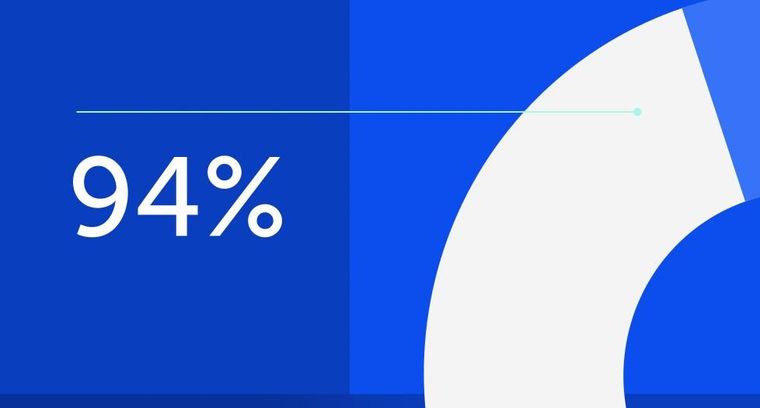
94% of researchers rate our articles as excellent or good
Learn more about the work of our research integrity team to safeguard the quality of each article we publish.
Find out more
ORIGINAL RESEARCH article
Front. Environ. Sci., 04 June 2018
Sec. Agroecology
Volume 6 - 2018 | https://doi.org/10.3389/fenvs.2018.00040
The majority of soil organic nitrogen (N) is bound in protein-like compounds and therefore its proteolysis in peptides and amino acids is considered the initial and rate limiting step of N mineralization. Proteolysis of N bound in organic fertilizer and subsequent provisioning for crops is a central element in agro-ecological intensification. Long-term farming system effects on N provisioning from organic fertilizer to crops and its underlying functional microbial communities were analyzed in experiments conducted in soils from the “DOK” system comparison trial (bio-Dynamic, bio-Organic, and “Konventionell”) subjected to optimal and future projected drought scenarios. A plant nutrition experiment using 15N labeled lupine as a fertilizer (green manure) identified 30% higher amounts of N derived from fertilizer in ryegrass grown on organically compared to conventionally managed soil, but only when subjected to dry conditions. A second experiment, also amended with lupine green manure, assessed the effect of farming system and drought stress on N cycling microbes with a focus on alkaline (apr) and neutral (npr) metallopeptidase encoding microbial communities. apr encoding microbial communities were more strongly affected by farming system and water treatment than npr encoding communities. Differences in structure and diversity of apr encoding microbial communities showed concomitant patterns with distinct N provisioning from organic fertilizer in the plant nutrition experiment. It is suggested that conventionally managed systems are less capable in maintaining diversity and initial structure of apr encoding microbial communities when subjected to drought scenarios. Overall, we demonstrated organically managed soils to provide a more stable N provisioning potential from organic fertilizer under future drought scenarios, likely facilitated by a distinct and more adaptive proteolytic microbial community. This work contributes to an in-depth comprehension of yet poorly studied fundamental soil processes and helps developing strategies to maintain a versatile and functioning microbial community in a rapidly changing environment.
In agricultural systems, microbial communities play an important role due to their involvement in various soil processes and functions such as nutrient transformation and release (Falkowski et al., 2008), pest and disease regulation (Garbeva et al., 2004) as well as erosion control (Lynch and Bragg, 1985; Rillig and Mummey, 2006). Microbes and their functioning are strongly affected by soil moisture content and thus precipitation. Across the globe, extreme weather events are prognosticated to become more intense and more often (IPCC, 2014) and thus, water scarcity but also floods will have direct and indirect effects on crop yields and their nutritional values, threatening agricultural production. The conversion of natural areas into agricultural land, strongly affects the microbiome and its functioning (Nsabimana et al., 2004; Cookson et al., 2007; Ding et al., 2007; Jangid et al., 2008). Furthermore, different kind of agricultural intensities and practices affect the microbiome and its functioning to different extents (Jangid et al., 2008; Hartmann et al., 2015). A previously conducted global systematic review revealed higher microbial abundance and activity as well as different microbial community structures in organic compared to conventional farming systems (Lori et al., 2017). With respect to climate change, highly diverse and thus adaptive microbial communities will be required to sustain microbial-mediated processes and ecosystem services (Emmerling et al., 2002; Van Der Heijden et al., 2008; Wagg et al., 2014; Nielsen et al., 2015; Bender et al., 2016) in order to produce sufficient food, feed, and fibers for the continuously growing human population.
Nitrogen (N) is a crucial element for plant growth. It is one of the most limiting nutrients in agroecosystems (Elser et al., 2007; Meisinger et al., 2008). Nowadays synthetic mineral N fertilizer are rather cheap in relation to the extra yield they contribute to. Therefore, the amount of applied N often exceeds plant uptake leading to severe environmental and human health problems (Galloway et al., 2008; Fowler et al., 2013). Thus, alternative fertilization strategies are required, which lead to sufficient yield by efficient use while simultaneously not further setting environmental health at risk but rather improve already distorted land. Ecological intensification aims to integrate ecological processes into management-strategies in order to enhance ecosystem services and to reduce anthropogenic inputs in agricultural systems (Bommarco et al., 2013)—herein often N bound in organic fertilizers are used to sustain plant N demand.
The soil organic N pool consists of complex forms and only a small share is directly available to plants and microbes. Soil organic N dynamics are defined by mineralization, bacterial immobilization, leaching, plant uptake, gaseous losses, as well as adsorption (Murphy et al., 2000, Figure 1). A large fraction of soil organic N is bound in proteins (Lipson and Näsholm, 2001) and thus its release into bioavailable molecules is of great ecological importance. Proteolysis is the first step of protein mineralisation and rather slow compared to further downstream acting amino acid mineralisation (Jan et al., 2009). Consequently proteolysis is considered to be the rate limiting step of N mineralization (Schimel and Bennett, 2004; Vranova et al., 2013). Proteases are ubiquitous and can be found across all kingdoms of life. The herein used term “protease encoding microbial communities” describes soil microorganisms producing extracellular proteases catalyzing the hydrolysis of peptide bonds in proteins found in soil (also see Baraniya et al., 2016).
Figure 1. Simplified scheme of soil microbial mediated nitrogen mineralization. Organic nitrogen (Norg) is mineralized into mineral nitrogen (Nmin) via different steps and enzymes. Functional genes encoding for the respective enzymes are highlighted in red and abbreviated as alkaline metallopeptidase (apr), neutral metallopeptidase (npr), urease (ureC), bacterial ammonia-oxidase (amoAOB), archaeal ammonia-oxidase (amoAOA). Nitrogen losses like leaching or N2O production are not considered in this scheme. Microbial bound nitrogen (Nmic), dissolved organic nitrogen (DON) and mineral nitrogen (Nmin = + + ) represent the labile N pool (Nlabile).
Green manures are crops primarily used as organic fertilizer soil amendment and thus nutrient source for subsequent crops. Especially leguminous green manures with their capacity of biological N fixation and their consequently low C/N ratio in early growth stages, offer a large quantity of organically bound N. Soils receiving organic fertilizers, such as green manures, heavily depend on proteolysis to provide plant-available N for crop growth (Jones and Kielland, 2002) and thus indigenous extracellular protease encoding microbial communities play an important role in regulating proteolysis and subsequent N transformations (Figure 1). Other N containing molecules in soil such as chitin and peptidoglycan in fungal and bacterial cell walls, respectively, or nucleotides in DNA can be broken down into smaller fragments and release plant available N too (see Schulten and Schnitzer, 1998; Geisseler et al., 2010).
Molecular tools assessing abundance and phylogeny of functional microbial communities are nowadays widely used to unravel the genetic diversity related to soil processes and functions. Oligonucleotides targeting genes encoding for the dominant bacterial extracellular alkaline (apr) and neutral (npr) metallopeptidase (Bach et al., 2001) are available to examine differences in abundance and phylogeny of protease encoding microbial communities in response to management practices, fertilizers, or soil types (Bach et al., 2002; Fuka et al., 2007; Sakurai et al., 2007; Baraniya et al., 2016). The first in-depth study on apr and npr encoding microbial communities assessed abundance and structure of apr and npr in soils amended with different N fertilizers (Sakurai et al., 2007). They measured enhanced proteolytic activity in soils supplied with organic and synthetic fertilizers and observed that fertilizer type affects apr and npr community structure analyzed by denaturing gradient gel electrophoresis (DGGE). Thereafter, temporal and spatial variability of npr abundance and phylogeny in arable fields was analyzed using terminal restriction fragment length polymorphism (T-RFLP) (Fuka et al., 2008, 2009). Recently, Baraniya et al. (2016) published the first amplicon sequencing data on the structure of protease encoding microbial communities. They identified higher apr and npr abundance and diversity in the rhizosphere of a maize line with higher N use efficiency compared to a maize line with lower N use efficiency. Besides apr and npr, several functional genes encoding for enzymes but acting at different stages in the N cycle, such as ureC (Gresham et al., 2007), amoAOA (Leininger et al., 2006) or amoAOB (Rotthauwe et al., 1997), are available, but of minor interest in the present study since they do not act on the “bottleneck” of the concerning soil process.
To date, the impact of long-term management on N provisioning from organic fertilizer and its underlying functional microbial communities under future projected drought scenarios has not been thoroughly investigated. We consider knowledge gain about N provisioning from organic fertilizers and its underlying processes to be highly relevant in order to develop fertilization strategies in a rapidly changing environment. The current work simulates rainfall variability in controlled environment experiments using soil from the “DOK” farming system comparison trial (bio-Dynamic, bio-Organic, and “Konventionell”; Mäder et al., 2002). Previous work analyzing the microbiome of DOK soil, based on 16S sequencing, identified distinct microbial community compositions between farming systems (Hartmann et al., 2015). Whether or not these differences also translate into different functionalities facing climate changed induced rainfall variability, is not yet investigated.
We therefore aimed to analyse the impact of long-term management history on N provisioning from organic fertilizer to crops and its underlying N-cycling microbes, especially protease encoding microbial communities, under optimal but also future projected drought scenarios. Two similar but individual experiments were carried out to eliminate side effects of differently well growing plants and their exudates on N cycling microbial communities:
i) The plant nutrition experiment assessed N provisioning for the crop from 15N labeled green manure in differently managed soils subjected to rainfall scenarios
ii) The incubation experiment assessed differences of N-cycling microbes, especially protease encoding microbial communities, and biogeochemical N pools in differently managed soils subjected to rainfall scenarios
We hypothesized differences in N provisioning from organic sources to plants in organically and conventionally managed soil to exist with stronger effects under drought scenarios. This phenomena might be explained by the well-known insurance hypothesis claiming that a higher species diversity insures ecosystems against declines in functioning when facing disturbances because many species enhance the guarantee that some will maintain functioning even though others may fail (Naeem and Li, 1997; Yachi and Loreau, 1999). We thus further hypothesized different structures/diversities of protease encoding microbial communities in organically and conventionally managed soils to exist which will be affected to different extends when facing disturbances, such as drought scenarios.
Soil material was collected from the DOK farming system comparison trial in Therwil, Switzerland (47°30′N 7°33′E), initiated in 1978. The DOK experiment comprises five different farming systems mainly varying in fertilization practice and plant protection. The current experiment is based on soils deriving from the bioorganic (BIOORG) and the conventional mineral (CONMIN) farming systems. The BIOORG system exclusively receives organic fertilizers while the CONMIN system exclusively receives mineral fertilizers. Plant protection in BIOORG is managed using mechanical and biological treatments while in CONMIN pesticides and synthetic plant growth regulators are applied (for further information see Mäder et al., 2002 and Fließbach et al., 2007). BIOORG and CONMIN are hereinafter referred to as organic (ORG) and conventional (CON), respectively. Bulk soil samples of 10–14 pooled cores per replicate (2.5 cm diameter, 0–15 cm depth) were collected shortly after winter wheat harvest in summer 2015 with soybean as pre-crop. During winter wheat growth, both systems received an equal total amount of 120 kg N per hectare though applied in different forms (slurry and calcium ammonium nitrate, respectively) and slightly different split dosages. Collected samples were sieved to 2 mm and stored at 4°C until experimental start. Soil across all treatments is composed of 16% clay, 70% silt, and 14% sand. Mean annual temperature and mean annual precipitation is 9.5°C and 792 mm, respectively.
Two hundred grams dry weight equivalents of soil were filled into pots (Ø 9 cm) and amended with 1,500 mg dried 15N labeled lupine litter (2.64% N per dry matter) homogenized into the soil (resulting in 39 mg N input per pot). Ryegrass seeds (Lolium multiflorum, 4n, var. Gemini) were uniformly distributed on the soil surface and covered with a thin layer of vermiculite to prevent moisture loss during germination. Six replicates per treatment (fully randomized) were established and adjusted to their desired water regime by adding sterile deionized water (dH2O). WET treatments were set at 80% of the soils maximum water holding capacity (mWHC) and DRY at 20% of the soils mWHC. Plants were grown for 56 days in a climate chamber with a day/night cycle of 10/14 h, 20–22°C and 60% relative humidity. Pots were regularly weighed to monitor water losses and adjusted by adding dH2O. Shoots were harvested at day 28 and 56 while the root system was harvested exclusively at day 56. Plant material was dried for 48 h at 45°C and ball milled for N analyses.
To account for natural 15N abundance, an identical side experiment was established using non-labeled litter.
Based on dry matter biomass and total N concentration, the total N content of the different plant parts (Nplantpart) was calculated. The calculations were done separately for the different shoot cuts and roots. Total N uptake of ray grass, total N derived from fertilizer (Ndff) and recovery of N (REC) was calculated as the sum of shoot cuts and roots.The amount of N derived from 15N-labeled green manure fertilizer in different plant parts (Ndffplantpart) was calculated according Equation 1 (Hauck and Bremner, 1976).
where Nplantpart corresponds to the total amount N (mg pot−1) in the corresponding plant parts, 15Nexplantpart corresponds to atom% 15N excess (%) in the different plant parts and 15Nexfertilizer corresponds to atom% 15N excess (%) of the labeled fertilizer.
The fraction of N derived from the labeled fertilizer (%) is expressed as:
The sum of the different Ndffplantpart (mg pot−1) equals N derived from fertilizer in the total plant [Ndff (mg pot−1)] and the sum of the different Nplantpart equals the total plant N [Nplant (mg)].
N derived from soil (Ndfs) was calculated as:
The fraction of total N recovered (REC) from the applied fertilizer was calculated as:
Where Ninput is the amount of N applied as fertilizer (mg).
15N labeled lupine litter (Lupinus alba) (2.6 atom% 15N) was produced by supplying one week old lupine seedlings growing on a sand/perlite substrate with a 15N amended, modified Hoagland solution (Gamborg and Wetter, 1975) containing K15NO3 and 15NH4Cl. Afterwards, shoots were harvested, dried at 50°C and milled.
Hundred gram dry weight equivalents of soil were gently filled into PVC incubation containers (Ø 6.3 cm). The lids contained holes allowing aeration as well as the bottoms, which were covered by a membrane to prevent soil losses. Soil in each container was amended with 750 mg dried and milled lupine litter (2.64% N) (resulting in 195 mg N input per kilogram dry weight soil). Four replicates per treatment (fully randomized) were established and adjusted to their desired amount of mWHC by adding dH2O. WET treatments were set at 80% of the soils mWHC and DRY at 20% of the soils mWHC. Containers were incubated for 56 days at 20–22°C and 50% humidity in the dark, regularly weighed to monitor water losses and adjusted accordingly by adding dH2O. Total nitrogen (Ntot), total carbon (Ctot), mineral nitrogen (Nmin = + + ), microbial carbon (Cmic), microbial nitrogen (Nmic), and dissolved organic nitrogen (DON) were measured at the start (T0) and at the end (T1) of the incubation period. Subsamples of T0 and T1 for DNA analyses were stored at −20°C until use. In order to assess N losses via N2O emissions and to monitor microbial respiration, gas samples were taken regularly as described in Soil Microbial Activity.
Ctot and Ntot was determined by combustion (dried at 60°C, milled, CN Vario Max; Elementar Analysensysteme GmbH, Hanau, Germany). Nmin and DON were extracted using 0.01 M CaCl2 (1:4 w/v) followed by filtration (MN 619EH; Macherey-Nagel, Düren, Germany) and photospectrometrical determination of ammonium and nitrate (SAN-plus Segmented Flow Analyzer; Skalar Analytical B.V., Breda, Netherlands) for Nmin while DON was analyzed on a TOC-TNb Analysator (Analytik Jena multi N/C 2100S, Analytik Jena, Jena, Germany). Fluxes (ΔN) of the respective N pools in the incubation experiment were calculated as the difference between T1 and T0. Approximated proteolysis of organic fertilizer amendment was assessed via differences in the labile N pool (Nlabile = Nmic +Nmin+ DON) at the end and the beginning of the incubation (Nlabile−T1 and Nlabile−T0), abbreviated as ΔNlabile. ΔNlabile represents an estimation about how much organically bound N underwent proteolysis during the incubation and thus ended up in the labile pool. It serves as an approximated potential of net proteolysis and does not reflect changes in plant available N or classical proteolytic potentials. Soil pHH2O was assessed in a 1:2.5 (w/v) dilution with dH2O.
Two milligram plant tissue was weighed in tin capsules (Tin capsules for solids, 3.2 × 4 mm, SÄNTIS Analytical AG, Teufen, Switzerland) to determine 15N abundance and N contents with an Isotope Ratio Mass Spectrometer/IRMS (delta V Advantage, Thermo Fisher, Dreieich, Germany) coupled to an Elemental Analyzer (Euro EA, Eurovector, Milano, Italy) at the Helmholtz Zentrum Munich, Germany.
Cmic and Nmic were assessed by the chloroform fumigation method (CFE) according to Vance et al. (Vance et al., 1987).
Incubation containers were placed into 1 L Mason jars, which were closed by airtight lids. To assess gas fluxes, four gas samples (20 mL) were taken within one hour from the headspace of the Mason jars with a syringe, were injected into pre-evacuated vials and subsequently analyzed by GC (7890A; Agilent Technologies, Santa Clara, CA). CO2 concentrations of samples were determined with a flame ionization detector (FID) after reduction to CH4 by a methanizer and N2O with an electron capture detector (μECD). Gas fluxes were calculated using a linear model. Trapezoidal integration method was used to calculate cumulative fluxes (mg CO2-C kg−1 dry soil):
where t = sampling time (h), f = gas flux (mg kg−1 h−1) and n = number of sampling events.
DNA was isolated from frozen samples using the FastDNA SPIN Kit for Soil and the FastPrep Instrument (MP Biomedicals, Californiq, USA) following the manufacturers' instruction. All samples were cleaned with the OneStep™ PCR Inhibitor Removal Kit (Zymo Research, California, USA). DNA extraction efficiency was assessed by spiking samples with an exact amount of plasmid carrying an artificial sequence prior to DNA extraction according to Thonar et al. (2012). After extraction, the amount of recovered spike was assessed similarly to the functional genes (see section Functional Gene Abundance) to calculate the extraction efficiency. DNA concentrations were assessed using Qubit Fluorometer (Thermo Fisher Scientific, Waltham, USA).
Abundance of functional genes was assessed by quantitative polymerase chain reaction (qPCR). Prior to qPCR, each oligonucleotide set was optimized using different soil dilutions and annealing temperatures to reach R2 > 0.999 and efficiencies between 0.8 and 1. qPCR reactions were performed in 12 μl using a SYBR green approach (Kapa SYBR® Fast qPCR Kit Master Mix (2×) Universal; Kapa Biosystems, Wilmington, MA) on a Rotor-Gene Q platform (Rotor-Gene Q; QIAGEN, Venlo, Netherlands). Master mix composition, cycling profiles and oligonucleotide sequences are listed in SI Table 1. Biological replicates were analyzed in technical duplicates. Negative controls and serial dilutions of plasmids carrying the gene of interest were included as triplicates in every qPCR run to calculate standard curves for absolute quantification. Size and quality of generated amplicons were controlled by melting curve analyses and gel electrophoresis on agarose gels.
DNA extracts from the incubation experiment were employed for Illumina sequencing by a two-step PCR approach. The first PCR using fluidigm-tagged apr and npr primers was performed in triplicates and subsequently pooled for visualization on agarose gels (1.75%). Bands were gel purified using the QIAquick PCR purification kit (QIAGEN). Primers, master mixes and cycling conditions are listed in SI Table 1. The second PCR and library preparation was performed at the Genome Quebec Innovation Center (Montreal, Canada) according to the amplicon guidelines provided by Illumina (San Diego, CA, USA). The library was sequenced on an Illumina MiSeq sequencing system using the 2 × 250 bp Reagent Kit v2 (Illumina, San Diego, CA, USA). Quality of sequencing data was assessed using FastQC (Andrews, 2010) and MultiQC (Ewels et al., 2016). Forward and reverse sequences were merged using Flash (Magoč and Salzberg, 2011) with an overlap of up to 250 base pairs (SI Table 2). Taxonomic annotation of individual sequences and rarefaction curves (SI Figure 1) were performed in MGX (Jaenicke et al., 2018) based on a homology search with NCBI GenBank as reference database (E-Value cutoff: 1e-5, minimum identity 0.95). Community structure and diversity was computed based on taxonomic classifications on species rank. In addition, an OTU analysis was performed to unravel the inner structure of the uncultured bacteria subset. UPARSE (Edgar, 2013) was used for OTU clustering on 97% similarity (SI Table 3). All raw sequences were deposited at ENA (https://www.ebi.ac.uk/ena) under the accession number PRJEB24038.
It has to be noted, that amplicon based information on community structure is never free of bias. Primers for functional genes, even though highly degenerated, may miss certain sequences or even different genes encoding for proteins with a similar function. PCR, library preparation and annotation of sequences come with biases, too (Van Dijk et al., 2014; Schirmer et al., 2015). In general, direct proof that a given sequence is associated with a microbial taxon or species is hard to obtain and, therefore, specific species names mentioned in this manuscript must be treated with caution. Whenever species are named, it is in reference to microbes that contain the respective annotated apr and npr gene sequence. Furthermore, the presence of chimeric sequences and horizontal gene transfer can not be excluded.
Two-way ANOVAs (JMP®, Version 11.2. SAS Institute Inc., NC) were applied to detect farming system (FS), water regime (WR) and FSxWR effects for the biochemical, gene abundance and diversity index datasets. Tuckey post hoc tests with a significance level of p = 0.05 identified differences between treatments. Normality of data was assessed by Shapiro-Wilk's Goodness-of-Fit test. Relationship between the abundance of functional genes and the corresponding fluxes in N pools were assessed using Spearman correlation. All graphs except the nonmetric multidimensional scaling (NMDS) ordination and heat maps were designed in SigmaPlot (Version 12.5 Systat Software, San Jose, CA).
Diversity was assessed based on the Shannon index using the vegan package (Oksanen et al., 2017). Structure of protease-encoding microbial communities was visualized using NMDS plots based on Bray-Curtis distance metrics using ggplot2 (Wickings et al., 2012) and vegan package (Oksanen et al., 2017) run on R 3.4.1 and RStudio1.0.153. FS, WR, and FSxWR effects on community structure were analyzed by permutational multivariate analysis of variance (PERMANOVA) using Bray-Curtis distance metrics implicated in the ADONIS function (vegan package). PairwiseAdonis (Arbizu, 2017), a multilevel pairwise comparison using ADONIS, was used to test for differences between FS within WRs. Species driving differentiation between treatments were assessed using the similarity percentages (SIMPER) function from the vegan package with a cumsum cut-off at 80%. Herewith identified species were fitted with the envfit function (vegan package) on the NMDS plots.
Soil biochemical parameters differed prior to incubation in Ntot, Nmic, and Cmic pools but not in Ctot (Table 1) when comparing the two farming systems. Sieved soils of both farming systems had initially a mWHC of 52 ± 0.7 g H2O per 100 g dry soil and water regimes were applied accordingly. No N losses occurred via leaching and none or only marginal N losses via gaseous emissions are assumed since measured N2O emission were below the detection limit (data not shown).
Table 1. Soil biochemical properties of organic and conventional farming systems at the start of the experiments after lupine litter amendments.
Plants growing on organically and conventionally managed soils produced equal amounts of biomass (shoots + roots) after 56 days under WET scenarios. Under DRY scenarios, biomass was reduced (p < 0.001), although plants grown on organically managed soil produced 23% more than plants grown on conventionally managed soil (Figure 2). Nitrogen derived from fertilizer (Ndff) measured in the plant biomass (shoots + roots) was taken as proxy for N provisioning from organic fertilizer (green manure). Ndff was significantly affected by WRxFS (p < 0.01) and exclusively differed between farming systems under DRY scenarios with 30% higher values in plants grown on organically managed soil (Figure 2). No significant differences between FS were identified under WET scenarios.
Figure 2. Effects of farming system (FS) and water regime (WR) on total plant dry weight biomass (A), total plant nitrogen (N) (B), N derived from fertilizer (Ndff) (C) and total N recovered from fertilizer (REC) (D) of ryegrass (shoot and root) after 15N labeled green manure amendment (plant nutrition experiment). Ryegrass was grown in organically (ORG) and conventionally (CON) managed soils for 56 days under controlled WET and DRY conditions. Two-way ANOVA and Tukey post hoc tests were applied to identify differences between treatments indicated with different letters. n = 6; error bar represent standard errors.
Overall, N recovery by the plants during the 56 day growth period was around 9% for the WET and 6.3 and 4.9% respectively for the organic and conventional DRY treatments (Figure 2). Total plant N content was significantly affected by FS and WR (p < 0.001, p < 0.001 respectively) with highest values in plants grown on organically managed soil under WET (16.2 ± 0.3 mg per pot) followed by conventionally managed soil under WET (12.6 ± 0.1 mg per pot), organically managed soil under DRY (14.78 ± 0.4 mg per pot) and conventionally managed soil under DRY (10.1 ± 0.2 mg per pot).
Cumulated CO2 emissions, as a proxy for microbial activity during the 56 days of incubation, were affected by WR (p < 0.001), with highest values in the conventional WET treatment followed by organic WET. Soil subjected to DRY scenarios showed around 40% lower CO2 emissions compared to soil subjected to WET with no difference between farming systems (SI Table 4). A control treatment with no lupine amendment showed on average across all treatments approximately six times lower CO2 emissions (data not shown).
Abundance of apr and npr was significantly affected only by WR (p < 0.05 and p < 0.01, respectively) with higher abundances under WET conditions (SI Table 4). Overall, apr encoding microbes were more abundant than npr encoding microbes. Abundances of functional genes involved in more downstream acting transformations in the N cycle, namely ureC, amoAOA, and amoAOB, were majorly affected by WR rather than FS (SI Table 4).
When correlating the abundance of apr and npr to the biogeochemical proxy for net proteolysis (ΔNlabile), a positive relationship was identified, as well as for net mineralization and net nitrification (Table 2). No correlation of biogeochemical N fluxes to amoAOB and ureC abundance was observable and no relationship between any of the functional genes and net microbial N immobilization was present (Table 2).
Since apr and npr represent functional genes acting on the initial and rate limiting step of proteinaceous N-mineralization (proteolysis), in depth analyses were undertaken in this regard. Illumina sequencing of both, apr and npr, revealed significant WRxFS effects on community structure dissimilarities observed by PERMANOVA (p < 0.05, Table 3). A significant FS effect within DRY treatments was identified for apr (p = 0.020, Table 3). NMDS ordinated apr community structure was affected more strongly by treatments than npr community structure (Figure 3). Based on SIMPER analysis, most influential “species” (organisms carrying the sequence annotated to the respective species) for the distinction between treatments (SI Table 5) were identified and fitted to the NMDS ordination displaying similar species to be associated to WRs as it was observable in the heatmap based on relative abundance (Figure 4).
Table 3. Effects of farming system (FS) and water regime (WR) on alkaline metallopeptidase (apr) and neutral metallopeptidase (npr) encoding microbial community structure.
Figure 3. Nonmetric multidimensional scaling (NMDS) ordination of alkaline metallopeptidase (apr) and neutral metallopeptidase (npr) encoding microbial communities based on Bray-Curtis distance metrics (incubation experiment). Treatments are labeled according to the legend with conventional (CON), organic (ORG) and the respective water treatments DRY and WET. Vectors indicate species (organisms carrying the respective sequence annotated to a species) being most influential for the differentiation between treatments (identified via SIMPER analysis).
Figure 4. Relative abundance heatmap of alkaline metallopeptidase (apr) and neutral metallopeptidase (npr) encoding microbial communities (incubation experiment). Only species (or organisms carrying the respective sequence annotated to a species) being more abundant than 0.5% are included in the heatmaps. Samples were clustered based on Bray-Curtis similarities (group-average linkage). Samples (y-axis) are colored according to the treatments conventional (CON), organic (ORG) and the respective water treatments DRY and WET. Color from white to blue indicates higher abundance.
At the start of the incubation, apr encoding microbial communities in organically and conventionally farmed soils were comparably diverse based on Shannon index (Table 4). At the end of the incubation, diversity in apr encoding microbial communities was significantly affected by FSxWR (p < 0.001). When comparing FS effects within WRs, higher apr diversity was identified in organically compared to conventionally managed soils but exclusively under DRY scenarios (p < 0.001). Contrary to apr, npr encoding microbial communities were not affected by FS, WR, and FSxWR when applying the Shannon index (Table 4). In addition, no differences were found between FS within WRs. Sequencing of apr revealed a total of two hundred different “species” (or organisms carrying the sequence annotated to the respective species) with Pseudomonas sp. GR6 02, Pseudomonas fluorescens, Ralstonia solanacearum but also the sum of uncultured bacteria as most abundant (Figure 4). Organisms carrying the gene annotated to Pseudomonas sp. GR6 02 were associated to DRY treatments with highest relative abundances in conventional soil (Figure 4, SI Table 5). Sequences annotated to R. solanacearum and the sum of all uncultured bacteria were affected by WR with lower relative abundances under DRY compared to WET scenarios; additionally, a FS effect within DRY treatments was present (Figure 4, SI Table 5). Sequencing of npr encoding microbial communities revealed in total 103 different “species” strongly dominated by Bacillus sp. IHB 7164 and the sum of uncultured bacteria (Figure 4). Comparably low FS and WR effects on relative abundance were observed.
Table 4. Diversity of alkaline metallopeptidase (apr) and neutral metallopeptidase (npr) encoding microbial communities based on Shannon index.
The sum of uncultured bacteria made up a substantial part of apr and npr encoding microbial communities and thus in-depth analyses were performed to unravel its inner structures. For apr and npr 164 and 159 OTUs (97%) were identified respectively. npr sequences were dominated by a few OTUs present in all samples while for apr no such effect was observable. PERMANOVA only revealed FSxWR effects on the inner structure of npr-encoding uncultured bacteria (p = 0.028) but no FS effects within WRs (SI Table 6). No treatment effects were detected on the inner structure of apr encoding uncultured bacteria. Shannon diversity of apr and npr encoding uncultured bacteria was only affected by WR (apr: p = 0.016, npr: p < 0.001, respectively) while FS effects within WRs were not observable (SI Table 7).
Given climate change induced rainfall variability and alternative fertilization strategies, it is of importance to study und understand N provisioning from organic fertilizer sources to plants and its underlying mechanisms. Classical enzyme assays analyzing proteolytic activities/potentials are run under optimal water conditions with organic N substrates of low complexity (Ladd and Butler, 1972). They rather inform about a potential under optimal conditions and are thus of limited value to answer the herein posed questions. The present study therefore focused on N provisioning from a complex organic N source to plants and differences in the underlying functional microbial communities in contrasting soils.
Enhanced proteolytic activity and net mineralization measured in soil potential assays can be misleading proxies for plant N availability (Schimel and Bennett, 2004), because after proteolysis, N can be immobilized into microbial biomass and even end up in the organic matter pool when microbes lyse. To circumvent this issue, a plant nutrition experiment using 15N labeled organic green manure was conducted. This experimental set up allowed us to trace and quantify the amount of organically bound N from the green manure transformed by microbial processes and taken up by the crop. It is likely, that also a small proportion of inorganic or bioavailable organic 15N was amended with the green manure and ended up in the plant without having undergone proteolysis beforehand. Though, this effect would have occurred in both soils amended identically and can thus be neglected. We identified around 30% higher values of N derived from organic fertilizer in plants grown on organically managed soil compared to plants grown on conventionally managed soil under DRY scenarios; no difference was observable under optimal soil water conditions (Figure 2). Total N accumulation in plants showed a slightly different pattern with higher values in plants grown on organically managed soil throughout WRs. This is likely due to a higher total soil N content prior to the start of the experiment in organically managed soil (Table 1) and thus reservoirs for N other than fertilizer input have had been bigger. Overall N recovery from fertilizer was rather low in the current experiment. One explanation is the high fertilization dose (approximately 234 kg N per hectare and year) in relation to the growing period of 56 days. Release of organically bound N by microbes, which is in general supposed to be slow (Tyson and Cabrera, 1993; Whitmore and Schröder, 1996; Sørensen and Amato, 2002; Gutser et al., 2005), might have been limited by time. Furthermore around four times higher N contents of N derived from soil than fertilizer were detected in the ryegrass mainly due to residual organic N reservoirs as carry over effect of the pre crop soybean. A prolonged duration of the experimental growth period, allowing to deplete existing organic N reservoirs on one side and on the other side allowing microbes to degrade and provide more N, might have pronounced the observed FS effects under DRY scenarios. Thus, further experiments should consider longer plant growth periods, if possible. However, our findings demonstrated that under DRY conditions likely microbial communities in organically managed soils were better able to sustain proteolysis and make organically bound N of the green manure plant available compared to conventionally managed soil. A previous field experiment in the DOK trial using 15N labeled sheep manure conducted by Bosshard et al. (2009) did not identify differences between organic and conventional farming systems in regard to plant N uptake derived from organic fertilizer. They identified N recovery fractions of applied sheep faces to be around 15% after the first vegetation period and N derived from soil values being around two and a half times bigger than N derived from fertilizer. The experiment was performed under normal rain scenario and is thus more in line with our findings under WET conditions (Figure 2). A field experiment using 15N labeled (green) manure combined with rainout shelters (such as Kundel et al., 2018) might be valuable in order to study N provisioning from organic fertilizer to crops under future projected drought scenarios in a less artificial set-up, including intact soil physics and higher trophic levels.
Overall, only few FS effects on abundance of N cycling functional genes were identified (SI Table 4). Correlations between functional genes and their associated biochemical fluxes yielded in positive results for apr, npr, and amoAOA (Table 2). A correlation between the proteolytic activity/potential and the abundance of apr was already shown by Brankatschk et al. (2011), who worked with soil from glacier forfields, whereas Fuka et al. (2008) identified a positive correlation between proteolytic potential and npr abundance in arable soil. In the present experiment, abundance of other functional genes involved in the N cycle but not directly related to proteolysis (ureC, amoAOA, amoAOB) were also assessed and no significant correlation other than amoAOA to approximated net proteolysis and net mineralization was detected (Table 4). However, gene abundance itself is often hardly able to accurately predict biogeochemical reactions (Rocca et al., 2015).
In order to gain in depth information about functional microbial communities directly being involved in proteolysis and likely subsequent N provisioning, apr and npr encoding microbial communities were studied regarding their structure and diversity. Our results indicate that the conventionally managed system was less capable in maintaining diversity and initial structure of apr encoding microbial communities under stressful DRY conditions compared to organically managed soils (Figure 3, Tables 3, 4). Overall, npr encoding microbial communities were less affected by FS and WR compared to apr encoding microbial communities (Figures 3, 4). npr encoding microbes' reduced responsiveness to WR might be explained by the domination of Bacillus sp. (or species carrying the sequence annotated to Bacilli) (Figure 4). Bacilli are known to form endospores in order to escape from unfavorable conditions (Nicholson et al., 2000; Nicholson, 2002). With this strategy, Bacilli might survive stressful conditions but remain detectable without contributing to soil processes. Since previous analyses of the DOK microbiome revealed Firmicutes to be associated with farmyard manure receiving systems (Hartmann et al., 2015), a stronger FS effect on npr encoding microbial communities was expected. Reduced “farmyard manure receiving” effects on npr encoding microbial communities are likely explained by another share of the Firmicutes being responsible for the effects observed in (Hartmann et al., 2015) though further analyses are required for clarification.
It has to be noted that analyses of functional genes bear certain risks of bias and should be interpreted carefully. Amplicon sequencing based work can supply information only as good as the oligonucleotides' coverage of the community is. A validation of apr and npr oligonucleotide coverage against the current database, 17 years after they have been designed, might unravel whether the generation of improved oligonucleotides should be considered. Another limitation disempowering the meaning of identified “species” is based on horizontal gene transfer, in which organisms can acquire genes deriving from close but also distantly related organisms (Thomas and Nielsen, 2005; Polz et al., 2013). Furthermore, amplification of highly homologues genes from complex DNA mixture can lead to chimeric sequences and thus false diversity/structure estimates (Gonzalez et al., 2005).
Regardless of the discussed limitations, though treated with caution, the majority of identified species are in line with results from previous work (Rösch et al., 2002; Zehr et al., 2003; Izquierdo and Nüsslein, 2006).
The insurance hypothesis claims that biodiversity provides an insurance or buffering function against environmental fluctuations (Naeem and Li, 1997; Yachi and Loreau, 1999). Species respond differently and thus a higher biodiversity may not be important for maintaining ecosystem functioning at constant conditions but can be of importance under changing conditions. Soil microbial diversity might directly influence ecosystem stability (resistance and resilience) under environmental changes such as rainfall variability as it was already shown for aboveground biodiversity (Isbell et al., 2015). Recently, Philippot et al. (2013) identified that loss of microbial diversity affected functioning of the N cycle, in particular denitrification. Wagg et al. (2014) identified a loss in microbial diversity and abundance to negatively impact soil functioning including N mineralisation. Further studies about the impact of biodiversity losses on other N cycling soil functions are highly needed to fully understand the consequences of this major threat to food security under expected climate change scenarios.
We identified a potential link between apr diversity and N provisioning from organic fertilizer to plants under drought scenarios. Further experimental manipulation of protease encoding microbial community diversity should be undertaken to assess its direct impact on functioning. Microbial wash “dilution and transplant” experiments, controlling for artificial farming system dependant side effects, combined with 15N labeled organic fertilizer and apr/npr sequencing would allow to analyse a direct link between functioning and diversity, might further confirm the insurance hypothesis and help identifying key players potentially serving as new bioeffector candidates. Up to date, there are some bioeffector products available consisting of selected plant growth-promoting rhizobacteria (PGPR) to which also species of the apr and npr encoding community belong. A recently conducted meta-study reported enhanced effectiveness of bioeffectors in arid and semi-arid climates over other climatic regions with bioeffector-mediated yield increases of up to 21% in dry climates compared to 10.1% in continental climates (Schütz et al., 2018). Thus, the identification, characterisation and application of drought-adapted species could harbor a potential to deal with future projected drought scenarios in agricultural systems.
Considering ecological-intensification (Bommarco et al., 2013) and the use of organic fertilizer, it is highly important to deepen our knowledge about release of organically bound N and subsequent provisioning to plants in order to develop plant nutrition strategies ideally supplying crops with N while simultaneously reducing losses. The present study neglected N losses such as e.g., leaching, since watering to 20 and 80% of soils mWHC did not result in drainage. Our work delivered first insights into different functionalities of soils regarding organic N provisioning potential and hereby involved microbial communities as affected by long-term management history combined with future projected drought scenarios. Results of our model study cannot yet directly be translated to agroecosystems but encourage for further work verifying our findings in less artificial systems and other soil types, climatic zones and farming systems. Field experiments (also including rain-out-shelters as described in Kundel et al., 2018) might test weather our findings as well hold true in an applied agronomic context. Furthermore, organic N sources were amended in a rather high fertilization dosage in order to assess potential of N provisioning trough mineralisation, though future field studies should strongly focus on applying fertilisztion dosages in an agronomical context according to the national regulations of the respective crops. Besides climate change induced rainfall variability, also changes in air and thus soil temperature are expected (IPCC, 2014). As differences in soil temperature has already been shown to affect microbial activity (Pietikäinen et al., 2005) and structure (Zogg et al., 1997), changes in temperature imply potential direct and indirect influences on the microbiome and its functioning. Therefore, we suggest further model and field studies assessing the effect of raising temperatures, potentially also combined with water treatments, on microbial mediated N provisioning. Such studies were started in the frame of the EU Biodiversa project SoilClim.
Besides proteolysis, also the breakdown and mineralisation of other N containing (macro-) molecules and biological N fixation contribute to plant nutrition and are important elements in ecological-intensification. Thus, detailed analyses of factors influencing the N fixation potential and microbial communities carrying the relevant nitrogenase gene (nifH) (Rösch et al., 2002; Zehr et al., 2003; Izquierdo and Nüsslein, 2006), are highly encouraged as well.
The raw data supporting the conclusions of this manuscript will be made available by the authors, without undue reservation, to any qualified researcher. All raw DNA sequences are deposited at ENA (https://www.ebi.ac.uk/ena) under the accession number PRJEB24038.
ML, SS, AnG, and PM contributed conception and design of the study; ML and NE conducted the experiments. ML conducted the majority of biochemical and all molecular analyses. FB conducted analyses of 15N plant samples, ML, SJ, and AlG performed bioinformatics analyses, ML and ST performed the statistical analysis; ML wrote the first draft of the manuscript. All authors contributed to manuscript revision, read, and approved the submitted version.
The DOK system comparison, from which the soil samples analyzed originate, is funded by the Swiss Federal Office of Agriculture. The project Sustainable provisioning of multiple ecosystem services in agricultural landscapes (ECO-SERVE) is part of BiodivERsA/FACCE-JPI and was funded by the Swiss National Science Foundation (SNF) National Research Programme (NRP 68) Soil as a resource. AnG received additional funding from the Mercator Foundation Switzerland (Grant No. 2011-0294).
The authors declare that the research was conducted in the absence of any commercial or financial relationships that could be construed as a potential conflict of interest.
Thank goes to the Genome Quebec Innovation Center (Montreal, Canada) and Pierre Lepage for excellent support and execution of Illumina sequencing. Further thanks go to Frank Rasche, University of Hohenheim, who initially supplied us with npr plasmid standards. The bioinformatics support of the BMBF-funded project Bielefeld-Gießen Center for Microbial Bioinformatics—BiGi (grant 031A533) within the German Network for Bioinformatics Infrastructure (de.NBI) is gratefully acknowledged. The authors kindly thank the two reviewers for their constructive feedback.
The Supplementary Material for this article can be found online at: https://www.frontiersin.org/articles/10.3389/fenvs.2018.00040/full#supplementary-material
Andrews, S. (2010). FastQC: A Quality Control Tool For High Throughput Sequence Data. Available online at: http://www.bioinformatics.babraham.ac.uk/projects/fastqc
Arbizu, M. (2017). Pairwiseadonis: Pairwise Multilevel Comparison Using Adonis. R Package Version 0.0.1. Available online at: https://github.com/pmartinezarbizu/pairwiseAdonis
Bach, H. J., Hartmann, A., Schloter, M., and Munch, J. C. (2001). PCR primers and functional probes for amplification and detection of bacterial genes for extracellular peptidases in single strains and in soil. J. Microbiol. Methods 44, 173–182. doi: 10.1016/S0167-7012(00)00239-6
Bach, H. J., Tomanova, J., Schloter, M., and Munch, J. C. (2002). Enumeration of total bacteria and bacteria with genes for proteolytic activity in pure cultures and in environmental samples by quantitative PCR mediated amplification. J. Microbiol. Methods 49, 235–245. doi: 10.1016/S0167-7012(01)00370-0
Baraniya, D., Puglisi, E., Ceccherini, M. T., Pietramellara, G., Giagnoni, L., Arenella, M., et al. (2016). Protease encoding microbial communities and protease activity of the rhizosphere and bulk soils of two maize lines with different N uptake efficiency. Soil Biol. Biochem. 96, 176–179. doi: 10.1016/j.soilbio.2016.02.001
Bender, S. F., Wagg, C., and van der Heijden, M. G. (2016). An underground revolution: biodiversity and soil ecological engineering for agricultural sustainability. Trends Ecol. Evol. 31, 440–452. doi: 10.1016/j.tree.2016.02.016
Bommarco, R., Kleijn, D., and Potts, S. G. (2013). Ecological intensification: harnessing ecosystem services for food security. Trends Ecol. Evol. 28, 230–238. doi: 10.1016/j.tree.2012.10.012
Bosshard, C., Sørensen, P., Frossard, E., Dubois, D., Mäder, P., Nanzer, S., et al. (2009). Nitrogen use efficiency of15N-labelled sheep manure and mineral fertiliser applied to microplots in long-term organic and conventional cropping systems. Nutr. Cycl. Agroecosyst. 83, 271–287. doi: 10.1007/s10705-008-9218-7
Brankatschk, R., Töwe, S., Kleineidam, K., Schloter, M., and Zeyer, J. (2011). Abundances and potential activities of nitrogen cycling microbial communities along a chronosequence of a glacier forefield. ISME J. 5, 1025–1037. doi: 10.1038/ismej.2010.184
Cookson, W. R., Osman, M., Marschner, P., Abaye, D. A., Clark, I., Murphy, D. V., et al. (2007). Controls on soil nitrogen cycling and microbial community composition across land use and incubation temperature. Soil Biol. Biochem. 39, 744–756. doi: 10.1016/j.soilbio.2006.09.022
Ding, W., Meng, L., Yin, Y., Cai, Z., and Zheng, X. (2007). CO2 emission in an intensively cultivated loam as affected by long-term application of organic manure and nitrogen fertilizer. Soil Biol. Biochem. 39, 669–679. doi: 10.1016/j.soilbio.2006.09.024
Edgar, R. C. (2013). UPARSE: highly accurate OTU sequences from microbial amplicon reads. Nat. Methods 10, 996–998. doi: 10.1038/nmeth.2604
Elser, J. J., Bracken, M. E. S., Cleland, E. E., Gruner, D. S., Harpole, W. S., Hillebrand, H., et al. (2007). Global analysis of nitrogen and phosphorus limitation of primary producers in freshwater, marine and terrestrial ecosystems. Ecol. Lett. 10, 1135–1142. doi: 10.1111/j.1461-0248.2007.01113.x
Emmerling, C., Schloter, M., Hartmann, A., and Kandeler, E. (2002). Functional diversity of soil organisms - a review of recent research activities in Germany. J. Plant Nutr. Soil Sci. 165, 408–420. doi: 10.1002/1522-2624(200208)165:4<408::AID-JPLN408>3.0.CO;2-3
Ewels, P., Magnusson, M., Lundin, S., and Käller, M. (2016). MultiQC: summarize analysis results for multiple tools and samples in a single report. Bioinformatics 32, 3047–3048. doi: 10.1093/bioinformatics/btw354
Falkowski, P. G., Fenchel, T., and Delong, E. F. (2008). The microbial engines that drive earth's biogeochemical cycles. Science 320, 1034–1039. doi: 10.1126/science.1153213
Fließbach, A., Oberholzer, H. R., Gunst, L., and Mäder, P. (2007). Soil organic matter and biological soil quality indicators after 21 years of organic and conventional farming. Agric. Ecosyst. Environ. 118, 273–284. doi: 10.1016/j.agee.2006.05.022
Fowler, D., Coyle, M., Skiba, U., Sutton, M. A., Cape, J. N., Reis, S., et al. (2013). The global nitrogen cycle in the twenty- first century. Philos. Trans. R. Soc. B. Biol. Sci. 368:20130165. doi: 10.1098/rstb.2013.0165
Fuka, M., Engel, M., Gattinger, A., Bausenwein, U., Sommer, M., Munch, J. C., et al. (2008). Factors influencing variability of proteolytic genes and activities in arable soils. Soil Biol. Biochem. 40, 1646–1653. doi: 10.1016/j.soilbio.2008.01.028
Fuka, M., Engel, M., Hagn, A., Munch, J. C., Sommer, M., and Schloter, M. (2009). Changes of diversity pattern of proteolytic bacteria over time and space in an agricultural soil. Microb. Ecol. 57, 391–401. doi: 10.1007/s00248-008-9416-5
Fuka, M. M., Engel, M., Munch, J. C., and Schloter, M. (2007). “Characterization of proteolytic microbes and their activities in soils,” in Biology of the Nitrogen Cycle, eds H. Bothe, S. J. Ferguson and W. E. Newton (Elsevier B.V.), 303–309. doi: 10.1016/B978-044452857-5.50020-5
Galloway, J. N., Townsend, A. R., Erisman, J. W., Bekunda, M., Cai, Z., Freney, J. R., et al. (2008). Transformation of the nitrogen cycle: recent trends, questions, and potential solutions. Science 320, 889–892. doi: 10.1126/science.1136674
Gamborg, O., and Wetter, L. (1975). Plant Tissue Culture Methods. Saskatoon: National Research Council of Canada, Prairie Regional Laboratory.
Garbeva, P., van Veen, J. A., and van Elsas, J. D. (2004). Microbial diversity in soil: selection of microbial populations by plant and soil type and implications for disease suppressiveness. Annu. Rev. Phytopathol. 42, 243–270. doi: 10.1146/annurev.phyto.42.012604.135455
Geisseler, D., Horwath, W. R., Joergensen, R. G., and Ludwig, B. (2010). Pathways of nitrogen utilization by soil microorganisms - a review. Soil Biol. Biochem. 42, 2058–2067. doi: 10.1016/j.soilbio.2010.08.021
Gonzalez, J. M., Zimmermann, J., and Saiz-Jimenez, C. (2005). Evaluating putative chimeric sequences from PCR-amplified products. Bioinformatics 21, 333–337. doi: 10.1093/bioinformatics/bti008
Gresham, T. L. T., Sheridan, P. P., Watwood, M. E., Fujita, Y., and Colwell, F. S. (2007). C-based primers for groundwater detection of urea-hydrolyzing bacteria. Geomicrobiol. J. 24, 353–364. doi: 10.1080/01490450701459283
Gutser, R., Ebertseder, T., Weber, A., Schraml, M., and Schmidhalter, U. (2005). Short-term and residual availability of nitrogen after long-term application of organic fertilizers on arable land. J. Plant Nutr. Soil Sci. 168, 439–446. doi: 10.1002/jpln.200520510
Hartmann, M., Frey, B., Mayer, J., Mäder, P., and Widmer, F. (2015). Distinct soil microbial diversity under long-term organic and conventional farming. ISME J. 9, 1177–1194 doi: 10.1038/ismej.2014.210
Hauck, R. D., and Bremner, J. M. (1976). Use of tracers for soil and fertilizer nitrogen research. Adv. Agron. 28, 219–266. doi: 10.1016/S0065-2113(08)60556-8
IPCC (2014). Climate Change 2014: Synthesis Report. Contribution of Working Groups I, II and III to the Fifth Assessment Report of the Intergovernmental Panel on Climate Change.
Isbell, F., Craven, D., Connolly, J., Loreau, M., Schmid, B., Beierkuhnlein, C., et al. (2015). Biodiversity increases the resistance of ecosystem productivity to climate extremes. Nature 526, 574–577. doi: 10.1038/nature15374
Izquierdo, J. A., and Nüsslein, K. (2006). Distribution of extensive nifH gene diversity across physical soil microenvironments. Microb. Ecol. 51, 441–452. doi: 10.1007/s00248-006-9044-x
Jaenicke, S., Albaum, S. P., Blumenkamp, P., Burkhard, L., Stoye, J., and Goesmann, A. (2018). Flexible metagenome analysis using the MGX framework. Microbiome 6, 1–9. doi: 10.1186/s40168-018-0460-1
Jan, M. T., Roberts, P., Tonheim, S. K., and Jones, D. L. (2009). Protein breakdown represents a major bottleneck in nitrogen cycling in grassland soils. Soil Biol. Biochem. 41, 2272–2282. doi: 10.1016/j.soilbio.2009.08.013
Jangid, K., Williams, M. A., Franzluebbers, A. J., Sanderlin, J. S., Reeves, J. H., Jenkins, M. B., et al. (2008). Relative impacts of land-use, management intensity and fertilization upon soil microbial community structure in agricultural systems. Soil Biol. Biochem. 40, 2843–2853. doi: 10.1016/j.soilbio.2008.07.030
Jones, D. L., and Kielland, K. (2002). Soil amino acid turnover dominates the nitrogen flux in permafrost-dominated taiga forest soils. Soil Biol. Biochem. 34, 209–219. doi: 10.1016/S0038-0717(01)00175-4
Kundel, D., Meyer, S., Birkhofer, H., Fliessbach, A., Mäder, P., Scheu, S., et al. (2018). Design and manual to construct rainout-shelters for climate change experiments in agroecosystems. Front. Environ. Sci. 6:14. doi: 10.3389/fenvs.2018.00014
Ladd, J. N., and Butler, J. H. A. (1972). Short-term assays of soil proteolytic enzyme activities using proteins and dipeptide derivatives as substrates. Soil Biol. Biochem. 4, 19–30. doi: 10.1016/0038-0717(72)90038-7
Leininger, S., Urich, T., Schloter, M., Schwark, L., Qi, J., Nicol, G. W., et al. (2006). Archaea predominate among ammonia-oxidizing prokaryotes in soils. Nature 442, 806–809. doi: 10.1038/nature04983
Lipson, D., and Näsholm, T. (2001). The unexpected versatility of plants: organic nitrogen use and availability in terrestrial ecosystems. Oecologia 128, 305–316. doi: 10.1007/s004420100693
Lori, M., Symnaczik, S., Mäder, P., De Deyn, G., and Gattinger, A. (2017). Organic farming enhances soil microbial abundance and activity—A meta-analysis and meta-regression. PLoS ONE 12:e0180442. doi: 10.1371/journal.pone.0180442
Lynch, J. M., and Bragg, E. (1985). Microorganisms and soil aggregate stability. Adv. Soil Sci. 2, 133–171.
Mäder, P., Fliessbach, A., Dubois, D., Gunst, L., Fried, P., and Niggli, U. (2002). Soil fertility and biodiversity in organic farming. Science 296, 1694–1697. doi: 10.1126/science.1071148
Magoč, T., and Salzberg, S. L. (2011). FLASH: fast length adjustment of short reads to improve genome assemblies. Bioinformatics 27, 2957–2963. doi: 10.1093/bioinformatics/btr507
Meisinger, J. J., Schepers, J. S., and Raun, W. R. (2008). Crop nitrogen requirement and fertilization historical perspective. NitrogenAgronomyMonograph Ch 14563, 563–612. doi: 10.2134/agronmonogr49
Murphy, D. V., Macdonald, A. J., Stockdale, E. A., Goulding, K. W. T., Fortune, S., Gaunt, J. L., et al. (2000). Soluble organic nitrogen in agricultural soils. Biol. Fertil. Soils 30, 374–387. doi: 10.1007/s003740050018
Naeem, S., and Li, S. (1997). Biodiversity enhances ecosystem reliability. Nature 390, 507–509. doi: 10.1038/37348
Nicholson, W. L. (2002). Roles of Bacillus endospores in the environment. Cell. Mol. Life Sci. 59, 410–416. doi: 10.1007/s00018-002-8433-7
Nicholson, W. L., Munakata, N., Horneck, G., Melosh, H. J., and Setlow, P. (2000). Resistance of Bacillus endospores to extreme terrestrial and extraterrestrial environments. Microbiol. Mol. Biol. Rev. 64, 548–72. doi: 10.1128/MMBR.64.3.548-572.2000
Nielsen, U. N., Wall, D. H., and Six, J. (2015). Soil biodiversity and the environment. Annu. Rev. Environ. Resour. 40, 63–90. doi: 10.1146/annurev-environ-102014-021257
Nsabimana, D., Haynes, R. J., and Wallis, F. M. (2004). Size, activity and catabolic diversity of the soil microbial biomass as affected by land use. Appl. Soil Ecol. 26, 81–92. doi: 10.1016/j.apsoil.2003.12.005
Oksanen, J., Guillaume Blanchet, F., Friendly, M., Kindt, R., Legendre, P., McGlinn, D., et al. (2017). Vegan: Community Ecology Package. R Package Version 2.4-3. Available online at: https://CRAN.R-project.org/package=vegan
Philippot, L., Spor, A., Hénault, C., Bru, D., Bizouard, F., Jones, C. M., et al. (2013). Loss in microbial diversity affects nitrogen cycling in soil. ISME J. 7, 1609–1619. doi: 10.1038/ismej.2013.34
Pietikäinen, J., Pettersson, M., and Bååth, E. (2005). Comparison of temperature effects on soil respiration and bacterial and fungal growth rates. FEMS Microbiol. Ecol. 52, 49–58. doi: 10.1016/j.femsec.2004.10.002
Polz, M. F., Alm, E. J., and Hanage, W. P. (2013). Horizontal gene transfer and the evolution of bacterial and archaeal population structure. Trends Genet. 29, 170–175. doi: 10.1016/j.tig.2012.12.006
Rillig, M. C., and Mummey, D. L. (2006). Mycorrhizas and soil structur. New Phytol. 171, 41–53. doi: 10.1111/j.1469-8137.2006.01750.x
Rocca, J. D., Hall, E. K., Lennon, J. T., Evans, S. E., Waldrop, M. P., Cotner, J. B., et al. (2015). Relationships between protein-encoding gene abundance and corresponding process are commonly assumed yet rarely observed. ISME J. 9, 1693–1699. doi: 10.1038/ismej.2014.252
Rösch, C., Mergel, A., and Bothe, H. (2002). Biodiversity of denitrifying and dinitrogen-fixing bacteria in an acid forest soil. Appl. Environ. Microbiol. 68, 3818–3829. doi: 10.1128/AEM.68.8.3818-3829.2002
Rotthauwe, J.-H., Witzel, K.-P., and Liesack, W. (1997). The ammonia monooxygenase structural gene amoa as a functional marker: molecular fine-scale analysis of natural ammonia-oxidizing populations. Appl. Environ. Microbiol. 63, 4704–4712.
Sakurai, M., Suzuki, K., Onodera, M., Shinano, T., and Osaki, M. (2007). Analysis of bacterial communities in soil by PCR-DGGE targeting protease genes. Soil Biol. Biochem. 39, 2777–2784. doi: 10.1016/j.soilbio.2007.05.026
Schimel, J. P., and Bennett, J. (2004). Nitrogen mineralization: challenges of a changing paradigm. Ecology 85, 591–602. doi: 10.1890/03-8002
Schirmer, M., Ijaz, U. Z., D'Amore, R., Hall, N., Sloan, W. T., and Quince, C. (2015). Insight into biases and sequencing errors for amplicon sequencing with the Illumina MiSeq platform. Nucleic Acids Res. 43:e37. doi: 10.1093/nar/gku1341
Schulten, H.-R., and Schnitzer, M. (1998). The chemistry of soil organic nitrogen: a review. Biol. Fertil. Soils 26, 1–15. doi: 10.1007/s003740050335
Schütz, L., Gattinger, A., Meier, M., Muller, A., Boller, T., Mäder, P., et al. (2018). Improving crop yield and nutrient use efficiency via biofertilization – a global meta-analysis. Front. Plant Sci. 8:2204. doi: 10.3389/fpls.2017.02204
Sørensen, P., and Amato, M. (2002). Remineralisation and residual effects of N after application of pig slurry to soil. Eur. J. Agron. 16, 81–95. doi: 10.1016/S1161-0301(01)00119-8
Thomas, C. M., and Nielsen, K. M. (2005). Mechanisms of, and barriers to, horizontal gene transfer between bacteria. Nat. Rev. Microbiol. 3, 711–721. doi: 10.1038/nrmicro1234
Thonar, C., Erb, A., and Jansa, J. (2012). Real-time PCR to quantify composition of arbuscular mycorrhizal fungal communities-marker design, verification, calibration and field validation. Mol. Ecol. Resour. 12, 219–232. doi: 10.1111/j.1755-0998.2011.03086.x
Tyson, S. C., and Cabrera, M. L. (1993). Nitrogen mineralization in soils amended with composted and uncomposted poultry litter. Commun. Soil Sci. Plant Anal. 24, 2361–2374. doi: 10.1080/00103629309368961
Vance, E. D., Brookes, P. C., and Jenkinson, D. S. (1987). An extraction method for measuring soil microbial biomass C. Soil Biol. Biochem. 19, 703–707. doi: 10.1016/0038-0717(87)90052-6
Van Der Heijden, M. G., Bardgett, R. D., and Van Straalen, N. M. (2008). The unseen majority: soil microbes as drivers of plant diversity and productivity in terrestrial ecosystems. Ecol. Lett. 11, 296–310. doi: 10.1111/j.1461-0248.2007.01139.x
Van Dijk, E. L., Jaszczyszyn, Y., and Thermes, C. (2014). Library preparation methods for next-generation sequencing: Tone down the bias. Exp. Cell Res. 322, 12–20. doi: 10.1016/j.yexcr.2014.01.008
Vranova, V., Rejsek, K., and Formanek, P. (2013). Proteolytic activity in soil: a review. Appl. Soil Ecol. 70, 23–32. doi: 10.1016/j.apsoil.2013.04.003
Wagg, C., Bender, S. F., Widmer, F., and van der Heijden, M. G. (2014). Soil biodiversity and soil community composition determine ecosystem multifunctionality. Proc. Natl. Acad. Sci. U.S.A. 111, 5266–5270. doi: 10.1073/pnas.1320054111
Whitmore, A. P., and Schröder, J. J. (1996). Modelling the change in soil organic C and N and the mineralization of N from soil in response to applications of slurry manure. Plant Soil 184, 185–194. doi: 10.1007/BF00010448
Wickings, K., Grandy, A. S., Reed, S. C., and Cleveland, C. C. (2012). The origin of litter chemical complexity during decomposition. Ecol. Lett. 15, 1180–1188. doi: 10.1111/j.1461-0248.2012.01837.x
Yachi, S., and Loreau, M. (1999). Biodiversity and ecosystem productivity in a fluctuating environment: The insurance hypothesis. Proc. Natl. Acad. Sci. U.S.A. 96, 1463–1468.
Zehr, J. P., Jenkins, B. D., Short, S. M., and Steward, G. F. (2003). Nitrogenase gene diversity and microbial community structure: a cross-system comparison. Env. Microbiol. 5, 539–554. doi: 10.1046/j.1462-2920.2003.00451.x
Keywords: organic farming, climate change, drought, nitrogen provisioning, protease encoding microbes
Citation: Lori M, Symanczik S, Mäder P, Efosa N, Jaenicke S, Buegger F, Tresch S, Goesmann A and Gattinger A (2018) Distinct Nitrogen Provisioning From Organic Amendments in Soil as Influenced by Farming System and Water Regime. Front. Environ. Sci. 6:40. doi: 10.3389/fenvs.2018.00040
Received: 03 April 2018; Accepted: 14 May 2018;
Published: 04 June 2018.
Edited by:
Urs Feller, Universität Bern, SwitzerlandReviewed by:
Marcello Mastrorilli, Consiglio per la Ricerca in Agricoltura e L'analisi Dell'economia Agraria (CREA), ItalyCopyright © 2018 Lori, Symanczik, Mäder, Efosa, Jaenicke, Buegger, Tresch, Goesmann and Gattinger. This is an open-access article distributed under the terms of the Creative Commons Attribution License (CC BY). The use, distribution or reproduction in other forums is permitted, provided the original author(s) and the copyright owner are credited and that the original publication in this journal is cited, in accordance with accepted academic practice. No use, distribution or reproduction is permitted which does not comply with these terms.
*Correspondence: Martina Lori, bWFydGluYS5sb3JpQGZpYmwub3Jn
Disclaimer: All claims expressed in this article are solely those of the authors and do not necessarily represent those of their affiliated organizations, or those of the publisher, the editors and the reviewers. Any product that may be evaluated in this article or claim that may be made by its manufacturer is not guaranteed or endorsed by the publisher.
Research integrity at Frontiers
Learn more about the work of our research integrity team to safeguard the quality of each article we publish.