- 1Environmental Science, Policy and Management Department, University of California, Berkeley, Berkeley, CA, United States
- 2Department of History, Simon Fraser University, Burnaby, BC, Canada
- 3School of Information, University of California, Berkeley, Berkeley, CA, United States
- 4History, Geography, and Environmental Studies, University of California, Santa Barbara, Santa Barbara, CA, United States
- 5Statewide Program in Informatics and GIS, Division of Agriculture and Natural Resources, University of California, Oakland, Oakland, CA, United States
Historical data play an important role in our understanding of environmental change and ecosystem dynamics. By lengthening the temporal scale of scientific inquiry, historical data reveal insights into the dynamic nature of ecosystems. However, most historical data has yet to make a full contribution, remaining “dark” and out of reach to the broader scientific community. This article responds to several calls stressing the importance of empirical historical materials and urges their preservation and accessibility. Despite the importance of historical data collections, few standards have emerged to integrate historical dark data into the larger digital data landscape. To encourage greater use of historical data across scientific disciplines it is vital to make data findable, accessible, interoperable, and reusable (e.g., the FAIR principles). In this paper we discuss the potential of historical dark data to contribute to the modern digital ecological data landscape. We do this by focusing on three cases from the University of California field and research stations and the groups that have worked to make historical dark data discoverable. Despite the common goal of maximizing the potential use of these data collections, each case and the methods employed are unique, and showcase varying levels of success in achieving the FAIR principles and shepherding historical data into the twenty-first century.
Introduction
Scientific research increasingly highlights large datasets for their transformative potential in solving enduring and complex problems, leading one recent analysis to declare data the “world's most valuable resource” (Hampton et al., 2013; Fosso Wamba et al., 2015; The Economist, 2017). Large “born digital” data from modern data streams have increased the scope of environmental inquiry in recent decades, advances in computing, databases, sensing technologies, cloud-based services, social media, and mobile data collection (among other things) have ushered in an era of “big data” characterized by a previously unimaginable volume, variety, and velocity of incoming data streams (Gandomi and Haider, 2015). While big data have garnered deserved attention, data generated from individual projects in small volumes at local scales (also called the “long tail of science”) (Heidorn, 2008; Hampton et al., 2013; Wallis et al., 2013) and “dark data” including both unstructured and unused digital data collected during routine business and research (Hampton et al., 2013; Wallis et al., 2013; Ferguson et al., 2014) as well as analog, unarchived, non-machine readable historical data (also known as legacy, or heritage data) (Bürgi and Gimmi, 2007; Salmond et al., 2012) have not. Such datasets are the foundations on which big data is often built (Ferguson et al., 2014) and represent a large portion of the data landscape that is currently underutilized but has recognized potential (Michener and Jones, 2012; Bi et al., 2013; Eitzel et al., 2016; Kelly et al., 2016). This paper responds to the need for new theory and methods to move what we call historical dark data—unarchived, non-digital legacy data—from file drawers to the cloud in order to realize its full potential and become an integral part of the digital data landscape. Historical dark data includes unarchived physical data collections such as accumulated reports, field notes, journals, biological specimens, correspondence, and artifacts.
These materials have three important roles. They: (1) elongate the temporal scale of potential scientific inquiry to include otherwise irretrievable past environments, (2) can provide a contextual foundation from which to assess change, and (3) situates the study of the environment in a wider disciplinary context. However, non-digital formats, variable physical location and condition of these data collections create barriers to productive scientific use and put them at risk of disposal and loss. Several calls have stressed the importance of these types of materials and their preservation, but few standards have emerged to shed light on historical data. To encourage greater use of historical data across scientific disciplines and ensure a future for our past it is important to make these collections findable, accessible, interoperable, and reusable (FAIR) (Wilkinson et al., 2016).
In this paper, we focus on historical environmental data collected in the field at research stations or research properties. These kinds of physical data collections are common—the result of a century of business-as-usual research and daily operations that focused on forestry, ecology and agriculture. We review three University of California (UC) projects that digitize and share historical data collections and evaluate the collections' journey out of the dark and into the larger digital data ecosystem with respect to the FAIR principles. These case studies reveal that historical data are complex, requiring diverse approaches to preservation and dissemination, but they also reveal that such efforts can be invaluable to the environmental sciences.
Data Stewardship Using FAIR Principles
The synthesis of historical and contemporary ecological data with predictive models can be a powerful approach to investigate the complex response of species, communities, and landscapes to changing biophysical conditions in time and space (Kelly et al., 2016). Scientists tackling complex socio-ecological questions regularly deal with large collections of heterogeneous data and recognize that principles from data science can help them in their work (Wolkovich et al., 2012; Hampton et al., 2013; Peters et al., 2014a; Lowndes et al., 2017; Morrison et al., 2017; Wilson et al., 2017). Principles for reproducible data science, such as transparency, reusability, collaboration, and communication (Pedersen et al., 2007; Lowndes et al., 2017) can streamline projects and make science more efficient. Data sharing is a fundamental part of these efforts. Effective data management and sharing are key for data integration, knowledge discovery, and continued use (Wilkinson et al., 2016). The current landscape of scientific data can be fragmented—developed and maintained by individuals or small academic groups, on focused areas, and concentrated in time (Michener et al., 1997; Michener, 2006; Kelly et al., 2016; Waide et al., 2017)—precluding efficient use.
The FAIR (Findable, Accessible, Interoperable, and Reusable) framework is one of several recent efforts to establish best practices and principles for effective data management by the global research community (Michener, 2006; Wilkinson et al., 2016). Guidelines for long term data stewardship are not new, but their adoption in practice can be ad hoc (Michener and Brunt, 2009; Borgman et al., 2015). FAIR principles serve as an umbrella concept for goal setting and evaluating success that may translate across institutional, educational, disciplinary, and technological barriers. Findable requires that data and/or metadata should be uniquely and persistently identified, indexed, and described in detail so that they may be discovered by potential researchers. For data to become accessible once found, they need to be published using standard, free, and open protocols. Using standard data formats and ontologies in this process makes data interoperable. Finally, data need to be reusable, ensuring that data provenance is preserved and well documented for the next user. Data projects that fulfill all FAIR principles are expected to have the most potential for use by new studies, in transdisciplinary research, and are therefore are highly valuable to science.
We believe the FAIR principles promote digital resilience (Wright, 2016) by fostering forward thinking approaches to data archiving, sharing, and use. FAIR can be conceptualized as a road map (Figure 1) with each step elevating the potential and value of data across a spectrum of dark unstructured collections that fulfill no FAIR principles to “open data” that fulfill FAIR (Ferguson et al., 2014). As dark data transition out of file drawers and into digital structures it increases the variety and volume of data that is readily available and can be integrated into scientific workflows, thereby expanding the temporal data record and increasing its potential reach. Achieving FAIR principles will enable the use of historical data in conjunction with contemporary data (Kelly et al., 2016), in transdisciplinary research (Michener, 2015; Beller et al., 2017), and in synthesis or meta-analysis (Wallis et al., 2013). This framework is useful in context of historical dark data because the principles are flexible, and even partial fulfillment can yield success and contribute toward increased use, potential and value of historical data in science. However, as we discuss in the following case studies, achieving FAIR principles is difficult and costly requiring long-term investment, stewardship, and expertise.
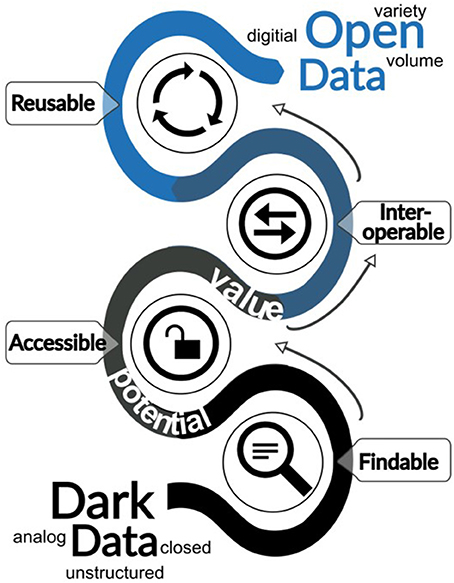
Figure 1. Conceptual diagram of the FAIR roadmap for dark data: each curve represents a step toward increasing the value and potential of dark data for science.
The Current Ecological Data Landscape
The current landscape of ecological data is complex and evolving. Within this landscape, science and synthesis centers (NASA, NCEAS, LTER, NEON, NCALM, and SESYNC) serve as the institutional leads in developing standards in file formats, protocols, tools; and by creating partnerships across institutions or groups that lead to data aggregation or increase the potential for integration (Michener et al., 2011; Rodrigo et al., 2013). These centers work to synthesize and collect heterogeneous environmental data from multiple sources including field observations and experiments, sensor networks, as well as climate and remote sensing data. Data from these centers have been used to study a wide range of environmental phenomena—including land use change, invasive species, phenology, aquatic environments, atmospheric processes, and ecosystem dynamics— largely since the 1970s. These centers have largely succeeded at using and re-using diverse datasets by linking them through standardized metadata and centralized repositories, some of which are created by the centers themselves (Jones et al., 2006), but the coverage of their data often misses key historical events that shaped contemporary ecosystems before 1970 (Alagona et al., 2012).
Recent and numerous efforts to make data discoverable and interoperable has resulted in several types of data repositories. First among these are the recent proliferation of domain-specific data repositories, especially for biological specimens and associated data that use taxonomically-specific language, protocols, and standards. These repositories range from taxon-specific (e.g., VertNet) to taxonomically broad (e.g., GBIF) and include many museum and herbarium records. Data include digitized physical specimens; records of species occurrence, abundance, tolerances; and insight on various other environmental conditions derived from the digitization of field notebooks and journals. Institution-or collection-focused field notebook digitization efforts, such as field notebooks from UC Berkeley libraries (http://ecoreader.berkeley.edu/) or Zooniverse Notes from Nature (https://www.notesfromnature.org/), have shown the potential of crowdsourcing platforms to integrate historical dark data into the digital data landscape through transcription.
Another type, generalist repositories, exist on a spectrum from centralized to decentralized models of data aggregation (Franklin et al., 2017), some serve as a data warehouse collating data from disparate institutions and partners while others collect metadata and finding aids and point to the original location of the data but do not store the data itself. Generalist repositories include university-based efforts (e.g., Harvard's Dataverse, Berkeley's HOLOS); government sponsored national spatial data portals or clearinghouses (e.g., National Map, DataOne) (Crompvoets et al., 2004; Maguire and Longley, 2005; Tait, 2005); and proprietary portals (e.g., ESRI's Living Atlas of the World). Generalist repositories are not unique to ecological, biological, and environmental data, and ecological data and materials often exist in generalist repositories that ecologist may be unaware of (e.g., Digital Public Library of America) (Waide et al., 2017). Allied data repositories may establish even greater interconnections using an Application Programming Interface (API), thus, creating gateways to larger data landscapes. APIs are applications that serve machine-readable data and functionality to applications that represent the data to users.
Data registries (e.g., Registry of Research Data Repositories and FairSharing) serve as guides to help users find appropriate data. Registries provide global indexes of research data repositories, allowing users to search, find, or connect with groups that may have similar data (Pampel et al., 2013). Several scientific journals that require data deposition upon submission (e.g., Nature, Science, PNAS) also guide researchers by listing supported discipline-specific and generalist repositories. Registries foster interconnectivity and potentially reduce redundancy in the creation of new repositories, experiments, and data collections.
Finally, we identify emergent participatory or citizen science data repositories driven by massive public data collection efforts. These include biodiversity databases (e.g., iNaturalist.org) and other distributed and public efforts to document changing climates (e.g., IceWatch). Data from these non-traditional and volunteered collective efforts have already enhanced scientific learning in numerous cases (Kearns et al., 2003; Dickinson et al., 2010, 2012; Connors et al., 2012) and will play a growing role in ecological data collection, sharing, and use. This evolving ecological data landscape (or über network Peters et al., 2014b; Michener, 2015) encourages data discovery, integration, and reuse. Sharing data is a public good that is generated by mutual commitment to scientific principles (Reichman et al., 2011; Hampton et al., 2013; Wallis et al., 2013; Michener, 2015). The value of data and metadata repositories is in their capability to help make collections of data FAIR. However, the growth and success of these repositories has tended to overlook vital elements (and indeed the majority) of the data landscape that were not born digital and are not yet FAIR (Jones et al., 2006).
Growing the Data Landscape With Historical Collections
With the exception of domain specific repositories for biodiversity collections and field notebooks, historical data are disproportionately underrepresented in modern ecological repositories leaving temporal gaps in the scientific record (Szabó and Hédl, 2011; McClenachan et al., 2015). Despite the consensus that historical data are necessary, these types of data are often underutilized in practice (Magurran et al., 2010; Szabó, 2010) due to the difficulty integrating non-digital historical data in routine research. Ecological research using historical data have demonstrated success in modeling the impact of climate change on species abundance and distribution (Shaffer et al., 1998; Tingley and Beissinger, 2009; Pyke and Ehrlich, 2010; Lavoie, 2013), cataloging drastic changes in forest structure, composition, and distribution (Petit et al., 2008; Easterday et al., 2016; Kelly et al., 2016), contextualizing evolutionary processes (Holmes et al., 2016), documenting the spread of infectious disease (Suarez and Tsutsui, 2004; Bradley et al., 2014; DiEuliis et al., 2016), and extending our knowledge of species lineages (Bi et al., 2013). Growing the reach of studies like these will likely depend on dispersed efforts by the many stewards and potential users of historical data.
Projects hoping to digitize and publish historical data face an overwhelming variety of platforms, technologies, standards, and protocols with few available guidelines. Historical data, due to their analog, unstructured nature, defy classic data deposition methods, and require specific approaches that go largely undocumented. The development of protocols to make this type of data FAIR are vitally needed, since any data without redundant and varied storage methods face heightened risk of permanent loss (Elizabeth Griffin, 2015).
Historical data emanating from distributed small research collections are often confronted with a lack of logical physical and digital storage options. This conflict forefronts the choice of either fitting the data to the needs of an existing infrastructure (like the repositories above) or developing a structure that fits the needs of the data. This choice is also constrained by current science funding structures that incentivize and value the creation of new repositories and data over the curation and integration of older ones.
Case Studies of Historical Data Preservation at University of California Research Centers
The University of California (UC) has been a leader in ecological, natural resource, and agricultural field research since the early twentieth century (Rapacciuolo et al., 2014, 2017; Chornesky et al., 2015). We provide three case studies of projects attempting to recover historical dark from the University using different methods and approaches to digitization.
The first data collection comes from nine Research and Extension Centers (RECs) of the UC Division of Agriculture and Natural Resources (ANR) which cover over 5,000 ha of California's Central Valley, Sierra Foothills, and Pacific Coast. Since 1912, ANR RECs have hosted research generating important discoveries across agricultural and ecological disciplines (Downing, 2016; White, 2017) (Figure 2). The second data collection comes from the UC Natural Reserve System (NRS), the largest university-administered network of research reserves and field stations in the world (Fiedler et al., 2013). The earliest NRS site was founded in 1937, and the NRS now manages 39 sites (covering over 303,500 ha) for field research, conservation, teaching, and public outreach. These sites represent nearly every major California bioregion, from the Channel Islands to the High Sierra, and from the Northwest Forest to the Mojave Desert (Figure 3). The third data collection is the California Vegetation Type Map (VTM) Project, which developed from a partnership between UC Berkeley and the U.S. Forest Service (USFS) California Forest and Range Experiment Station (now Southwest Research Station). The VTM Project mapped nearly 40 million ha of the state's natural areas in the 1930's (Wieslander, 1961; Colwell, 1977). The full VTM collection includes detailed vegetation maps, floristic and environmental plot data, landscape photographs, maps showing photographer vantage point and record locations, and herbarium specimens for species recorded on vegetation maps and sample plots (Figure 4).
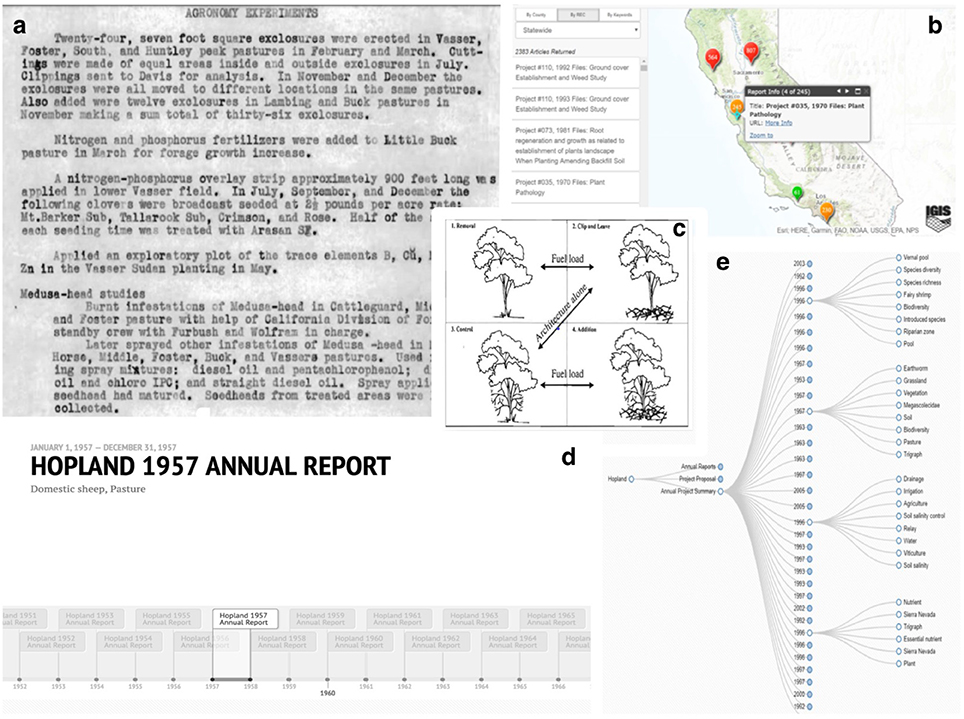
Figure 2. Panel of images from the REC project: (a) example of an original research report; (b) Web mapping platform providing access to the original scanned PDF documents, searchable by REC, keywords, and date; (c) example of image from an original research report showing a fuel reduction experiment; (d) timeline visualization of researchers and topics conducted on Hopland REC; (e) interactive visualization of keywords extracted from research reports screenshot of the interactive website.
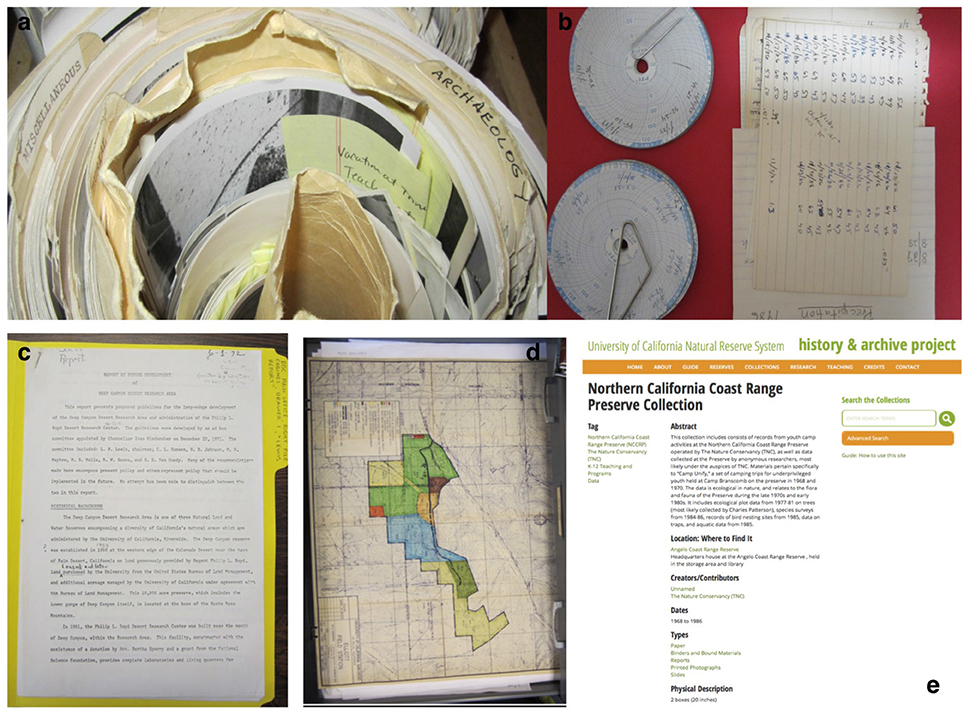
Figure 3. Panel of images from the NRS project: (A) damaged photographic prints at Sweeney Granite Mountains Desert Research Center (GMDRC); (B) historical climate data from GMDRC. (C) Administrative records of land use data from Boyd Deep Canyon Desert Research Center; (D) maps from the UC San Diego NRS campus office; and (E) a sample records collection description for the Angelo Coast Range Reserve on the NRSHAP website (http://archives.nrs.ucsb.edu/).
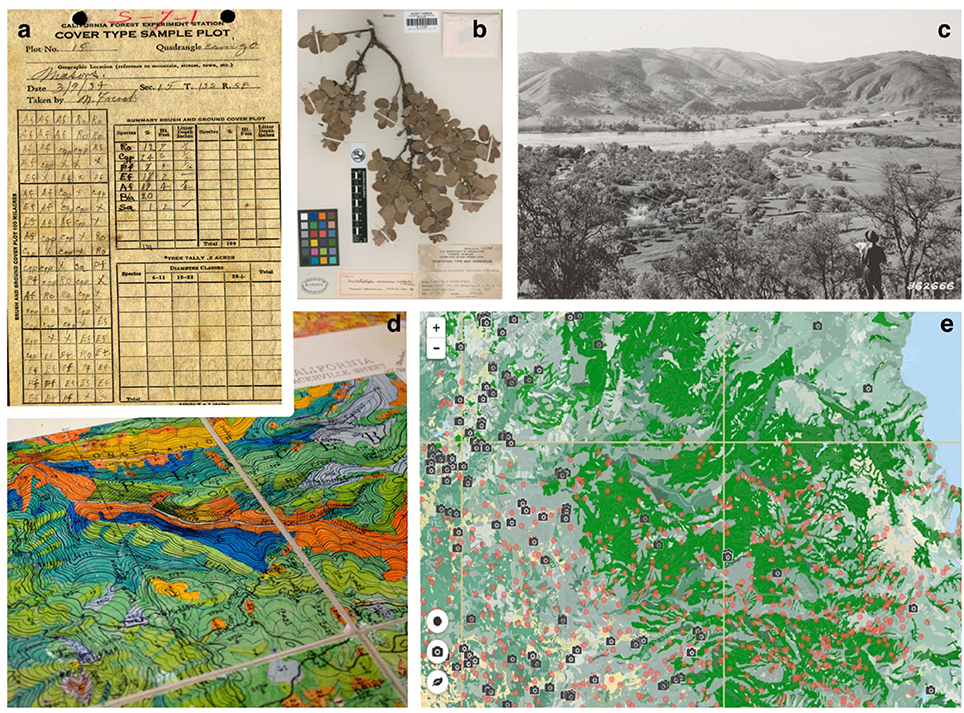
Figure 4. Examples of the components of the VTM collection: (a) plot card recording floristic species; (b) herbarium specimen (Arctostaphylos morroensis, San Luis Obispo Co.); (c) a landscape photograph San Antonio River, Monterey Co. 1938; (d) vegetation type map (Placer Co.); and (e) the digital representation (vtm.berkeley.edu) of maps, plots, and photographs, showing part of Lake Tahoe. In (e) the background colors represent different vegetation types, red dots are plot locations, and black icons are photograph locations.
These three case studies exemplify the data-related problems and opportunities of long-term sites of place-based learning (Alagona and Paulson, 2018). Because of their unique initiatives as centers of science and experimentation, these sites and projects can provide qualitative and quantitative information on human-natural interactions for over a century (Watson et al., 2014; Erb et al., 2016). However, each of these places are sites where valuable data are dark due to lack of infrastructure, incentive, and investment (National Research Council, 2014). The three case studies examined take different approaches to digitizing distributed datasets: one data-driven and led by ecologists and geographers, one metadata-focused and led by historians and archivists, and one object-driven and led by administrators and data scientists. While the approaches taken in digitization were different for each project, determined by expertise and project goal, all of the data within these collections were at risk of loss or destruction. In this way, these cases exemplify varying levels of success in achieving data interoperability and moving the data collection out of the dark and into the modern digital data landscape.
Case Study One: Creating an Object-Based Digital Collection
Background and Need
The ANR RECs project originated out of a pressing need to digitize routine research documents (annual reports, project proposals, and annual project summaries) prior to their physical destruction. Each REC had accumulated large volumes of these documents, and the need for space drove a rapid preservation effort, in which all documents were scanned.
Methods
During the project, a single staff member traveled to each REC and used a digital scanner to digitize all available paper documents (total 3,152) as PDF files. Each one of these documents was stored in an SQL relational database (a database that implements a structured query language to manage the data within it) and given a unique article number, title, coordinates associated with the REC it was retrieved from, year, and URL of the digital document. To make these documents findable to the broader research community, an interactive web application using the ESRI (ESRI ArcGIS Desktop, 2017) web application stack was created (http://igis.ucanr.edu/Infobase/InfobaseExplorer/). The interactive map-based user interface enabled a spatial representation of the entire document repository and allows for simple queries of information within the database. The web application displayed and made the documents discoverable and allowed users to find and download scanned PDFs.
The documents were scanned using a Fujitsu fi-6140Zdj scanner at 300 dpi (Figure 2a) and run through Adobe Acrobat Professional 11.0 optical character recognition (OCR) tools. The resulting extracted character string was also stored in the database. OCR enables the conversion of images of typed, handwritten, or printed text into machine-readable format text (Holley, 2009) enabling search, storage, display and analysis. The project improved upon the original OCR with an automated workflow by testing several programmatic options on an initial site (Hopland REC, n = 564 documents).
The project chose a machine learning (ML) approach, using a series of Natural Language Processing (NLP) tools to extract information on key people, organizations, topics, and scientific keywords from the scanned documents. NLP is an area of computer science focused on training computers to process large collections of written texts. This project developed a machine learning pipeline using NLP techniques to interpret the scanned documents when trained with common subject matter libraries of agricultural, biological, and ecological text. We investigated several tools to improve our ability to extract information from the documents including Ocular, a tool that uses unsupervised learning methods to recognize text from scanned historical documents including opaque text (Berg-Kirkpatrick et al., 2013), and Tesseract, a Google-funded open-source OCR system. Tesseract yielded more complete results compared to the baseline OCR text. The output text strings were analyzed with Alchemy, a NLP tool based on IBM's artificial intelligence Watson machine, which uses deep machine learning algorithms to analyze massive amounts of structured and unstructured content. Critical information (e.g., keywords, organizations, people, and scientific topics) from the processed OCR strings were extracted and visualized by year and document type using the D3 javascript library.
Data Uses
This project has primarily served internal, custodial goals, and although there has been little external use of the data, the project was able to mine the preserved documents to capture key data on researchers, research projects, scientific concepts, and keywords over a 60-year period at Hopland REC through the ML process described above. For example, we were able to extract a summary of researchers and research conducted at Hopland annually from 1951—present (e.g., “animal science,” “pasture forage,” “spring fertilizer application,” “herbage production,” “biodiversity”). This kind of information, derived automatically from scanned documents, can assist future researchers to find related data for their own projects. This information is also valuable for tracking and understanding the evolution of research and science at the RECs and the intensity, scope, scale, and frequency of management actions taken at each site. Documenting past research and management treatments is needed to understand implications for ongoing and future research projects. Making data findable and accessible to the broader research community would greatly increase the success of this preservation effort and now that it is digitally captured can be ingested into existing repositories with a wider reach such as the Biodiversity Heritage Library.
Case Study Two: Creating a Digital Metadata Archive
Background and Need
The UC Natural Reserve System History and Archive Project (NRSHAP) represents an initial effort to preserve the historical materials of the NRS and promote their use for research and education in both science and history. NRSHAP operated for 7 years (2011–2017) with funding from the National Science Foundation and UC to identify, index, preserve, and promote historical records held on or pertaining to the NRS. NRSHAP was led by historians and archivists who adapted standard archival protocols to non-traditional sites (The University of Chicago, 2006; Young, 2006; Society of American Archivists, 2013). The NRS field station historical datasets come in diverse formats (Figure 3) therefore, the goal of the project was to provide enough information about the existing records that potential researchers may identify data types and provide for their own use of the materials on site rather than develop individualized digitization workflows. The outcome was a digital metadata archive. Metadata is commonly defined as “data about data” and can be used to locate, describe, and retain data provenance. NRSHAP is a unique document archiving project because of its spatial coverage including 39 field stations and reserves, 8 campus offices, one system-wide administrative office, several independent archives, and personal collections across the state of California.
Methods
NRSHAP developed a multi-step data-preservation method for field stations and other remote or dispersed organizations/sites with potential archives. Initially, NRSHAP distributed questionnaires to all site contacts to assess the scope of potential historical records. this questionnaire was followed by extensive research into the known history of the NRS and its sites. NRSHAP teams traveled to each site and conducted a records inventory following established archival methods (The University of Chicago, 2006; Young, 2006; Society of American Archivists, 2013).
The inventory was divided into either “active records”—still in use for the regular operation of the station— and “inactive records”—no longer used but still of value. The inactive records were then grouped into collections and information on the physical location, the creators, date, physical material types, the arrangement (by subject, chronological) and the physical description of the size of the collection (e.g., linear feet) was tagged and used to create an archival collection description or “finding aid” following established standards (Society of American Archivists, 2013). This information was documented in a database and published online (http://archives.nrs.ucsb.edu) creating a metadata archive. However, not all records pertaining to the NRS existed on site, and throughout the years several material types and collections were sent to various institutions. NRSHAP researchers identified other collections relating to the NRS history that were held in other archives (e.g., Bancroft Library); affiliate organization offices such as State Parks; and the personal offices and homes of past staff and researchers and linked to these existing collections. Therefore, published descriptions of the field station archives may also sometimes be found on existing archival networks and search engines, such as the Online Archive of California or Archivegrid. NRSHAP made recommendations to station managers regarding the best means of preserving and promoting their historical collections. Preparing for this involved meeting with potential institutions and repositories across California regarding their interest in acquiring and managing NRS materials. Finally, NRSHAP developed a plan for regular review of metadata accuracy, document health (if still held on site), and ongoing off-site research for relevant collections.
Data Uses
When NRSHAP began, the project was on the cusp of a broader awakening among scientific researchers and field station managers to the potential of historical documents or dark data. Along the way we encountered lots of support and encouragement from people invested in the NRS system, but many also expected the effort to involve digitization of the records themselves. Historians have used archives as their primary data method for almost 200 years, but archival research methodologies are mostly project-specific and have never been standardized or fully articulated. Potential data users should not see this as a hindrance, but an opportunity, since archival methods are flexible and can be adapted for inclusion in projects involving other types of data collection and analysis. Morrison et al. (2017) argue that these kinds of connections will be necessary for the future of ecology. NRSHAP bridged epistemological and methodological divides across disciplines to create new opportunities for more robust research and collaborations. Metadata archives hold great potential for data reuse. However, the success of field station metadata archives will inevitably rely on targeted and continued efforts promoting use of the archive itself and educating those on best practices once it has been created.
NRSHAP affiliates have promoted use of the metadata archive by speaking at NRS system-wide meetings and academic conferences, using the website as a teaching tool in undergraduate classes, and conducting their own research projects. NRSHAP has attracted interest from researchers across the UC system and is already being used by one, ongoing international collaborative research project.
Case Study Three: Creating a Completely Digitized Data Collection
Background and Need
The Wieslander Vegetation Type Mapping (VTM) collection, named after director Albert Wieslander, was an exhaustive and detailed effort to map California land cover in the early twentieth century. During the 1920–30s, VTM crews surveyed 16 million ha (40%) of California's wildlands. They collected vegetation information at over 18,000 plots, produced detailed maps of dominant vegetation for over 100,000 km2, gathered over 23,000 herbarium specimens, and took over 3,000 photographs (Colwell, 1977; Kelly et al., 2016). Until recently, the full collection was distributed throughout libraries and labs statewide. Significant, and partly unknown, portions of the collection were lost to custodial needs and competing collections' demand on space (Kelly et al., 2016). Overt risk of loss, combined with the tremendous depth of content, provided the impetus for many individual digitization efforts across the state, which eventually combined in the early twenty-first century (Kelly et al., 2016).
Methods
The digitization of the vegetation maps, plots, plot maps, photographs, and locations of herbarium specimens took place over a decade, in several UC labs and libraries including the Marian Koshland Biosciences Library and the Museum of Vertebrate Zoology (MVZ). Linework from the vegetation maps was manually digitized and polygon values linked to a spatial database; plot data were transcribed manually and joined to plot locations which were manually digitized from plot location maps; photographs were scanned and where possible attributed with a geographic location; and herbarium specimens were georeferenced using analog accompanying information (Kelly et al., 2008, 2016, 2017).
All digital VTM data (geographic, ecological, and photographic) were stored using PostgreSQL, a relational database that supports the storage, analysis, and transfer of geospatial vector data through a PostGIS extension (Kelly et al., 2016). The data itself is downloadable as standard text and spatial data formats that can be used in numerous GIS and statistical software packages. An interactive web map interface was built for exploring, searching, aggregating, and downloading the VTM data collection using Leaflet (a JavaScript mapping library for web mapping) and Open Street Map base layers. The VTM website (vtm.berkeley.edu) was built using the HOLOS API from which the VTM data is linked to the structured digital database and allows for analysis of raw data, integration with contemporary data, as well as rapid interaction and visualization (Thorne et al., 2008; Dolanc et al., 2013; McIntyre et al., 2015; Easterday et al., 2016).
Data Uses
The VTM data has been used since the mid- twentieth century, and its digitization has increased the scope and scale of the types of analysis performed (Kelly et al., 2016). The vegetation data found in the plot database have been used to develop vegetation classification schemes and to examine changes to chaparral and forest communities around the state enabling prediction of community structure and shifts under a changing climate. The vegetation maps have been used to document regional changes in vegetation communities, to investigate legacies of land use change, and to support land use planning.
Discussion—Evaluation of Cases With the Fair Principles
These three cases provide different protocols for data digitization, and we evaluate them here with respect to the FAIR principles and summarized our findings in Table 1. The REC collections of historical research documents were completely digitized via scanning and OCR and made available via the web. These data “objects” were findable as text strings through a simple database web search. Several machine learning (ML) algorithms were used to reconstruct context and make the data accessible, however, the digitization process did not result in interoperable or reusable data because the data remained unstructured. The major advantage of this approach was speed; the complete collection of physical records can be made digital with limited technical skill and made available to a broader audience.
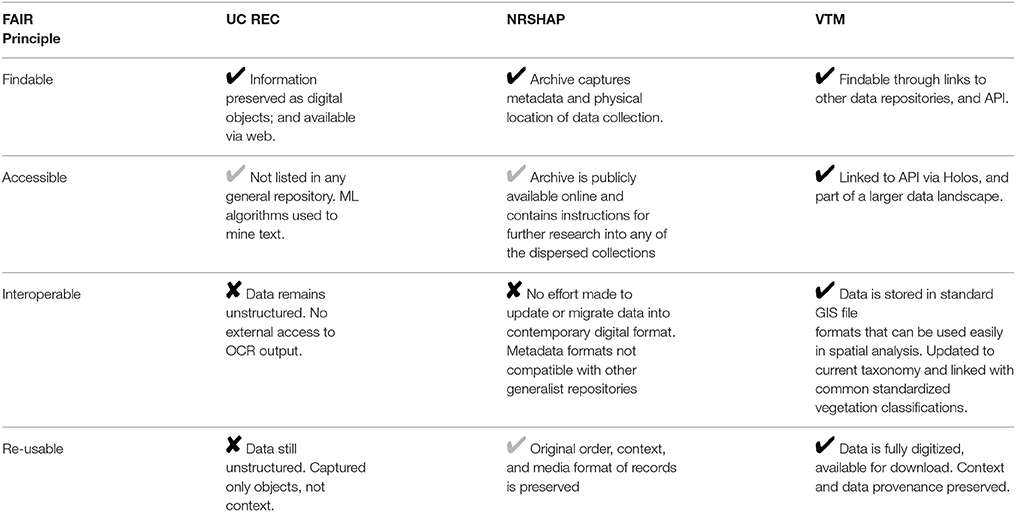
Table 1. Evaluation of three case studies according to FAIR data management principles; = successful;
= partially successful; and
= not successful.
NRSHAP focused on making physical objects findable through metadata, and accessible as the metadata contained essential instructions for finding the data. The data itself remained on site in curated and semi-curated collections. The major limitation of the metadata archive approach is the limited access to the data itself. The data can now be discovered but requires further investment to use. The VTM project provides an example of a completely digitized data collection that reaches all the benchmarks of the FAIR standard. Data are findable and accessible through links from several data repositories, through an API and as part of a larger data landscape supported by HOLOS; data are interoperable as it is stored in standard spatial data file formats that can be used easily in most common spatial analysis and statistical software with updated nomenclature to be readily used in conjunction with contemporary species and vegetation codes; and data is reusable because the digitization methodology and data provenance are fully documented.
Analysis of these case studies finds that FAIR is a valuable tool for data preservation planning and evaluation, though not all projects will accomplish FAIR fully. Making datasets findable and accessible, alone, creates awareness, but is often insufficient to ensure data reuse and longevity. All of these projects faced challenges, yet they all ultimately increased the potential and value of the datasets through their efforts. For example, in our first case, some success was achieved in resurrecting critical components of the historic scientific record at the RECs, and this information was shared via a web application. However, the workflow in extracting value-added information from the documents was not without flaw and most of the information therefore remains unstructured in a non-machine-readable format. Efforts that span the entire FAIR process require diverse skill sets and multidisciplinary teams with some combination of computer scientists, data scientists, ecologists, historians, librarians, land managers, and web developers working together. Indeed, all our cases required input from multidisciplinary teams. When the FAIR is achieved data can be used in unexpected ways, making valuable, transdisciplinary analysis possible. In the case of VTM, there was a documented increase in the scope and scale of research conducted with the dataset once it was made digitally available (Kelly et al., 2016).
However, since there is often a time lag between digitizing, sharing, and the use of a data collection, management requires long term stewardship. Each case study dealt with collections of heterogeneous materials that did not readily fit into existing repositories. Rather than separating the collections, individualized databases were created to host and make the materials accessible. In this way dark data from small projects gained recognition and use amongst the immediate research community, but their reach remains limited (Van Noorden, 2013). Potentially mirroring key parts of a collection—such as field notebooks or biological specimens—that have readily recognized repositories can increase this reach but this risks loss of data provenance and potentially reduces the use of other materials from the same collection. Balancing these risks requires careful planning.
Not all collections lend themselves to traditional data digitization. As shown in the case of NRSHAP, the metadata archive approach works well for field stations because it is designed for geographically remote or distributed data collections; it provides for either internal hosting or third-party data-management options as appropriate; it serves both data promotion and long-term data management; and does not usually require reorganization of documents. The metadata archive approach focuses on the kind of information that both outside researchers and station staff need to be able to reuse existing historical datasets and does not require the archivist to know or anticipate the character or media format of future scientific reuses. Further, some speculate that physical archives are the best way to save information, since physical materials (even under threat of pests, mold, paper acids, and natural disaster) have a much longer shelf life than any known digital forums, and remain legible to human eyes long after advancing computer technologies make current digital information obsolete (Scott, 2007; Klein, 2008; Clement et al., 2013; Wright, 2014). However, best practice is to have redundant collections of both physical and digital renditions. Finally, automated approaches to digitization do not always save time in the long run, since considerable human input might be required to ensure data is fully interoperable and reusable. As demonstrated in the ANR case, historic documents can be difficult to digitize meaningfully. Uneven typesets, faded ink, and handwriting all pose common and serious obstacles to automated information retrieval.
The FAIR principles provide flexible guidelines for the stewardship of heterogeneous data types, yet do not address the need to first make historical data digitally discoverable. Sharing examples of how historical dark data is made digital, and then FAIR will lead to an exchange of successful protocols that may lead to eventual standards. Developing standards and ontologies is paramount to the interoperability and reuse of all data (Jones et al., 2006), but is largely lacking for historical dark data. Adopting contemporary data science standards, such as FAIR, for historical data will help to integrate historical and contemporary data, but the high standards of “open data” should not preclude preservation of historical data. Primarily, the first two principles —findable and accessible should have scalar and adaptive rules that are relative to the project's goals, the different stages in which data are created, and to the overarching goal of creating maximum potential use. For example, none of the projects succeeded in assigning the collections persistent identifiers (PIs) including Digital Object Identifiers (DOIs) or Archival Resource Keys (ARKs) that would make them findable the way FAIR is defined. Each of these projects understood that FAIR must be relative to the quality of data, the resources at hand, the projects goal, and the communities' standards. These three case studies made their collections more findable and accessible to their immediate research communities including those presently most interested in using and reusing the data, yet each given the time and resources would open these data collections to a much broader research community and create potential for further discovery.
Conclusions
Comprehending the temporal and human dynamics of ecosystems is a central challenge of science in the Anthropocene (Robin and Steffen, 2007; Safford et al., 2012). This requires synthesis and sharing of transdisciplinary, heterogeneous datasets over long time periods and large spatial scales (Kelly et al., 2016; Lowndes et al., 2017). Within the last half century, the increasingly large streams of data from sensor networks, mobile technology, and remote sensing has created both opportunities, “big data” (Gandomi and Haider, 2015), and challenges, “data deluge” (Porter et al., 2012; Hampton et al., 2013), establishing the need for better data science workflows and training across most disciplines. Often overlooked in this discussion are the large majority of scientific data that are created by small research groups with limited resources for data planning and management (Hampton et al., 2013). The majority of scientific data potentially available for future research and synthesis never make it into a discoverable repository and remain inaccessible to the broader community (Heidorn, 2008). Without proper incentive, support, or standards in place to consistently capture data and make it accessible, it often goes “dark”—limiting the scope and potential of scientific research.
Historical data are vital to current ecological research: they provide benchmarks from which to compare change, they can be linked to modern ecological data to create new knowledge, and they can be modeled to help predict future changes and validate models. We argue that these data are “dark” until they are effectively digitized and made discoverable to a wider audience. In the strictest definition, dark data is unstructured, untagged and untapped data that is created through routine activities yet has not been analyzed or processed. Dark data is increasingly recognized in business and economics as vulnerable, underutilized, and valuable (Heidorn, 2008), and we argue that the same is true of historical data for science.
Achieving successful sharing of historical data can be difficult and time consuming, since these collections are often analog, unstructured, and physically distributed. Our review of three novel approaches to digitizing historical field data showcase some of these challenges. We evaluated each approach with respect to the FAIR principles (findable, accessible, interoperable and reusable) (Wilkinson et al., 2016), and revealed both the value of the framework and its limitations in practice. The most effective digitization projects demand lots of human, technical, and capital resources. Making datasets findable and accessible is a necessary first step to creating demand, but not sufficient to ensure data reuse and longevity. Second, efforts that span the entire FAIR process require diverse skill sets and transdisciplinary work often with some combination of land stewards, ecologists, historians, librarians, archivist, data scientists, computer scientists, and web developers working together.
An encouraging antidote to the challenges facing those working to digitize historical data can be the foresight provided by leaders of early twentieth century field data collection. Joseph Grinnell, founder of MVZ and a preeminent field scientist of the day, wrote of his own preservation efforts: “After the lapse of many years, possibly a century, the student of the future will have access to the original record of faunal conditions in California” (Grinnell, 1910). Potential use of Grinnell's and others' data only grows as technologies increase to repurpose the data to answer questions unimagined at the time of their collection (Morrison et al., 2017).
We, as a global scientific community, have the responsibility to continue to shed light on historical data through digitization, adding scientific knowledge, strengthening cultural heritage, and increasing public good. Field research and research reserves are not only major producers and repositories of scientific data, but also can be key agents in making data shareable for researchers and the public. Thus, field stations, research reserves, and field data projects are critical nodes in the nexus of big and dark data: enlarging and enriching a growing data landscape. Going forward capturing the intellectual infrastructure from these sites will require systematic investment, strategy, and leadership to preserve and maintain ecological records for future generations. Envisioning a future for historical data will require an exchange of tools, technology, methodology, and transdisciplinary work at the intersection of data science, history, ecology, and ecoinformatics, and is a vision that if achieved ensures that future generations have access to the past.
Author Contributions
KE and MK lead the conceptualization and design of the work. KE led the writing of the manuscript. PA and TP were responsible for the UCNRS case study and contributed to the writing of the manuscript. MK and KE conducted initial analysis on the VTM and the UC REC case study with SF and PD.
Conflict of Interest Statement
The authors declare that the research was conducted in the absence of any commercial or financial relationships that could be construed as a potential conflict of interest.
Acknowledgments
We would first like to acknowledge support from the Institute for the Study of Ecological and Evolutionary Climate Impacts (ISEECI) in funding the historical ecology working group that brought the authors and therefore the case studies together as well as the support of KE through a graduate research fellowship. The UC NRS History and Archive Project acknowledges the support of the National Science Foundation, Mellon Foundation, and University of California, with special thanks to Laurie Hannah, Andrew Esch, Olivia Herr, Marissa Lopez, and all partners and collaborators in the NRS. This work was also supported by USDA National Institute of Food and Agriculture.
References
Alagona, P., and Paulson, T. (2018).“From the classroom to the countryside: the university of california's natural reserve system and the role of field stations in american academic life,” in Landscape and the Academy, eds J. Beardsley and D. Bluestone (Washington DC: Dumbarton Oaks Research Library & Collection), 207–228.
Alagona, P. S., Sandlos, J., and Wiersma, Y. F. (2012). Past imperfect: using historical ecology and baseline data for conservation and restoration projects in North America. Environ. Philos. 9, 49–70. doi: 10.5840/envirophil2012914
Beller, E., McClenachan, L., Trant, A., Sanderson, E. W., Rhemtulla, J., Guerrini, A., et al. (2017). Toward principles of historical ecology. Am. J. Bot. 104, 645–648. doi: 10.3732/ajb.1700070
Berg-Kirkpatrick, T., Durrett, G., and Klein, D. (2013). “Unsupervised transcription of historical documents,” in Proceedings of the 51st Annual Meeting of the Association for Computational Linguistics, Vol. 1 (Sofia: Long Papers), 207–217.
Bi, K., Linderoth, T., Vanderpool, D., Good, J. M., Nielsen, R., and Moritz, C. (2013). Unlocking the vault: next-generation museum population genomics. Mol. Ecol. 22, 6018–6032. doi: 10.1111/mec.12516
Borgman, C. L., Darch, P. T., Sands, A. E., Pasquetto, I. V., Golshan, M. S., Wallis, J. C., et al. (2015). Knowledge infrastructures in science: data, diversity, and digital libraries. Int. J. Digital Libraries 16, 207–227. doi: 10.1007/s00799-015-0157-z
Bradley, R. D., Bradley, L. C., Garner, H. J., and Baker, R. J. (2014). Assessing the value of natural history collections and addressing issues regarding long-term growth and care. BioScience 64, 1150–1158. doi: 10.1093/biosci/biu166
Bürgi, M., and Gimmi, U. (2007). Three objectives of historical ecology: the case of litter collecting in Central European forests. Landsc. Ecol. 22, 77–87. doi: 10.1007/s10980-007-9128-0
Chornesky, E. A., Ackerly, D. D., Beier, P., Davis, F. W., Flint, L. E., Lawler, J. J., et al. (2015). Adapting California's Ecosystems to a Changing Climate. Bioscience 65, 247–262. doi: 10.1093/biosci/biu233
Clement, T., Hagenmaier, W., and Knies, J. L. (2013). Toward a notion of the archive of the future: impressions of practice by librarians, archivists, and digital humanities scholars. Libr. Q. 83, 112–130. doi: 10.1086/669550
Colwell, W. L. Jr. (1977). “The status of vegetation mapping in California today,” in Terrestrial Vegetation of California, eds M. G. Barbour and J. A. Major (New York, NY: Wiley and Sons).
Connors, J. P., Lei, S., and Kelly, M. (2012). Citizen science in the age of neogeography: utilizing volunteered geographic information for environmental monitoring. Ann. Assoc. Am. Geogr. 102, 1267–1289. doi: 10.1080/00045608.2011.627058
Crompvoets, J., Bregt, A., Rajabifard, A., and Williamson, I. (2004). Assessing the worldwide developments of national spatial data clearinghouses. Int. J. Geogr. Inf. Sci. 18, 665–689. doi: 10.1080/13658810410001702030
Dickinson, J. L., Shirk, J., Bonter, D., Bonney, R., Crain, R. L., Martin, J., et al. (2012). The current state of citizen science as a tool for ecological research and public engagement. Front. Ecol. Environ. 10:236. doi: 10.1890/110236
Dickinson, J. L., Zuckerberg, B., and Bonter, D. N. (2010). Citizen science as an ecological research tool: challenges and benefits. Annu. Rev. Ecol. Evol. Syst. 41, 149–172. doi: 10.1146/annurev-ecolsys-102209-144636
DiEuliis, D., Johnson, K. R., Morse, S. S., and Schindel, D. E. (2016). Specimen collections should have a much bigger role in infectious disease research and response. Proc. Natl. Acad. Sci.U.S.A. 113, 4–7. doi: 10.1073/pnas.1522680112
Dolanc, C. R., Thorne, J. H., and Safford, H. D. (2013). Widespread shifts in the demographic structure of subalpine forests in the Sierra Nevada, California, 1934 to 2007. Glob. Ecol. Biogeogr. 22, 264–276. doi: 10.1111/j.1466-8238.2011.00748.x
Downing, J. (2016). Sierra foothill REC: quantifying IPM benefits in rangeland systems. Calif. Agric. 70, 174–174. doi: 10.3733/ca.2016a0022
Easterday, K. J., McIntyre, P. J., Thorne, J. H., Santos, M. J., and Kelly, M. (2016). Assessing threats and conservation status of historical centers of oak richness in California. Urban Planning 1, 65–78. doi: 10.17645/up.v1i4.726
Eitzel, M. V., Kelly, M., Dronova, I., Valachovic, Y., Quinn-Davidson, L., Solera, J., et al. (2016). Challenges and opportunities in synthesizing historical geospatial data using statistical models. Ecol. Inform. 31, 100–111. doi: 10.1016/j.ecoinf.2015.11.011
Elizabeth Griffin, R. (2015). When are old data new data? GeoResJ 6, 92–97. doi: 10.1016/j.grj.2015.02.004
Erb, K.-H., Luyssaert, S., Meyfroidt, P., Pongratz, J., Don, A., Kloster, S., et al. (2016). Land management: data availability and process understanding for global change studies. Glob. Chang. Biol. 23, 512–533. doi: 10.1111/gcb.13443
ESRI ArcGIS Desktop (2017). ESRI ArcGIS Desktop. Redlands, CA: Environmental Systems Research Institute
Ferguson, A. R., Nielson, J. L., Cragin, M. H., Bandrowski, A. E., and Martone, M. E. (2014). Big data from small data: data-sharing in the “long tail” of neuroscience. Nat. Neurosci. 17, 1442–1447. doi: 10.1038/nn.3838
Fiedler, P. L., Rumsey, S. G., and Wong, K. M. (2013). The Environmental Legacy of the UC Natural Reserve System. Berkeley; Los Angeles, CA: University of California Press.
Fosso Wamba, S., Akter, S., Edwards, A., Chopin, G., and Gnanzou, D. (2015). How “big data” can make big impact: findings from a systematic review and a longitudinal case study. Int. J. Prod. Econ. 165, 234–246. doi: 10.1016/j.ijpe.2014.12.031
Franklin, J., Serra-Diaz, J. M., Syphard, A. D., and Regan, H. M. (2017). Big data for forecasting the impacts of global change on plant communities. Glob. Ecol. Biogeogr. 26, 6–17. doi: 10.1111/geb.12501
Gandomi, A., and Haider, M. (2015). Beyond the hype: big data concepts, methods, and analytics. Int. J. Inf. Manage. 35, 137–144. doi: 10.1016/j.ijinfomgt.2014.10.007
Hampton, S. E., Strasser, C. A., Tewksbury, J. J., Gram, W. K., Budden, A. E., Batcheller, A. L., et al. (2013). Big data and the future of ecology. Front. Ecol. Environ. 11, 156–162. doi: 10.1890/120103
Heidorn, P. B. (2008). Shedding light on the dark data in the long tail of science. Libr. Trends 57, 280–299. doi: 10.1353/lib.0.0036
Holley, R. (2009). How good can it get? Analysing and improving OCR accuracy in large scale historic newspaper digitisation programs. D-Lib Magazine 15.
Holmes, M. W., Hammond, T. T., Wogan, G. O., Walsh, R. E., LaBarbera, K., Wommack, E. A., et al. (2016). Natural history collections as windows on evolutionary processes. Mol. Ecol. 25, 864–881. doi: 10.1111/mec.13529
Jones, M. B., Schildhauer, M. P., Reichman, O. J., and Bowers, S. (2006). The new bioinformatics: ecological data integrating the gene to the from biosphere. Annu. Rev. Ecol. Evol. Syst. 37, 519–544. doi: 10.1146/annurev.ecolsys.37.091305.110031
Kearns, F. R., Kelly, M., and Tuxen, K. A. (2003). Everything happens somewhere: using WebGIS as a tool for sustainable natural resource management. Front. Ecol. Environ. 1:541–548. doi: 10.2307/3868165
Kelly, M., Easterday, K., Koo, M., Thorne, J. H., Mukythar, S., and Galey, B. (2017). Geospatial informatics key to recovering and sharing historical ecological data for modern use. Photogrammetric Eng. Remote Sens. 83, 779–786. doi: 10.14358/PERS.83.10.779
Kelly, M., Easterday, K., Rapicullio, G., Koo, M., McIntyre, P., and Thorne, J. (2016). Rescuing and sharing historical vegetation data for ecological analysis: the California vegetation type mapping project. Biodivers. Inf. 11, 40–62. doi: 10.17161/bi.v11i0.5886
Kelly, M., Ueda, K.-I., and Allen-Diaz, B. (2008). Considerations for ecological reconstruction of historic vegetation: analysis of the spatial uncertainties in the California vegetation type map dataset. Plant Ecol. 194, 37–49. doi: 10.1007/s11258-007-9273-1
Klein, D. (ed.). (2008). Identifying Museum Insect Pest Damage. Washington, DC: National Park Service Museum Management Program. Available online at: https://www.nps.gov/museum/publications/conserveogram/03-11.pdf
Lavoie, C. (2013). Biological collections in an ever changing world: herbaria as tools for biogeographical and environmental studies. J. PPEES Sourc. 15, 68–76. doi: 10.1016/j.ppees.2012.10.002
Lowndes, J. S. S., Best, B. D., Scarborough, C., Afflerbach, J. C., Frazier, M. R., O'Hara, C. C., et al. (2017). Our path to better science in less time using open data science tools. Nat. Ecol. Evol. 1:160. doi: 10.1038/s41559-017-0160
Maguire, D. J., and Longley, P. A. (2005). The emergence of geoportals and their role in spatial data infrastructures. Comput. Environ. Urban Syst. 29, 3–14. doi: 10.1016/S0198-9715(04)00045-6
Magurran, A. E., Baillie, S. R., Buckland, S. T., Dick, J. M., Elston, D. A., Scott, E. M., et al. (2010). Long-term datasets in biodiversity research and monitoring: assessing change in ecological communities through time. Trends Ecol. Evol. 25, 574–582. doi: 10.1016/j.tree.2010.06.016
McClenachan, L., Cooper, A. B., McKenzie, M. G., and Drew, J. A. (2015). The importance of surprising results and best practices in historical ecology. Bioscience 65, 932–939. doi: 10.1093/biosci/biv100
McIntyre, P. J., Thorne, J. H., Dolanc, C. R., Flint, A. L., Flint, L. E., Kelly, M., et al. (2015). Twentieth-century shifts in forest structure in California: denser forests, smaller trees, and increased dominance of oaks. Proc. Natl. Acad. Sci.U.S.A. 112, 1458–1463. doi: 10.1073/pnas.1410186112
Michener, W. K. (2006). Meta-information concepts for ecological data management. Ecol. Inform. 1, 3–7. doi: 10.1016/j.ecoinf.2005.08.004
Michener, W. K. (2015). Ecological data sharing. Ecol. Inform. 29, 33–44. doi: 10.1016/j.ecoinf.2015.06.010
Michener, W. K., and Brunt, J. W. (2009). Ecological Data: Design, Management and Processing. Franklin Township, NJ: John Wiley & Sons.
Michener, W. K., Brunt, J. W., Helly, J. J., Kirchner, T. B., and Stafford, S. G. (1997). Nongeospatial metadata for the ecological sciences. Ecol. Appl. 7, 330–342. doi: 10.1890/1051-0761(1997)007[0330:NMFTES]2.0.CO;2
Michener, W. K., and Jones, M. B. (2012). Ecoinformatics: supporting ecology as a data-intensive science. Trends Ecol. Evol. 27, 85–93. doi: 10.1016/j.tree.2011.11.016
Michener, W. K., Porter, J., Servilla, M., and Vanderbilt, K. (2011). Long term ecological research and information management. Ecol. Inform. 6, 13–24. doi: 10.1016/j.ecoinf.2010.11.005
Morrison, S. A., Sillett, T. S., Funk, W. C., Ghalambor, C. K., and Rick, T. C. (2017). Equipping the 22nd-century historical ecologist. Trends Ecol. Evol. 32, 578–588. doi: 10.1016/j.tree.2017.05.006
National Research Council (2014). Enhancing the Value and Sustainability of Field Stations and Marine Laboratories in the 21st Century. Washington, DC: The National Academies Press. doi: 10.17226/18806
Pampel, H., Vierkant, P., Scholze, F., Bertelmann, R., Kindling, M., Klump, J., et al. (2013). Making research data repositories visible: the re3data.org Registry. PLoS ONE 8:e78080. doi: 10.1371/journal.pone.0078080
Pedersen, B., Kearns, F., and Kelly, M. (2007). Methods for facilitating web-based participatory research informatics. Ecol. Inform. 2, 33–42. doi: 10.1016/j.ecoinf.2007.02.003
Peters, D. P. C., Havstad, K. M., Cushing, J., Tweedie, C., Fuentes, O., and Villanueva-Rosales, N. (2014a). Harnessing the power of big data: infusing the scientific method with machine learning to transform ecology. Ecosphere 5, 1–15. doi: 10.1890/ES13-00359.1
Peters, D. P. C., Loescher, H. W., SanClements, M. D., and Havstad, K. M. (2014b). Taking the pulse of a continent: expanding site-based research infrastructure for regional- to continental-scale ecology. Ecosphere 5, 1–23. doi: 10.1890/ES13-00295.1
Petit, R. J., Hu, F. S., and Dick, C. W. (2008). Forests of the past: a window to future changes. Science 320, 1450–1452. doi: 10.1126/science.1155457
Porter, J. H., Hanson, P. C., and Lin, C.-C. (2012). Staying afloat in the sensor data deluge. Trends Ecol. Evol. 27, 121–129. doi: 10.1016/j.tree.2011.11.009
Pyke, G. H., and Ehrlich, P. R. (2010). Biological collections and ecological/environmental research: a review, some observations and a look to the future. Biol. Rev. Camb. Philos. Soc. 85, 247–266. doi: 10.1111/j.1469-185X.2009.00098.x
Rapacciuolo, G., Ball-Damerow, J. E., Zeilinger, A. R., and Resh, V. H. (2017). Detecting long-term occupancy changes in Californian odonates from natural history and citizen science records. Biodivers. Conserv. 26, 1–17. doi: 10.1007/s10531-017-1399-4
Rapacciuolo, G., Maher, S. P., Schneider, A. C., Hammond, T. T., Jabis, M. D., Walsh, R. E., et al. (2014). Beyond a warming fingerprint: individualistic biogeographic responses to heterogeneous climate change in California. Glob. Chang. Biol. 20, 2841–2855. doi: 10.1111/gcb.12638
Reichman, O. J., Jones, M. B., and Schildhauer, M. P. (2011). Challenges and opportunities of open data in ecology. Science 331, 703–705. doi: 10.1126/science.1197962
Robin, L., and Steffen, W. (2007). History for the Anthropocene. Hist. Compass 5, 1694–1719. doi: 10.1111/j.1478-0542.2007.00459.x
Rodrigo, A., Alberts, S., Cranston, K., Kingsolver, J., Lapp, H., McClain, C., et al. (2013). Science incubators: synthesis centers and their role in the research ecosystem. PLoS Biol. 11:e1001468. doi: 10.1371/journal.pbio.1001468
Safford, H. D., Hayward, G. D., Heller, N. E., and Wiens, J. A. (2012). “Historical ecology, climate change, and resource management: can the past still inform the future?,” in Historical Environmental Variation in Conservation and Natural Resource Management, eds J. A. Wiens, G. D. Hayward, H. D. Safford, and C. M. Giffen (Chichester, UK: John Wiley & Sons, Ltd.), 46–62.
Salmond, A., Lythberg, B., and Newell, J. (2012). Old objects, new media: historical collections, digitization and affect. J. Mater. Cult. 17, 287–306. doi: 10.1177/1359183512453534
Scott, R. E. (2007). e-Records in health–preserving our future. Int. J. Med. Inform. 76, 427–431. doi: 10.1016/j.ijmedinf.2006.09.007
Shaffer, H. B., Fisher, R. N., and Davidson, C. (1998). The role of natural history collections in documenting species declines. Trends Ecol. Evol. 13, 27–30. doi: 10.1016/S0169-5347(97)01177-4
Society of American Archivists (2013). Describing Archives: A Content Standard, 2nd Edn. Chicago, IL: Society of American Archivists.
Suarez, A. V., and Tsutsui, N. D. (2004). The value of museum collections for research and society. Bioscience 54:66. doi: 10.1641/0006-3568(2004)054[0066:TVOMCF]2.0.CO;2
Szabó, P. (2010). Why history matters in ecology: an interdisciplinary perspective. Environ. Conserv. 37, 380–387. doi: 10.1017/S0376892910000718
Szabó, P., and Hédl, R. (2011). Advancing the integration of history and ecology for conservation. Conserv. Biol. 25, 680–687. doi: 10.1111/j.1523-1739.2011.01710.x
Tait, M. G. (2005). Implementing geoportals: applications of distributed GIS. Comput. Environ. Urban Syst. 29, 33–47. doi: 10.1016/S0198-9715(04)00047-X
The University of Chicago (2006). Uncovering New Chicago Archives Project (UNCAP): Models for Library, Faculty, Student Collaboration.
Thorne, J. H., Morgan, B. J., and Kennedy, J. A. (2008). Vegetation change over sixty years in the central sierra nevada, California, USA. Madroño 55, 223–237. doi: 10.3120/0024-9637-55.3.223
Tingley, M. W., and Beissinger, S. R. (2009). Detecting range shifts from historical species occurrences: new perspectives on old data. Trends Ecol. Evol. 24, 625–633. doi: 10.1016/j.tree.2009.05.009
Van Noorden, R. (2013). Data-sharing: everything on display. Nature 500, 243–245. doi: 10.1038/nj7461-243a
Waide, R. B., Brunt, J. W., and Servilla, M. S. (2017). Demystifying the landscape of ecological data repositories in the United States. Bioscience 67, 1044–1051. doi: 10.1093/biosci/bix117
Wallis, J. C., Rolando, E., and Borgman, C. L. (2013). If we share data, will anyone use them? Data sharing and reuse in the long tail of science and technology. PLoS ONE 8:e67332. doi: 10.1371/journal.pone.0067332
Watson, S. J., Luck, G. W., Spooner, P. G., and Watson, D. M. (2014). Land-use change: incorporating the frequency, sequence, time span, and magnitude of changes into ecological research. Front. Ecol. Environ. 12, 241–249. doi: 10.1890/130097
White, H. (2017). Lindcove REC: developing citrus varieties resistant to huanglongbing disease. Calif. Agric. 71, 18–20. doi: 10.3733/ca.2017a0004
Wieslander, A. E. (1961). California's Vegetation Maps: Recent Advances in Botany. Toronto, ON: University of Toronto Press.
Wilkinson, M. D., Dumontier, M., Aalbersberg, I. J., Appleton, G., Axton, M., Baak, A., et al. (2016). The FAIR Guiding Principles for scientific data management and stewardship. Sci. Data 3:160018. doi: 10.1038/sdata.2016.18
Wilson, G., Bryan, J., Cranston, K., Kitzes, J., Nederbragt, L., and Teal, T. K. (2017). Good enough practices in scientific computing. PLoS Comput. Biol. 13:e1005510. doi: 10.1371/journal.pcbi.1005510
Wolkovich, E. M., Regetz, J., and O'Connor, M. I. (2012). Advances in global change research require open science by individual researchers. Glob. Chang. Biol. 18, 2102–2110. doi: 10.1111/j.1365-2486.2012.02693.x
Wright, D. J. (2016). Toward a digital resilience. Elem. Sci. Anth. 4:82. doi: 10.12952/journal.elementa.000082
Wright, J. (2014). Paper vs. Electronic: The Not-So-Final Battle [WWW Document]. Smithsonian Institution Archives. Available online at: https://siarchives.si.edu/blog/paper-vs-electronic-not-so-final-battle [Accessed November 3, 2018].
Keywords: dark data, data science, historical data, field stations, open data
Citation: Easterday K, Paulson T, DasMohapatra P, Alagona P, Feirer S and Kelly M (2018) From the Field to the Cloud: A Review of Three Approaches to Sharing Historical Data From Field Stations Using Principles From Data Science. Front. Environ. Sci. 6:88. doi: 10.3389/fenvs.2018.00088
Received: 17 May 2018; Accepted: 20 July 2018;
Published: 01 October 2018.
Edited by:
Peng Liu, Institute of Remote Sensing and Digital Earth (CAS), ChinaReviewed by:
Americo Montiel, University of Magallanes, ChileClive Reginald McMahon, Sydney Institute of Marine Science, Australia
Copyright © 2018 Easterday, Paulson, DasMohapatra, Alagona, Feirer and Kelly. This is an open-access article distributed under the terms of the Creative Commons Attribution License (CC BY). The use, distribution or reproduction in other forums is permitted, provided the original author(s) and the copyright owner(s) are credited and that the original publication in this journal is cited, in accordance with accepted academic practice. No use, distribution or reproduction is permitted which does not comply with these terms.
*Correspondence: Maggi Kelly, maggi@berkeley.edu