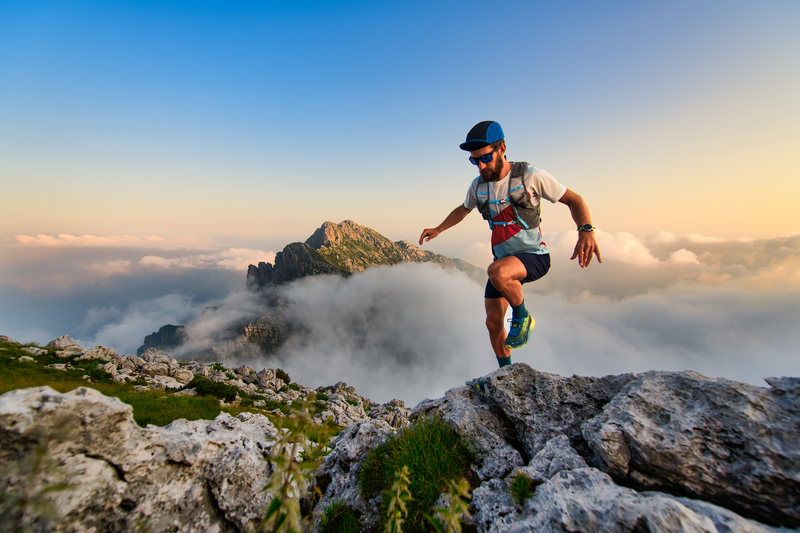
94% of researchers rate our articles as excellent or good
Learn more about the work of our research integrity team to safeguard the quality of each article we publish.
Find out more
ORIGINAL RESEARCH article
Front. Environ. Econ. , 06 February 2025
Sec. Agricultural Economics
Volume 4 - 2025 | https://doi.org/10.3389/frevc.2025.1519767
This article is part of the Research Topic Sustainable Innovations in Agriculture: Economic Analysis of Climate Smart Practices View all 3 articles
Introduction: Black soldier fly larvae (BSFL) are increasingly promoted as cost-effective, and environmentally friendly source of protein in animal nutrition. However, there is limited information about farmers' adoption rates and the factors influencing their adoption decisions in Sub-Saharan Africa (SSA). This article assesses livestock farmers' actual and potential adoption rates of BSFL and determinants of their adoption decisions.
Methods: In this paper, we used the treatment effect framework approach on data collected from 1,885 fish, poultry, and pig smallholder farmers in five cities of four West and Central African countries, including Kinshasa and Bukavu in the Democratic Republic of Congo (DRC), Accra in Ghana, Bamako in Mali, and Niamey in Niger.
Results and discussion: The results show that about 20 percent of surveyed farmers are aware of BSFL as a protein source in animal feed, and the actual adoption rate of BSFL is four percent. However, the treatment effect analysis showed that the adoption rate could quadruple if all farmers were aware. This result suggests that successful awareness creation can boost the actual adoption of BSFL, which currently stands at four percent. The awareness creation should target educated livestock farmers with access to group membership, credit, extension services, and diversified income sources to influence their decisions to adopt BSFL as a source of protein in animal feed.
Insufficient protein consumption is Africa's primary source of malnutrition as animal protein is too expensive for most people (Schönfeldt and Hall, 2012; Moughan, 2021). While the protein requirement is 0.7 g per kilogram body weight per day, the consumption level in most Sub-Saharan African (SSA) countries falls under this threshold as most consumers cannot afford meat products (Schönfeldt and Hall, 2012; OECD-FAO, 2021; Font-i-Furnols, 2023). The low consumption level is due to the high cost of meat products primarily caused by the high costs of animal feed protein ingredients, including fishmeal and soybean, which hampers the profitability and growth of local meat production (Chia et al., 2019; Hu et al., 2023).
Recently, interest in insects has been growing as a novel, cost-efficient, and environmentally friendly technology as an alternative protein source in animal feed (Franco et al., 2021a,b; Suloma et al., 2014; Weththasinghe et al., 2022; Romano et al., 2023; Raman et al., 2022). In particular, black soldier fly larvae (BSFL) are increasingly promoted as a sustainable ingredient in livestock diets, especially poultry, pigs, and fish, for several reasons. First, BSFL is a powerful tool for biowaste recycling as the larvae feed on various biowaste materials (Weththasinghe et al., 2022; Romano et al., 2023; Raman et al., 2022; Siddiqui et al., 2022; Yildirim-Aksoy et al., 2020; Liu et al., 2018; Scieuzo et al., 2023; Surendra et al., 2020). Second, by feeding on biowaste materials, BSFL is a climate-smart technology as it contributes to reducing Greenhouse Gas Emissions generated by the decomposition of urban waste that otherwise would be dumped into unregulated landfills (Liu et al., 2018; Scieuzo et al., 2023; Surendra et al., 2020; Jung et al., 2022) Third, BSFL has a high protein content, and their lipid is useful in cosmetics and personal care products, and biodiesel (Hu et al., 2023; Franco et al., 2021a,b; Raman et al., 2022; Labella et al., 2024). Finally, BSFL in animal feed has benefits such as reducing the risk of animal-transmitted disease, improving overall health and growth of livestock, and significantly reducing the cost of livestock production (Chia et al., 2019; Romano et al., 2023; Yildirim-Aksoy et al., 2020). Based on this background, BSFL production will likely increase in the coming years as a sustainable substitute for conventional protein sources.
Although the BSFL technology has received considerable attention in the rest of the world, little is known about its awareness and adoption rates and their determinants among livestock farmers in SSA (Olutegbe and Ojuoluwa, 2022). The purpose of this paper is to investigate the adoption of BSFL among livestock farmers and factors influencing their decision to adopt BSFL in five cities of four West and Central African countries, including Kinshasa and Bukavu in the Democratic Republic of Congo (DRC), Accra in Ghana, Bamako in Mali, and Niamey in Niger as part of the Norwegian Agency for Development Cooperation (NORAD)-funded BSF for Bio- circular Economy and Sustainability (BBEST) project. Our work contributes to the growing but sparse literature on BSF-derived technologies.
The specific objectives are 2-fold:
• To estimate the true population adoption rate of BSFL, i.e., the adoption rate that would result if all livestock farmers were aware of the BSFL (Diagne and Demont, 2007; Dontsop Nguezet et al., 2011; Dontsop Nguezet et al., 2013; Kathage et al., 2016; Simtowe et al., 2011).
• To identify the factors that explain the non-adoption in cases where livestock farmers were aware of the BSFL technology but did not adopt it.
To the best of our knowledge, this is the first study to thoroughly investigate the awareness and actual and potential adoption of BSFL as an animal protein source in SSA.
This study relies on a household survey conducted as part of the Norwegian Agency for Development Cooperation (NORAD)-funded BSF for Bio-circular Economy and Sustainability (BBEST) project. Its goal is to improve the livelihoods of smallholder chicken, fish, pigs, and vegetable farmers and contribute to improved urban sanitation and climate change mitigation in four African countries. The BBEST project started in Ghana, Mali, and Niger in 2021 and expanded to DRC in 2022. This project focuses on five cities in four countries, which constitute our case studies and include: Accra, Bamako, Niamey, Kinshasa, and Bukavu. The first four cities represent the capital cities of the studied countries with high populations and high production of urban waste. The fifth city is Bukavu in DRC; it was chosen for two reasons. First, to capture the intra country contrast between west and east, as Bukavu is located in eastern DRC with a different context backgroup compared to Kinshasa. Second, Bukavu was chosen as a medium-to-high altitude city to contrast other cities all at low altitudes.
Figure 1 summarizes the Black Soldier fly larvae cycle and derived technologies. Five steps form the classic cycle of BSF : (1) fly, (2) eggs, (3) young larvae, (4) larvae, and (5) pre-pupa and pupa. However, the cycle length depends on several factors, including the substrate, the cultivation environment, temperature, and the strain, among many others (Makate et al., 2019; Daberkow and McBride, 2003; Liu et al., 2017). What matters here is the number of derived products from BSF production. First, the larvae, which constitute the main product, are an essential source of protein for animals and are processed in many forms depending on the end-uses. When dried and powdered, the BSFL is a suitable meal for chicken, pig, and other small livestock; when pelleted, the BSFL is ideal for fish due to its floatier characteristics (Weththasinghe et al., 2022; Romano et al., 2023; Raman et al., 2022; Siddiqui et al., 2022; Yildirim-Aksoy et al., 2020). Second, the BSFL has a higher lipid content; when processed, the derived product is insect oil with great health benefits (Hu et al., 2023; Franco et al., 2021a,b). Third, after the lifecycle, the excrement of the larvae and dyed flies, often called BSF frass (BSFF), are an essential source of soil nutrients (Liu et al., 2017; Tomberlin et al., 2009; Lomonaco et al., 2024; Myers et al., 2014). The latter product positively impacts vegetables, potatoes, and other crops of the same category to increase productivity when used as organic fertilizer. Finally, the remaining feedstock constitutes a very rich organic compost. In this study, we focused on the protein meal product.
Figure 1. Black soldier fly lifecycle and derived products. Three main products can be identified throughout the black soldier fly's (BSF) lifecycle. First, the key product is the BSF protein meal, which is directly obtained from harvested BSFL. Second, the BSF soil nutrient is composed of BSF frass and the remaining feedstock, and it can be utilized as organic fertilizer. Lastly, the BSF oil can be used in various products such as cosmetics, personal care items, and even biodiesel. Each of these products can be obtained at different stages of the BSF lifecycle, which has been condensed into five stages. Authors' conception.
Under partial awareness, which means only a portion of the study population is aware of the BSFL technology, the estimation of the adoption rate outside the treatment effect framework is subject to some bias (Diagne and Demont, 2007). The treatment effect framework refers to the causal effect of awareness on BSFL adoption among livestock farmers (Ravallion, 2001). Concretly, the treatment effect framework is relevant in this study for two reasons. First, although the valorization of BSF technology in SSA has recorded significant progress over the last few years, it has not been widely disseminated (Raman et al., 2022; Romano et al., 2023). Thus, a small fraction of the farming community is aware of its existence. Second, farmers' exposure to the BSF technologies is not random, implying selection biases due to self-selection. Therefore, relying on the treatment effect framework allows us to control for non-exposure or partial awareness and selection biases while estimating true population adoption rates and the determinants of adoption (Diagne and Demont, 2007; Dontsop Nguezet et al., 2011, 2013; Kathage et al., 2016; Simtowe et al., 2011).
Conceptually, under the treatment effect framework, the true population adoption rate corresponds to the adoption rate that would have happened if all farmers were aware of BSFL technology, and it is measured by the average treatment effect (ATE), the first indicator of interest in our analysis. The treatment is “awareness,” such that aware farmers are the “treated group” while those unaware are the “control group.” Empirically, we used the propensity score matching (PSM) technique, which is robust in controlling for the selection bias based on observable characteristics. The PSM is a causal effect approach that simulates an experimental study by creating an artificial control group from the non-exposure group using livestock farmers' propensity score or likelihood to be aware of the BSFL technology (Dontsop Nguezet et al., 2011, 2013; Kathage et al., 2016; Simtowe et al., 2011).
For concreteness, we focus on the adoption of BSF-based animal feed as the outcome variable and awareness as the treatment variable. Under the ATE framework, each farmer is assumed to have two potential outcomes: with and without exposure to technology. This study used a dummy variable to capture the adoption status because we are interested in analyzing the factors that explain the likelihood of being an adopter rather than the demand for BSFL that can be captured using a continuous variable, such as the quantity used of BSFL (Etzioni et al., 2020).
After estimating the true population adoption rate (ATE)—the adoption rate that would result if all livestock farmers were aware of the BSFL as animal feed protein source—the other estimations of interest are: first, the difference between the ATE and the actual adoption rate—corresponding to the observed adoption rate within the sample—corresponds to the population adoption gap, reflecting the unmet population demand for the technology due to a lack of information (Diagne and Demont, 2007). Secondly, the adoption outcome in the exposed subpopulation corresponds to the average treatment effect on the treated (ATE1 or ATT). Finally, the difference between the ATE and ATE1 is the population selection bias (PSB) that measures the extent to which awareness of the BSF-based animal feed is not randomly distributed.
Practically, following Diagne and Demont (2007), let the status of exposure to or awareness of BSFL technology Eh be represented by the latent response model in Equation 1:
Where Eh is the observed awareness of BSFL technology, denotes a continuous latent variable, meaning not directly observed, μ is a vector of parameters to be estimated, and μh is the error term. The binary response “adoption of BSFL” Ah is also defined as follows in Equations 3 and 4:
Where Ah is the main outcome variable and represents a continuous latent variable, μ represents a vector of parameters to be estimated, ϕ is the coefficient of the endogenous treatment dummy, and μh is the error term.
The treatment effect for the household hth is measured by the difference between the actual outcome value Ah1, with adoption, and the counterfactual outcome Ah0, without adoption. Therefore, by definition, the expected population adoption impact of exposure to the new technology is given by the mean value E(Ah1 − Ah0), the ATE. The problem, in this case, is that the two responses A1 and A0 for the hth household can not be observed simultaneously. Besides, Ah is assumed to depend on the endogenous dummy Eh and a vector of explanatory variables Xh. The endogenous dummy Eh also depends on a vector of explanatory variables, Zh. There is a possibility that vectors Xh and Zh share elements. Direct estimation of Equation 3 and interpreting ϕ as the causal effect of awareness on adoption would result in biased estimates due to unobserved endogeneity. For more details on the causal effect terminology, see Ravallion (2001).
However, in the adoption context, the estimation of the ATE is based generally on the conditional independence (CI) assumption (Diagne and Demont, 2007; Dontsop Nguezet et al., 2011, 2013; Kathage et al., 2016). This assumption states that the treatment status Eh is independent of the potential outcomes A0 and A1 conditional on an observed set of covariates P(Ah = 1|E, X) = P(Ai = 1|X); j = 0, 1. The conditional independence assumption in this context does not imply that unobservable characteristics cannot influence the treatment variable Eh and the outcomes A0 and A1 but that the existence of unobservable does not bias estimates of the treatment effects. Hence, instrumental variables are not required to correct the potential endogeneity issue (Kathage et al., 2016; Simtowe et al., 2011).
As mentioned above, in this study, we relied on the propensity score matching (PSM) approach to estimate the ATE estimators using the Kernel Matching approach following Kathage et al. (2016) and Simtowe et al. (2011). We performed additional robustness checks on the estimation strategy using other matching methods, such as the nearest neighbor (NN) matching and the augmented inverse probability weighted approach, which is a double-robust estimator of the causal effect [see Kurz (2022) for further details].
Estimating the determinants of awareness and adoption is crucial as they provide valuable information regarding the factors explaining livestock farmers' exposure to BSFL technology and their decision to adopt it. The factors influencing livestock farmers' awareness differ significantly from those affecting the adoption decision. We relied on the logit regression for this purpose and justified and presented variables in Section Variable description.
We used STATA software for estimation.
The explained variables in this paper are awareness and adoption of BSFL technology by livestock farmers, all of which are dummy variables evaluated by “aware = 1, unaware = 0” and “adopt = 1, not adopt = 0”.
The core explanatory variables of this paper are grouped into two categories: farmers' access to development services and social networks and income and asset ownership. Access to information and knowledge about agricultural technologies is necessary for adoption in Africa (Kathage et al., 2016; Manyong et al., 2022; Nguru et al., 2021). Empirically, farmer groups and extension services have been given a particular interest and remain solid learning channels through which the diffusion of new technologies occurs, mainly free of charge (Bell et al., 2018; Manda et al., 2016; Khataza et al., 2018). Moreover, people's livelihoods can be enhanced when they are members of groups due to local solidarity (Manyong et al., 2022). Information and communication technologies (ICT), such as mobile phones, can improve efficiency (Nyamuhirwa et al., 2022). We focused on group membership, access to extension, and mobile phone ownership as informational factors determining awareness and adoption.
Income and asset ownership are key determinants of adopting agricultural technologies because new technologies require complementary resources (Nguru et al., 2021; Manda et al., 2016; Tambo and Abdoulaye, 2012). We considered livestock ownership and the number of sources of income as variables. Several studies argue that livestock is a form of insurance as it can be used as collateral, reducing farmers' risk aversion as adopting new technologies is not risk-free (Manda et al., 2016; Autio et al., 2021; Makate et al., 2019; Daberkow and McBride, 2003). Similarly, the more diversified the household income, the less risk-averse a farmer is so he can test new ideas and the latest knowledge. We measured the diversity of income in terms of the number of sources of income owned by a farmer, and livestock ownership is a dummy variable applied to four types of livestock, including cattle, small livestock—such as goats and pigs –, poultry, and fish.
To ensure the accuracy of the estimation results when using PSM to estimate the processing effects, we included as many covariates as possible of those that affect the awareness and adoption of new technologies as the control variables. Based on relevant literature search results, this study includes the head of household characteristics, location of the farmers, and the livestock farmer category (fish, poultry, and pig producers) as identified during the data collection activity. We included nine control variables that affect farmers' awareness and technology adoption status: status of the respondent (head of the household or not), sex of the respondent, age of the household head, education, marital status, household size, livestock farmer category, country of location, and city. Tables 1, 2 provide the descriptive statistics of each variable by awareness and adoption status.
Sampling and data collection followed a multistage sampling approach. In the first stage, we randomly selected 3 to 5 sub-urban districts located within a radius of 50 km (about 31.07 mi) from the point where the BSF facility was planned to be built under the BBEST project in each city and which is known for a strong engagement in the production of fish, poultry, and pigs as well as the presence of significant volume of biowastes to make the unit operational. These suburban districts formed the primary sampling unit from which smallholder farmers were selected. We pre-identified the corresponding census of farmers engaged in the selected value chains, constituting the BSF-based products' end users/buyers. The lists of farmers were obtained from farmers' unions, cooperatives, and associations to which non-farmer members were added after using a snowball identification approach. Concretely, the snowball identification approach allowed us first to identify members of cooperatives and ask them to name their peers who are not members but engaged in fish, poultry, or pig farming in their neighborhood.
In the second stage, from the selected suburban districts, a minimum of at least 150 livestock farmers were randomly selected in each value chain in each city. The pig value chain counted only for Kinshasa and Bukavu in DRC and not for the West African urban cities for cultural reasons. The survey was conducted between March and October 2023 and reached a total number of 933 small producers in Kinshasa and Bukavu in DRC, 325 producers in Accra, Ghana, 315 in Niamey, Niger, and 312 in Bamako, Mali, forming a total sample size of 1,885 small livestock producers (Figure 2). Among other questions, each sampled livestock farmer was specifically asked two questions: 1) whether they were aware/exposed to BSFL-based animal feed, regardless of their characteristics. 2) If the answer was yes, they were asked whether they had used the BSFL in the past years to feed their livestock. Awareness of BSFL is defined as a yes answer to the first question, and adoption of the BSFL is a yes answer to the second question.
Trained enumerators and supervisors with a minimum BSc level, some years of experience conducting socioeconomic surveys and speaking the local language, conducted face-to-face interviews. The training included theoretical sessions in classrooms followed by practical sessions in the fields. We used computing tablets, and the Ona server enabled data aggregation (www.odk.ona.io). Two-step data reviews were conducted to ensure data quality, first by supervisors at the end of each day and remotely by the survey manager, and mistakes were brought back to the attention of the field team for correction. Each enumerator received a sample of dried, powdered, and pelleted BSFL in a zipper bag for awareness creation and to stimulate livestock farmers' visual memory to capture their awareness adequately. The survey collected data on the sociodemographic characteristics of farmers, awareness and usage of BSF-based technologies, perception of derived food products of animal feed on BSF, households' assets, and access to essential services such as finance, credit, extension, and training.
The core explanatory and control variables of respondents include (i) household and farmer characteristics, (ii) access to development services and social networks, (iii) Income and livestock ownership, and (iv) contextual factors. Tables 1, 2 summarize respondents' characteristics by awareness and adoption status. All variables differ significantly between aware and unaware farmers and adopters and non-adopters. About one-fifth of surveyed farmers are aware of BSFL as a protein source in animal feed, with slight heterogeneity across countries, and about four percent ever used BSFL to feed their livestock in the population and 20 percent among aware farmers. Although the actual adoption rate of four percent is low, it shows that some BSFL activities already exist in the four countries. The latter adoption rate constitutes a baseline indicator for the BBEST project aiming to scale up the BSF technology system. We used videos, photos, and samples of BSF products to avoid confusion between BSF and other flies during the data collection.
Aware and adopter farmers tend to own cell phones, participate in farmers'groups, associations, cooperatives, or unions, and receive information about BSFL technology from family and friends, farmer groups, associations, or unions. The average household size is slightly larger among aware farmers than non-aware farmers. Aware and adopter farmers are relatively younger and are likely to own cattle, small livestock, poultry, and fishponds and dispose of a diversified source of income.
Tables 3, 4 present the estimated marginal effects of the BSFL technology awareness and adoption models. The estimated models fit well as we rejected the null hypothesis of parameters equal to zero in the two models (awareness and adoption) based on the Wald test results. Our discussion focuses on the pooled sample [column (1)], while columns (2) to (5) in Tables 3, 4 summarize results on determinants of awareness and adoption of BSFL technology by country.
As expected, all variables related to information factors were strongly associated with the awareness and adoption of BSFL system technology. First, farmers who owned cell phones were likelier to be aware of BSFL. Second, the likelihood of awareness and adoption of BSFL technology was positively associated with group membership. These results are consistent with a wide range of previous studies that have evidenced that access to information and knowledge about agricultural technologies is necessary for adoption in Africa (Kathage et al., 2016; Nguru et al., 2021). A rational explanation is that access to information is an edge in getting knowledge and adopting agricultural technologies (Rodenburg et al., 2021; Mango et al., 2017). Farmer groups remain a solid social learning channel through which farmer-to-farmer learning is effective, and information and knowledge are mainly free to access (Bell et al., 2018; Manda et al., 2016; Khataza et al., 2018).
Capital constraint constitutes another primary constraint of agricultural technology adoption, so we assessed its influence on BSFL technology awareness and adoption through livestock ownership and the number of sources of income. The latter variable was insignificantly associated with awareness and adoption (Tambo and Abdoulaye, 2012; Suri and Udry, 2022). Farmers who own poultry and fish activities were more likely to be aware of and adopt BSFL technology as a protein source in animal feed. The likelihood of awareness increased by 8% for farmers with poultry ownership and 6% for fish activity ownership, and adoption increased by 2 and 4%, respectively. This could be explained by the fact that the BSFL is widely used to feed fish and poultry compared to other types of livestock. As poultry meat is expected to represent 41% of all the protein from meat sources in 2030, BSFL will mainly be used for this sector (Suri and Udry, 2022).
Moreover, adopting agricultural technologies such as BSFL requires complementary resources (Nguru et al., 2021; Manda et al., 2016; Tambo and Abdoulaye, 2012). Livestock constitutes a form of insurance as it can be used as collateral, reducing farmers' risk aversion as adopting new technologies is not risk-free. These results are in line with several studies in Africa that have highlighted the role of capital constraint under diverse forms, such as land or off-farm income, in the adoption of agricultural technologies (Manda et al., 2016; Tambo and Abdoulaye, 2012; Makate et al., 2019; Daberkow and McBride, 2003). The number of sources of income was insignificant.
Being educated increases the likelihood of BSFL technology awareness but not of adoption. As Manda et al. (2016) emphasized, education as human capital comes into play in treating information about technologies, understanding their importance, and their utilization. Other household characteristics were negatively associated with awareness and adoption, including being the household head and the respondent's age. One could infer that younger farmers are eager to learn about no traditional technologies and, therefore, could be a good target in promoting BSF technologies.
The contextual variables were all negatively or insignificantly associated with awareness and adoption, meaning that farmers in the study countries were less likely to be aware of and adopt BSFL technologies. This finding may support a hypothesis of technological stagnation in African agriculture, as argued by Suri and Udry (2022). Even though the factors mentioned earlier substantially affect BSFL technology adoption, the adoption process in SSA is complex. It involves many factors beyond the intervention's control sphere and behavioral factors with farmer-to-farmer-specific characteristics.
Before presenting results on the predicted adoption rates under the full awareness assumption, we performed various diagnostics to check the matching quality. Figure 3 provides a visual inspection of the density distribution of the propensity scores and the overlap in the distribution of the propensity scores, indicating that the common support condition is satisfied. Moreover, the diagnostic test also shows a relatively low pseudo R2, high total bias reduction, and insignificant p-values of the LR test after matching (Table 5), which provides evidence that the proposed specification is successful in terms of balancing the distribution of the covariates between the aware and non-aware livestock farmers.
Figure 3. Common support condition and distribution of propensity scores (Source: Author's analysis).
We use the ATE estimates to predict adoption rates with and without awareness constraints for the total sample and separately by country. Table 6 summarizes the results. For the ATE indicator, we interpret the results obtained under the kernel matching model (column 3) and use the NN matching (column 4) and augmented inverse probability weighted (AIPW; column 5) for robustness check. The actual adoption rate estimations are in column (1), while the ATE1 is in column (2). Columns (6) and (7) show the population adoption gap (GAP) and Population selection bias (PSB).
The potential adoption rate within the pooled sample was 16% (column 3), while the actual adoption rate was about 4% (column 1), leading to a population adoption gap of about 11% (column 6). This result is robust under the NN matching and the AIPW models (columns 4 and 5). Column 7 shows that the population selection bias is about 4%. These results mean that the actual adoption rate of BSFL technologies would have quadrupled if all the farmers had been aware of BSFL technologies.
Although an increasing rate from 4% of the actual adoption rate to 16% under the full awareness assumption seems low, this finding is similar to previous studies. Diagne and Demont (2007) found that the adoption rate could increase from an actual rate of 4 to 18% under the full awareness assumption, a tripled rate. Similarly, Simtowe et al. (2011) found an actual adoption rate of 16% and passed to 62% under the full awareness assumption, a quadrupled rate. Finally, Dontsop Nguezet et al. (2013) found an actual adoption rate of 19% and passed to 54% under the same assumption, almost tripled. The bottom line of these studies is to have shown that increasing awareness among farmers would make the adoption rate pass from double to quadruple, as demonstrated. These findings complete the previous studies and reinforce the widespread evidence that when farmers are aware of agricultural technologies, they may adopt them and open to consuming the derived foods (Diagne and Demont, 2007; Siddiqui et al., 2022; Channa et al., 2019; Caldwell et al., 2019; Bridle et al., 2020; Ouko et al., 2022; Olutegbe and Ojuoluwa, 2022; Dontsop Nguezet et al., 2024).
Considering regions and countries, the results showed that the potential adoption rate was higher in Western African countries, as it was estimated at 20, 16, and 13% in Ghana, Mali, and Niger, respectively, compared to Central Africa, where it was at 10% in DRC (column 3). These values were consistent with the NN matching (column 4) and AIPW (column 5) results. However, the adoption gap attributed to lack of awareness was higher in Western Africa than in Central Africa, meaning that the BSFL technology remains unknown and less widespread in Western Africa. Comparing countries, we found that the adoption rate of BSFL technologies was consistently lower in DRC. Our results are robust from model to model, as shown in columns (3) to (5).
This study used the average treatment effect framework to contribute to the growing but scanty literature on BSF system technology by assessing the actual and potential adoption rate of BSFL as a protein source for animal feed amongst a sample of 1,885 smallholder livestock farmers in DRC, Ghana, Mali, and Niger. BSFL are increasingly promoted as a sustainable friendly environmenet ingredient in livestock diets, especially poultry, pigs, and fish. However, their adoption is very low in SSA, and less is known about the determinants of livestock farmers' decision to adopt BSFL. We shed light on exposure or awareness of BSFL technology as necessary to fill the BSFL adoption gap and gain from their multiple advantages in livestock farming.
We found that information-related factors, such as group membership or mobile phone, were significantly associated with both awareness and adoption, meaning farmers are more likely to be aware of and adopt BSFL technology as information about this technology directly reaches farmers. The finding highlights that BSFL adoption is a function of households' ability to access information on technology. Also, education, taken as a component of human capital, was significantly associated with awareness and adoption as information treatment about technologies is a function of farmers' human capital. Finally, we found that poultry and fish farmers were likelier to adopt BSFL.
The prediction of the adoption rate shows that the adoption rate is understated if the adoption rate is estimated without considering full awareness of the technologies. Concretely, the ATE results show that the actual adoption rate of BSFL technologies would have quadrupled if all farmers were aware. Analyzing factors that drive farmers' awareness and adoption demonstrated that successful awareness creation can boost the adoption of BSFL. Still, awareness creation alone would not be sufficient to lead to the massive adoption of BSL-derived products. Other important constraints that need to be leveraged include targeting educated farmers and those who are group members, access to credit, extension services, and income diversification to support farmers' decisions to adopt the new products that aim at sustainable livestock farming built on a bio-circular economy.
Considering regions' heterogeneity, we found that the adoption gap attributed to lack of awareness was higher in Western Africa than in Central Africa, meaning that the BSFL technologies remain unknown and less widespread in Western Africa. In sum, if more efforts could be devoted to directly providing information about the BSF technology system to farmers, its adoption gap in SSA would be somewhat filled.
This study showed good perspectives on adoption of BSFL-derived animal feed among livestock farmers in SSA as a climate-smart technology. However, it has one main empirical limitation. Since there was no technology promotion or awareness campaign formally by extension services or informally through a farmer-to-farmer dissemination mechanism, both awareness and adoption were livestock farmers' self-reports. They did not consider the intrinsic or extrinsic characteristics of BSFL but tacit knowledge. Therefore, further research is needed to advance knowledge around the socioeconomic of the BSF technology system after the implementation of the BBEST project and others and scale it up to the benefit of farmers and local communities.
The original contributions presented in the study are included in the article/Supplementary material, further inquiries can be directed to the corresponding author.
The studies involving humans were approved by International Institute of Tropical Agriculture Internal Review Board (IRB). The studies were conducted in accordance with the local legislation and institutional requirements. Written informed consent for participation was not required from the participants or the participants' legal guardians/next of kin because All participant provided their oral informed consent to participate in the survey..
D-MN: Conceptualization, Data curation, Formal analysis, Investigation, Methodology, Writing – original draft, Writing – review & editing. SF: Conceptualization, Supervision, Writing – review & editing. P-MD: Conceptualization, Supervision, Writing – review & editing. DS: Writing – review & editing. BM: Writing – review & editing. A-GK: Writing – review & editing. SZ: Writing – review & editing. TA: Conceptualization, Funding acquisition, Supervision, Writing – review & editing. VM: Conceptualization, Funding acquisition, Supervision, Writing – review & editing.
The author(s) declare financial support was received for the research, authorship, and/or publication of this article. The International Institute of Tropical Agriculture (IITA) funded conducted this study under the BBEST project funded by from the Norwegian Agency for Development Cooperation (NORAD) grant number QZA-21/019: Chicken and Fish Feed and Organic Fertilizer Value Chain Development Using BSF-Based Urban Biowaste Processing in Ghana, Mali, and Niger. The funders had no role in study design, data collection and analysis, decision to publish, or manuscript preparation of the manuscript and decision to publish.
We acknowledge the financial support received from the BBEST project, a Norwegian Agency for Development Cooperation (NORAD) funded project. Moreover, we are gratefull for the contributions of Dr. Mawufe Komi Agbodzavu and Mr. Doudou Dunia to the data collection activities in DRC and Mr. Ankrah Twumasi in Ghana. We thank farmers and enumerators from all four countries involved in the data collection. Moreover, we acknowledge all the reviewers who devoted their time to this study and provided constructive comments.
The authors declare that the research was conducted in the absence of any commercial or financial relationships that could be construed as a potential conflict of interest.
The author(s) declare that no Gen AI was used in the creation of this manuscript.
All claims expressed in this article are solely those of the authors and do not necessarily represent those of their affiliated organizations, or those of the publisher, the editors and the reviewers. Any product that may be evaluated in this article, or claim that may be made by its manufacturer, is not guaranteed or endorsed by the publisher.
The Supplementary Material for this article can be found online at: https://www.frontiersin.org/articles/10.3389/frevc.2025.1519767/full#supplementary-material
Autio, A., Johansson, T., Motaroki, L., Minoia, P., and Pellikka, P. (2021). Constraints for adopting climate-smart agricultural practices among smallholder farmers in Southeast Kenya. Agric. Syst. 194:103284. doi: 10.1016/j.agsy.2021.103284
Bell, A. R., Zavaleta Cheek, J., Mataya, F., and Ward, P. S. (2018). Do as they did: peer effects explain the adoption of conservation agriculture in Malawi. Water 10:51. doi: 10.3390/w10010051
Bridle, L., Magruder, J., McIntosh, C., and Suri, T. (2020). Experimental Insights on the Constraints to Agricultural Technology Adoption. UC Berkeley; Center for Effective Global Action. Available at: https://escholarship.org/uc/item/79w3t4ds
Caldwell, R., Lambert, R., Magruder, J., McIntosh, C., and Suri, T. (2019). Improving Agricultural Extension and Information Services in the Developing World. Durham, UK: VoxDev.
Channa, H., Chen, A. Z., Pina, P., Ricker-Gilbert, J., and Stein, D. (2019). What drives smallholder farmers' willingness to pay for a new farm technology? Evidence from an experimental auction in Kenya. Food policy 85, 64–71. doi: 10.1016/j.foodpol.2019.03.005
Chia, S. Y., Tanga, C. M., van Loon, J. J., and Dicke, M. (2019). Insects for sustainable animal feed: inclusive business models involving smallholder farmers. Curr. Opin. Env. Sust. 41, 23–30. doi: 10.1016/j.cosust.2019.09.003
Daberkow, S. G., and McBride, W. D. (2003). Farm and operator characteristics affecting the awareness and adoption of precision agriculture technologies in the US. Prec. Agric. 4, 163–177. doi: 10.1023/A:1024557205871
Diagne, A., and Demont, M. (2007). Taking a new look at empirical models of adoption: average treatment effect estimation of adoption rates and their determinants. Agric. Econ. 37, 201–210. doi: 10.1111/j.1574-0862.2007.00266.x
Dontsop Nguezet, P. M., Diagne, A., Okoruwa, O. V., Ojehomon, V., and Manyong, V. (2013). Estimating the actual and potential adoption rates and determinants of NERICA rice varieties in Nigeria. J. Crop Improv. 27, 561–585. doi: 10.1080/15427528.2013.811709
Dontsop Nguezet, P. M., Diagne, A., Okoruwa, V. O., and Ojehomon, V. (2011). Impact of improved rice technology (NERICA varieties) on income and poverty among rice farming households in Nigeria: a local average treatment effect (LATE) approach. Q. J. Int. Agric. 50, 267–291.
Dontsop Nguezet, P. M., Nyamuhirwa, D. M. A., Shiferaw, F., Manyong, V., Sissoko, D., Moussa, B., et al. (2024). Cross-country evidence of consumers' perception of food from animals fed on insects in DR Congo, Mali, and Niger. J. Agric. Food Res. 17:111243. doi: 10.1016/j.jafr.2024.101243
Etzioni, R., Mandel, M., Gulati, R., Etzioni, R., Mandel, M., and Gulati, R. (2020). “Binary and categorical outcomes,” in Statistics for Health Data Science: An Organic Approach (Cham: Springer), 65–92. doi: 10.1007/978-3-030-59889-1_4
Font-i-Furnols, M. (2023). Meat consumption, sustainability and alternatives: an overview of motives and barriers. Foods 12:2144. doi: 10.3390/foods12112144
Franco, A., Salvia, R., Scieuzo, C., Schmitt, E., Russo, A., and Falabella, P. (2021a). Lipids from insects in cosmetics and for personal care products. Insects 13:41. doi: 10.3390/insects13010041
Franco, A., Scieuzo, C., Salvia, R., Petrone, A. M., Tafi, E., Moretta, A., et al. (2021b). Lipids from Hermetia illucens, an innovative and sustainable source. Sustainability 13:10198. doi: 10.3390/su131810198
Hu, Z., Li, H., Liu, S., Xue, R., Sun, J., and Ji, H. (2023). Assessment of black soldier fly (Hermetia illucens) larvae meal as a potential substitute for soybean meal on growth performance and flesh quality of grass carp Ctenopharyngodon idellus. Anim. Nutr. 14, 425–449. doi: 10.1016/j.aninu.2023.06.006
Jung, S., Jung, J. M., Tsang, Y. F., Bhatnagar, A., Chen, W. H., Lin, K. Y. A., et al. (2022). Biodiesel production from black soldier fly larvae derived from food waste by non-catalytic transesterification. Energy 238:121700. doi: 10.1016/j.energy.2021.121700
Kathage, J., Kassie, M., Shiferaw, B., and Qaim, M. (2016). Big constraints or small returns? Explaining non-adoption of hybrid maize in Tanzania. Appl. Econ. Perspect. P. 38, 113–131. doi: 10.1093/aepp/ppv009
Khataza, R. R., Doole, G. J., Kragt, M. E., and Hailu, A. (2018). Information acquisition, learning and the adoption of conservation agriculture in Malawi: a discrete-time duration analysis. Tech. Forecast. Soc. Change 132, 299–307. doi: 10.1016/j.techfore.2018.02.015
Kurz, C. F. (2022). Augmented inverse probability weighting and the double robustness property. Med. Decis. Mak. 42, 156–167. doi: 10.1177/0272989X211027181
Labella, R., Bochicchio, R., Addesso, R., Labella, D., Franco, A., Falabella, P., et al. (2024). Germination behavior and geographical information system-based phenotyping of root hairs to evaluate the effects of different sources of black soldier fly (Hermetia illucens) larval frass on herbaceous crops. Plants 13:230. doi: 10.3390/plants13020230
Liu, X., Chen, X., Wang, H., Yang, Q., Rehman, K., Li, W., et al. (2017). Dynamic changes of nutrient composition throughout the entire life cycle of black soldier fly. PLoS ONE 12:e0182601. doi: 10.1371/journal.pone.0182601
Liu, Z., Minor, M., Morel, P. C., and Najar-Rodriguez, A. J. (2018). Bioconversion of three organic wastes by black soldier fly (Diptera: Stratiomyidae) larvae. Envir. Entomol. 47, 1609–1617. doi: 10.1093/ee/nvy141
Lomonaco, G., Franco, A., De Smet, J., Scieuzo, C., Salvia, R., and Falabella, P. (2024). Larval frass of hermetia illucens as organic fertilizer: composition and beneficial effects on different crops. Insects 15:293. doi: 10.3390/insects15040293
Makate, C., Makate, M., Mango, N., and Siziba, S. (2019). Increasing resilience of smallholder farmers to climate change through multiple adoption of proven climate-smart agriculture innovations. Lessons from Southern Africa. J. Environ. Manage. 231, 858–868. doi: 10.1016/j.jenvman.2018.10.069
Manda, J., Alene, A. D., Gardebroek, C., Kassie, M., and Tembo, G. (2016). Adoption and impacts of sustainable agricultural practices on maize yields and incomes: evidence from rural Zambia. J. Agric. Econ. 67, 130–153. doi: 10.1111/1477-9552.12127
Mango, N., Makate, C., Tamene, L., Mponela, P., and Ndengu, G. (2017). Awareness and adoption of land, soil and water conservation practices in the Chinyanja Triangle, Southern Africa. Int. Soil Water Conserv. Res. 5, 122–129. doi: 10.1016/j.iswcr.2017.04.003
Manyong, V., Bokanga, M., Nyamuhirwa, D. M. A., Bamba, Z., Adeoti, R., Mwepu, G., et al. (2022). COVID-19 outbreak and rural household food security in the Western Democratic Republic of the Congo. World Dev. Perspect. 28:100469. doi: 10.1016/j.wdp.2022.100469
Moughan, P. J. (2021). Population protein intakes and food sustainability indices: the metrics matter. Glob. Food Secur. 29:100548. doi: 10.1016/j.gfs.2021.100548
Myers, H. M., Tomberlin, J. K., Lambert, B. D., and Kattes, D. (2014). Development of black soldier fly (Diptera: Stratiomyidae) larvae fed dairy manure. Envir. Entomol. 37, 11–15. doi: 10.1093/ee/37.1.11
Nguru, W. M., Gachene, C. K., Onyango, C. M., and Girvetz, E. H. (2021). Factors constraining the adoption of soil organic carbon enhancing technologies among small-scale farmers in Ethiopia. Heliyon 7:e08497. doi: 10.1016/j.heliyon.2021.e08497
Nyamuhirwa, D. M. A., Awotide, B. A., Kusinza, D. B., Bishikwabo, V. K., Mignouna, J., Bamba, Z., et al. (2022). A comparative analysis of technical efficiency and profitability of agribusiness and non-agribusiness enterprises in Eastern DRC. Sustainability 14:8384. doi: 10.3390/su,14148384
Olutegbe, N. S., and Ojuoluwa, O. (2022). Overcoming social barrier to adoption of black soldier fly (Hermetia illucens) as a protein source for poultry: how tall is the order? Chem. Proc. 10:73. doi: 10.3390/IOCAG2022-12321
Ouko, K. O., Mukhebi, A. W., Obiero, K. O., and Opondo, F. A. (2022). Using technology acceptance model to understand fish farmers' intention to use black soldier fly larvae meal in Nile tilapia production in Kenya. All Life 15, 884–900. doi: 10.1080/26895293.2022.2112765
Raman, S. S., Stringer, L. C., Bruce, N. C., and Chong, C. S. (2022). Opportunities, challenges, and solutions for black soldier fly larvae-based animal feed production. J. Clean. Prod. 373:133802. doi: 10.1016/j.jclepro.2022.133802
Ravallion, M. (2001). The mystery of the vanishing benefits: an introduction to impact evaluation. World Bank Econ. Rev. 15, 115–140. doi: 10.1093/wber/15.1.115
Rodenburg, J., Büchi, L., and Haggar, J. (2021). Adoption by adaptation: moving from conservation agriculture to conservation practices. Int. J. Agric. Sustain. 19, 437–455. doi: 10.1080/14735903.2020.1785734
Romano, N., Datta, S. N., Pande, G. S. J., Sinha, A. K., Yamamoto, F. Y., Beck, B. H., et al. (2023). Dietary inclusions of black soldier fly (Hermetia illucens) larvae frass enhanced production of channel catfish (Ictalurus punctatus) juveniles, stevia (Stevia rebaudiana, and lavender (Lavaridula angustifolia) in an aquaponic system. Aquac. 575:739742. doi: 10.1016/j.aquaculture.2023.739742
Schönfeldt, H. C., and Hall, N. G. (2012). Dietary protein quality and malnutrition in Africa. Br. J. Nutr. 108, S69–S76. doi: 10.1017/S0007114512002553
Scieuzo, C., Franco, A., Salvia, R., Triunfo, M., Addeo, N. F., Vozzo, S., et al. (2023). Enhancement of fruit byproducts through bioconversion by Hermetia illucens (Diptera: Stratiomyidae). Insect Sci. 30, 991–1010. doi: 10.1111/1744-7917.13155
Siddiqui, S. A., Ristow, B., Rahayu, T., Putra, N. S., Yuwono, N. W., Mategeko, B., et al. (2022). Black soldier fly larvae (BSFL) and their affinity for organic waste processing. Waste Manag. 140, 1–13. doi: 10.1016/j.wasman.2021.12.044
Simtowe, F., Kassie, M., Diagne, A., Asfaw, S., Shiferaw, B., Silim, S., et al. (2011). Determinants of agricultural technology adoption: the case of improved pigeonpea varieties in Tanzania. Q. J. Int. Agric. 50, 325–345.
Suloma, A., El-Husseiny, O. M., Hassane, M. I., Mabroke, R. S., and El-Haroun, E. R. (2014). Complementary responses between hydrolyzed feather meal, fish meal, and soybean meal without amino acid supplementation in Nile tilapia Oreochromis niloticus diets. Aquac. Int. 22, 1377–1390. doi: 10.1007/s10499-014-9753-y
Surendra, K. C., Tomberlin, J. K., van Huis, A., Cammack, J. A., Heckmann, L. H. L., and Khanal, S. K. (2020). Rethinking organic wastes bioconversion: evaluating the potential of the black soldier fly (BSF) [Hermetia illucens (L.)](Diptera: Stratiomyidae). Waste Manag. 117, 58–80. doi: 10.1016/j.wasman.2020.07.050
Suri, T., and Udry, C. (2022). Agricultural technology in Africa. J. Econ. Perspect. 36, 33–56. doi: 10.1257/jep.36.1.33
Tambo, J. A., and Abdoulaye, T. (2012). Climate change and agricultural technology adoption: the case of drought tolerant maize in rural Nigeria. Mitig. Adapt. Strateg. Glob. Chang. 17, 277–292. doi: 10.1007/s11027-011-9325-7
Tomberlin, J. K., Adler, P. H., and Myers, H. M. (2009). Development of the black soldier fly (Diptera: Stratiomyidae) in relation to temperature. Envir. Entomol. 38, 930–934. doi: 10.1603/022.038.0347
Weththasinghe, P., Hansen, J. Ø., Mydland, L. T., and Øverland, M. (2022). A systematic meta-analysis-based review on black soldier fly (Hermetia illucens) as a novel protein source for salmonids. Rev. Aquac. 14, 938–956. doi: 10.1111/raq.12635
Keywords: environment-friendly technology, black-soldier fly larvae, livestock farming, animal nutrition, treatment effect framework, adoption
Citation: Nyamuhirwa D-M, Feleke S, Dontsop Nguezet P-M, Sissoko D, Moussa B, Kouakou A-G, Zakari S, Abdoulaye T and Manyong V (2025) Perspectives on the adoption of black-soldier fly larvae for animal feed among livestock farmers in Sub-Saharan Africa. Front. Environ. Econ. 4:1519767. doi: 10.3389/frevc.2025.1519767
Received: 30 October 2024; Accepted: 13 January 2025;
Published: 06 February 2025.
Edited by:
Shobha Poudel, Fondazione Eni Enrico Mattei, ItalyReviewed by:
Georgiana Raluca Ladaru, Bucharest Academy of Economic Studies, RomaniaCopyright © 2025 Nyamuhirwa, Feleke, Dontsop Nguezet, Sissoko, Moussa, Kouakou, Zakari, Abdoulaye and Manyong. This is an open-access article distributed under the terms of the Creative Commons Attribution License (CC BY). The use, distribution or reproduction in other forums is permitted, provided the original author(s) and the copyright owner(s) are credited and that the original publication in this journal is cited, in accordance with accepted academic practice. No use, distribution or reproduction is permitted which does not comply with these terms.
*Correspondence: Dieu-Merci Akonkwa Nyamuhirwa, ZGlldS1tZXJjaS5ha29ua3dhLm55YW11aGlyd2FAYWcudW5pLWdpZXNzZW4uZGU=
Disclaimer: All claims expressed in this article are solely those of the authors and do not necessarily represent those of their affiliated organizations, or those of the publisher, the editors and the reviewers. Any product that may be evaluated in this article or claim that may be made by its manufacturer is not guaranteed or endorsed by the publisher.
Research integrity at Frontiers
Learn more about the work of our research integrity team to safeguard the quality of each article we publish.