- 1Department of Africana Studies, SUNY Binghamton University, Binghamton, NY, United States
- 2Center for Health Equity & Evaluation Research (CHEER), Texas A&M, College Station, TX, United States
- 3Department of Health Sciences, Sargent College of Health and Rehabilitation Sciences, Boston University, Boston, MA, United States
- 4Department of Global Health, Boston University School of Public Health, Boston, MA, United States
- 5Global Health Systems R.I.S.E Lab, Sargent College of Health and Rehabilitation Services, Boston University, Boston, MA, United States
This study investigates the relationship between neighborhood Socioeconomic Position (SEP) and the prevalence of hypertension and coronary heart disease (CHD) across New York City's community districts. By using a composite SEP index derived from multiple socioeconomic indicators, the research seeks to uncover health disparities that may be obscured in broader geographic analyses. A primary objective is to identify community districts where hypertension and CHD prevalence is significantly higher than expected, even after adjusting for SEP. This focus helps pinpoint areas where targeted public health interventions may be urgently required, especially when the anticipated inverse relationship between SEP and health outcomes does not hold. A linear mixed-effects model (LMM) was used to analyze the association between SEP and these health outcomes, incorporating both fixed and random effects to account for variation across the city's 59 community districts. The analysis revealed a significant negative association between SEP and both hypertension and CHD, with higher SEP levels generally correlating with lower prevalence rates. However, some districts exhibited high random effects, indicating a higher-than-expected prevalence of these conditions, even after controlling for SEP. The authors suspect that additional factors-such as environmental exposures, healthcare accessibility, or community infrastructure-may contribute to these unexplained disparities. The findings emphasize the need for public health strategies that are sensitive to local contexts and the multifactorial nature of health inequities. Understanding the complex drivers behind these disparities can inform policies aimed at reducing health inequities in diverse urban environments.
1 Introduction
Neighborhood characteristics, encompassing both social and physical conditions, play a critical role in influencing the health and well-being of individuals and communities (1). This study focuses on the social context, particularly the socio-economic determinants of health, as they serve as key intermediaries that impact health outcomes at both individual and population levels. The social fabric of a neighborhood is often defined by its socio-economic and demographic composition, typically measured through indicators such as median income and the percentage of residents living below the poverty line. These socio-economic factors are crucial as they shape access to resources, exposure to stress, and overall living conditions, which, in turn, significantly influence health outcomes (2–4).
Grounded in Neighborhood Effects Theory, which posits that the characteristics of communities substantially influence the health of their residents (5, 6), this study conceptualizes neighborhood characteristics through the lens of Neighborhood Socioeconomic Position (SEP). SEP encompasses multiple dimensions, including income, education, and occupation, and has been consistently linked to health outcomes across public health literature. Numerous studies have established that lower SEP is associated with poorer health outcomes (7–9), underscoring the critical role that socio-economic conditions play in driving health disparities across different communities (10).
In New York City, neighborhoods are traditionally categorized by various geographic delineations, such as Public Use Microdata Areas (PUMAs), Community Districts (CDs), Neighborhood Tabulation Areas (NTAs), Zip Code Tabulation Areas (ZCTAs), and United Hospital Fund (UHF) (11) neighborhoods. Among these, the UHF and CD designations are particularly prevalent in health research due to their alignment with zip code-based data commonly available in hospital records and other health datasets (8, 11). However, while these broader delineations offer administrative convenience, they may not be optimal for small-area health outcome analysis or the effective allocation of public health resources (4, 12). In fact, these broader units can obscure nuanced health disparities that manifest at more granular levels, such as the census tract level (4, 12).
A study by Kang et al. (13) highlighted the limitations of traditional resource allocation methods, which often rely on broad geographic delineations like boroughs, potentially overlooking significant health disparities within smaller community districts. Their research, which analyzed data from the NYC Department of Health and Mental Hygiene's 2015 Community Health Profile reports, revealed considerable variability in health outcomes across the city's 59 community districts. Kang et al., found that some districts with moderate health disparities, particularly in Queens and Brooklyn, might not receive adequate attention under conventional resource allocation strategies that prioritize areas with more widespread health issues. Their findings underscore the importance of utilizing small-area data to identify otherwise overlooked health disparities.
Building on the findings of Kang et al., this study aims to further refine our understanding of health disparities in New York City by focusing specifically on coronary heart disease (CHD) and hypertension. These conditions were selected not only due to their significant impact on public health (14, 15) but also because they are among the leading causes of morbidity and mortality in the United States. Their high prevalence and association with socio-economic factors (16–18) make them critical markers for assessing neighborhood-level health disparities.
Hypertension and CHD are well-documented for their robust association with neighborhood socioeconomic position (SEP) (17, 19), making them reliable indicators for understanding how socioeconomic disparities manifest in cardiovascular health.
Moreover, CHD and hypertension are often interrelated, with shared risk factors such as obesity, diabetes, and lifestyle behaviors that are influenced by neighborhood conditions (19–23). By analyzing these two conditions together, the study can indirectly account for the complex interplay between SEP and their common co-morbidities, offering insights into the broader implications for public health interventions.
Lastly, when the prevalence rates of hypertension and CHD do not exhibit the expected inverse relationship with neighborhood SEP, it suggests the presence of other influencing factors that warrant further investigation. This targeted approach serves as a process of elimination, signaling a need to investigate other variables, such as environmental exposures or access to healthcare, that may contribute to health disparities beyond what is captured by SEP alone.
Our investigation was guided by two research questions:
1. How does the relationship between neighborhood SEP and health outcomes (hypertension and CHD) vary across different community districts? This question investigates the variability in the SEP-health outcome relationship, exploring how some districts may experience better or worse health outcomes than expected based on their SEP.
2. Which community districts exhibit significantly higher prevalence of hypertension and CHD than expected, even after accounting for neighborhood SEP? This question aims to identify districts where health outcomes are worse than predicted by SEP alone, thereby pinpointing areas with potential health disparities that warrant targeted public health interventions.
2 Methods & materials
To articulate the socioeconomic and spatial fabric of New York City, our study implemented the Modified Darden-Kamal Composite Socioeconomic Index (Darden-Kamal Index) (4). This index harnesses a spectrum of publicly available 2016–2020 American Community Survey (ACS) (24) variables, specifically selected to provide a multifaceted and robust measure of socioeconomic position (SEP) at the census tract level. The variables were chosen based on their demonstrated relevance to socioeconomic status, their potential impact on public health, and their ability to capture diverse aspects of economic and social well-being. These variables include:
1. Educational Attainment: The percentage of residents aged 25 and older with at least a bachelor's degree. Higher educational levels correlate with better health outcomes and greater economic stability (25, 26).
2. Median Household Income: Reflecting the financial resources available to families and individuals, this measure is a fundamental determinant of access to health-enhancing goods and services (27, 28).
3. Employment in Managerial and Professional Roles: The proportion of the workforce in higher-ranking occupations serves as an indicator of job quality, income, and associated health benefits (28).
4. Housing Value: The median value of owner-occupied housing units, indicative of wealth accumulation and investment in living conditions that can influence health (29).
5. Gross Rent: The combination of contract rent and utility costs, reflecting the cost of living and the economic burden on households (30, 31).
6. Homeownership Rates: Serving as a measure of stability and long-term investment, homeownership is often linked with improved health outcomes and community engagement (31, 32).
7. Poverty Level: The percentage of families below the poverty threshold, which is directly associated with various adverse health conditions and access to care (33).
8. Unemployment Rate: A key indicator of economic vitality, unemployment has profound implications for mental health, access to health care, and overall well-being (3).
9. Vehicle Ownership: Access to a vehicle is not only a marker of economic status but also affects mobility, access to health services, and employment opportunities (34).
Each variable contributes to a Composite Socioeconomic Index (CSI) z-score (Formula 1) — for census tract i, the sum of z scores for the SES variables j, relative to New York City SES; NYC is New York City's area; k is the number of variables in the index; Vi j is the jth SEP variable for a given census tract i; Vj NYC is the mean of the jth variable New York City; and S (Vj NYC) is standard deviation of the jth variable in New York City. After calculating the index score, the values were ranked from least to greatest, which allowed me to divide the New York City into five levels (i.e., ranges of SEP) with boundaries at the 20th, 40th, 60th, and 80th percentiles(quintiles) of the CSI frequency distribution. This categorization allowed for the division of census tracts of residence into five socioeconomic characteristic groups with approximately equal proportions of the population in each group: 1 = very low socioeconomic position (VLSEP), 2 = low socioeconomic position (LSEP), 3 = middle socioeconomic position (MSEP), 4 = high socioeconomic position (HSEP), and 5 = very high socioeconomic position (VHSEP). After calculating the SEP scores across New York City, Table 1 details the spatial and social structures of New York City.
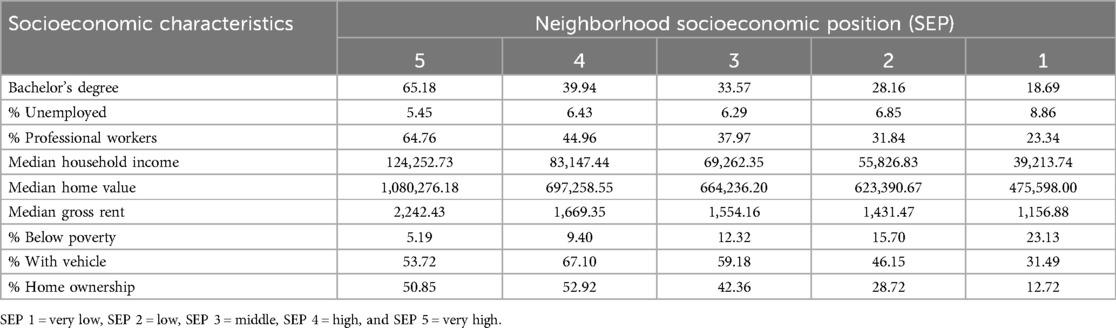
Table 1. Spatial and social structure of New York City, based on the modified Darden-Kamel composite socioeconomic index (CSI): 2016–2020.
Formula 1 Composite Socioeconomic Index Formula.
After assessing neighborhood SEP across New York City's census tracts, the publicly available CDC PLACES 2022 (35) dataset, which provides data collected in 2020, was crucial for analyzing the prevalence of chronic diseases at a detailed level within the city's community districts. The CDC PLACES 2022 dataset was chosen for its temporal alignment with the ACS 2016–2020 data, ensuring consistency in the analysis period. PLACES data offers model-based estimates of key health outcomes, health behaviors, preventive services, and chronic disease risk factors across all counties, places, census tracts, and zip codes in the United States, including essential indicators like hypertension and coronary heart disease.
To integrate the CDC PLACES data with our socioeconomic data, we utilized a data merging process in RStudio. Each census tract-level health outcome from the CDC PLACES dataset was precisely matched with the corresponding neighborhood socioeconomic position (SEP) score using 11-digit geographic identifiers (GEOIDs). This approach ensured accurate alignment of health outcomes with specific socioeconomic conditions across New York City. As highlighted in the introduction, this study focuses on CHD and hypertension due to their significant public health impact (14, 15) and their well-documented association with socioeconomic factors (16–18, 20, 21). These conditions have been thoroughly studied in relation to SEP, making them robust indicators of how socioeconomic factors influence health outcomes. It suggests the presence of other underlying factors that warrant further investigation, when hypertension and CHD prevalence rates do not exhibit the expected inverse relationship with neighborhood SEP.
The granularity of the CDC PLACES dataset was particularly advantageous for our analysis, as it allowed us to assess health disparities at the census tract level rather than at broader geographic units, which can obscure important neighborhood level variations. The reliability and comprehensiveness of the dataset further enhanced the robustness of our findings, enabling us to conduct a detailed examination of the relationship between neighborhood SEP and chronic disease prevalence. This integration of health outcomes and socioeconomic data facilitated a detailed understanding of health disparities within the city's community districts, supporting the study's overall objective of identifying health outcome variance across New York City's community districts.
2.1 Analysis
This study employs a linear mixed-effects model (LMM) to analyze the relationship between neighborhood Socioeconomic Position (SEP) and the prevalence of two critical health outcomes: hypertension (BPHIGH) and coronary heart disease (CHD) across New York City's community districts. The analysis was conducted in multiple steps, including data preparation, model fitting, and evaluation of interaction effects between SEP and health outcomes.
2.1.1 Data preparation
The dataset was created by merging data from multiple sources, including the 2016–2020 American Community Survey (ACS) (24), Community District Tabulation Area Data (CDTA) from the NYC Open Data portal (36), and the CDC PLACES 2022 release data (35), which were collected in 2020. Initially, ACS data were merged with community district names listed in the CDTA dataset using an 11-digit geographic identifier (GEOID) as the match variable. After matching the community district names to the ACS dataset there were 2337 observations. We proceeded with merging the ACS and PLACES datasets. Non-numeric characters were removed from the BPHIGH and CHD crude prevalence rate columns to ensure that the data could be accurately analyzed. Rows with missing SEP values were omitted, resulting in a final dataset of 2,230 observations across 59 community districts, after excluding 97 rows due to missing SEP values. Our decision to perform a complete case analysis resulted in omitting 4.58% of observations from analysis.
The first model, a basic LMM, was designed to examine the fixed effect of SEP on health outcomes while accounting for random effects across community districts. The model included SEP as a fixed effect and community districts as a random intercept to capture the variability between different districts.
Formula 2 Linear Mixed-Effects Model.
yij represents the health outcome (e.g., hypertension or CHD) for individual i in district j, β0 is the intercept, β1 is the fixed effect of SEP, γj is the random effect for district j, and ɛij is the residual error.
To explore how the relationship between SEP and health outcomes varies across community districts, an interaction model was fitted. This model included an interaction term between SEP and the specific health outcomes (BPHIGH and CHD) to assess whether the impact of SEP on each health outcome differed while also accounting for the potential confounding relationship between CHD and hypertension (22, 23, 37–39). Importantly, the model treated one health outcome (e.g., CHD) as a predictor when the other (e.g., hypertension) was the outcome variable, and vice versa. This approach helps determine if SEP influences these conditions differently and clarifies how the interrelationship between CHD and hypertension may affect the overall impact of SEP on each health outcome.
Formula 3 Linear Mixed-Effects Model: SEP∼Health Outcomes.
yij represents the health outcome (hypertension or CHD) for individual i in community district j. β0 is the intercept, β1 measures the effect of SEP, and β2 captures the effect of the other health outcome (CHD when hypertension is the outcome, and vice versa). The interaction term β3 (SEPi × Outcomei) explores whether the relationship between SEP and the outcome varies depending on the level of the other health outcome. γj accounts for random effects specific to each community district, and ɛij represents the residual error term.
In addition to modeling the main and interaction effects, the analysis also concentrated on random effects at the district level. These random effects were assumed to follow a normal distribution: , where represents the variance of the random effects across community districts. The analysis involved extracting and scrutinizing the random intercepts for each district to identify those with the most significant deviations from the overall trend. While technically any district with a random effect value above zero could be considered high, our analysis focused on districts within the top 10% of random effect values.
The identification of these key districts provides a critical context for understanding how socioeconomic position (SEP) influences health outcomes across different areas. Building on this, further analysis was conducted to explore the relationship between hypertension and CHD. By calculating the correlation between the prevalence of these conditions within each community district and relating it tohe mean SEP, we aimed to uncover how variations in SEP might affect the interplay between these two significant. health outcomes.
Formula 4 Correlation Analysis Between Hypertension and CHD.
ρ is the Pearson correlation coefficient, Cov is the covariance between hypertension and CHD, and σ represents the standard deviations of these variables.
3 Results
The analysis produced several key findings regarding the relationship between SEP and the prevalence of hypertension and CHD across New York City's community districts.
3.1 Basic mixed-effects model
The basic LMM revealed a significant negative association between SEP and both hypertension and CHD prevalence. The fixed effects of the model showed that districts with higher SEP levels had lower prevalence rates of both conditions. Specifically, the fixed effect estimates for SEP levels 2 through 5 ranged from −1.90 to −5.23, indicating that as SEP improves, the prevalence of hypertension and CHD decreases. The model's R-squared values (marginal R2 = 0.017, conditional R2 = 0.028) and an AIC of 31,037.43 suggest a modest fit to the data, with much of the variability explained by district-level differences.
3.2 Interaction model
The interaction model demonstrated that the relationship between SEP and health outcomes is more complex than suggested by the basic model. A significant interaction was found between SEP and CHD prevalence (t-values ranging from 7.69 to 23.25 for different SEP levels), indicating that the effect of SEP on CHD prevalence differs from its effect on hypertension. For example, while SEP had a strong negative effect on hypertension prevalence, this effect was moderated when considering CHD, particularly in higher SEP levels. The interaction model showed a much better fit (marginal R2 = 0.902, conditional R2 = 0.932, AIC = 20,737.68), highlighting the importance of considering both health outcomes together.
3.3 District-Level random effects
The analysis identified Queens Village, Jamaica/Hollis, Wakefield/Eastchester, East Flatbush, Ocean Hill/Brownsville, and Coney Island as being within the top 10% of districts with the highest random effects. Notably, three of these districts—Queens Village, East Flatbush, and Coney Island (Figure 1)—were also highlighted by Kang et al. as areas at risk of being overlooked in public health resource allocation. These findings underscore significant variability in the impact of SEP on health outcomes within these districts, suggesting that factors beyond SEP alone are contributing to the observed health disparities.
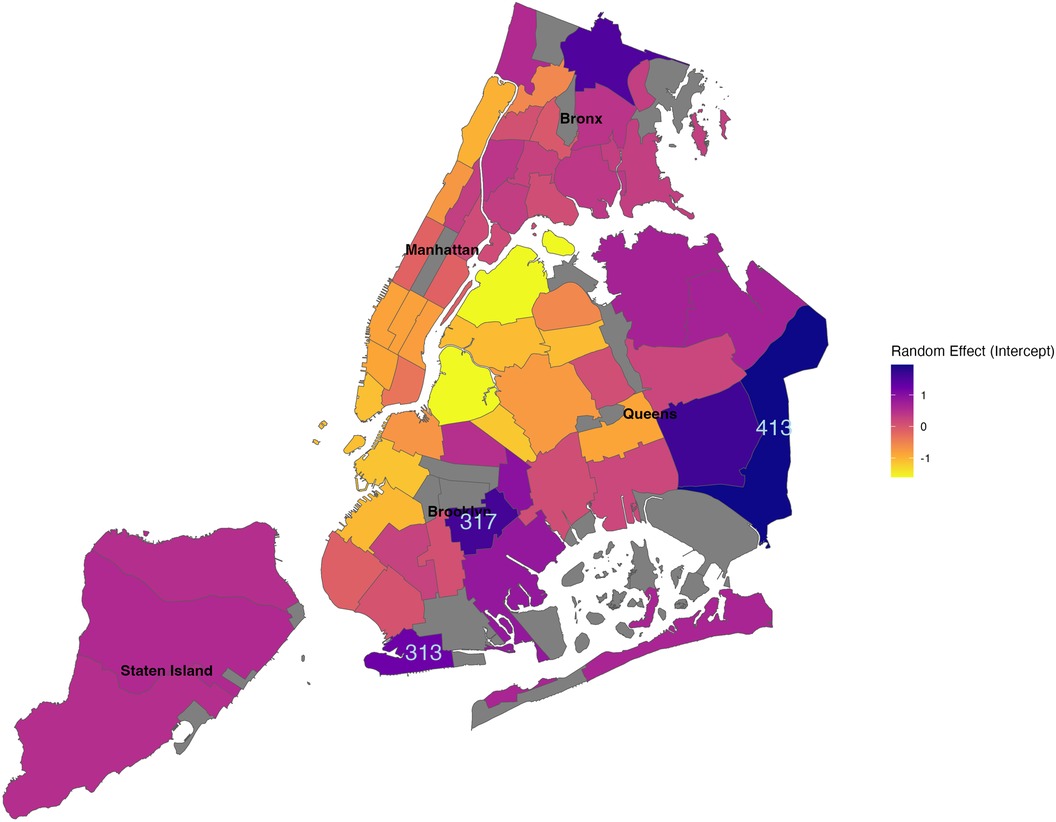
Figure 1. NYC community districts with highest random effects. District Codes: Queens Village (413), East Flatbush (317), and Coney Island (313).
3.4 Correlation between hypertension and CHD
The correlation analysis between CHD and hypertension within each community district revealed a positive slope of 0.020, indicating that as SEP increases, the correlation between these two health outcomes also increases (Figure 2). This finding suggests that in higher SEP districts, hypertension and CHD are more closely related, possibly due to shared underlying risk factors that become more pronounced in wealthier neighborhoods.
4 Discussion
While this study contributes valuable insights into the relationship between neighborhood Socioeconomic Position (SEP) and health outcomes within New York City's diverse community districts, there are key limitations that should be noted. Firstly, our investigation was confined to only two health outcomes: hypertension and coronary heart disease (CHD). Although this focus excludes other potentially relevant health outcomes, it offers several significant advantages.
By concentrating on CHD and hypertension, the study addresses two critical public health challenges that are leading causes of morbidity and mortality in the U.S., particularly among racial and ethnic minorities who bear a disproportionate burden of these conditions (40–42). Moreover, CHD and hypertension are significant comorbidities (43, 44) associated with a range of other chronic diseases, including obesity, kidney disease, and diabetes. This focus allows the study to capture key indicators that reflect broader health issues within the population.
For analytical purposes, CHD and hypertension were intentionally selected because of their well-documented association with neighborhood socioeconomic position (SEP). These conditions have been extensively studied in relation to SEP, making them reliable indicators of how socioeconomic factors influence health outcomes. Therefore, in instances where hypertension and CHD prevalence rates do not display an expected inverse relationship with neighborhood SEP, it suggests that other factors may be at play and need further investigation.
This targeted approach enables a more precise examination of the well-established associations between SEP and the specified health conditions, contributing to a deeper understanding of how socioeconomic disparities manifest in these areas. It also allows for methodologically rigorous analysis, ensuring the reliability and clarity of the results, and provides actionable insights that can inform targeted public health interventions, particularly in community districts where SEP alone does not fully account for the high prevalence of these conditions.
While expanding the range of health outcomes studied could provide a more comprehensive understanding of SEP's impact on public health, the focused analysis of CHD and hypertension lays a strong foundation for future research. Future studies could build on these findings by incorporating additional health conditions, environmental exposures such as neighborhood-level PM 2.5 estimates, and other socio-economic data such as in- and out-migration trends across New York City. These factors are crucial as they may further elucidate the pathways through which SEP influences health outcomes, potentially uncovering more complex interactions and helping to identify additional areas for targeted public health interventions.
Secondly, the study's analysis did not include controls for individual-level confounders due to the nature of the data and persistent limitations in accessing small-area health data. Key demographic and socioeconomic factors such as race, age, sex, income, and insurance status—each of which could significantly influence health outcomes—were not accounted for in our analysis. This limitation arises from the reliance on aggregate health data at the census tract level, which does not provide a breakdown by race, age, sex, or other critical variables. Consequently, restricted access to individual census tract level data limited our ability to conduct an analysis that incorporates these important confounders. Including individual-level health data at census tract level would enable a more detailed and accurate assessment of the factors contributing to health disparities across different neighborhoods.
Despite the outlined limitations, the findings from the linear mixed-effects models offer significant insights into the relationship between neighborhood Socioeconomic Position (SEP) and the prevalence of hypertension and coronary heart disease across New York City's community districts. By employing both a basic model and an interaction model, this study not only confirms the expected negative association between SEP and these health outcomes but also uncovers complexities that warrant further investigation.
The basic LMM model demonstrated a statistically significant negative relationship between SEP and the prevalence of both hypertension and CHD, indicating that as SEP increases, the prevalence of these health conditions decreases. This finding is consistent with existing literature, which shows that higher socioeconomic status is generally linked to better health outcomes. However, random effects analysis highlights that certain community districts diverge from this overall trend, suggesting that additional factors beyond SEP are influencing health outcomes in some districts more than others. The random effects analysis partially compensates for the lack of control of key confounders by effectively identifying districts where the expected inverse relationship between SEP and the studied health outcomes does not hold. Though this is not ideal, it was the best option considering the limitations of the aggregate health dataset.
The interaction model provided a deeper understanding of how the relationship between SEP and health outcomes varies between hypertension and CHD. The inclusion of an interaction term between SEP and health outcomes revealed that the negative effect of SEP on CHD prevalence is more pronounced than on hypertension. This suggests that socioeconomic factors might play a more critical role in mitigating CHD compared to hypertension. The model's strong goodness-of-fit metrics (R2 = 0.90 for the marginal model and R2 = 0.93 for the conditional model) further support the importance of considering the interplay between SEP and specific health outcomes.
Random effects analysis identified Queens Village, Jamaica/Hollis, Wakefield/Eastchester, East Flatbush, Ocean Hill/Brownsville, and Coney Island as falling within the top 10% of districts with the highest random effects. Notably, three of these districts—Queens Village, East Flatbush, and Coney Island—were also highlighted by Kang et al. as areas at risk of being overlooked in public health resource allocation. The overlap between the findings of this study and those of Kang et al. underscores the need for a more granular approach to analyzing health disparities. Another possibility to consider is that these findings could suggest that districts with high random effects may contain a few neighborhoods (census tracts) with disproportionately high prevalence of CHD and hypertension and these neighborhoods could significantly contribute to the overall high random effect observed at the district level. While Kang et al. focused on districts with moderate disparities that might be neglected under broader resource allocation strategies, the current study adds depth by indicating that in the moderate disparity districts, the drivers of health disparities may not be as closely tied to socioeconomic factors as in high disparities districts. By concentrating on hypertension and coronary heart disease—conditions strongly influenced by SEP, as supported by the findings of this study and previous studies— it is reasonable to presume that the outlier high random effects districts are unique cases. Therefore, a qualitative case study of Queens Village, East Flatbush, and Coney Island is recommended to further explore these findings.
This observation is a key contribution to the current research. It suggests that public health officials and policymakers should investigate neighborhood characteristic variability of the highlighted community districts more closely to identify other underlying factors that may be contributing to poor health outcomes. Possible factors could include exposure to environmental toxins, access to healthcare, social cohesion, or other neighborhood-specific variables that are not captured by SEP. By providing this more refined analysis, the study offers a potential starting point for targeted public health interventions, especially in districts where SEP does not significantly account for the observed health disparities.
This study advances health disparities research by identifying specific districts where the causes of poor health outcomes are likely multifaceted and not solely attributable to socioeconomic factors. This refined focus can help direct more precise public health efforts, ensuring that interventions are tailored to the unique needs of each district and addressing the root causes of health disparities more effectively.
Data availability statement
Publicly available datasets were analyzed in this study. This data can be found here: (Health Data) https://data.cdc.gov/500-Cities-Places/PLACES-Local-Data-for-Better-Health-Census-Tract-D/cwsq-ngmh/about_data (2-ACS Data) https://data.census.gov/.
Ethics statement
Ethical approval was not required for the study involving humans in accordance with the local legislation and institutional requirements. Written informed consent to participate in this study was not required from the participants or the participants’ legal guardians/next of kin in accordance with the national legislation and the institutional requirements.
Author contributions
CM-I: Writing – original draft, Visualization, Methodology, Investigation, Formal Analysis, Data curation, Conceptualization. LW: Writing – review & editing, Validation, Supervision, Formal Analysis. TO: Writing – review & editing, Validation, Supervision.
Funding
The author(s) declare that no financial support was received for the research, authorship, and/or publication of this article.
Conflict of interest
The authors declare that the research was conducted in the absence of any commercial or financial relationships that could be construed as a potential conflict of interest.
Publisher's note
All claims expressed in this article are solely those of the authors and do not necessarily represent those of their affiliated organizations, or those of the publisher, the editors and the reviewers. Any product that may be evaluated in this article, or claim that may be made by its manufacturer, is not guaranteed or endorsed by the publisher.
References
1. Northridge ME, Sclar ED, Biswas P. Sorting out the connections between the built environment and health: a conceptual framework for navigating pathways and planning healthy cities. J Urban Health. (2003) 80(4):556–68. doi: 10.1093/jurban/jtg064
2. Mohnen SM, Schneider S, Droomers M. Neighborhood characteristics as determinants of healthcare utilization- A theoretical model. Health Econ Rev. (2019) 9(1):6–7. doi: 10.1186/s13561-019-0226-x
3. Piccolo RS, Duncan DT, Pearce N, McKinlay JB. The role of neighborhood characteristics in racial/ethnic disparities in type 2 diabetes: results from the Boston area community health (BACH) survey. Soc Sci Med. (2015) 130:79–90. doi: 10.1016/j.socscimed.2015.01.041
4. Darden J, Rahbar M, Jezierski L, Li M, Velie E. The measurement of neighborhood socioeconomic characteristics and black and white residential segregation in metropolitan Detroit: implications for the study of social disparities in health. Ann Assoc Ame Geogr. (2010) 100:154–5. doi: 10.1080/00045600903379042
5. Schnake-Mahl AS, Jahn JL, Subramanian SV, Waters MC, Arcaya M. Gentrification, neighborhood change, and population health: a systematic review. J Urban Health. (2020) 97(1):1–25. doi: 10.1007/s11524-019-00400-1
6. Galster GC. The mechanism(s) of neighbourhood effects: theory, evidence, and policy implications. In: van Ham M, Manley D, Bailey N, Simpson L, Maclennan D, editors. Neighbourhood Effects Research: New Perspectives. New York: Springer (2012). p. 23–56. doi: 10.1007/978-94-007-2309-2_2
7. Health NC for CDP and HP (US) O on S and. How Neighborhoods Shape Health and Opportunity. 2021 [cited 2024 14]; Available online at: https://www.ncbi.nlm.nih.gov/books/NBK568862/
8. Black JL, Macinko J, Dixon LB, Fryer GE. Neighborhoods and obesity in New York city. Health Place. (2010) 16(3):489–99. doi: 10.1016/j.healthplace.2009.12.007
9. Smith GS, McCleary RR, Thorpe RJ. Racial disparities in hypertension prevalence within US gentrifying neighborhoods. Int J Environ Res Public Health. (2020) 17(21):7889. doi: 10.3390/ijerph17217889
10. Kim D, Glazier RH, Zagorski B, Kawachi I, Oreopoulos P. Neighbourhood socioeconomic position and risks of major chronic diseases and all-cause mortality: a quasi-experimental study. BMJ Open. (2018) 8(5):e018793. doi: 10.1136/bmjopen-2017-018793
11. Neighborhood boundaries in health data. [cited 2024 Mar 13]. Available online at: https://a816-dohbesp.nyc.gov/IndicatorPublic/data-stories/geographies/
12. Ogojiaku CN, Allen JC, Anson-Dwamena R, Barnett KS, Adetona O, Im W, et al. The health opportunity Index: understanding the input to disparate health outcomes in vulnerable and high-risk census tracts. Int J Environ Res Public Health. (2020) 17(16):5767. doi: 10.3390/ijerph17165767
13. Kang JX, Seligson AL, Dragan KL. Peer reviewed: identifying New York city neighborhoods at risk of being overlooked for interventions. Prev Chronic Dis. (2020) 17:1–16. doi: 10.5888/pcd17.190325
14. Kalogeropoulos AP, Butler J. Worsening cardiovascular disease epidemiology in the United States: the time for preparation is now. J Am Coll Cardiol. (2022) 80(6):579–83. doi: 10.1016/j.jacc.2022.05.035
15. Mohebi R, Chen C, Ibrahim NE, McCarthy CP, Gaggin HK, Singer DE, et al. Cardiovascular disease projections in the United States based on the 2020 census estimates. J Am Coll Cardiol. (2022) 80(6):565–78. doi: 10.1016/j.jacc.2022.05.033
16. White-Williams C, Rossi LP, Bittner VA, Driscoll A, Durant RW, Granger BB, et al. Addressing social determinants of health in the care of patients with heart failure: a scientific statement from the American Heart Association. Circulation. (2020) 141(22):e841–63. doi: 10.1161/CIR.0000000000000767
17. Patel SA, Krasnow M, Long K, Shirey T, Dickert N, Morris AA. Excess 30-day heart failure readmissions and mortality in black patients increases with neighborhood deprivation. Circ Heart Fail. (2020) 13(12):E007947. doi: 10.1161/CIRCHEARTFAILURE.120.007947
18. Clennin MN, Daugherty SL. Neighborhoods and health: peering beyond the clinic walls. Circ Heart Fail. (2020) 13(12):E008003. doi: 10.1161/CIRCHEARTFAILURE.120.008003
19. Powell-Wiley TM, Baumer Y, Baah FO, Baez AS, Farmer N, Mahlobo CT, et al. Social determinants of cardiovascular disease. Circ Res. (2022) 130(5):782–99. doi: 10.1161/CIRCRESAHA.121.319811
20. Wallach JD, Serghiou S, Chu L, Egilman AC, Vasiliou V, Ross JS, et al. Evaluation of confounding in epidemiologic studies assessing alcohol consumption on the risk of ischemic heart disease. BMC Med Res Methodol. (2020) 20(1):1–10. doi: 10.1186/s12874-020-0914-6
21. Ain QU, Sarfraz M, Prasesti GK, Dewi TI, Kurniati NF. Confounders in identification and analysis of inflammatory biomarkers in cardiovascular diseases. Biomolecules. (2021) 11(10):1464. doi: 10.3390/biom11101464
22. Levy D, Larson MG, Vasan RS, Kannel WB, Ho KK. The progression from hypertension to congestive heart failure. JAMA. (1996) 275(20):1557–62. doi: 10.1001/jama.1996.03530440037034
23. Benjamin EJ, Muntner P, Bittencourt MS. Heart disease and stroke statistics-2019 update: a report from the American Heart Association. Circulation. (2019) 139:e56–528. doi: 10.1161/CIR.0000000000000659
24. Social Explorer. [cited 2024 Aug 15]. Available online at: https://www.socialexplorer.com/tables/ACS2020_5yr
25. Johnston WR. Assessing the benefits of a rising tide: educational attainment and increases in neighborhood socioeconomic advantage. Soc Sci Res. (2017) 62:335–49. doi: 10.1016/j.ssresearch.2016.08.015
26. Ross CE, Mirowsky J. Neighborhood socioeconomic status and health: context or composition? City & Community. (2008) 7(2):163–79. doi: 10.1111/j.1540-6040.2008.00251.x
27. Robinette JW, Charles ST, Gruenewald TL. Neighborhood socioeconomic status and health: a longitudinal analysis. J Community Health. (2017) 42(5):865. doi: 10.1007/s10900-017-0327-6
28. Nocon M, Keil T, Willich SN. Education, income, occupational status and health risk behaviour. J Public Health (Bangkok). (2007) 15(5):401–5. doi: 10.1007/s10389-007-0120-6
29. Coffee NT, Lockwood T, Hugo G, Paquet C, Howard NJ, Daniel M. Relative residential property value as a socio-economic status indicator for health research. Int J Health Geogr. (2013) 12(1):1–10. doi: 10.1186/1476-072X-12-22
30. Samarin M, Sharma M. A typology of U.S. metropolises by rent burden and its major drivers. GeoJournal. (2023) 88(5):1. doi: 10.1007/s10708-023-10898-3
31. Pollack CE, Griffin BA, Lynch J. Housing affordability and health among homeowners and renters. Am J Prev Med. (2010) 39(6):515–21. doi: 10.1016/j.amepre.2010.08.002
32. Mehdipanah R. Without affordable, accessible, and adequate housing, health has No foundation. Milbank Q. (2023) 101(S1):419–43. doi: 10.1111/1468-0009.12626
33. Kolak M, Bhatt J, Park YH, Padrón NA, Molefe A. Quantification of neighborhood-level social determinants of health in the continental United States. JAMA Netw Open. (2020) 3(1):e1919928. doi: 10.1001/jamanetworkopen.2019.19928
34. Goitia JJ, Onwuzurike J, Chen A, Wu YL, Shen AYJ, Lee MS. Association between vehicle ownership and disparities in mortality after myocardial infarction. Am J Prev Cardiol. (2023) 14:100500. doi: 10.1016/j.ajpc.2023.100500
35. PLACES: Census Tract Data (GIS Friendly Format), 2022 release | Data | Centers for Disease Control and Prevention. [cited 2024 Aug 15]. Available online at: https://data.cdc.gov/500-Cities-Places/PLACES-Census-Tract-Data-GIS-Friendly-Format-2022-/shc3-fzig/about_data
36. Census - Download and Metadata. [cited 2024 Aug 18]. Available online at: https://www.nyc.gov/site/planning/data-maps/open-data/census-download-metadata.page
37. Oh GC, Cho HJ. Blood pressure and heart failure. Clin Hypertens. (2020) 26(1):1–8. doi: 10.1186/s40885-019-0132-x
38. Lloyd-Jones DM, Larson MG, Leip EP, Beiser A, D'Agostino RB, Kannel WB, et al. Lifetime risk for developing congestive heart failure: the Framingham heart study. Circulation. (2002) 106:3068–72. doi: 10.1161/01.CIR.0000039105.49749.6F
39. Di Palo KE, Barone NJ. Hypertension and heart failure: prevention, targets, and treatment. Heart Fail Clin. (2020) 16(1):99–106. doi: 10.1016/j.hfc.2019.09.001
40. Geronimus AT, Hicken M, Keene D, Bound J. “Weathering” and age patterns of allostatic load scores among blacks and whites in the United States. Am J Public Health. (2006) 96(5):826–33. doi: 10.2105/AJPH.2004.060749
41. Cockerham WC, Hamby BW, Oates GR. The social determinants of chronic disease. Am J Prev Med. (2017) 52(1):S5–12. doi: 10.1016/j.amepre.2016.09.010
42. Simons RL, Lei MK, Klopack E, Zhang Y, Gibbons FX, Beach SRH. Racial discrimination, inflammation, and chronic illness among African American women at midlife: support for the weathering perspective. J Racial Ethn Health Disparities. (2021) 8(2):339–49. doi: 10.1007/s40615-020-00786-8
43. Zaki N, Alashwal H, Ibrahim S. Association of hypertension, diabetes, stroke, cancer, kidney disease, and high-cholesterol with COVID-19 disease severity and fatality: a systematic review. Diab Metab Syndr. (2020) 14(5):1133–42. doi: 10.1016/j.dsx.2020.07.005
Keywords: health geography, social determinants of health (SDOH), neighborhoods, health disparities, public health
Citation: Martin-Ikpe C, Were LPO and Okoror TA (2024) The granular landscape of health disparities: a socio-spatial examination of chronic disease prevalence within New York City's community districts. Front. Environ. Health 3:1426585. doi: 10.3389/fenvh.2024.1426585
Received: 1 May 2024; Accepted: 11 September 2024;
Published: 25 September 2024.
Edited by:
Lutchmie Narine, Syracuse University, United StatesReviewed by:
Qian Zhiyuan, Changzhou University, ChinaGabriel Alonso González Medina, Pontificia Universidad Católica de Chile, Chile
Copyright: © 2024 Martin-Ikpe, Were and Okoror. This is an open-access article distributed under the terms of the Creative Commons Attribution License (CC BY). The use, distribution or reproduction in other forums is permitted, provided the original author(s) and the copyright owner(s) are credited and that the original publication in this journal is cited, in accordance with accepted academic practice. No use, distribution or reproduction is permitted which does not comply with these terms.
*Correspondence: Cordelia Martin-Ikpe, Y21hcnRpbmlrcGVAYmluZ2hhbXRvbi5lZHU=