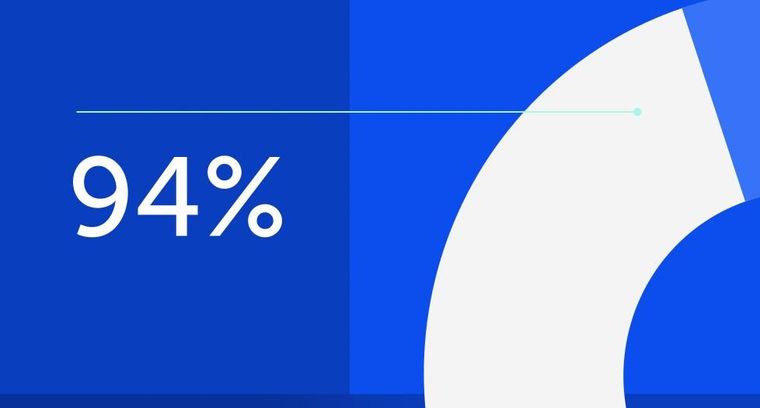
94% of researchers rate our articles as excellent or good
Learn more about the work of our research integrity team to safeguard the quality of each article we publish.
Find out more
PERSPECTIVE article
Front. Environ. Sci., 09 September 2015
Sec. Conservation and Restoration Ecology
Volume 3 - 2015 | https://doi.org/10.3389/fenvs.2015.00063
Social media data have been extensively used in numerous fields of science, but examples of their use in conservation science are still very limited. In this paper, we propose a framework on how social media data could be useful for conservation science and practice. We present the commonly used social media platforms and discuss how their content could provide new data and information for conservation science. Based on this, we discuss how future work in conservation science and practice would benefit from social media data.
Rates of species extinctions and habitat loss are unprecedented and on-going (Butchart et al., 2010). International policy have set global targets to reverse the biodiversity “crisis” (Convention on Biological Diversity, 2010). One important limitation in reaching global conservation goals is that the data available to inform conservation decision-making is limited and both regionally and taxonomically biased (Schulman et al., 2007; Brooks, 2014; Di Minin and Toivonen, 2015). Another important issue is that global to regional information on threats affecting biodiversity persistence is generally missing. Collecting more data is expensive and resources are woefully inadequate.
Crowd-sourcing data collection to nature enthusiasts is considered as one of the ways to effectively collect data when resources are limited. Citizen science and crowd-sourcing of biological data collection have long been used in many countries, but have recently been facilitated by the availability of smart phones equipped with Global Positioning System (GPS), high resolution cameras, and continuous internet connection. As a result, citizen-science is being increasingly used to actively engage non-professionals in real scientific research (Goodchild, 2007; Cohn, 2008). This involvement has taken many forms, ranging from collecting data on species distribution and abundance to crowd-sourcing the data interpretation online (Dickinson et al., 2012; Swanson et al., 2015). Eventually, data collected by citizen-scientists can be used by researchers for a number of purposes in conservation science (Devictor et al., 2010; Tulloch et al., 2013). An important limitation, however, is that citizen science requires systematic organization, marketing, commitment and skills, and is thus mostly focused in the developed world (Kennett et al., 2015).
In addition to deliberately planned citizen science campaigns, an increasingly interesting source of data is user-generated content produced via various social media platforms. The use of social media has been increasing dramatically during the last few years. Furthermore, most social media platforms are providing an interface to extract knowledge from the billions of posts provided by millions of people globally. No wonder that social media data have been extensively used in numerous fields of science from identifying crime hotspots (Malleson and Andresen, 2014), to measuring foreign policy dynamics (Zeitzoff et al., 2015), or monitoring public health (Denecke et al., 2013). However, the use of social media data in conservation science has so far been limited to only a few examples, including ecosystem services mapping (Casalegno et al., 2013; Richards and Friess, 2015); conservation marketing and education (Wood et al., 2013; Roberge, 2014); species (Stafford et al., 2010; Barve, 2014), ecosystem (Daume et al., 2014) and management (Barry, 2014) monitoring; and conservation communication (Papworth et al., 2015).
A number of social media platforms where users can create and share media data and annotate to them are available (http://www.thesocialmediahat.com/active-users). Typically such user-generated content includes text, tags, pictures, or videos. In addition to the content itself, social media data provides a wide array of information about the users and the social/communication networks between them. These networks can be formed explicitly from the links between items or users (such as hashtags or followers) or implicitly by using quality ratings (e.g., likes on Facebook or re-tweets on Twitter). Different platforms are used to share content in different ways. Instagram and Twitter, for example, are widely used for real-time posting of individual pictures and text. Besides, Instagram is mainly used to share self-generated content, while Twitter is also being used to pass on content and links provided by others. In addition to real-time posting, Facebook is often used to share individual pictures, or photo albums (e.g., from a trip) but not (necessarily) in real time. Panoramio is an interesting platform particularly for sharing landscape pictures. For analyses purposes, the public content of most social media platforms can be accessed via ready-made analysis interfaces (e.g., http://onemilliontweetmap.com/), openly available tools for extracting data from application programming interfaces (e.g., Tweepy for Python or TwitteR package for R), or from data resellers such as Gnip (https://gnip.com/). Interfaces provide data with varying restrictions and terms of use. Restricting the content that will be downloaded is typically controlled by specifying search parameters, such as the geographic radius or key words (e.g., “elephant” or “landscape”).
While used for various different purposes, social media platforms also provide a venue for sharing biodiversity related content and posts on nature experiences. Conservation science is increasingly extracting knowledge from platforms such as Facebook, Twitter, Panoramio, and Flickr (Stafford et al., 2010; Casalegno et al., 2013; Wood et al., 2013; Barry, 2014; Barve, 2014; Daume et al., 2014; Roberge, 2014; Papworth et al., 2015; Richards and Friess, 2015). In order to get a broader understanding on the use and popularity of the different social media platforms for nature experiences, we carried out a survey where respondents were asked which social media platforms were more relevant for them (Table 1; see Appendix S1 in Supplementary Materials for the relevant questions). The survey was disseminated online via university mailing lists in Finland and Germany and a wide social media campaign, so the respondents were self-selected. The survey consisted of multiple choice questions where the respondents had to select which social media platforms they used for posting content (texts, pictures, and videos) related to nature-based experiences. Respondents were also asked to select which social media platforms they used to post content related to any type of experience. Finally, respondents were asked with whom they shared content on social media platforms and whether they geotagged the content of their posts. The sample size (n = 590) was considered appropriate for a 5% margin of error considered to be acceptable in survey research (Bartlett et al., 2001). The respondents from different backgrounds and from all continents clearly rated Facebook as the most popular social media platform for their nature related posts, followed by Instagram, Twitter, and YouTube (Table 1). However, the popularity of the other platforms varied according to the content type (text, picture, or video) of the posts (Table 1). Among the respondents, 61% used public profiles to post some content and 40% geotagged their posts.
In social media, each post contains information about when (day, hour, minute) the content was created or shared on social media. When geotagged, the pictures and texts have specific coordinates of latitude and longitude, or place name, showing the location where they were taken or posted from. Therefore, social media content have the potential to represent mobile populations at, in fact, higher spatial and temporal resolutions than many other available data (Longley et al., 2015). Social media posts also include a wealth of information about what users find meaningful to post about (e.g., species they have spotted or landscapes they like). Content of the posts may also reveal different activities of the users that can be used to understand e.g., why users were visiting specific locations. Finally, the profiles and contents of social media users can be used to extract background information about who the users are, e.g., their country of origin, the language spoken, and gender. Overall, as framed in Figure 1, the knowledge extraction from social media content may be used to answer questions like “where?” and “when?” (spatial, temporal, and spatio-temporal analyses), but also reveal more about “what” and “why” the users posted about (content analyses) and, indirectly, “who” the users are. Additional information can be obtained about who else is interested in the experiences and posts of the users (social network analyses).
In this section, we explore how answering the questions posed in Figure 1 could help inform systematic conservation planning, as well as management and conservation marketing.
Conservation science usually benefits from spatially explicit information. For example, social media data can be incorporated at different stages in systematic conservation planning (Margules and Pressey, 2000; Knight et al., 2006). An important stage deals with compiling data on the biodiversity of the planning region. Geo-tagged posts on the occurrence of selected biodiversity features for which spatial information is missing, for example, could be used directly in conservation planning assessments or to model species distributions (Elith et al., 2006). Social media data could also be used to identify the imminence of threat to biodiversity features that are poorly or not yet protected, and the threats posed to areas that are not yet protected.
It has been suggested that social media content can be used to analyze temporal trends on visitation patterns of tourists in places of interest, such as national parks (Wood et al., 2013). On the other hand, spatio-temporal analyses could be used to understand changes in the content of the posts over both space and time (Ardon et al., 2013). Combined with content analysis, social media could be used to understand e.g., species population trends, invasive species spread and how landscapes have changed over time in relation to ecological processes or disturbance.
Image and text content analyses can be used to analyze the content of social media posts. In many cases, proper classification and interpretation of the social media content requires a human analyst (Poorthuis et al., in press). Going through social media contents manually is, however, a laborious task. Luckily the tools for automatic data classification that may help in simple content analyses are developing quickly (e.g., Deng et al., 2009; Laurance et al., 2009; Angus et al., 2010; Ozel and Park, 2012; Lin et al., 2014; Zhou et al., 2014; Karpathy and Fei-Fei, 2015; Russakovsky et al., 2015; Schwartz and Ungar, 2015). Practical applications utilizing such automatic classification approach are also emerging by using e.g., image recognition, such as the smartphone app CamFind (http://camfindapp.com/). In addition to providing potentially interesting information on e.g., species/landscape observations, social media data can be used to assess the attention received by species or ecosystems in social media platforms. This could provide important information to different stakeholders, ranging from international non-governmental organizations needing to raise funding for conservation to park managers needing to promote ecotourism in their parks. As opposed to other data collected using e.g., stated preference techniques, social media data could provide a direct behavioral basis for measuring actual public engagement in biodiversity conservation. Again, temporal analyses of social media data could be used to understand changes in preference for biodiversity features over time.
Social media content may reveal the activities of people very effectively, particularly on platforms like Instagram where people usually post pictures in real-time. While mobile phone data may provide accurate and representative information on where people are and when they are there, social media may reveal the motivations behind the posts. Our preliminary tests with 30,000 Instagram pictures from Kruger National Park in South Africa show clearly that activities from game driving to walking safaris are well documented in social media, and can to some extent be classified with visual interpretation. Social media posts may also reveal illegal activities and be useful in fighting illegal wildlife trade (e.g., illegal killing of rhinoceros for their horn Di Minin et al., 2015).
Social media posts and user profile descriptions quite often include text content that carries a wealth of information about the users, and may even reveal their background, nationality or preferences. From common selfies one can already try estimate people's gender and age (https://how-old.net). Clustering analyses or other techniques adopted from econometrics (see e.g., Di Minin et al., 2013) can then be used to identify different type of users. Social media data could also be used to identify important stakeholders to engage in the implementation strategy in systematic conservation planning (Knight et al., 2006). At the same time, social media data can be used to unveil opportunities for protection in areas where e.g., private landowners and communities are supportive of conservation measures or against unsustainable development.
While we believe that social media data can contribute to conservation science, a number of challenges, including geographic and content biases, self-selecting users, and ethical concerns (Elwood and Leszczynski, 2011; Crampton et al., 2013; Goodchild, 2013; Longley et al., 2015), need to be addressed when using social media data in future research or to inform management decisions. While the amount of user generated content is really high, much of this is produced by a small number of highly active users (Li et al., 2013) and only a small proportion of all of the data is open. In addition, the location information for many posts may be locally incorrect or completely missing. Yet, further exploring who the social media users are (see e.g., Longley et al., 2015), filtering out spatially irrelevant or biased observations (e.g., clusters near accommodation with WiFi inside parks), as well as sampling/normalizing data based on user profiling or geographical distribution of observations, may help address some of these issues. Additionally, research questions asked and, particularly, the interpretations made should be considered carefully based on data limitations and biases caused by the fact that not everyone uses social media and not everything seen or experienced is being reported. Finally, there are many ethical issues, such as privacy, free speech, data leakage, and revealing users' identities (e.g., in relation to illegal activities) (Light and McGrath, 2010), which should be considered carefully prior to using social media data in conservation science.
Analyses of social media data could avoid some other shortcomings of more traditional methods, such as questionnaires, by avoiding potential self-reporting errors and non-response bias. Therefore, social media data could be particularly useful to cross-validate data collected from more traditional surveys or the opposite. Finally, social media data are currently biased geographically, especially to the developed world. However, improved mobile phone coverage will increase the potential of social media as a means to collect information for conservation science purposes also in the developing world. In areas where resources for field work and data collection are scarce, social media may potentially help save costs and allow directing professional data collection to less known or more poorly accessible areas.
Social media data can potentially play an important role in conservation science. In addition to crowd-sourced citizen science data, which have been extensively used to monitor biodiversity, we suggest that social media data could be used to learn more about the spatio-temporal patterns, values, and activities related to biodiversity conservation of different groups of people. Such information is essential in order to identify both threats to biodiversity and opportunities for conservation. While social media users are currently not intentionally involved in data collection, more awareness could be raised in social media platforms (for instance by campaigning) to increase their monitoring role when visiting natural areas. As a result, more people could potentially get involved with data collection and become more aware about biodiversity conservation. At the same time, social media platforms could be more directly targeted for directed citizen science campaigns. Finally, social media data are far from a panacea for all data challenges in conservation science, but, combined with other sources of data, can provide innovative ways to address the information needs of future conservation challenges.
The authors declare that the research was conducted in the absence of any commercial or financial relationships that could be construed as a potential conflict of interest.
EDM and TT thank the ERC-StG Grant 260393 and the Academy of Finland Centre of Excellence Programme 2012–2017, Grant 250444, for support. HT thanks the DENVI doctoral programme at the University of Helsinki for support.
The Supplementary Material for this article can be found online at: http://journal.frontiersin.org/article/10.3389/fenvs.2015.00063
Angus, E., Stuart, D., and Thelwall, M. (2010). Flickr's potential as an academic image resource: an exploratory study. J. Libr. Inf. Sci. 42, 268–278. doi: 10.1177/0961000610384656
Ardon, S., Bagchi, A., Mahanti, A., Ruhela, A., Seth, A., Tripathy, R. M., et al. (2013). “Spatio-temporal and events based analysis of topic popularity in twitter,” in Proceedings of the 22nd ACM International Conference on Information & Knowledge Management - CIKM '13 (New York, NY: ACM Press), 219–228. doi: 10.1145/2505515.250552510
Barry, S. J. (2014). Using social media to discover public values, interests, and perceptions about cattle grazing on park lands. Environ. Manage. 53, 454–464. doi: 10.1007/s00267-013-0216-4
Bartlett, J. E., Kotrlik, J. W., and Higgins, C. (2001). Organizational research: determining appropriate sample size in survey research appropriate sample size in survey research. Inf. Technol. Learn. Perform. J. 19, 43.
Barve, V. (2014). Discovering and developing primary biodiversity data from social networking sites: a novel approach. Ecol. Inform. 24, 194–199. doi: 10.1016/j.ecoinf.2014.08.008
Butchart, S. H. M., Walpole, M., Collen, B., van Strien, A., Scharlemann, J. P. W., Almond, R. E. A., et al. (2010). Global biodiversity: indicators of recent declines. Science 328, 1164–1168. doi: 10.1126/science.1187512
Casalegno, S., Inger, R., DeSilvey, C., and Gaston, K. J. (2013). Spatial covariance between aesthetic value & other ecosystem services. PLoS ONE 8:e68437. doi: 10.1371/journal.pone.0068437
Cohn, J. P. (2008). Citizen science: can volunteers do real research? Bioscience 58, 192–197. doi: 10.1641/B580303
Convention on Biological Diversity. (2010). Report of the Tenth Meeting of the Conference of the Parties to the Convention on Biological Diversity. UNEP, Montreal, QC.
Crampton, J. W., Graham, M., Poorthuis, A., Shelton, T., Stephens, M., Wilson, M. W., et al. (2013). Beyond the geotag: situating “big data” and leveraging the potential of the geoweb. Cartogr. Geogr. Inf. Sci. 40, 130–139. doi: 10.1080/15230406.2013.777137
Daume, S., Albert, M., and Von Gadow, K. (2014). Forest monitoring and social media - Complementary data sources for ecosystem surveillance? For. Ecol. Manage. 316, 9–20. doi: 10.1016/j.foreco.2013.09.004
Denecke, K., Krieck, M., Otrusina, L., et al. (2013). How to exploit twitter for public health monitoring? Methods Inf. Med. 52, 326–339. doi: 10.3414/ME12-02-0010
Deng, J., Dong, W., Socher, R., and Li, L. (2009). ImageNet: a large-scale hierarchical image database. Proc. CVPR 248–255. doi: 10.1109/CVPR.2009.5206848
Devictor, V., Whittaker, R. J., and Beltrame, C. (2010). Beyond scarcity: citizen science programmes as useful tools for conservation biogeography. Divers. Distrib. 16, 354–362. doi: 10.1111/j.1472-4642.2009.00615.x
Dickinson, J. L., Shirk, J., Bonter, D., Bonney, R., Rhiannon, L. C., Martin, J., et al. (2012). The current state of citizen science as a tool for ecological research and public engagement. Front. Ecol. Environ. 10, 291–297. doi: 10.1890/110236
Di Minin, E., Fraser, I., Slotow, R., and MacMillan, D. C. (2013). Understanding heterogeneous preference of tourists for big game species: implications for conservation and management. Anim. Conserv. 16, 249–258. doi: 10.1111/j.1469-1795.2012.00595.x
Di Minin, E., Laitila, J., Montesino-Pouzols, F., Leader-Williams, N., Slotow, R., Goodman, P. S., et al. (2015). Identification of policies for a sustainable legal trade in rhinoceros horn based on population projection and socioeconomic models. Conserv. Biol. 29, 545–555. doi: 10.1111/cobi.12412
Di Minin, E., and Toivonen, T. (2015). Global protected area expansion: creating more than paper parks. Bioscience 65, 637–638. doi: 10.1093/biosci/biv064
Elith, J., Graham, C. H., Anderson, R. P., et al. (2006). Novel methods improve prediction of species' distributions from occurrence data. Ecography 2, 129–151. doi: 10.1111/j.2006.0906-7590.04596.x
Elwood, S., and Leszczynski, A. (2011). Privacy, reconsidered: new representations, data practices, and the geoweb. Geoforum 42, 6–15. doi: 10.1016/j.geoforum.2010.08.003
Goodchild, M. F. (2007). Citizens as sensors: the world of volunteered geography. GeoJournal 69, 211–221. doi: 10.1007/s10708-007-9111-y
Goodchild, M. F. (2013). The quality of big (geo)data. Dialogues Hum. Geogr. 3, 280–284. doi: 10.1177/2043820613513392
Karpathy, A., and Fei-Fei, L. (2015). “Deep visual-semantic alignments for generating image descriptions,” in CVPR 2015: 28th IEEE Conference on Computer Vision and Pattern Recognition (Boston).
Kennett, R., Danielson, F., and Silvius, K. (2015). Citizen science is not enough on its own. Nature 521, 161. doi: 10.1038/521161d
Knight, A. T., Cowling, R. M., and Campbell, B. M. (2006). An operational model for implementing conservation action. Conserv. Biol. 20, 408–419. doi: 10.1111/j.1523-1739.2006.00305.x
Laurance, W. F., Goosem, M., and Laurance, S. G. W. (2009). Impacts of roads and linear clearings on tropical forests. Trends Ecol. Evol. 24, 659–669. doi: 10.1016/j.tree.2009.06.009
Light, B., and McGrath, K. (2010). Ethics and social networking sites: a disclosive analysis of Facebook. Inf. Technol. People 23, 290–311. doi: 10.1108/09593841011087770
Li, L., Goodchild, M. F., and Xu, B. (2013). Spatial, temporal, and socioeconomic patterns in the use of Twitter and Flickr. Cartogr. Geogr. Inf. Sci. 40, 61–77. doi: 10.1080/15230406.2013.777139
Lin, H., Jia, J., Guo, Q., Xue, Y., Li, Q., Huang, J., et al. (2014). “User-level psychological stress detection from social media using deep neural network,” Proceedings of the ACM International Conference on Multimedia MM' 14 (Orlando, FL).
Longley, P. A., Adnan, M., and Lansley, G. (2015). The geotemporal demographics of Twitter usage. Environ. Plan. A 47, 465–484. doi: 10.1068/a130122p
Malleson, N., and Andresen, M. A. (2014). The impact of using social media data in crime rate calculations: shifting hot spots and changing spatial patterns. Cartogr. Geogr. Inf. Sci. 42, 112–121. doi: 10.1080/15230406.2014.905756
Margules, C. R., and Pressey, R. L. (2000). Systematic conservation planning. Nature 405, 243–253. doi: 10.1038/35012251
Ozel, B., and Park, H. W. (2012). Online image content analysis of political figures: an exploratory study. Qual. Quant. 46, 1013–1024. doi: 10.1007/s11135-011-9445-x
Papworth, S. K., Nghiem, T. P. L., Chimalakonda, D., Posa, M. R. C., Wijedasa, L. S., Bickford, D., et al. (2015). Quantifying the role of online news in linking conservation research to Facebook and Twitter. Conserv. Biol. 29, 825–833. doi: 10.1111/cobi.12455
Poorthuis, A., Zook, M., Shelton, T., Graham, M., and Stephens, M. (in press). “Using geotagged digital social data in geographic research,” in Key Methods in Geography, eds N. Clifford, S. French, M. Cope, T. Gillespie (London: Sage).
Richards, D. R., and Friess, D. A. (2015). A rapid indicator of cultural ecosystem service usage at a fine spatial scale: content analysis of social media photographs. Ecol. Indic. 53, 187–195. doi: 10.1016/j.ecolind.2015.01.034
Roberge, J. M. (2014). Using data from online social networks in conservation science: which species engage people the most on Twitter? Biodivers. Conserv. 23, 715–726. doi: 10.1007/s10531-014-0629-2
Russakovsky, O., Deng, J., Su, H., Krause, J., Satheesh, S., Ma, S., et al. (2015). ImageNet Large Scale Visual Recognition Challenge. arXiv:1409.0575.
Schulman, L., Toivonen, T., and Ruokolainen, K. (2007). Analysing botanical collecting effort in Amazonia and correcting for it in species range estimation. J. Biogeogr. 34, 1388–1399. doi: 10.1111/j.1365-2699.2007.01716.x
Schwartz, H. A., and Ungar, L. H. (2015). Data-driven content analysis of social media: a systematic overview of automated methods. Ann. Am. Acad. Pol. Soc. Sci. 659, 78–94. doi: 10.1177/0002716215569197
Stafford, R., Hart, A. G., Collins, L., et al. (2010). Eu-social science: the role of internet social networks in the collection of bee biodiversity data. PLoS ONE 5:e14381. doi: 10.1371/journal.pone.0014381
Swanson, A., Kosmala, M., Lintott, C., et al. (2015). Snapshot Serengeti, high-frequency annotated camera trap images of 40 mammalian species in an African savanna. Sci. Data 2, 150026. doi: 10.1038/sdata.2015.26
Tulloch, A. I. T., Possingham, H. P., Joseph, L. N., et al. (2013). Realising the full potential of citizen science monitoring programs. Biol. Conserv. 165, 128–138. doi: 10.1016/j.biocon.2013.05.025
Wood, S. A., Guerry, A. D., Silver, J. M., and Lacayo, M. (2013). Using social media to quantify nature-based tourism and recreation. Sci. Rep. 3:2976. doi: 10.1038/srep02976
Zeitzoff, T., Kelly, J., and Lotan, G. (2015). Using social media to measure foreign policy dynamics: an empirical analysis of the Iranian-Israeli confrontation (2012–13). J. Peace Res. 52, 368–383. doi: 10.1177/0022343314558700
Keywords: social media, Twitter, instagram, Facebook, biodiversity conservation, management, big data, national parks
Citation: Di Minin E, Tenkanen H and Toivonen T (2015) Prospects and challenges for social media data in conservation science. Front. Environ. Sci. 3:63. doi: 10.3389/fenvs.2015.00063
Received: 15 June 2015; Accepted: 27 August 2015;
Published: 09 September 2015.
Edited by:
Krithi K. Karanth, Wildlife Conservation Society, IndiaReviewed by:
Margaret Buck Holland, University of Maryland, Baltimore County, USACopyright © 2015 Di Minin, Tenkanen and Toivonen. This is an open-access article distributed under the terms of the Creative Commons Attribution License (CC BY). The use, distribution or reproduction in other forums is permitted, provided the original author(s) or licensor are credited and that the original publication in this journal is cited, in accordance with accepted academic practice. No use, distribution or reproduction is permitted which does not comply with these terms.
*Correspondence: Enrico Di Minin, Finnish Centre of Excellence in Metapopulation Biology, Department of Biosciences, Biocenter 3, University of Helsinki, PO Box 65 (Viikinkaari 1), 00014 Helsinki, Finland; School of Life Sciences, Westville Campus, University of KwaZulu-Natal, PO Box 54001 (University Road), Durban 4000, South Africa,ZW5yaWNvLmRpLm1pbmluQGhlbHNpbmtpLmZp;
Tuuli Toivonen, Finnish Centre of Excellence in Metapopulation Biology, Department of Biosciences, Biocenter 3, University of Helsinki, PO Box 65 (Viikinkaari 1), 00014 Helsinki, Finland; Department of Geosciences and Geography, University of Helsinki, PO Box 64 (Gustaf Hällströminkatu 2a), 00014 Helsinki, Finland,dHV1bGkudG9pdm9uZW5AaGVsc2lua2kuZmk=
†These authors have contributed equally to this work.
Disclaimer: All claims expressed in this article are solely those of the authors and do not necessarily represent those of their affiliated organizations, or those of the publisher, the editors and the reviewers. Any product that may be evaluated in this article or claim that may be made by its manufacturer is not guaranteed or endorsed by the publisher.
Research integrity at Frontiers
Learn more about the work of our research integrity team to safeguard the quality of each article we publish.