation (ENSO), which determine the year-to-year variability of the precipitation. Multidecadal variations are related to the Inter-decadal Pacific Oscillation (IPO; Garreaud et al., 2013). The SAM mode presents a significant impact on regional climate, where positive SAM is associated with higher temperatures (Gillett et al., 2006), an intensification of the westerlies toward higher latitudes (Hall and Visbeck, 2002) and an increase in precipitation over the south of the study area. Negative SAM leads to opposite effects. This is particularly of interest since SAM has shown a positive trend during the second half of the 20th century (Marshall, 2003) and is associated with anthropogenic effects (Abram et al., 2014) that will potentially affect climatological trends in coming decades.
Although the southern Patagonian Andes is a relatively low-elevation mountain belt, it has an influence on the longitudinal distribution of the precipitation in the region and generates an extreme climatic gradient, leading to very humid western slopes and dry eastern slopes (Schneider et al., 2003; Smith and Evans, 2007; Lenaerts et al., 2014). Garreaud et al. (2013) showed that the precipitation at regional scale is positively or negatively correlated with zonal wind component at 850 hPa, in the western or eastern sectors of the Andes, respectively, due to the mechanical effect of the mountain chain that forces air to ascend in the western sector (windward side). On the other hand, these conditions favor downslope subsidence, and thus lead to arid conditions on the leeward side (Garreaud et al., 2013). Given these conditions, annual mean precipitation varies between 5,000 and 10,000 mm yr−1 on the windward side, while it decreases to < ~300 mm yr−1 on the leeward side (Garreaud et al., 2013).
In addition to the precipitation characteristics, increases in air temperature are considered to have an impact on the mass balance (Cook et al., 2003), as these glaciers lie in the range of the 0°C isotherm. For instance, increases in air temperature may cause a compound effect; first, more energy becomes available for melting, and second, with a small increase in air temperature during the accumulation season, air temperature may rise above 0°C and alter the partitioning between rain and snow (Rasmussen et al., 2007). These changes eventually have an impact on the glacier's net mass balance. This indicates that the Patagonian glaciers are mainly sensitive to air temperature increase, since ablation is dominated by melt (Sagredo and Lowell, 2012). It is also stated that warming is the main cause for glacier retreat over the region (Masiokas et al., 2009). Between 43 and 49°S, a warming in the land surface temperature of 0.78°C/per decade in the period 2001–2016 was determined by Olivares-Contreras et al. (2018). In Southern Patagonia (~50°S), warming of ~0.5°C in the last 40 years was detected by Rasmussen et al. (2007) using NCEP-NCAR reanalysis at 850 hPa level.
Observations
We used data obtained from two ultrasonic depth gauges (UDGs) (Figure 1C). These UDGs (model SR50) were installed directly on the glacier snow surface over the plateau (CECs-DGA, 2016). In the same structure, two air temperature sensors were also installed at an initial height of 2 and 4 m above ground level. Data obtained at 15 min time steps from these stations, referred to as Glacier Boundary Layer (GBL) air temperature stations (GBL1, GBL2 in Figure 1C and Table 1), are used to validate and compare snow accumulation against the estimated values obtained with the different PPMs (next sections). First, we separate the data between accumulation and ablation, before visually filtering the SR50 data to discard outlier values. Then, a moving average at hourly scale was applied in order to reduce the noise. Finally, the hourly data in meters was converted to mm w.e. using the method of estimation of density (ρs) of Hedstrom and Pomeroy (1998) based on air temperature (Ta) only:
Based on these results, we were able to estimate the daily accumulation.
Data Compilation
We compiled snow accumulation estimates of the Patagonian Icefields from previous studies to compare with the snow accumulation estimations performed in this work. Some studies measured the ablation and accumulation using short-term direct observation through the use of stakes. There is a lack of long-term mass balance measurements using the glaciological method on the NPI and SPI. In view of this fact, we also considered ice core and modeling estimations in this study.
We also compiled glaciological data related to observed glacier changes mainly between 2000 and 2015 in both the NPI and SPI. In the current study, these data obtained at glacier scale are used to support our analysis of glacier response and its implications. The compilation corresponds to elevation changes in the SPI during the period 2000–2015 (Malz et al., 2018), elevation changes in the NPI during the period 2000–2014 (Braun et al., 2019), ice velocity in both Icefields (Mouginot and Rignot, 2015), frontal changes in the SPI (Sakakibara and Sugiyama, 2014), and estimation of the accumulation-area ratio (AAR) in the NPI (Rivera et al., 2007) and in the SPI (Malz et al., 2018). Finally, we compiled glacier characteristics from the Randolph Glacier Inventory 6.0 (RGI Consortium, 2017).
Regional Climate Model (RegCM4.6)
Regional Climate Model, version 4.6 (RegCM4.6) is a three-dimensional, primitive equation, hydrostatic regional climate model maintained by the International Center for Theoretical Physics (ICTP). RegCM4 was originally developed by Giorgi et al. (1993a,b) and its dynamical core is based on the hydrostatic version of the Penn State/NCAR mesoscale model MM5 (Grell et al., 1994). RegCM has been used in numerous regional climate model simulations, even over regions dominated by complex terrain features such as the Atacama Desert (Bozkurt et al., 2016), the polar regions (Grassi et al., 2013; Bozkurt et al., 2018), and the European Alps (Giorgi et al., 2016). Multiple physical schemes are available in the model. RegCM4 simulations used in the present study are based on (1) the land surface model Biosphere-Atmosphere Transfer Scheme (BATS) of Dickinson et al. (1993); (2) the planetary boundary layer of Holtslag et al. (1990); (3) the radiative scheme of the NCAR Community Climate System Model Version 3 (CCSM3) (Kiehl et al., 1996); (4) the ocean flux parameterization of Zeng et al. (1998); and (5) the scheme of Pal et al. (2000) for representing resolvable precipitation. In terms of cumulus parameterization, there are several options for convective precipitation in the model; the Kuo-type scheme of Anthes (1977), the Grell scheme (Grell, 1993), the Emanuel scheme (Emanuel, 1991), and mixed schemes. Based on a couple of test simulations done with different convective schemes, the Grell scheme with a cumulus closure scheme of Fritsch and Chappell (Fritsch and Chappell, 1980) was applied in the simulations. A more detailed description of the model and physical parameterizations can be found in Giorgi et al. (2012).
The simulations were performed on two domains at 0.44° (~50 km) and 0.09° (~10 km) spatial resolutions and 23 vertical sigma levels with a one-way nesting approach. The topography of the icefields from 10 km simulations is given in Figure S1. The mother domain has 192 × 202 grid cells covering all South America and the nested domain has 320 × 520 grid cells centered on Chile and southwest South America based on a Rotated Mercator projection. Initial and boundary conditions for the mother domain were provided by the European Center for Medium-Range Weather Forecasts (ECMWF, ERA-Interim) Reanalysis dataset at 6-h intervals with a grid spacing of 0.75° resolution. The nested domain was then forced by the 3D atmospheric outputs of the model domain at 6-h intervals. ERA-Interim sea surface temperature fields (6-h intervals, 0.75° resolution) were used as surface boundary conditions. The simulations were performed continuously from 1 January 1979 to 31 December 2015. The first year of simulations (1979) was selected as the spin-up period and, thus, was not considered in the analysis.
Ongoing work found that, overall, RegCM4 is capable of reproducing mean spatial fields of important large-scale features such as South Pacific Subtropical Anticyclone dry regime, westerlies over Patagonia and low-level moisture distribution along both sides of the Andes barrier. The same study also demonstrates that the simulations tend to have stronger westerlies over Patagonia and further suggest that 10-km simulation results exhibit a reasonable representation of spatial and temporal variability of temperature and precipitation. For instance, high-resolution simulation results reproduce reasonably well the observed spatial pattern of frost days with a close estimate of the observed number of days over the Patagonian Icefields.
Phase Partitioning Methods (PPMs)
To calculate and assess the snow accumulation under different schemes, four methods were used to separate between snowfall and rain. The primary input is the 10-km total precipitation simulations from RegCM4.6, as our intention is to assess the snow accumulation under four PPMs, three of them previously used in the Patagonian Icefields. In addition, 10-km simulations from RegCM4.6 of near-surface air temperature, relative humidity (at 2 m), and atmospheric surface pressure were used. In all cases, we used daily model output. In all these methods, the required inputs are air temperature and total precipitation, while in the fourth method a broader range of meteorological inputs are needed.
The first method considers a threshold value in the air temperature to separate between rainfall and snowfall. The chosen threshold temperature is 2°C as this value was used in studies of the San Rafael Glacier in the NPI (Koppes et al., 2011) and the Chico Glacier in the SPI (Rivera, 2004). In this article, we refer to this method as 2C. This static temperature threshold is close to that used by the BATs scheme
The second method was used by Weidemann et al. (2018) (WE, hereafter), where the fraction of solid precipitation (r) is estimated according to:
In this equation, the proportion of solid precipitation to total precipitation is smoothly scaled between 100 and 0% within an air temperature range of 0–2°C, meaning that under 0°C the fraction of solid precipitation is 100%, while above the 2°C the fraction of solid precipitation is 0%. This method has been also applied to the Gran Campo Nevado Icefield in southernmost Patagonia (Möller et al., 2007; Weidemann et al., 2013).
The third method was used by Schaefer et al. (2013, 2015) in the NPI and SPI (SC, hereafter). The accumulation (q) is the fraction of the solid precipitation which is determined by the temperature (Ta) in the grid cell (I):
Finally, the fourth method corresponds to the parameterization proposed by Ding et al. (2014) (DI, hereafter). This scheme was constructed using climate data from China and only evaluated over China; however, evaluations for further regions are recommended (Ding et al., 2014). This scheme defines three types of precipitation (snow, sleet and rain) using minimum and maximum temperatures (Tmin and Tmax) and the wet-bulb temperature Tw [°C] as threshold:
Tw is calculated using:
where esat(Ta) is the saturated vapor pressure which is only a function of the air temperature and is calculated using the empirical equation of Bolton (1980), and ps is the surface atmospheric pressure in hPa.
The three parameters (ΔT, ΔS, T0) of this model depend not only on air temperature but also on other conditions such as relative humidity (f, ranges from 0 to 1) and the elevation of the point in km (Z):
ΔT is the difference between the temperature of the probability threshold for snow (or snow and sleet) and the temperature of the centralized probabilities curve. ΔS represents a temperature scale in which an increase in value leads to widening of the temperature range of snow/ratio transitions. T0 is the temperature that approximately represents the center of Tw range in which snow/rain transitions happens. This method is dynamic as it is dual-threshold when f > 78% and single threshold method when f ≤ 78%. The complete parameterization scheme and explanation for precipitation type discrimination and data used can be found in Ding et al. (2014).
To analyze the spatial differences in the snow accumulation spatial patterns and annual cycle, we divide the study area into six zones, classifying the glaciers according to their location. The NPI is separated into west (NPI W; the San Rafael and San Quintin glaciers) and east (NPI E; the Nef, Colonia, Pared Norte, Soler, Leones, etc. glaciers) while the SPI is separated into four zones, SPI north-west (SPI NW, the Jorge Montt, Bernardo, Témpano, Occidental, Greve, and Pío XI glaciers), SPI north-east (SPI NE; the O'Higgins, Chico, and Viedma glaciers), SPI south-west (SPI SW; the HPS29, HPS31, HPS34, Asia, and Amalia glaciers) and SPI south-east (SPI SE; the Ameghino, Perito Moreno, Gray, and Tyndall glaciers).
Trend Analysis
In order to identify trends in annual and seasonal snow accumulation for the four PPM in the period 1980–2015, we used two methods—linear least-squares slopes and Sen's slope tests. The linear least-square slopes and the Sen's slope test allow to compute the magnitude of the trend. The slope of the snow accumulation at each season and at annual scale is regressed on time and hence used to quantify temporal trends. The Sen's slope test uses a linear model to estimate the slope of the trend choosing the median of the slopes, and the variance of the residuals should be constant in time (Salmi et al., 2002). We also estimate the statistical significance at 95% (p-value <0.05) using the Mann-Kendall test. The trend calculation is also applied to seasonal time series values of each zone [Figure 1 and defined in section Phase Partitioning Methods (PPMs)] and to individual glaciers in the period 2000–2015, in order to compare with the trends in the geodetic mass balance over the same period.
Results
Inter-comparison of PPMs
The spatial patterns in the distribution of annual snow accumulation are similar between the four PPM methods (Figure 2). All PPM methods driven by the data from RegCM4.6 show that the west sides of both Patagonian Icefields receive a higher amount of snowfall relative to the east side, indicating the capability of RegCM4.6 to capture the extreme orographic effect (Table 2 and Figure 2). Overall, in all the zones, the 2C method shows the highest amounts of snow accumulation, while the DI method shows the lowest values (Table 2). Interestingly, if we consider the sleet type as accumulation in the DI method, the total (snow plus sleet, Table 2 and Figure S2) is of a similar magnitude to that of the 2C method. This suggests that the air temperature threshold used in the 2C method is appropriate for estimating the total rain, if we compare with the DI method. The WE and SC method are of similar magnitude at annual and season scale (Figure 2; Tables 2, S2). The interannual variability (Table 2) and seasonal scale (Table S1) is higher on the west side in both icefields. Finally, the sleet type precipitation (Figure S2) shows a higher rate on the western side of both icefields, in line with the more humid conditions that would be expected at the windward side of the Andes.
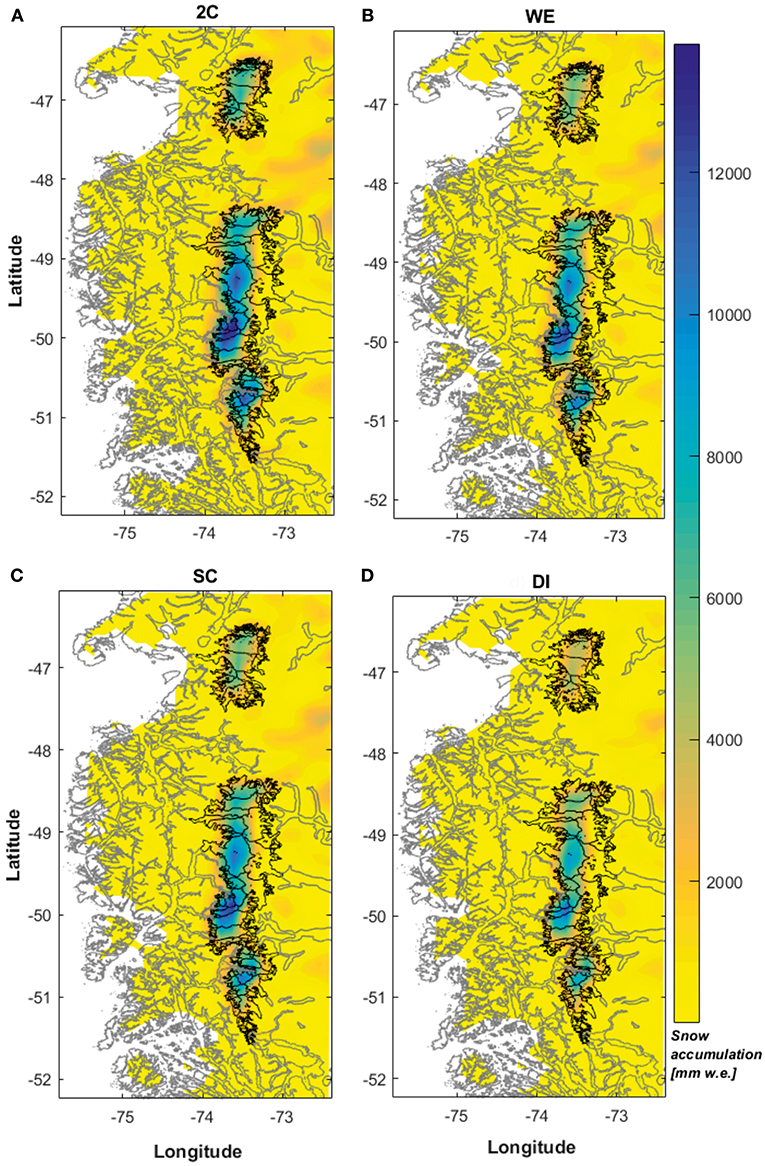
Figure 2. 1980–2015 annual mean value of snow accumulation (mm w.e.) for each PPM. (A) 2C, (B) WE, (C) SC, and (D) DI method. Icefield contours in black were obtained from RGI consortium (2017) and edited using a Landsat 8 image (OLI) of the 8 April 2014. Coastlines are in gray.
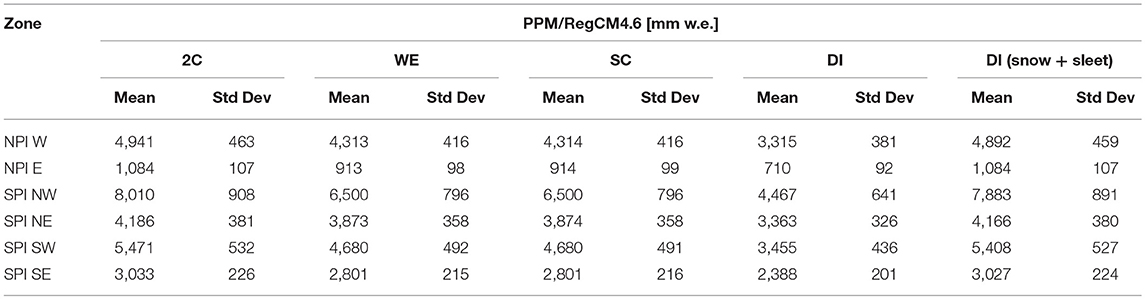
Table 2. Annual mean and standard deviation 1980–2015 of the snow accumulation values by zone and PPM.
A comparison of the maximum values of each PPM with respect to latitudinal variation of snow accumulation is given in Figure 3. Maximum values reach 14,000 mm w.e. in the 2C method, the DI model reaches 11,500 mm w.e., and the WE and SC methods are around 13,000 mm w.e. at the same latitude (Figure 3). This maximum accumulation point corresponds to the HPS15, HPS19, and Penguin glaciers in the SPI. Other areas with a high amount of accumulation are the accumulation zones of the Pío XI and HPS34 glaciers, with maximum values around 12,000 mm w.e. (2C method) and 9,000 mm w.e. (DI method). For the NPI, a similar amount of snow accumulation is estimated throughout the accumulation zones of the glaciers on the west side, with values between 4,000 and 7,500 mm w.e. The maximum value in the NPI depends on the PPM used (Figure 3). For instance, 2C shows a peak value (7,000 mm w.e.) in the accumulation zone of the San Quintin Glacier (~47°S) while the DI method shows maximum value on the San Rafael Glacier accumulation zone and on the Benito, HPN1, and Acodado glaciers (~5,000 mm w.e.).
The annual mean differences between the models that give the highest (2C) and lowest amounts of snow accumulation (DI) reach a mean value of 1,626 mm w.e. in NPI W, 373 mm w.e.in NPI E, 3,542 mm w.e. in SPI NW, 823 mm w.e. in SPI NE, 2,015 mm w.e. in SPI SW and 646 mm w.e. in SPI SE (Table 2). Details of the maximum and minimum amount of snow accumulation per zone and per season are given in Table S2.
The mean annual cycle of snow accumulation for each PPM indicates that, overall, the minimum and maximum values exist in February and August, respectively (Figure 4). However, the annual cycles show some differences in the amplitude depending on the location. For instance, east side glaciers in all the zones exhibit snow accumulation equally distributed throughout the year, while west zones show a marked cycle in the snow accumulation. In the north-south direction, the east side zones show that the amplitude of the annual cycle is small in the SPI NE and SPI SE zones relative to the NPI E.
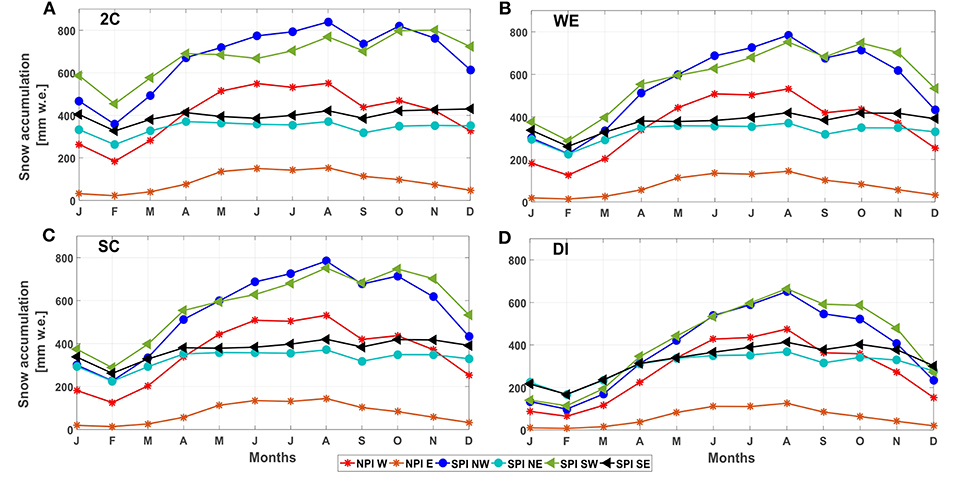
Figure 4. Annual cycle of snow accumulation for the sub-regions of both Patagonia Icefields. (A) 2C, (B) WE, (C) SC, (D) DI.
In summary, an overall spatial and temporal consistency exists between the four PPMs as all of them show consistently larger amounts of snow accumulation throughout the year on the west side zones of the icefields in comparison to those of the eastern zones. Also, similar annual cycles are simulated at each zone between the four PPMs. However, the absolute values in the snow accumulation simulated by the four PPMs differ widely in some cases.
Seasonal Trends 1980–2015
We further analyzed the seasonal trends obtained by three of the four PPMs (2C, WE, and DI) as the WE and SC methods show almost identical values and inter-annual variability. Trends obtained using Sen's slope and linear least-square slopes are similar spatially and in magnitude, therefore in this section we analyzed the result of the least-square slope (Figure 5), while the results of the Sen's slope are shown in the Supplementary Material, Figure S3.
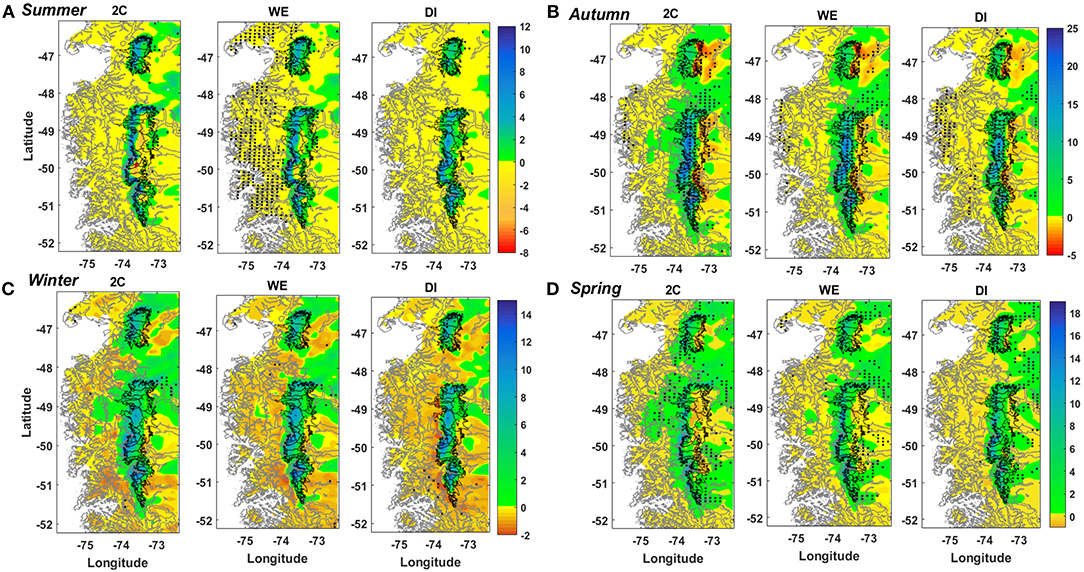
Figure 5. Seasonal trends 1980−2015 in mm w.e. yr−1 for three Phase Partitioning Methods. Points indicate statistically significant trend (p < 0.05). (A) Summer, (B) Autumn, (C) Winter and, (D) Spring. Icefield contours are in black and coastlines in gray.
Spatial heterogeneity exists in the trends, with some areas showing an increasing trend in snow accumulation while other areas show a decreasing trend or no change (Figure 5). Overall, throughout all the seasons, areas of no significant change correspond to terrain outside of both icefields. On the icefields, areas with increasing and decreasing trends in snow accumulation are concentrated on the plateau on the west and east sides of both icefields, respectively. Statistically significant positive trends are detected on the SPI in autumn. The location of these positive trends corresponds to the plateau or accumulation zones of the glaciers. On the other hand, in the ablation zones, especially those in the north-west section of the SPI (e.g., Témpano, Bernardo, Pío XI) there are no significant positive trends, and in some areas negative trends exist. In autumn, the significant negative trends are concentrated on the east side of the NPI and on the east side of the SPI in the zones of the O'Higgins, Chico, Upsala, Viedma, Ameghino, and Perito Moreno glaciers. In these areas, negative trends are also detected in spring but the values are not significant. In winter, a predominance of positive, although not significant, trends is determined on both icefields.
The time series of each zone are similar between the PPMs and [see section Phase Partitioning Methods (PPMs)] shows an important inter-annual variability. For instance, for the DI method in autumn (Figure 6), the generally positive trend was interrupted in 1997, with the snow accumulation values sharply dropping more than 500 mm w.e. for both icefields. Interestingly, the highest snow accumulation corresponds to the winter season of the same year, with values reaching around 3,000 mm w.e. in the west side of the SPI. Using 2C and WE methods, the winter 1998 values are close to 4,000 mm w.e. (not shown).
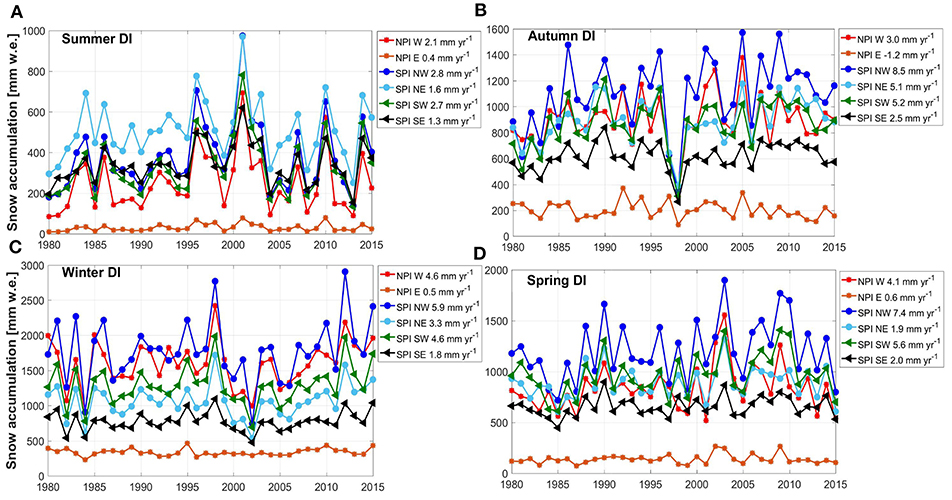
Figure 6. Seasonal time series and trends of the snow accumulation for six sub-regions of the study area using the DI method. (A) Summer, (B) Autumn, (C) Winter and, (D) Spring. The legend also indicates the annual trend.
In summary, spatial and temporal differences exist in the snow accumulation trends. Spatially, positive trends are concentrated on the west side of both icefields throughout all seasons, while negative trends are concentrated in some areas on the east side of both icefields during autumn, winter and spring. However, in both cases significant trends are only present in the autumn season.
Comparisons With Measured data
UDG Observations
We compared the estimated snow accumulations from the four PPMs, with the observations from the UDGs at locations GBL1 and GBL2 during the period October–December 2015 (Figure 7). Both stations are located at the same grid point of the RegCM4.6. Nevertheless, given that the elevation of this grid is 1,259 m a.s.l.; the grid characteristics are considered to be closer to the GBL2 (see Table 1). Regarding the GBL1, we used the grid point adjacent to the SW with an elevation of 1,492 m a.s.l., which is close to the real elevation of GBL1 (Table 1).
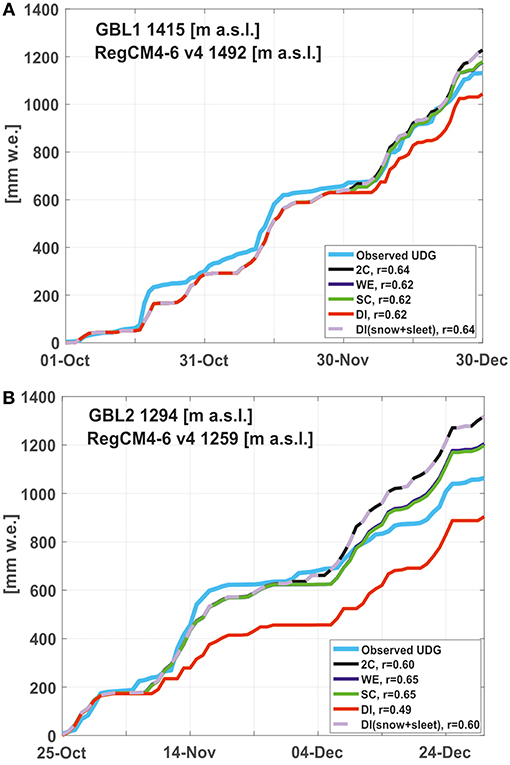
Figure 7. Comparison of accumulated snowfall using four PPMs and observations at (A) GBL1 and (B) GBL2. The correlation coefficient (r) values of each method are calculated in comparing with the observations.
At GBL1, the PPMs show a total accumulation between 1,000 and 1,200 mm w.e. while the data from the UDG suggests an accumulation of 1,100 mm w.e. The observed accumulations during October and November are of a higher magnitude relative to the estimated values of PPMs. Daily correlation coefficients (r) between the different PPMs and the observations are very close to each other (0.62 to 0.64). At GBL2, PPMs show some differences in the accumulated values, ranging between 900 and 1,300 mm w.e. while the observations suggest 1,050 mm w.e. Similar to GBL1, the r values are in the order of 0.60 and 0.65, except the one for the DI method (0.49, Figure 7). In the same location, the r value is higher (0.60) if the sleet type is considered as accumulation. Although some uncertainties may exist in estimating the accumulation from the UDGs (e.g., filter used), estimated values of the fourth PPMs using RegCM4.6 data perform well-compared to those obtained from the UDGs, especially at GBL1. The estimated values based on four PPMs are in the range of the observed values from the UDGs, but in terms of absolute values, the WE method is the most accurate at GBL1 (difference of 46 mm w.e.) while the SC method is the most accurate at GBL2 (difference of 135 mm w.e.). It is important to note that the regional climate models themselves may introduce uncertainty to the simulated variables associated with the physical configuration used in the model (e.g., radiation and cumulus schemes). Therefore, some inherent uncertainties may exist in the estimated values as well.
Previous Snow Accumulation Estimations and Comparisons
Details of the location of ice cores collected on the Patagonian Icefields are given in Table 3. These data were analyzed in order to obtain snow accumulation rates across the NPI and SPI (Table 3). Ice core data estimates an accumulation of 5,600 mm w.e at Nef Glacier in the NPI (Matsuoka and Naruse, 1999), while the estimated values based on the RegCM4.6 data are in the range of 4,100 to 5,000 mm w.e. for the same glacier. On the other hand, at Tyndall Glacier located in the SPI, differences between the ice core and RegCM4.6 estimates are even larger, reaching (in the case of the DI method) a maximum difference of 11,400 mm w.e. in the period between summer 1998 and summer 1999. Other comparisons of ice core and RegCM4.6-based estimates are given in Table 3. In all cases, estimations using Tyndall Glacier ice core data are larger than those four PPMs using the RegCM4.6 data. The opposite relation is found when comparing the RegCM4.6-based snow accumulation values with those estimated by ice core data (Schwikowski et al., 2013) from the accumulation zone of the Pío XI Glacier (~2,600 m a.s.l.). For instance, this ice core data estimates a mean annual accumulation of 5,800 mm w.e. (with a range of 3,400–7,100 mm w.e.) between the years 2001 and 2005. These values are not as high as others have previously estimated for the SPI. At this point, the estimate based on RegCM4.6 data illustrates a higher amount of accumulation values with differences of ~4,500 mm in 2001 and < ~800 mm w.e. in 2002. Other studies estimate a mean accumulation of 1,200 mm w.e. yr−1 in Perito Moreno Glacier of the SPI (50°38′S, 73°15′W, 2,680 m a.s.l., Aristarain and Delmas, 1993), which is lower than the annual rates of the current work.
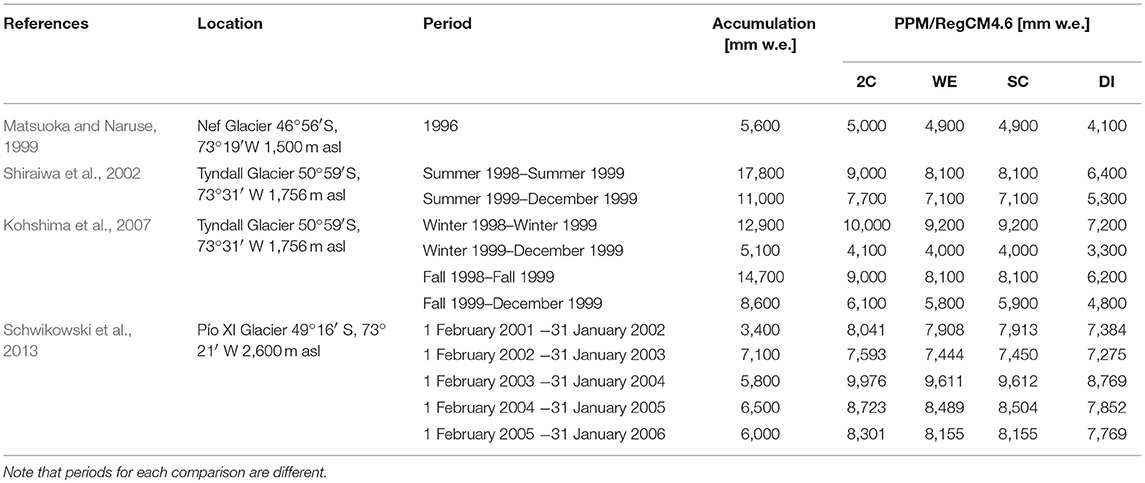
Table 3. Comparison of previous snow accumulation estimates using ice core data with the snow accumulation obtained in this work.
In terms of modeling snow accumulation, a recent study by Weidemann et al. (2018) estimated the total mean precipitation for two glaciers of the SPI—the Tyndall and Gray glaciers. The precipitation distribution was modeled using an analytical orographic precipitation model at 1 km resolution, then the SC method was applied to obtain the amount of solid precipitation. The comparison of these results with those obtained in the current study for the same hydrological years is presented in Figures 8A,B. Overall, the inter-annual variability (2000–2015) between the time series of the two estimations is similar, yet there are some differences in the estimated snow accumulation values. At Gray Glacier, the annual accumulation estimated by Weidemann et al. (2018) is in the range of values determined by the four PPMs. In the first six hydrological years, snow accumulation estimates based on DI and SC-WE methods are close to those estimated by Weidemann et al. (2018). However, in the later hydrological years, the estimates of the 2C method give closer values to those in Weidemann et al. (2018). At Tyndall Glacier, the estimates from Weidemann et al. (2018) are in the range of those estimated by the four PPMs, mainly concentrated between the values estimated using the DI and SC-WE methods.
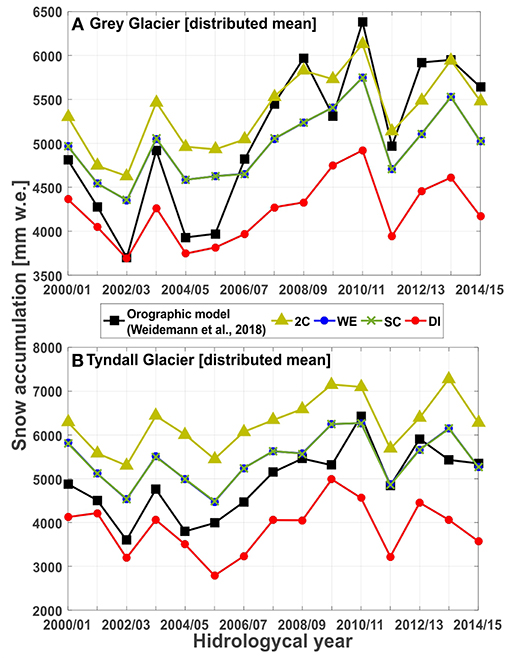
Figure 8. Comparison of annual snow accumulation using the four PPMs and estimates from Weidemann et al. (2018). (A) Distributed mean at Gray Glacier, and (B) distributed mean at Tyndall Glacier.
Previous observations of snow accumulation using stakes are limited to short and discrete periods, and to the ablation season. For example, Rivera (2004) used three stakes located at 1,445, 1,577, and 1,833 m a.s.l., and measured values of 9,630 ± 1,360 and 4,070 ± 540 mm yr−1 at the highest and lower stakes, respectively. The RegCM4.6-based estimates give rates in the order of 1,000 to 3,000 mm w.e. The distributions of the observed snow accumulation give an exponential relationship between precipitation and altitude, but if the precipitation is extrapolated to the higher altitudes of the Chico Glacier, the values reach 33,000 mm yr−1, which seem to be unrealistic (Rivera, 2004) and approximately three times larger than the rates estimated in the current work. It is important to note that these observations were only collected over a period of 14 to 34 days and then extrapolated to annual values.
In summary, good agreement is found in comparing UDG observations with modeled data on the plateau area of the SPI during a short time period; however, there are important differences between rates of snow accumulation determined in this work and previous estimates using ice core and stake observation data at annual scale.
Discussion
Performance of the RegCM4.6 Model and PPMs Parametrization
Overall, at a regional scale the RegCM4.6 reproduces the main precipitation characteristics of the study area, which are dominated by extreme orographic effects. All four PPMs lead to higher amounts of snow accumulation on the west side relative to the east side. Other models have also shown similar features. For instance, Lenaerts et al. (2014) used a high-resolution regional atmospheric climate model over Patagonia (RACMO2, 1979–2012) to show extreme orographic precipitation due to the narrow Andes barrier separating the wet windward side from the dry leeward side. Specifically, in terms of spatial differences in snow accumulation, Mernild et al. (2016b) indicated that snow accumulation at the same elevation is higher at the west side relative to the east side. Another interesting point is that sleet is higher at the west side of both icefields than that at the east side, indicating more humid and warmer conditions observed on the west side, eventually allowing for the occurrence of the mixed phase precipitation. Remote sensing observations also suggest the influence of the humid conditions on the snow facies. For instance, De Angelis et al. (2007) determined a relatively higher area of advanced snow metamorphism on the west side compared to the east side of the SPI. Outside of the icefields, in the north-east of the NPI (upper Baker River Basin), Krogh et al. (2015) estimated that snowfall is 28.5% of the total precipitation at basin scale. This value is in the range of the fractions estimated for this region in our work (25–35%).
On the other hand, some notable differences exist when comparing the previous estimates mainly with ice core-derived accumulation with those calculated in our work. The causes of this disparity are unclear but could conceivably be associated with differences between the local conditions at the ice core site extraction vs. the spatial resolution of RegCM4.6. Furthermore, erroneous estimates from ice core data due to water melt percolation (Rivera, 2004) or a more general underestimation of snow accumulation by the RegCM4.6 can be considered for the discrepancies in estimation of snow accumulation. Regardless, these differences highlight that uncertainties in quantifying the snow accumulation still exist in this extreme environment.
Another explanation of the differences between measured and modeled snow accumulation may be wind transport of blowing snow particles at local scale. For example, the annual differences between the snow accumulation derived in our analysis and the data from Schaefer et al. (2015) as well as the snow accumulation derived from Pío XI ice core (Table 3) suggest that even at a local point in the flat plateau of the Pío XI site, the influence of snow drift cannot totally be excluded. Coincidently, the ERA-Interim zonal wind speed shows that the year of 2002, in which lower differences in snow accumulation exist (Table 3), had lower wind speed distribution at 700 and 850 hPa levels (Figure S4). In contrast to this, the years of 2001 and 2003—with the largest differences in snow accumulation (Table 3)—had higher wind speeds at these same levels (Figure S4). Given that RegCM4 is forced by ERA-Interim, the wind speed variability can be further amplified by the regional climate model itself. Indeed, RegCM4 gives a systematic overestimation of 850 hPa zonal wind speed over large parts of the Icefields (Figure S5). These results illustrate the crucial role of local winds in controlling rates of snow accumulation, which is also indicated by Schwikowski et al. (2013) and Schaefer et al. (2015). In addition, Aristarain and Delmas (1993) also indicated the same mechanism to explain the low annual snow accumulation rate estimated in the accumulation zone of the Perito Moreno Glacier using an ice core. Thus, the derived net accumulation rates from these ice cores could represent lower limits in the snow accumulation.
In contrast, the estimated snow accumulation based on different PPMs using the RegCM4.6 data seems to agree with the observed snow accumulation, even at daily scale (r = ~0.6), during the October-December period of 2015. One reason is that UDG measurements are at 15 min time steps, capturing the actual snow accumulation. Therefore, UDGs are not affected by the effect of wind on the snow drift after the accumulation events. The location of the UDGs on the plateau, where gentle topography is present, is likely to reduce the differences between model and observation. On the other hand, the comparison of the hypsometric curve between the topography under 10 km and at 1 km resolutions (Figure S1) shows important differences at elevations over ~1,800 m a.s.l., which correspond to around the 20% of the total area of both icefields. Therefore, the higher elevations are not represented by the topography used in the RegCM4.6 simulations, illustrating a limitation of the model, which could explain the differences between the snow accumulation determined by Weidemann et al. (2018) for the Tyndall and Gray glaciers, where a higher resolution model (1 km) was used. It is important to note that local precipitation amount and snow accumulation depend on the correct representation of topography (Lenaerts et al., 2014). Therefore, given that ~10 km grid resolution is not able to resolve always and exactly the complex topography in the region, the total amount of estimated snow accumulation, especially in narrow glacier valleys (e.g., Chico and Nef glaciers), steep slopes and in the ice-rock transitions of both icefields, must be taken with caution. A robust statistical downscaling or bias correction method should therefore be applied if these data are to be used to drive mass balance estimations at glacier scale.
Snow Accumulation Changes 1980–2015
Previous modeling studies have also estimated an increase in snow accumulation over annual to decadal timescale. For example, Schaefer et al. (2013) simulated an increase of accumulation on the NPI, from 1990–2011 as compared to 1975–1990, and Mernild et al. (2016a) also estimated positive trends on the plateaus of both NPI and SPI during the period 1979–2014. These trends were detected at higher elevations above ~1,200 m a.s.l. and on the west side of the divide.
In contrast to the increased tendency of snow accumulation at higher elevations, observational-based studies focusing on the analysis of satellites images (e.g., MODIS, LANDSAT) show a reduction in snow cover area. For example, Pérez et al. (2018) estimated a non-significant decrease in snow cover over the Aysén River Catchment located north-east of our study area (45°–46°S). In the south of our study region, other studies suggested a reduction in snow cover area. For instance, further south, at Brunswick Peninsula (53°S), results show a significant decreasing trend of snow extent by 19% for the 1972–2016 period (Aguirre et al., 2018), while in Cordillera Darwin, Rojas-Zamorano et al. (2017) determined a decline of snow cover over the period from June 2005 to June 2016. It is important to note that the reduction in snow cover occurring at lower elevations is driven by its dependence on air temperature, at least at regional scale (Lopez et al., 2008). Therefore, an overall reduction in snow-covered area does not necessarily contradict the observed snow accumulation increase at the highest elevations. It should be noted, however, that the lack of long-term records of snow accumulation in this sector of the Andes makes it difficult to reach definitive results.
Previous studies show an increasing trend of the snow accumulation over the high latitudes in a warmer climate (e.g., Thomas et al., 2017; Medley and Thomas, 2019). These changes are mainly confined to regional scales and determined by thermodynamical (e.g., moisture-holding capacity of air) and dynamical (e.g., wind speed) responses under warmer climatic conditions. Indeed, the increase in snow accumulation determined in the current work occurs under a warming trend. The fields of the air temperature from the RegCM4.6, which are used to calculate the snow accumulation, show positive trends, especially in autumn and winter (Figure S6) when larger increases in snow accumulation are determined. However, rather than the regional scale, the positive trend in snow accumulation detected in this study and previous ones is limited to the local scale, mainly concentrated at elevations over ~1,000 m a.s.l on the west side of both icefields. Lower elevation terrain shows no trend and even a negative trend in snow accumulation on the eastern side of the icefields.
The spatial differences in snow accumulation trend could be associated with the location of the study area since it corresponds to the borders where a shift in the position of the zonal winds has been observed (Gupta and England, 2006). Indeed, an increase in the total precipitation of ~200 mm decade−1 was observed south of 50°S for the period 1961–2000 (Garreaud et al., 2013). However, Garreaud et al. (2013) also indicated a negative trend in the west Andean flank lying north of 50°S and recently, Boisier et al. (2019) also indicated a negative trend in the total precipitation based on local observations during the period 1960–2016 on the eastern side of the NPI and in northern Patagonia. These regional differences are associated with spatial differences in the tendency of the westerlies, since weaker and stronger westerlies have been detected at mid-latitudes (~45°S) and around 60°S, respectively. This opposite trend in westerlies has been linked to the positive phase of the SAM. During this phase, a low pressure anomaly over Antarctica extends to ~55°S with an out of phase circumpolar positive anomaly centered at 45°S. This pressure difference is associated with a zonal geostrophic wind anomaly that is positive south of 45°S and negative to the north (Gupta and England, 2006). The positive phase of SAM has also been associated with warming sea surface temperatures in the western Pacific (Thomas et al., 2017). Hence, this is an area of transition where different temporal and spatial impacts are expected to exist. In fact, the positive trends in snow accumulation, especially in autumn and winter, tend to be larger toward the south (~50°S), suggesting some influence of the dominant positive phase of SAM in the last decades (Marshall, 2003).
Spatial differences in snow dynamics are also evident in snow persistence (fraction of time that snow is present on the ground). This area is a transition zone between a dominant decrease (north of 46°) and dominant increase (south of 50°S) of snow persistence (Hammond et al., 2018). In the west-east direction, SAM also influences the snow accumulation trends as the precipitation at the regional scale is positively or negatively correlated with a zonal wind component of 850 hPa in the western or eastern sectors of the Andes, respectively, due to the mechanical effect of the Andes (Garreaud et al., 2013). This effect forces air masses to ascend in the western sector (windward), favoring conditions for saturation and hence the occurrence of precipitation; on the other hand, it results in subsidence and inhibition of the precipitation in the eastern sector (leeward) (Garreaud et al., 2013). Hence, an increase in the westerly winds and moisture content associated with a dominant positive phase of SAM as well as windward precipitation enhancement (and leeward inhibition) due to orographic effects could explain the snow accumulation increase over 1,000 m a.s.l. on the western side and the reduction on the east side in the context of climate warming (Rasmussen et al., 2007; Aguirre et al., 2018; Olivares-Contreras et al., 2018).
Implications for Glacier Mass Balance
The three methods previously used to define the snow accumulation in the icefields show some important differences in determining the absolute values. The difference in values between the 2C method and the WE-SC methods could reach as high as ~3,000 mm w.e. The differences are even higher when comparing the 2C method with the DI method. Therefore, the use of different schemes to derive the snow/rain ratio may lead surface mass balance modeling efforts to differ considerably (Weidemann et al., 2018). This could partly explain the differences between the positive modeling mass balance (e.g., Schaefer et al., 2013, 2015; Mernild et al., 2016b; Weidemann et al., 2018) and the negative geodetic mass balance (e.g., Dussaillant et al., 2018; Malz et al., 2018) that have been documented in the literature.
Another issue to consider is if the sleet precipitation type should be considered as surface accumulation since the differences between the 2C and DI (snow plus sleet) methods are reduced considerably, especially on the western side of both icefields. Sleet is defined as a mixture of snow and rain and is associated with refreezing before reaching the ground, which forms an ice core surrounded by liquid water (Whiteman, 2000). Sleet particles are usually formed near the freezing point, where both rain and snow particles coexist (Ostrometzky et al., 2015). In this sense, it is probably valid that an undetermined percentage of sleet could be considered as surface accumulation (the ice core), while the liquid section percolates into the glacier and cannot therefore be considered as surface accumulation. It is beyond the aim of this work to define this percentage, but it seems that an accumulation in the range between the 2C and DI (snow plus sleet) and DI (snow) is a more realistic estimation. The SC and WE methods are in this range and are the closest to the accumulation estimated with the UDG observations. Other types of accumulation have been described in this zone, such as rime accretion due to supercooled water droplets transport by the strong winds (Whiteman and Garibotti, 2013); nevertheless, quantification and implications for surface mass balance have not been studied in detail to date.
At long-term scale, the increase in snow accumulation during winter months has been discussed as the cause of glacier mass balance stability and even glacier expansion in the Karakoram region (the pattern that has been termed the Karakoram anomaly; Kapnick et al., 2014). To explore the long-term glacier response, we compare annual snow accumulation trends from this work and results from geodetic mass balance at glacier scale during the period 2000–2014/2015. Elevation change data for the SPI covers the period from 2000 to 2015 (Malz et al., 2018), whereas it covers the period from 2000 to 2014 for the NPI (Braun et al., 2019). Overall, at the glacier scale, elevation change data seem to suggest that the positive values correspond to the glaciers with accumulation area ratio (AAR) over 0.8 and classified as marine-terminating glaciers according to the Randolph Glacier Inventory (RGI 6.0; RGI Consortium, 2017). According to the data in this work, these glaciers also showed a larger annual positive trend in snow accumulation in the period 2000–2015 (Figure 9). Some exceptions exist such as Jorge Montt and HPS 12 glaciers, which besides being marine terminating glaciers, show mostly negative rates of elevation change (Malz et al., 2018). The other exception is the San Rafael Glacier which besides having a large AAR (0.85, Rivera et al., 2007) and being a marine-terminating glacier, shows a negative rate of elevation change, however the snow accumulation trend is less than those of glaciers located in the west side of the SPI. Glaciers with these characteristics (marine terminating, AAR over 0.8 and high positive snow accumulation trends) also showed positive or stable frontal position rates in the period 2000–2010/2011 (Sakakibara and Sugiyama, 2014). Furthermore, they also indicate the highest observed ice velocity in the period 1984–2014 (Mouginot and Rignot, 2015).
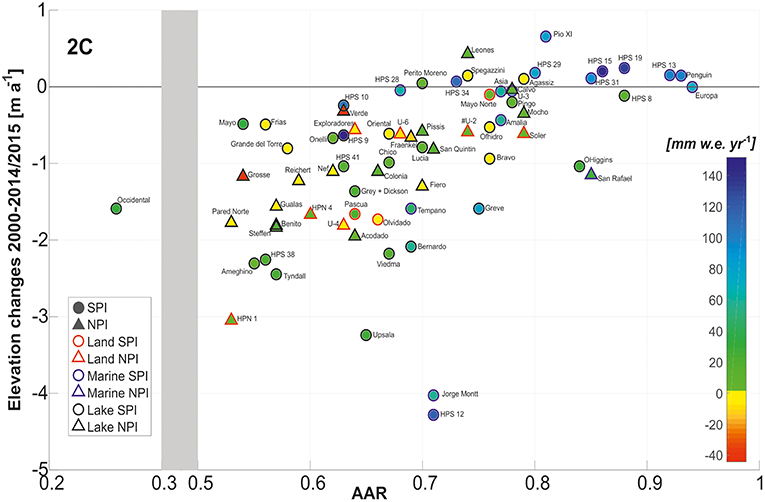
Figure 9. Observed elevation changes in the period 2000–2014/2015 for both icefields vs. the accumulation-area ratio (AAR). The color represents the snow accumulation trends by glaciers using the 2C as PPM. Terminus type is also indicated depending the color of the contour for each symbol, triangle for the SPI, and circle for the NPI (see legend). Note that the x-axis is broken. Due to space issues some glaciers names are not indicated.
Although previous studies suggest that elevation changes are dominated by ice dynamics (Mouginot and Rignot, 2015), the data in our work suggest a climate forcing in glaciers where positive elevation changes and stable/positive frontal changes were observed. The Pío XI Glacier, for example, is known for its large cumulative advance since 1945 (Wilson et al., 2016), and it has a large AAR (~0.8; Rivera and Casassa, 1999) as well as one of the highest rates of snow accumulation (and with a positive trend) according to our observations. Most of the other glaciers, where positive elevation changes have been observed, also show significant positive trends in snow accumulation. The exceptions of the Jorge Montt and HPS12 glaciers with positive snow accumulation trends and negative surface elevation changes, may then be explained by ice dynamics (Mouginot and Rignot, 2015), in which ice thinning is a result of the drawdown of the plateaus rather than a decrease in snow accumulation. Other observed processes in the icefields such as an increase in supraglacial debris-cover area (Glasser et al., 2016) or in glacial lake area (Loriaux and Casassa, 2013) could also play a role in the surface glacier mass balance of these glaciers through different feedback mechanisms, and they must be considered in elucidating the present and future evolution of these glaciers.
Conclusions
In this work, we have assessed both spatial and temporal patterns in snow accumulation in both the North Patagonia Icefield and the South Patagonia Icefield. We used a regional climate model, RegCM4.6, short-term snow accumulation observations using ultrasonic depth gauges (UDG) and previous snow accumulation estimations derived from ice core data, stake observations and modeling approaches. Snow accumulation derived using the RegCM4.6 and the four Phase Partitioning Methods (PPMs) replicated the snow accumulation gradient that is expected to exist in this area. Snow accumulation rates are higher on the west side relative to the east side for both icefields. A maximum amount of snow accumulation is determined at around 50°S on the west side of the SPI. The values depend on the PPM used and reach a maximum mean difference of 1,504 mm w.e. between 2C and DI methods, with some areas reaching differences higher than 3,500 mm w.e. These differences could lead to divergent mass balance estimations, depending on the scheme used, so we suggest that future works should adopt a multi-scheme parameterization to define the snow accumulation.
There are important differences between rates of snow accumulation determined in this work and previous estimations using ice core data at an annual scale. These differences could be related to the scale disparity between the datasets, with each grid point of the model representing an area of ~100 km2, while ice core data are derived at the point-scale. Wind transport, with areas where erosion is dominant (e.g., Pío XI glacier ice core site), and areas where the deposition is dominant (e.g., Tyndall glacier ice core site), also seem to be reasonable explanations for these differences. However, good agreement is found when comparing UDG observations with modeled data in the plateau area of the SPI over a short time period.
Snow accumulation trends are mostly positive on the plateau on the west side of both icefields. In the SPI, significant positive trends are mainly present in the autumn season. For the rest of the area, and during other seasons, no significant changes can be determined, except on the east side between 48.5° and 50.5°S, where zones with significant negative trends in autumn were observed. Over annual timescales, glaciers previously observed to be exhibiting positive and stable elevation and frontal changes coincide with areas that show snow accumulation increases according to this study. This suggests that the increase in snow accumulation attenuates the response of the glaciers in a context of overall glacier retreat due to climate warming in Patagonia. The interplay with other factors such as the glacier terminus type and accumulation-area ratio also seems to explain some of the glacier response (e.g. De Angelis, 2014), as for example the glacier advance observed in the Pío XI glacier.
Robustly validated climate model data therefore appear to be useful for exploring the relationship and spatial differences in glacier response to present and future climate change, especially in Patagonia where meltwater makes a strong contribution to sea level rise and where only a limited number of observational in situ efforts exist.
Data Availability
The datasets generated for this study are available on request to the corresponding author.
Author Contributions
CB, DJQ, and ANR designed the study. CB analyzed the data and prepared the first draft of the paper. DB performed the RegCM4.6 simulations in addition to data processing and contributed to the writing. ÁG-R and CB performed the statistical analysis and analyzed the results. DF-B compiled and analyzed glaciological data. DJQ, ANR, and MR oversaw the research, and reviewed the manuscript. All authors discussed the results and reviewed the manuscript.
Conflict of Interest Statement
The authors declare that the research was conducted in the absence of any commercial or financial relationships that could be construed as a potential conflict of interest.
Acknowledgments
We acknowledge the Dirección General de Aguas de Chile (DGA) for providing their data for analysis. The Centro de Estudios Científicos (CECs) installed the GBL stations and provided all the metadata of these stations. CB and DF-B acknowledge support from the National Committee of Sciences and Technology (CONICYT) Programa Becas de Doctorado en el Extranjero, Beca Chile for the doctoral scholarship. ÁG-R acknowledges CONICYT Doctorado Nacional 2016-21160642 for the doctoral scholarship.
Supplementary Material
The Supplementary Material for this article can be found online at: https://www.frontiersin.org/articles/10.3389/fenvs.2019.00030/full#supplementary-material
References
Abram, N. J., Mulvaney, R., Vimeux, F., Phipps, S. J., Turner, J., and England, M. H. (2014). Evolution of the southern annular mode during the past millennium. Nat. Clim. Change 7, 564–569. doi: 10.1038/nclimate2235
Aguirre, F., Carrasco, J., Sauter, T., Schneider, C. H., Gaete, K., Garín, E., et al. (2018). Snow cover change as a climate indicator in Brunswick Peninsula, Patagonia. Front. Earth Sci. 6:8. doi: 10.3389/feart.2018.00130
Aniya, M., Sato, H., Naruse, R., Skvarca, P., and Casassa, G. (1996). The use of satellite and airborne imagery to inventory outlet glaciers of the Southern Patagonia Icefield, South America. Photogramm. Eng. Rem. S. 62, 1361–1369.
Anthes, R. A. (1977). A cumulus parameterization scheme utilizing a one-dimensional cloud model. Mon. Wea. Rev. 105, 270–286. doi: 10.1175/1520-0493(1977)105<0270:ACPSUA>2.0.CO;2
Aristarain, A., and Delmas, R. (1993). Firn-core study from the southern Patagonia ice cap, South America. J. Glaciol. 39, 249–254. doi: 10.1017/S0022143000015914
Beck, H. E., Zimmermann, N. E., McVicar, T. R., Vergopolan, N., Berg, A., and Wood, E. F. (2018). Present and future Köppen-Geiger climate classification maps at 1-km resolution. Sci. Data. 5:180214 doi: 10.1038/sdata.2018.214
Behrangi, A., Yin, X., Rajagopal, S., Stampoulis, D., and Ye, H. (2018). On distinguishing snowfall from rainfall using near-surface atmospheric information: comparative analysis, uncertainties, and hydrologic importance. Quart. J. Roy. Meteor. Soc. 144, 89–102. doi: 10.1002/qj.3240
Boisier, J. P., Alvarez-Garreton, C. R., Cordero, R., Damiani, A., Gallardo, L., Garreaud, R., et al. (2019). Anthropogenic drying in central-southern Chile evidenced by long term observations and climate model simulations. Elem Sci. Anth. 6:74. doi: 10.1525/elementa.328
Bolton, D. (1980). The computation of equivalent potential temperature. Mon. Weather Rev. 108, 1046–1053. doi: 10.1175/1520-0493(1980)108<1046:TCOEPT>2.0.CO;2
Bozkurt, D., Rondanelli, R., Garreaud, R., and Arriagada, A. (2016). Impact of warmer eastern tropical Pacific SST on the March 2015 Atacama floods. Mon. Wea. Rev. 144, 4441–4460. doi: 10.1175/MWR-D-16-0041.1
Bozkurt, D., Rondanelli, R., Marín, J., and Garreaud, R. (2018). Foehn event triggered by an atmospheric river underlies record-setting temperature along continental Antarctica. J. Geophys. Res. Atmospheres 128, 3871–3892. doi: 10.1002/2017JD027796
Braun, M. H., Malz, P., Sommer, C., Farías-Barahona, D., Sauter, T., Casassa, G., et al. (2019). Constraining glacier elevation and mass changes in South America. Nat. Clim. Change 9, 130–136. doi: 10.1038/s41558-018-0375-7
Carrasco, J., Casassa, G., and Rivera, A. (2002). “Meteorological and climatological aspects of the Southern Patagonia Icefields,” in The Patagonian Icefields. A Unique Natural Laboratory for Environmental and Climate Change Studies, eds G. Casassa, F. Sepulveda, and R. Sinclair (New York, NY: Kluwer Academic; Plenum Publishers), 29–41. doi: 10.1007/978-1-4615-0645-4_4
CECs-DGA (2016). Línea de Base Glaciológica del Sector Norte de Campo de Hielo Sur: Glaciares Jorge Montt, Témpano y O'Higgins. SIT N° 404, DGA, Technical Report in Spanish.
Cook, K., Yang, X., Carter, C., and Belcher, B. (2003). A modelling system for studying climate controls on mountain glaciers with application to the Patagonian Icefields. Clim. Change 56, 339–367. doi: 10.1023/A:1021772504938
Davies, B. J., and Glasser, N. F. (2012). Accelerating shrinkage of Patagonian glaciers from the little ice Age (~AD 1870) to 2011. J. Glaciol. 58, 1063–1084. doi: 10.3189/2012JoG12J026
De Angelis, H. (2014), Hypsometry and sensitivity of the mass balance to change in equilibrium-line altitude: the case of the Southern Patagonia Icefield. J. Glaciol. 60, 14–28. doi: 10.3189/2014JoG13J127.
De Angelis, H., Rau, F., and Skvarca, P. (2007). Snow zonation on Hielo Patagonico Sur, Southern Patagonia, derived from Landsat 5 TM data. Global Planet. Change 59, 149–158. doi: 10.1016/j.gloplacha.2006.11.032
Dickinson, R. E., Henderson-Sellers, A., and Kennedy, P. J. (1993). Biosphere-Atmosphere Transfer Scheme (BATS) Version 1e as Coupled to the NCAR Community Climate Model. NCAR Tech. Note NCAR/TN-387+STR, NCAR, Boulder.
Ding, B., Yang, K., Qin, J., Wang, L., Chen, Y., and He, X. (2014). The dependence of precipitation types on surface elevation and meteorological conditions and its parameterization. J. Hydrol. 513,154–163. doi: 10.1016/j.jhydrol.2014.03.038
Dussaillant, I., Berthier, E., and Brun, F. (2018). Geodetic mass balance of the Northern Patagonian Icefield from 2000 to 2012 using two independent methods. Front. Earth Sci. 6:8. doi: 10.3389/feart.2018.00008
Emanuel, K. A. (1991). A scheme for representing cumulus convection in large-scale models. J. Atmos. Sci. 48, 2313–2335. doi: 10.1175/1520-0469(1991)048<2313:ASFRCC>2.0.CO;2
Foresta, L., Gourmelen, N., Weissgerber, F., Nienow, P., Williams, J. J., Shepherd, A., et al. (2018). Heterogeneous and rapid ice loss over the Patagonian Ice Fields revealed by CryoSat-2 swath radar altimetry. Remote Sens. Environ. 211, 441–455. doi: 10.1016/j.rse.2018.03.041
Fritsch, J. M., and Chappell, C. F. (1980). Numerical prediction of convectively driven mesoscale pressure systems. Convective parameterization. J. Geophys. Res. 37, 1722–1733. doi: 10.1175/1520-0469(1980)037<1722:NPOCDM>2.0.CO;2
Gardner, A. S., Moholdt, G., Cogley, J. G., Wouters, B., Arendt, A. A., Wahr, J., et al. (2013). A reconciled estimate of glacier contributions to sea level rise: 2003 to 2009. Science 340, 852–857. doi: 10.1126/science.1234532
Garreaud, R., Lopez, P., Minvielle, M., and Rojas, M. (2013). Large-scale control on the patagonian climate. J. Clim. 26, 215–230. doi: 10.1175/JCLI-D-12-00001.1
Gillett, N. P., Kell, T. D., and Jones, P. D. (2006). Regional climate impacts of the Southern Annular Mode. Geophys. Res. Lett. 33:L23704. doi: 10.1029/2006GL027721
Giorgi, F., Coppola, E., Solmon, F., Mariotti, L., Sylla, M. B., Bi, X., et al. (2012). RegCM4: model description and preliminary tests over multiple CORDEX domains. Clim. Res. 52, 7– 29. doi: 10.3354/cr01018
Giorgi, F., Marinucci, M. R., and Bates, G. T. (1993a). Development of a second generation regional climate model (RegCM2). Part I: Boundary layer and radiative transfer processes. Mon. Wea. Rev. 121, 2794–2813. doi: 10.1175/1520-0493(1993)121<2794:DOASGR>2.0.CO;2
Giorgi, F., Marinucci, M. R., Bates, G. T., and DeCanio, G. (1993b). Development of a second generation regional climate model (RegCM2). Part II: convective processes and assimilation of lateral boundary conditions. Mon. Wea. Rev. 121, 2814–2832. doi: 10.1175/1520-0493(1993)121<2814:DOASGR>2.0.CO;2
Giorgi, F., Torma, C., Coppola, E., Ban, N., Schar, C., and Somot, S. (2016). Enhanced summer convective rainfall at Alpine high elevations in response to climate warming. Nat. Geosci. 9, 584–589. doi: 10.1038/ngeo2761
Glasser, N. F., Holt, T. O., Enas, Z. D., Davies, B. J., Pelto, M., and Harrison, S. (2016). Recent spatial and temporal variations in debris cover on Patagonian glaciers. Geomorphology 273, 202–216. doi: 10.1016/j.geomorph.2016.07.036
Gourlet, P., Rignot, E., Rivera, A., and Casassa, G. (2016). Ice thickness of the northern half of the Patagonia Icefields of South America from high-resolution airborne gravity surveys. Geophys. Res. Lett. 43, 241–249. doi: 10.1002/2015GL066728
Grassi, B., Redaelli, G., and Visconti, G. (2013). Arctic sea ice reduction and extreme climate events over the Mediterranean region. J. Clim. 26, 10101–10110. doi: 10.1175/JCLI-D-12-00697.1
Grell, G. A. (1993). Prognostic evaluation of assumptions used by cumulus parameterizations. Mon. Wea. Rev. 121, 764–787. doi: 10.1175/1520-0493(1993)121<0764:PEOAUB>2.0.CO;2
Grell, G. A., Dudhia, J., and Stauffer, D. R. (1994). Description of the fifth generation Penn State/NCAR Mesoscale Model (MM5). NCAR Tech. Note NCAR/TN-398+STR, NCAR, Boulder.
Gupta, A. S., and England, M. H. (2006). Coupled ocean–atmosphere–ice response to variations in the Southern Annular Mode. J. Clim. 19, 4457–4486. doi: 10.1175/JCLI3843.1
Hall, A., and Visbeck, M. (2002). Synchronous variability in the southern hemisphere atmosphere, sea ice, and ocean resulting from the annular mode. J. Clim. 15, 3043–3057. doi: 10.1175/1520-0442(2002)015<3043:SVITSH>2.0.CO;2
Hammond, J. C., Saavedra, F. A., and Kampf, S. K. (2018). Global snow zone maps and trends in snow persistence 2001–2016. Int. J. Climatol. 38, 4369–4383. doi: 10.1002/joc.5674
Harpold, A. A., Kaplan, M. L., Klos, P. Z., Link, T., McNamara, J. P., Rajagopal, S., et al. (2017). Rain or snow: hydrologic processes, observations, prediction, and research needs. Hydrol. Earth Syst. Sci. 21, 1–22. doi: 10.5194/hess-21-1-2017
Hedstrom, N. R., and Pomeroy, J. W. (1998). Measurements and modeling of snow interception in the boreal forest. Hydrol. Process. 12, 1611–1625. doi: 10.1002/(SICI)1099-1085(199808/09)12:10/11<1611::AID-HYP684>3.0.CO;2-4
Holtslag, A. A. M., de Bruijn, E. I. F., and Pan, H. L. (1990). A high resolution air mass transformation model for short-range weather forecasting. Mon. Wea. Rev. 118, 1561–1575. doi: 10.1175/1520-0493(1990)118<1561:AHRAMT>2.0.CO;2
Kapnick, S. B. T. L, Delworth, T. L., Ashfaq, M., Malyshev, S., and Milly, P. C. D. (2014). Snowfall less sensitive to warming in Karakoram than in Himalayas due to a unique seasonal cycle. Nat. Geosci. 7, 834–840. doi: 10.1038/ngeo2269
Kiehl, J. T., Hack, J. J., Bonan, G. B., Boville, B. A., Breigleb, B. P., Williamson, D., et al. (1996). Description of the NCAR Community Climate Model (CCM3). NCAR Tech. Note NCAR/TN-420+STR, NCAR, Boulder.
Kohshima, S., Takeuchi, N., Uetake, J., Shiraiwa, T., Uemura, R., Yoshida, N., et al. (2007). Estimation of net accumulation rate at a Patagonian glacier by ice core analyses using snow algae. Global Planet. Change 59, 236–244. doi: 10.1016/j.gloplacha.2006.11.014
Koppes, M., Conway, H., Rasmussen, L. A., and Chernos, M. (2011). Deriving mass balance variations and calving variations from reanalysis data and sparse observations, Glaciar San Rafael, northern Patagonia, 1950-2005. Cryosphere 5, 791–808. doi: 10.5194/tc-5-791-2011
Krogh, S., Pomeroy, J., and McPhee, J. (2015). Physically based mountain hydrological modeling using reanalysis data in Patagonia. J. Hydrometeorol. 16, 172–193. doi: 10.1175/JHM-D-13-0178.1
Lenaerts, J. T. M., van den Broeke, M. R., van Wessem, J. M., van de Berg, W. J., van Meijgaard, E., van Ulft, L., et al. (2014). Extreme precipitations and climate gradients in patagonia revealed by high-resolution regional atmospheric climate modeling. J. Clim. 27, 4607–4621. doi: 10.1175/JCLI-D-13-00579.1
Lopez, P., Sirguey, P., Arnaud, Y., Pouyaud, B., and Chevallier, P. (2008). Snow cover monitoring in the Northern Patagonia Icefield using MODIS satellite images (2000–2006). Global Planet. Change 61, 103–116. doi: 10.1016/j.gloplacha.2007.07.005
Loriaux, T., and Casassa, G. (2013). Evolution of glacial lakes from the Northern Patagonia Icefield and terrestrial water storage in a sea-level rise context. Global Planet. Change 102, 33–40. doi: 10.1016/j.gloplacha.2012.12.012
Malz, P., Meier, W., Casassa, G., Ja-a, R., Skvarca, P., and Braun, M. H. (2018). Elevation and mass changes of the southern patagonia icefield derived from TanDEM-X and SRTM Data. Remote Sens. 10:188. doi: 10.3390/rs10020188
Marshall, G. (2003). Trends in the southern annular mode from observations and reanalyses. J. Clim. 16, 4134–4143. doi: 10.1175/1520-0442(2003)016<4134:TITSAM>2.0.CO;2
Masiokas, M., Rivera, A., Espizúa, L., Villalba, R., Delgado, S., and Aravena, J. C. (2009). Glacier fluctuations in extratropical South America during the past 1000 years. Palaeogeogr. Palaeoec. 281, 242–268. doi: 10.1016/j.palaeo.2009.08.006
Matsuoka, K., and Naruse, R. (1999). Mass balance features derived from a firn core at Hielo Patagonico Norte, South America. Arct. Antarct. Alp. Res. 31, 333–340.
Medley, B., and Thomas, E. R. (2019). Increased snowfall over the Antarctic Ice Sheet mitigated 20th century sea-level rise. Nat. Clim. Change 9, 34–39. doi: 10.1038/s41558-018-0356-x
Meier, W. J. H., Grießinger, J., Hochreuther, P., and Braun, M. H. (2018). An updated multi-temporal glacier inventory for the Patagonian Andes with changes between the little ice age and 2016. Front. Earth Sci. 6:8. doi: 10.3389/feart.2018.00062
Mernild, S. H., Liston, G. E., Hiemstra, C. A., Malmros, J. K., and McPhee, J. (2016a). The andes cordillera. part i: snow distribution, properties, and trends (1979–2014). Int. J. Climatol. 37, 1680–1980. doi: 10.1002/joc.4804
Mernild, S. H., Liston, G. E., Hiemstra, C. A., and Wilson, R. (2016b). The andes cordillera. part III: glacier surface mass balance and contribution to sea level rise (1979–2014). Int. J. Climatol. 37, 3154–3174. doi: 10.1002/joc.4907
Möller, M., Schneider, C., and Kilian, R. (2007). Glacier change and climate forcing in recent decades at Gran Campo Nevado, southernmost Patagonia. Ann. Glaciol. 46, 136–144. doi: 10.3189/172756407782871530
Mouginot, J., and Rignot, E. (2015). Ice motion of the patagonian icefields of South America: 1984–2014. Geophys. Res. Lett. 42, 1441–1449. doi: 10.1002/2014GL062661
Olivares-Contreras, V. A., Mattar, C., Gutierrez, A. G., and Jimenex, J. C. (2018). Warming trends in Patagonian subantartic forest. In. J. Appl. Earth Obs. Geoinform. 76, 51–65. doi: 10.1016/j.jag.2018.10.015
Ostrometzky, J., Cherkassky, D., and Messer, H. (2015). Accumulated mixed precipitation estimation using measurements from multiple microwave links. Adv. Meteorol. 2015:707646. doi: 10.1155/2015/707646
Pal, J. S., Small, E. E., and Eltahir, E. A. B. (2000). Simulation of regional-scale water and energy budgets: Representation of surged cloud and precipitation processes within RegCM. J. Geophys. Res. 105, 29579–29594. doi: 10.1029/2000JD900415
Pellicciotti, F., Ragettli, S., Carenzo, M., and McPhee, J. (2014). Changes of glaciers in the Andes of Chile and priorities for future work. Sci. Total Environ. 493, 1197–1210. doi: 10.1016/j.scitotenv.2013.10.055
Pérez, T., Mattar, C., and Fuster, R. (2018). Decrease in snow cover over the Aysén River catchment in Patagonia, Chile. Water 10:619. doi: 10.3390/w10050619
Rasmussen, L. A., Conway, H., and Raymond, C. F. (2007). Influence of upper air conditions on the Patagonia icefields. Global Planet. Change 59, 203–216. doi: 10.1016/j.gloplacha.2006.11.025
RGI Consortium (2017). Randolph Glacier Inventory – A Dataset of Global Glacier Outlines: Version 6.0: Technical Report, Global Land Ice Measurements From Space. Digital Media.
Rignot, E., Rivera, A., and Casassa, G. (2003). Contribution of the patagonia icefields of South America to sea level rise. Science 302, 434–436, 2003. doi: 10.1126/science.1087393
Rivera, A. (2004). Mass Balance Investigations at Glaciar Chico, Southern Patagonia. Doctoral dissertation, University of Bristol, Icefield, Chile.
Rivera, A., Benham, T., Casassa, G., Bamber, J., and Dowdeswell, J. A. (2007). Ice elevation and areal changes of glaciers from the Northern Patagonia icefield, Chile. Global Planet. Change 59, 126–137. doi: 10.1016/j.gloplacha.2006.11.037
Rivera, A., and Casassa, G. (1999). Volume changes on Glaciar Pío XI, Patagonia: 1975-1995. Global Planet. Change 22, 233–244. doi: 10.1016/S0921-8181(99)00040-5
Rivera, A., and Casassa, G. (2002). “Ice thickness measurements on the Southern Patagonia Icefield,” in The Patagonian Icefields. A Unique Natural Laboratory for Environmental and Climate Change Studies, eds G. Casassa, F. Sepulveda, and R. Sinclair (New York, NY: Kluwer Academic; Plenum Publishers), 101–115. doi: 10.1007/978-1-4615-0645-4_10
Rojas-Zamorano, C., Maier, E., Arigony-Neto, J., de Almeida Espinoza, J. M., Jaa, R., and Gonzalez, I. (2017). Snow coverage mapping in Darwin Mountain Ranges through satellite images optical and its relationship with data climate (2005–2016) (in Portuguese). Rev. Brasil. Cartogr. 69, 743–758.
Sagredo, E., and Lowell, T. (2012), Climatology of Andean glaciers: a framework to understand glacier response to climate change. Global Planet Change 86–87, 101–109. doi: 10.1016/j.gloplacha.2012.02.010
Sakakibara, D., and Sugiyama, S. (2014). Ice-front variations and speed changes of calving glaciers in the Southern Patagonia Icefield from 1984 to 2011. J. Geophys. Res. Earth 119, 2541–2554. doi: 10.1002/2014JF003148
Salmi, T., Maatta, A., Anttila, P., Ruoho-Airola, T., and Amnell, T. (2002). Detecting Trends of Annual Values of Atmospheric Pollutants by the Mann-Kendall Test and Sen's Slope Estimates—The Excel Template Application Makesens. Helsinki: Finnish Meteorological Institute Publications on Air Quality No. 31.
Schaefer, M., Machguth, H., Falvey, M., and Casassa, G. (2013). Modeling past and future surface mass balance of the Northern Patagonian Icefield. J. Geophys. Res. Earth 118, 571–588. doi: 10.1002/jgrf.20038
Schaefer, M., Machguth, H., Falvey, M., Casassa, G., and Rignot, E. (2015). Quantifying mass balance processes on the Southern Patagonia Icefield. Cryosphere 9, 25–35. doi: 10.5194/tc-9-25-2015
Schneider, C., Glaser, M., Killian, R., Santana, A., Butorovic, N., and Casassa, G. (2003). Weather observations across the Southern Andes at 53°S. Phys. Geogr. 24, 97–119. doi: 10.2747/0272-3646.24.2.97
Schwikowski, M., Schläppi, M., Santiba-ez, P., Rivera, A., and Casassa, G. (2013). Net accumulation rates derived from ice core stable isotope records of Pío XI glacier, Southern Patagonia Icefield. Cryosphere 7, 1635–1644. doi: 10.5194/tc-7-1635-2013
Shiraiwa, T., Kohshima, S., Uemura, R., Yoshida, N., Matoba, S., Uetake, J., et al. (2002). High net accumulation rates at Campo de Hielo Patagonico Sur, South America, revealed by analysis of a 45.97 m long ice core. Ann. Glaciol. 35, 84–90. doi: 10.3189/172756402781816942
Smith, R., and Evans, J. (2007). Orographic precipitation and water vapor fractionation over the Southern Andes. J. Hydrometeorol. 8, 3–19. doi: 10.1175/JHM555.1
Thomas, E. R., van Wessem, J. M., Roberts, J., Isaksson, E., Schlosser, E., Fudge, T. J., et al. (2017). Regional Antarctic snow accumulation over the past 1000 years. Clim. Past 13, 1491–1513. doi: 10.5194/cp-13-1491-2017
Villarroel, C., Carrasco, J., Casassa, G., and Falvey, M. (2013). Modeling near-surface air temperature and precipitation using WRF with 5-km resolution in the Northern Patagonia Icefield: a pilot simulation. Int. J. Geosci. 4, 1193–1199. doi: 10.4236/ijg.2013.48113
Weidemann, S., Sauter, T., Schneider, L., and Schneider, C. (2013). Impact of two conceptual precipitation downscaling schemes on mass-balance modeling of gran campo nevado ice cap, patagonia. J. Glaciol. 59, 1106–1116. doi: 10.3189/2013JoG13J046
Weidemann, S. S., Sauter, T., Malz, P., Ja-a, R., Arigony-Neto, J., Casassa, G., et al. (2018). Glacier mass changes of lake-terminating grey and tyndall glaciers at the southern patagonia icefield derived from geodetic observations and energy and mass balance modeling. Front. Earth Sci. 6:81. doi: 10.3389/feart.2018.00081
White, A., and Copland, L. (2015). Decadal-scale variations in glacier area changes across the Southern Patagonian Icefield since the 1970s. Arct. Antarct. Alp. Res. 47, 147–167. doi: 10.1657/AAAR0013-102
Whiteman, C. D., and Garibotti, R. (2013). Rime mushrooms on mountains: description, formation, and impacts on mountaineering. Bull. Amer. Meteor. Soc. 94, 1319–1327. doi: 10.1175/BAMS-D-12-00167.1
Willis, M., Melkonian, A., Pritchard, M., and Rivera, A. (2012). Ice loss from the Southern Patagonian Ice Field, South America, between 2000 and 2012. Geophys. Res. Lett. 39:L17501. doi: 10.1029/2012GL053136
Wilson, R., Carrión, D., and Rivera, A. (2016). Detailed dynamic, geometric and supraglacial moraine data for Glaciar Pío XI, the only surge-type glacier of the Southern Patagonia Icefield. Ann. Glaciol. 57,119–130. doi: 10.1017/aog.2016.32
Yamada, T. (1987). Glaciological characteristics revealed by 37.6-m deep core drilled at the accumulation area of San Rafael Glacier, the Northern Patagonia Icefield. Bull. Glac. Res. 4, 59–67.
Keywords: snow accumulation, patagonian icefields, snow trends, glacier mass-balance, regional climate model
Citation: Bravo C, Bozkurt D, Gonzalez-Reyes Á, Quincey DJ, Ross AN, Farías-Barahona D and Rojas M (2019) Assessing Snow Accumulation Patterns and Changes on the Patagonian Icefields. Front. Environ. Sci. 7:30. doi: 10.3389/fenvs.2019.00030
Received: 30 November 2018; Accepted: 20 February 2019;
Published: 19 March 2019.
Edited by:
Ricardo Villalba, Consejo Nacional de Investigaciones Científicas y Técnicas (CONICET), ArgentinaReviewed by:
Jorge Carrasco, University of Magallanes, ChileChenghai Wang, Lanzhou University, China
Copyright © 2019 Bravo, Bozkurt, Gonzalez-Reyes, Quincey, Ross, Farías-Barahona and Rojas. This is an open-access article distributed under the terms of the Creative Commons Attribution License (CC BY). The use, distribution or reproduction in other forums is permitted, provided the original author(s) and the copyright owner(s) are credited and that the original publication in this journal is cited, in accordance with accepted academic practice. No use, distribution or reproduction is permitted which does not comply with these terms.
*Correspondence: Claudio Bravo, Z3ljYWJsQGxlZWRzLmFjLnVr