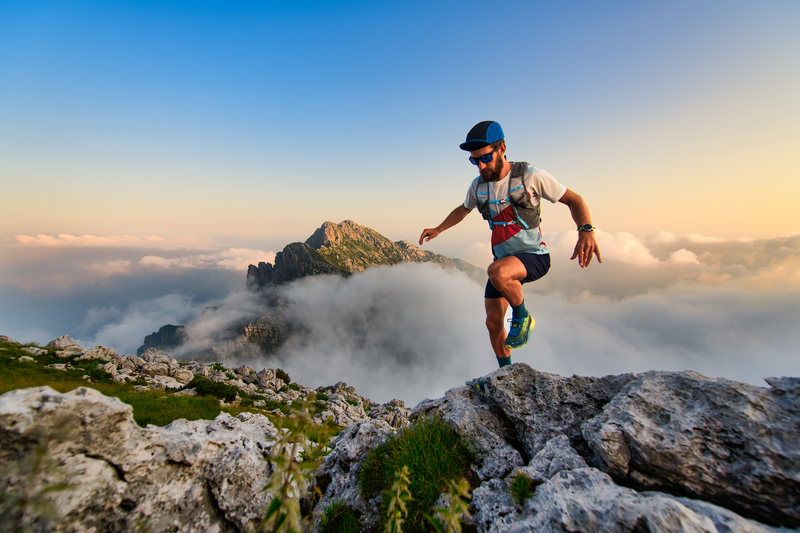
95% of researchers rate our articles as excellent or good
Learn more about the work of our research integrity team to safeguard the quality of each article we publish.
Find out more
ORIGINAL RESEARCH article
Front. Environ. Econ. , 10 December 2024
Sec. Energy Economics
Volume 3 - 2024 | https://doi.org/10.3389/frevc.2024.1511080
Digital economy, as an innovative digital technology, can promote environmental governance and support low-carbon development. Under the backdrop of China's dual carbon strategy, the country faces significant challenges in achieving coordinated development across the domains of economy, environment, and energy. Based on panel data from 30 Chinese provinces between 2012 and 2022, this paper constructs a coupling coordination model to measure the level of integrated development among digital economy, environmental regulation, and carbon emission intensity. The study also examines the spatiotemporal evolution of this coordination using kernel density estimation and spatial correlation tests. Furthermore, empirical analysis via regression models was employed to investigate key influencing factors. The findings suggest that: (1) The coupling coordination level has shown an upward trend over the sample period, with no extreme regional disparities; (2) Spatially, the development of coupling coordination exhibits “high-high” and “low-low” clustering effects; (3) Development levels are influenced by various external driving factors, with significant regional differences in primary influencing factors. For these findings, the following recommendations are proposed: enhance digital infrastructure, optimize regulatory frameworks, and promote the integration of digital economy with green and low-carbon development.
The rapid development and widespread application of information technology have accelerated the growth of emerging industries, such as the service sector and high-tech industries, effectively promoting the structural optimization and upgrading of traditional industries. Digital economy represents a new trend in technological revolutions and industrial transformations, as well as an innovative approach to achieving high-quality economic growth and ecological civilization. Digital economy technologies invigorate enterprises' capacity for green innovation, allowing them to tackle issues related to energy emissions and pollution (Yuan et al., 2021; Li et al., 2024). Among these challenges, climate change risks have posed severe global difficulties in terms of environmental governance. Greenhouse gas emissions, primarily carbon dioxide (CO2), are the main contributors to global climate problems. Therefore, strengthening environmental regulation, promoting energy conservation, reducing carbon emissions, and enhancing energy efficiency are critical tasks for achieving green, low-carbon development and mitigating climate change (Tao et al., 2023; Barratt et al., 2022). On December 12, 2015, the 21st United Nations Climate Change Conference culminated in the signing of the Paris Agreement by 178 member states, establishing a unified global action plan to address climate change during the industrial era. During the third industrial revolution, China experienced rapid economic growth, which significantly improved the living standards of its citizens (Dong et al., 2017). However, the early stages of this development were largely driven by resource-intensive, high-consumption, and high-pollution practices, which resulted in substantial environmental degradation and resource waste (Chen et al., 2020). The conflict between industrial economic development and environmental governance has become increasingly apparent, prompting the Chinese government in 2020 to establish the dual carbon goals of achieving peak carbon emissions by 2030 and carbon neutrality by 2060. According to World Bank data, China, as the world's largest emitter of carbon, generated 10,944.686 million tons of carbon emissions in 2020, accounting for ~32.61% of global emissions. The establishment of the dual carbon strategy represents a significant commitment to the sustainable development of the global ecological environment. It demonstrates China's leadership in supporting green technological innovation and optimizing production structures. Consequently, research on the coupling and coordinated development of digital economy proliferation, appropriate environmental regulation, and carbon emission intensity are of strategic importance for government regulation and corporate governance (Tang and Qin, 2022; Zhang et al., 2022).
The theory of digital economy was first introduced by Don Tapscott in the 1980's, identifying digital information as a key element and the internet as its carrier. This form of economic activity effectively harnesses information and communication technologies (ICT) to drive efficiency and optimize economic structures (Tapscott, 1996; OECD, 2014). In recent years, digital economy has gained widespread application through advancements in artificial intelligence (AI), cloud computing, big data algorithms, and 5G technologies. Countries around the world have gradually recognized and prioritized the power of digital economy, incorporating it as a key strategic direction for national development (Lee et al., 2021). Beyond fostering a range of emerging industries, digital economy also promotes technological innovation within enterprises by optimizing resource allocation, reducing costs, and enhancing labor productivity. These developments have significantly accelerated the transformation and upgrading of traditional industries. Since the G20 Summit in 2016, the Chinese government has accelerated the digitization of industries. The 2019 Report on the Progress of Digital China indicates that China's digital economy has shown rapid growth, contributing 35.8 trillion yuan in added value, with a contribution rate of 67.7% to GDP growth (Liu et al., 2022). Additionally, digital technology, as a knowledge-intensive and “clean” production factor, is widely applied in regional industries and corporate operations (Eichhorst et al., 2017). These technologies foster green technological innovation within enterprises, improve energy efficiency, and promote the widespread use of clean energy while enhancing coal usage efficiency.
Since the onset of the low-carbon economy, scholars have increasingly focused on the issue of balancing economic growth with surging carbon emissions. Reducing carbon emissions while promoting economic growth is a critical and pressing challenge (Soytas and Sari, 2009). Although there is no universally accepted definition of carbon emission intensity in existing literature, most scholars regard it as a measure of carbon energy efficiency, typically calculated as the amount of carbon dioxide emissions relative to economic output. At the same time, carbon intensity is closely linked to economics, human geography, energy and related institutions, and economic complexity and fossil energy consumption are identified as necessary conditions for high carbon intensity under the NCA model (Kazemzadeh et al., 2023). Under China's new vision for green and low-carbon development, improving energy efficiency plays a crucial role in achieving energy conservation, emission reduction, and addressing climate change. Many scholars believe that digital economy technologies can enhance the development of industrial e-commerce and internet operations, which helps to phase out carbon-intensive industries, thereby optimizing urban structures and improving environmental conditions. Research into the relationship between digital economy and carbon emission intensity has deepened, primarily focusing on the following aspects: (1) Empirical studies by Dong et al. analyzed the impact of digital economy on global carbon emissions, revealing that digital economy can effectively reduce carbon intensity (Hou et al., 2024); (2) In studies on digital economy and energy efficiency at the city level in China, digital technologies were found to promote green technological innovation, which, in turn, improves energy efficiency. As a threshold variable, green energy efficiency affects the relationship between digital economy and carbon emissions. When green energy efficiency is low, the digital economy promotes carbon emissions, and when green energy efficiency is high, the digital economy reduces carbon emissions (Yu et al., 2022); (3) Measurement analysis has primarily focused on the coupling coordination of digital economy and green, low-carbon development, as well as the spatiotemporal evolution and obstacles to this coordination. Research has found that the primary factors hindering the coupling coordination, ranked from most to least significant, are digital industrialization, green ecology, industrial digitization, green production, and green living. These factors also differ across time and regions. Based on these findings, scholars have proposed policy recommendations to strengthen support for digital economy, solidify its foundation, and implement tailored development strategies in line with local conditions (Jin and Liu, 2024).
However, in the process of promoting industrial digital transformation and the low-carbon green development of enterprises, government-enforced environmental regulations provide essential oversight and safeguards. Environmental regulation is an effective policy tool that balances the interests of the government, enterprises, and the public while addressing the internalization of environmental externalities. By formulating and implementing policies and regulations related to environmental protection, a robust environmental governance system can be established, leading to effective monitoring mechanisms and achieving a win-win scenario for both economic and sustainable development (Lian et al., 2024). Most scholars agree that government-imposed environmental regulations can strictly oversee corporate pollution and carbon emissions, prompting enterprises to engage in technological innovation, reduce pollution control costs, and enhance energy efficiency, thus improving their productivity and competitiveness (Blackman et al., 2018; Hao et al., 2018; Ulucak et al., 2020). How to exercise the role of environmental regulation has also gradually become the focus of scholars. Silva et al. (2024) conducted a fuzzy-set qualitative comparative analysis of 156 countries from a global perspective and found that economic development and the level of governmental governance affect environmental performance, which provides an important reference value for governments to carry out effective environmental regulation.
In fact, the “3E” issue—Economy, Environment, and Energy—has long attracted the attention and research of many scholars. Lenzen and Dey (2002) was the first to establish the “3E” system evaluation framework, providing standards and criteria for assessing the effectiveness of the system's operations. Lazzaretto and Toffolo (2004) further explained this complex system from a systems dynamics perspective, using system dynamics methods to explore how changes in one subsystem affect the entire system and how optimization can be achieved. Today, digital economy is not only a new economic model but also an innovative digital technology. In the realm of environmental pollution, governance and control primarily fall under governmental oversight, while energy efficiency is closely linked to carbon emissions (Duan and Sun, 2024).
With the rapid advancement of information technology and the resulting innovations in economic systems, research on the 3E system has gradually expanded into more detailed fields such as digital economy, green finance, energy efficiency, carbon emission intensity, environmental governance, and ecological protection. At the same time, the introduction of various initiatives and government reports has heightened the focus on governmental decision-making and regulatory methods. Consequently, the relationship between digital economy, environmental regulation, and carbon emission intensity has become increasingly intertwined, and scientific research into this relationship holds significant implications for industrial development. Although many scholars have conducted empirical analyses exploring the interactions among these three elements, research on the measurement of their coordinated development is relatively scarce, or such research often focuses only on two of the three elements. The study of the coordinated development among digital economy, environmental regulation, and carbon emission intensity is of considerable value for promoting balanced development and informing decision-making on climate change issues (Wang H. et al., 2024; Wang S. et al., 2024). Therefore, this paper utilizes panel data from 30 Chinese provinces and applies the entropy weight method to calculate the development levels of digital economy, environmental regulation, and carbon emission intensity from 2012 to 2022. Furthermore, a coupling coordination model is constructed to investigate the development trends of coordination among these systems during the sample period. Additionally, a spatial autocorrelation model is employed to empirically analyze the spatial correlation effects of the coupling coordination among digital economy, environmental regulation, and carbon emission intensity in China. Lastly, regression models are used to examine the external factors influencing the development of this coupling coordination.
Digital economy has become a key driver of global industrial restructuring, and its influence on carbon emission intensity has been the subject of extensive research and debate. Due to its highly penetrative and interconnected nature, digital economy promotes green technological innovation, which enhances the economic efficiency of enterprises' carbon emissions (Long, 2024). First, it improves internal green governance within companies. Digital economy tightly integrates information technology with corporate production processes, promoting a flatter organizational governance structure and accelerating the sharing of low-carbon information across different departments (Wang and Shao, 2023; Xu et al., 2022) The development of digital economy is inherently tied to the innovation and application of technology, and these innovations help boost total factor productivity, thereby improving carbon emission efficiency and reducing carbon emission intensity. Second, it provides a secure environment for carbon trading. As China's carbon trading market continues to expand, digital monitoring platforms and blockchain technology offer a secure and transparent medium for recording carbon emissions, which helps control emissions and transactions, thus further promoting low-carbon development (Wang et al., 2018). Third, it strengthens external regulatory oversight. As digital economy empowers traditional industries, it also drives advancements in digital technology. The application of digital technology in environmental protection has transformed traditional environmental monitoring methods, reducing the cost of monitoring technology and enhancing real-time environmental assessment capabilities (Song et al., 2022). Finally, in the era of e-commerce, digital economy provides various collaborative governance tools for governments, offering convenient channels for information sharing, reducing information asymmetry, and lowering search costs. This saves resources previously consumed by individual entities in their search for information. Based on the perspectives of 60 countries around the world, Dong et al. (2022) empirically examined the impact of the digital economy on carbon emission intensity and the related transmission mechanisms and found that the development of the digital economy significantly reduces carbon emission intensity but promotes an increase in per capita carbon emissions. This suggests that the effectiveness of the digital economy in promoting low-carbon development is limited and that there are significant differences in the level and impact of the digital economy between “hyper-digitalized countries” and “under-connected countries” (Dong et al., 2022).
Environmental regulation refers to government-led policies and measures aimed at controlling pollutant emissions and improving the efficiency of energy resource utilization (Zhang et al., 2020). Current theoretical research on the relationship between environmental regulation and carbon emission intensity is limited, with most scholars referencing the Porter Hypothesis (Porter, 1991). This hypothesis posits that environmental regulation increases monitoring of corporate carbon emissions, raising costs for companies and thereby pressuring them to innovate technologically. This, in turn, enhances economic efficiency while maintaining established output levels. Furthermore, by adopting mandatory environmental governance standards and efficiency-enhancing measures, local environmental management systems increase the entry barriers for enterprises, eliminating technologically outdated industrial companies and improving the industrial sector's carbon emission capacity (Porter and van der Linde, 1995). Well-designed environmental policies can create an “innovation compensation effect,” which not only enhances a company's environmental performance but also boosts its overall capacity for innovation. However, environmental regulation varies in its type, method, and intensity across different periods. In regions where environmental oversight still needs to be improved, the adoption of stringent environmental regulations may lead to a reduction in investments in productive research and development. Increased use of new energy sources and economic growth can reduce outdoor air pollution and thus protect public health and safety (Shahbaz et al., 2022). Environmental and energy policies can be influenced by the degree of normative implementation and the experience of the subjects, and there is inter-economy differentiation in the rule schemes applied in different countries. In particular, Galeotti et al. (2020) measure and analyze the effectiveness of environmental policies and energy innovations in 19 OECD countries (all of them developed countries), showing that nationally appropriate environmental policies and rational means of implementation are the key to achieving the objectives, but that it is important to take into account the relationship between environmental and energy policies and economic development, competitiveness and wellbeing, and to continue to learn from the experience of coordinated development (Kazemzadeh et al., 2022).
In the realm of digital economy, environmental regulation has a significant impact on business operations, particularly in areas such as production costs, supply chain management, and market entry. Appropriate environmental regulations can encourage companies to increase their investment in research and development, fostering technological innovation and promoting the shift toward green transformation and sustainable development (Galeotti et al., 2020). Additionally, the development of digital economy infrastructure provides the government with more efficient and convenient information service platforms (Shao and Razzaq, 2022). Through big data governance and “Internet + government services” initiatives, local governments can further extend the scope and depth of their governance. Moreover, digital technologies not only enhance government transparency but also enable the sharing of data on corporate carbon emissions (He and Liu, 2019). This reduces information asymmetry, helping enterprises better understand policy directions and make timely adjustments. Simultaneously, digital technology increases the accuracy of government monitoring of corporate activities, improving local governance efficiency, optimizing resource utilization, and reducing pollutant emissions. Empirical evidence from 42 OBRI countries found that digital finance can effectively promote technological innovation and economic growth, but it can contribute to higher carbon emissions and affect environmental protection, so it is still necessary to further implement the relevant environmental policy constraints to adjust the relationship between the two (Ozturk and Ullah, 2022).
In practice, governments and enterprises are faced with uncertainties such as pollution levels, energy prices, and the economic environment. Adequate consideration of economic development, technological innovation, ecological protection, and low-carbon development is a difficult and necessary problem. Digital economy-enabled front-end technology generation can be used to solve the problem of continuous ecological decline. However, the popularization of digital technology to improve production efficiency may prompt the investment of more energy to enhance economic benefits. At the same time, the innovation of green technologies and the uncertainty of traditional energy prices are conducive to the large-scale investment of new energy, thereby reducing carbon emissions. Environmental policies have means to constrain the supervision of corporate carbon emissions, conserve energy, and reduce pollution. As pollution levels continue to rise, the role of environmental regulations will significantly weaken (Dao et al., 2024). Various studies indicate that there is a complex relationship between the digital economy, environmental regulations, and carbon emission intensity. Some existing literature has issues with incomplete measurements or outdated calculation times, so this article will further explore the coordinated development of the three.
Digital economy, following the agricultural and industrial economies, represents a primary economic form in which data resources serve as key elements, modern information networks function as the main carriers, and the integration and application of information and communication technologies (ICT) drive the digital transformation of all factors (Ge et al., 2022). This new economic paradigm seeks to balance equity and efficiency more effectively. Following the principles of data availability, scientific validity, and consistency (Wang J. et al., 2021; Ren et al., 2021), this study constructs an indicator system consisting of three primary indicators—digital infrastructure, digital industrialization, and industrial digitization—along with 11 secondary indicators as shown in Table 1.
Most literature concerning environmental regulation uses indicators such as the number of environmental protection personnel, investment in pollution control research and development, pollution tax rates, or pollution control costs to measure governmental environmental governance. However, these indicators assess only specific aspects of environmental governance and need to fully capture the government's regulatory measures in a comprehensive manner. Therefore, this study adopts the approach of Chen et al. (2016), Chen and Chen (2018), and Deng and Yang (2019), selecting 15 environment-related keywords from provincial government work reports as shown in Table 2. By calculating the total frequency of these terms within the texts and applying a logarithmic transformation after adding 1, this study measures the extent of governmental environmental governance.
This paper follows the carbon emission model provided by IPCC Reports (Intergovernmental Panel on Climate Change) and Zhang and Li (2022) to estimate the carbon emissions for each province. The CO2 emissions are determined by calculating the consumption of eight energy sources: coal, coke, crude oil, gasoline, kerosene, diesel, fuel oil, and natural gas. The carbon dioxide emission factors for each energy type are as follows.1 The formula for carbon emission intensity (CARBE) is:
The ratio of carbon dioxide emissions to regional GDP measures the carbon emission intensity for each province. Therefore, among the research objects selected in this paper, environmental regulation and carbon emission intensity can be obtained by simple direct measurement or arithmetic formula. In contrast, digital economy measurement needs to fully consider the influence of factors in the indicator framework. For the system of individual measurement indicators (environmental regulation, carbon intensity emissions) to take the dimensionless processing to derive the score, the digital economy system uses the entropy weight method to assign each indicator to measure the overall score.
This study utilizes panel data from 30 Chinese provinces between 2012 and 2022 (Tibet is excluded due to severe data deficiencies). The primary data sources include the official website of the National Bureau of Statistics of China, as well as annual editions of the China Statistical Yearbook, China Information Yearbook, China Information Industry Yearbook, Peking University Digital Financial Inclusion Index, China Environmental Statistics Yearbook, and local government work reports.
Entropy weighting method is an objective weighting method that determines the weight of indicators based on the amount of information contained in each indicator. In the multi-attribute evaluation framework, there are differences in the impact of different indicators on the overall evaluation score, so it is necessary to set weights for each indicator. The core idea of entropy weight method is to measure the information content of each indicator based on the size of information entropy, and determine the importance of the indicator accordingly. The smaller the entropy value, the greater the amount of information and the higher the weight should be. This method can effectively avoid the interference of subjective factors, making the determination of weights more scientific and objective. The comprehensive score of the digital economy can be obtained through the following calculation steps (Shannon, 1948).
Due to the differences in dimensions and magnitudes of various indicators in the digital economy system, the original data needs to be dimensionless first, and the weights of the indicators and the comprehensive scores of each system need to be further calculated. Environmental regulations and carbon intensity emissions can be directly calculated through positive and negative indicators to obtain standardized scoring results.
Standardization of indicators refers to standardizing the dimensions and units of different indicators into a unified unit of measurement. The calculation formula is as follows:
Positive indicators:
Negative indicators:
Calculation of the number of j The weight of the first sample value under the indicator i of the sum of all sample values for that indicator Yij:
The information entropy of the first j Information entropy of the indicator ej:
Information Entropy Redundancy dj:
Evaluation indicator weights wj:
Composite Score Ui:
Finally, Ui represents the comprehensive evaluation index for each system, while wj is the weight of the j indicator. denotes the standardized value of each indicator.
Coupling originates from the concept of physics, which refers to the phenomenon that two or more systems or forms of motion are interconnected, promoted and constrained by various interconnections. From the perspective of synergetics, the coupling effect and the degree of coupling determine the tendency of a system to move from disorder to order when it reaches a critical region, indicating the relationship between multiple systems that interact and influence each other. The degree of coordination emphasizes the harmonious synchronization between systems in the process of economic development and whether they show benign interaction. When systems are in a state of coordination, they can promote each other, develop together and maximize the overall benefits. The degree of coupling coordination is an important index for assessing the synergistic effect and interaction between systems, which can judge the degree of interdependence and coupling between systems (Lu et al., 2008; Wang S. et al., 2021). By constructing a specific indicator system and calculation method, the coupling coordination model quantitatively evaluates the degree of coupling and coordination between systems, and judges the overall development level between multiple systems.
This study constructs both a three-system coupling coordination model—consisting of digital economy, environmental regulation, and carbon emission intensity—and three two-system coupling coordination model that analyzes pairs of systems. The calculation formula is as follows:
Where Ci represents the coupling degree between systems for each sample, with a value range between [0,1], reflecting the degree of interaction among the systems as shown in Table 3. Ui1, Ui2, Ui3... denote the comprehensive development levels of each subsystem. However, the coupling degree alone does not fully capture the level of coordinated development, necessitating the introduction of the coupling coordination degree model for further analysis:
T represents the comprehensive evaluation index of the whole system, which reflects the level of system coordination role. D For the coupling coordination degree, the D value range is [0,1], and this paper refers to the division standard of coordination level defined by Liao (1999) to divide the model calculation results.
Exploratory Spatial Data Analysis (ESDA) is used to explain the spatial structure, spatial patterns, and spatial trends inherent in data, including both global spatial autocorrelation analysis and local spatial autocorrelation analysis (Anselin, 1995; Gao and Li, 2020; Yang and Cheng, 2024). This paper employs Moran's I index to measure the spatial distribution of the coupling coordination degree among digital economy, environmental regulation, and carbon emission intensity.
Moran's I values range from−1 to 1. A value >0 indicates that the coupling coordination degree among the three systems across provinces exhibits positive spatial autocorrelation, meaning that higher clustering is observed as the value approaches 1. A value of 0 indicates no spatial clustering, while a value < 0 indicates negative spatial autocorrelation, suggesting a dispersion pattern.
It was validated using the Z-test:
While the global Moran's index can indicate whether spatial correlation exists in the sample region, specific landmarks of the spatial aggregation phenomenon need to be tested using the local Moran's index, which is formulated as follows:
Among them:
Moran's IndexIi For:
Ii> 0 indicates a positive spatial correlation of system coupling coordination, and Ii < 0 indicates a negative correlation. Both exhibit spatial aggregation.
Based on Equations 2–8, this section evaluates the scores of the three subsystems—digital economy, environmental regulation, and carbon emission intensity—and calculates the comprehensive index for the entire system. The results are shown in the Table 4.
Table 4. Development levels of the digital economy, environmental regulation, and carbon emission intensity, and comprehensive index by region.
Digital economy reflects the degree of digital industrial development in a region. The eastern region of China exhibits significantly higher levels of digitalization compared to other areas, with the western region showing the lowest level of digital economy development. The top five regions in terms of digital economy development are Shanghai, Beijing, Jiangsu, Guangdong, and Zhejiang. Environmental regulation scores represent the effectiveness of regional governments' environmental governance. The scores for environmental regulation are relatively similar across the eastern, central, and western regions, with the northeastern region lagging behind. However, the overall level of environmental regulation in China is generally strong. Carbon emission intensity reflects energy economic efficiency to a certain extent. The regions with higher energy efficiency are primarily located in the central and eastern areas, though the differences between the western and northeastern regions are not pronounced. The range of comprehensive index values falls between [0.281, 0.684], with Ningxia having the lowest comprehensive index and Guangdong the highest.
The coupling coordination degree for both the two-system and three-system models was calculated using the coupling coordination degree model. Table 5 presents the average coupling coordination degree for each region from 2012 to 2022.
Table 5. Coupling coordination degrees between the digital economy, environmental regulation, and carbon emission intensity across regions.
Table 5 illustrates the coupling coordination degree for the following combinations: digital economy-environmental regulation, digital economy-carbon emission intensity, and environmental regulation-carbon emission intensity, as well as the three-system coupling coordination degree. Most of the coupling coordination values for the systems exceed 0.5. In the two-system model, the coupling coordination between environmental regulation and carbon emission intensity is significantly higher than that between digital economy-environmental regulation and digital economy-carbon emission intensity, indicating a stronger interaction between government environmental governance and carbon consumption efficiency. Looking at the results of the three-system coupling coordination degree, the top five regions are Beijing, Shanghai, Guangdong, Jiangsu, and Zhejiang, which correspond to the regions with the highest digital economy scores. This suggests that the level of digital economy development may be a key factor influencing coupling coordination.
Figure 1 illustrates the kernel density estimation results of the coupling coordination levels among digital economy, environmental regulation, and carbon emission intensity for 30 provinces from 2012 to 2022. The MATLAB model results reveal that the kernel density results by region are similar to the national results, prompting this paper to conduct an overall national analysis (Rosenblatt, 1956; Parzen, 1962). The central point of the coupling coordination level of the three systems shows a gradual shift to the right, with a relatively stable peak from 2012 to 2020, and a slow rise in the peak from 2020 to 2022. This indicates that the coupling coordination level has been steadily improving, with an increasingly concentrated distribution. Additionally, the number of peaks in the kernel density curve remained unchanged, suggesting no extreme differences between regions during the study period.
Figure 2 demonstrates the temporal evolution of the coupling coordination degree among digital economy, environmental regulation, and carbon emission intensity for 30 provinces across China's four major regions from 2012 to 2022. The overall trend shows a gradual increase, with the national average coupling coordination degree rising from 0.568 in 2012 to 0.659 in 2022. This suggests that the coupling between digital economy, environmental regulation, and carbon emission intensity has been continuously strengthening. In particular, the eastern regions, such as Beijing, Tianjin, Jiangsu, and Shanghai, consistently show coupling coordination levels above the national average. These areas have a higher degree of industrial digitization and carbon efficiency compared to other regions, and their governments place a strong emphasis on environmental governance, putting them at the forefront of coordinated development. On the other hand, regions such as Hebei, Inner Mongolia, Guizhou, Qinghai, and Heilongjiang exhibit lower coupling coordination levels than the national average. These regions may be limited by digital infrastructure, with a low degree of digital transformation in industrial structure, and relatively low energy utilization efficiency. Provinces with lower coupling coordination levels require further improvements in industrial digitization, environmental governance, and energy efficiency.
Figure 2. Temporal changes in the development levels of coupling coordination degrees across regions.
To further investigate the spatiotemporal evolution characteristics of the coupling coordination development between digital economy, environmental regulation, and carbon emission intensity, this study utilizes ArcGIS to map the spatial distribution of the coupling coordination degree across Chinese provinces for the years 2012, 2017, and 2022, as shown in Figure 3.
Figure 3. Spatial changes in the development levels of coupling coordination degrees across regions.
Overall, the coupling coordination degree of the three systems in most regions falls within the “barely coordinated” range or higher, with Guangdong and Shanghai reaching the “well-coordinated” level by 2022. Over time, most provinces and cities have seen improvements in the coupling coordination development among digital economy, environmental regulation, and carbon emission intensity. This suggests that from 2012 to 2022, industries in China gradually achieved digital integration, society placed increasing importance on environmental protection, and energy-consuming sectors enhanced their economic energy efficiency through technological innovation and energy-saving measures. In terms of spatial distribution, the eastern coastal regions exhibit significantly higher development levels than other regions, while western regions such as Sichuan and Chongqing also demonstrate notable improvements. These areas have benefited from high-quality economic development, driving industrial transformation, upgrading, and environmental monitoring and governance. However, by 2022, the northwestern regions and Hainan Province show relatively low levels of coupling coordination development, with Ningxia exhibiting the lowest level, approaching a state of imbalance. These regions are not only constrained by their economic environment but also lag behind in carbon reduction technologies within their energy consumption sectors.
As shown in Table 6, the global Moran's I-values for the coupling coordination degree among digital economy, environmental regulation, and carbon emission intensity range between [0.192, 0.312] during the sample period. This indicates a relatively stable positive spatial correlation in the coupling coordination development across space. The test Z-value is >1.65, indicating that the coupling coordination degree of the sample area shows spatial clustering. The p-values for the estimated results are all significant at the 5% level, validating the appropriateness of the spatial correlation test model.
To further explore the local spatial characteristics of the coupling coordination degree development among digital economy, environmental regulation, and carbon emission intensity in various regions from 2012 to 2022, the local Moran's I-values for the years 2012, 2017, and 2022 were calculated as shown in Figure 4.2
Throughout the sample period, most regions fall into “low-low” and “high-high” clusters, indicating an evident spatial clustering effect for the coupling coordination degree of China's digital economy, environmental regulation, and carbon emission intensity. The “high-high” clusters are primarily concentrated in the eastern and central regions (Beijing, Tianjin, Jiangsu, Zhejiang, Anhui, Fujian, etc.), while the “low-low” clusters are mostly located in the western and northeastern regions (Yunnan, Gansu, Ningxia, Heilongjiang, Jilin, etc.). In particular, the clustering effects in Ningxia and Gansu are relatively weak, which may be due to lower levels of economic development and industrial digitization, as well as relatively poor energy efficiency in these regions. Because of the loss of data in Tibet, there may be some errors in the overall spatial clustering effect.
From the perspective of integration depth, there are significant differences between regions. The integration of digital economy, environmental regulation, and carbon emission intensity in the northwest and northeast regions still needs to be balanced, and digital infrastructure needs to be more sufficient. The lack of key digital technology reserves limits the integration of digital economy and green low-carbon development. Weak economic foundations and digital innovation technology make it difficult to promote local green transformation and carry out more effective green special governance measures. In addition, in terms of industrial structure, these regions are richer in natural resources such as ores, oil and metals. China's traditional industries are mainly concentrated in these regions, with a single industrial structure, small-scale enterprises, and a lack of capital and technological capacity to promote digital technological innovation. Traditional industries are characterized by high energy consumption and pollution, which hinders the local government's efforts to strengthen environmental protection and implement green policies, and makes ecological governance inefficient. In order to comply with high-quality economic development and the implementation of the Environmental Law, local enterprises should optimize, adjust, transform, and upgrade their production structure and quality in parallel under government administrative orders and mandatory measures, increase investment in green research and development, and vigorously develop green industries. Give full play to the decisions of the government and market on the allocation of social resources, and at the same time, improve the production efficiency of production enterprises, that is, enhance the efficiency of resource utilization.
The main reason for the good coupling and coordination in the eastern region is that the digital economy started earlier and has a larger stock. Since the reform and opening up, the eastern region has developed rapidly and has the financial strength to invest in digital economy empowerment of resource-based industries and improve the level of green technology innovation. Ultimately, it presents a significant advantage of digital economy empowering the green transformation of resource-based industries. Sichuan Province and Chongqing Municipality, which have been the focus of China's Western development in recent years, have received funding for a series of infrastructure projects, and the digital drive has led to a quantum leap in the local economies. The two cities have developed cultural and tourism resources in accordance with local conditions, vigorously developed the service industry, and achieved remarkable success in combating natural ecological pollution. They have shifted from the traditional “all-encompassing” governmental governance to the community governance of the whole society, which is based on “joint construction and sharing,” so as to realize the transformation of ecological and environmental governance from “effective” to “long-lasting,” thus contributing to the green development and comprehensive revitalization of the two places.
The coupling coordination development among digital economy, environmental regulation, and carbon emission intensity is a highly complex mechanism, influenced not only by the internal interactions of the three systems but also by a series of external driving factors. Based on existing research, this study examines the influencing factors from five aspects: Industrial Structure (Str), Digital Technology Innovation (Tec), The Degree of Government Intervention (Gov), Urbanization Level (Urd), and The Degree of Openness (Open). Among them, the industrial structure represents whether regional industries are developing toward advanced, informalized and service-oriented development, reflecting the sustainability characteristics of local industries. Digital technological innovation refers to the degree of investment in innovation and R&D by residents, enterprises, and the government. It is also a reflection of the efficiency of the application of digital infrastructure in each region. The degree of government intervention indicates the importance the government attaches to the digital economy and green development, and the higher the attention, the higher the government's financial investment. Urbanization is a necessary path to modernization and an important way to solve the problems of agriculture, rural areas, and farmers. The level of urbanization development well reflects the trend of rural to urban evolution. However, the degree of external development demonstrates the comprehensive economic strength of a region. The specific ways of measurement are shown in Table 7.
The previously measured values and drivers of coupling coordination are substituted into the regression model and empirically analyzed using the least squares (OLS) method with fixed provinces and time. In this paper, the data are first cleaned and screened to exclude samples with missing values of relevant variables, and all continuous variables are subjected to upper and lower 1% shrinking tail treatment. The measurement results are shown in the Table 8.
The results indicate that industrial structure, digital technology innovation, government intervention, urbanization level, and openness all have significant effects on the coupling coordination development among digital economy, environmental regulation, and carbon emission intensity in China. However, regional differences exist in the regression results. In the eastern region, factors other than government intervention are the main influences, with urbanization level having the most significant impact. In the Western region, digital technology innovation plays a major role, suggesting significant potential for industrial innovation development in the West. The central region is mainly influenced by government intervention and openness. Notably, government intervention is negatively correlated with coupling coordination development, indicating that excessive government intervention may not be conducive to the coupling coordination development of digital economy, environmental regulation, and carbon emission intensity and may lead to some degree of resource waste. In the northeastern region, openness and urbanization levels are the primary influencing factors, possibly due to the region's constrained economic development.
This paper examines the coupling coordination degree and the spatiotemporal evolution characteristics of digital economy, environmental regulation, and carbon emission intensity in 30 Chinese provinces from 2012 to 2022. The serious lack of data in Tibet may lead to deficiencies in China-wide measurement. Also, the number of influencing factors may limit the experimental results. This study explores the coupled coordination in Chinese provinces, which provides scholars with experiences and lessons for further research on cities and firms. The study finds that, over the sample period, the coupling coordination degree among China's digital economy, environmental regulation, and carbon emission intensity shows an upward trend with increasingly concentrated distribution. Kernel density analysis reveals no extreme differences in the coupling coordination degree across regions. Spatially, the eastern region displays higher levels of coupling coordination development compared to other areas, while the northeastern and northwestern regions exhibit relatively lower levels, primarily due to differences in industrial digitalization development. Spatial correlation tests indicate a relatively stable positive spatial correlation for the coupling coordination development of digital economy, environmental regulation, and carbon emission intensity, with most regions showing “low-low” and “high-high” clustering patterns. The factor analysis reveals that industrial structure, digital technology innovation, government intervention, urbanization level, and openness all have significant effects on the coupling coordination development. However, the primary influencing factors vary across regions.
Based on the conclusions above, the following recommendations are proposed.
Enhance industrial digitalization infrastructure and promote the integration of digital economy with green and low-carbon development. In response to the “14th Five-Year Plan for National Informatization,” we will promote the construction of a new-generation information infrastructure, accelerate the realization of higher-quality interconnections and provide solid information infrastructure support for the development of the digital economy, so as to popularize the scope of digital technology, explore the mode of environmental regulation that involves all people, and expand the promotion of the digital economy for green development. Effectiveness of the digital economy in promoting green development. Deeply integrate digital technologies with production sectors, government departments, and enterprises to fully harness the potential of digital economy in promoting high-quality economic development across regions while effectively improving the economic efficiency of carbon emissions. By leveraging digital technology innovations, carbon emissions in production sectors can be more effectively monitored and controlled. High-efficiency and precise carbon reduction technologies can further improve energy utilization and energy economic efficiency. The government is the key to guiding the digital economy and green development, while enterprises are an important component of achieving digital innovation and energy conservation and emission reduction. Manufacturing enterprises should accelerate the digital transformation of industrial structure and green transformation, improve resource utilization and production efficiency, and gradually form a sustainable development trend of new technologies, new business forms and new services. The logistics industry can realize the intelligent sharing of information and the pairing of supply chains with the support of digital economy technologies (e.g., information broadband, mobile networks, etc.), and the promotion of new energy sources will also reduce the emission of pollutants in its industry.
Optimize government intervention methods and strengthen the effectiveness of environmental regulation. Under the challenge of the carbon peaking and carbon neutrality goals and global climate change, the Chinese government has introduced the Environmental Protection Law, the Ecological Environment Policy, and the Green Credit Guidelines to manage the environment and constrain carbon emissions.
The factor analysis reveals that government intervention negatively impacts the coupling coordination development of China's digital economy, environmental regulation, and carbon emission intensity. This is likely due to overly strong fiscal control by the government and a lag in regulatory measures. In some regions, the failure of resource allocation and uncoordinated environmental governance without considering local circumstances leads to inefficient use of funds, with limited positive effects on digital economy and environmental governance. Therefore, local governments should take into account specific regional policy conditions, comprehensively deploying digital economy development and environmental governance initiatives, and using digital technology innovations to improve regulatory efficiency while reducing the time lag in intervention measures. Additionally, governments should increase support for corporate green technology innovation, encouraging companies to use digital technologies for energy conservation and carbon reduction innovations. Enterprises with high pollution and low energy efficiency should be regulated and reformed.
Strengthen regional cooperation and narrow gaps between different regions. From a provincial perspective, the coupling coordination degree of digital economy, environmental regulation, and carbon emission intensity exhibits positive spatial autocorrelation, with a clear “high-high” and “low-low” clustering pattern. The eastern region, being a key “high-high” cluster, should continue to leverage its regional advantages, further driving industrial structure optimization and technological innovation. The western and northeastern regions should actively learn from higher development regions, increase investment in digital technology innovation, advance the digitalization of industrial structures, and improve policy environments. Strengthening cooperation between regions, establishing digital platforms, and fostering regional technical collaboration can help achieve coordinated pollution control and carbon emission reduction across regions. For the late formation of digital infrastructure and intensive heavy industry in the northwest and northeast regions, they will benefit greatly from the government's increased funding and policy support and other regulatory measures. These regions can learn from the experience of Chongqing Municipality, Sichuan Province and other southwestern regions, the government actively encourages the development of green industry, and provides a series of financial subsidies, land policies. At the same time, these regions continue to improve infrastructure, Southwest China to accelerate the construction and coverage of 5G networks, fiber optic broadband and other communication networks, on this basis to further form a big data center landing, further use of the rich local resources, but also the formation of the phenomenon of convergence of external resources to the inside. Regions with backward coupling coordination should coordinate the relationship between regional economy, resource exploitation, and carbon emissions, and not overly pursue economic production while neglecting ecological environment governance. By building a digital information platform and leveraging the ability to integrate resources, digital production can be formed, thereby improving resource utilization efficiency and reducing waste and pollution. In addition, emphasis is placed on local enterprises' green technology innovation, production of green products, and vigorous development of new energy to reduce the use of traditional fossil fuels.
The original contributions presented in the study are included in the article/supplementary material, further inquiries can be directed to the corresponding author.
S-BT: Writing – original draft, Writing – review & editing.
The author(s) declare that no financial support was received for the research, authorship, and/or publication of this article.
The author declares that the research was conducted in the absence of any commercial or financial relationships that could be construed as a potential conflict of interest.
All claims expressed in this article are solely those of the authors and do not necessarily represent those of their affiliated organizations, or those of the publisher, the editors and the reviewers. Any product that may be evaluated in this article, or claim that may be made by its manufacturer, is not guaranteed or endorsed by the publisher.
1. ^Coal at 1.9003 kg CO2/kg, coke at 2.8604 kg CO2/kg, crude oil at 3.0202 kg CO2/kg, gasoline at 2.9251 kg CO2/kg, kerosene at 3.0179 kg CO2/kg, diesel at 3.0959 kg CO2/kg, fuel oil at 3.1705 kg CO2/kg, and natural gas at 2.1622 kg CO2/m3.
2. ^1. Beijing, 2. Tianjin, 3. Hebei, 4. Shanxi, 5. Inner Mongolia, 6. Liaoning, 7. Jilin, 8. Heilongjiang, 9. Shanghai, 10. Jiangsu, 11. Zhejiang, 12. Anhui, 13. Fujian, 14. Jiangxi, 15. Shandong, 16. Henan, 17. Hubei, 18. Hunan, 19. Guangdong, 20. Guangxi, 21. Hainan, 22. Chongqing, 23. Sichuan, 24. Guizhou, 25. Yunnan, 26. Shaanxi, 27. Gansu, 28. Qinghai, 29. Ningxia, 30. Xinjiang.
Anselin, L. (1995). The local indicators of spatial association (LISA). Geograph. Anal. 27, 93–115. doi: 10.1111/j.1538-4632.1995.tb00338.x
Barratt, A. L., Bell, K. J. L., Charlesworth, K., and McGain, F. (2022). High-value health care is low-carbon health care. Culling low-value care will cut health care carbon emissions. Med. J. Austr. 216, 67–68. doi: 10.5694/mja2.51331
Blackman, A., Li, Z., and Liu, A. A. (2018). Efficacy of command-and-control and market-based environmental regulation in developing countries. Ann. Rev. Resour. Econ. 10, 381–404. doi: 10.1146/annurev-resource-100517-023144
Chen, P., Xie, R., and Lu, M. (2020). “Resource conservation” or “environmental friendliness”: how do urban clusters affect total-factorecological performance in China? Int. J. Environ. Res. Publ. Health 17:4361. doi: 10.3390/ijerph17124361
Chen, S., and Chen, D. (2018). Smog pollution, government governance, and high-quality economic development. Econ. Res. J. 2, 20–34.
Chen, Z., Kahn, M. E., Liu, Y., and Wang, Z. (2016). The consequences of spatially differentiated water pollution regulation in China. NBER Work. Pap. 2016:w22507. doi: 10.3386/w22507
Dao, N. B., Dogan, B., Ghosh, S., Kazemzadeh, E., and Radulescu, M. (2024). Toward sustainable ecology: how do environmental technologies, green financial policies, energy uncertainties, and natural resources rents matter? Clean Technol. Environ. Pol. 24:2887. doi: 10.1007/s10098-024-02887-y
Deng, H., and Yang, L. (2019). Smog control, local competition, and industrial green transformation. China Industr. Econ. 10, 118–136.
Dong, F., Hu, M., Gao, Y., Liu, Y., Zhu, J., and Pan, Y. (2022). How does digital economy affect carbon emissions? evidence from global 60 countries. Sci. Tot. Environ. 852:158401. doi: 10.1016/j.scitotenv.2022.158401
Dong, K., Sun, R., and Hochman, G. (2017). Do natural gas and renewable energy consumption lead to less CO2 emission? empirical evidence from a panel of BRICS countries. Energy 141, 1466–1478. doi: 10.1016/j.energy.2017.11.092
Duan, H., and Sun, X. (2024). Research on technology spillover of digital economy affecting energy consumption intensity in Beijing-Tianjin-Hebei Region. Sustainability 16:114562. doi: 10.3390/su16114562
Eichhorst, W., Hinte, H., Rinne, U., and Tobsch, V. (2017). How big is the gig? assessing thepreliminary evidence on the effects of digitalization on the labor market. Manag. Rev. 28, 298–318 doi: 10.5771/0935-9915-2017-3-298
Galeotti, M., Salini, S., and Verdolini, E. (2020). Measuring environmental policy stringency: approaches, validity, and impact on environmental innovation and energy efficiency. Energy Pol. 136:111052. doi: 10.1016/j.enpol.2019.111052
Gao, X., and Li, W. (2020). Spatial distribution characteristics and influencing factors of digital economy development in China: Based on the Moran index and spatial econometric model. J. Comput. Econ. 45, 1–12.
Ge, W., Xu, Y., Liu, G., Shen, B., Su, X., Liu, L., et al. (2022). Exploring the impact of the digital economy on carbon emission efficiency under factor misallocation constraints: new insights from China. Front. Environ. Sci. 10:953070. doi: 10.3389/fenvs.2022.953070
Hao, Y., Deng, Y., Lu, Z.-N., and Chen, H. (2018). Is environmental regulation effective in China? evidence from city-level panel data. J. Clean.Prod. 188, 966–976. doi: 10.1016/j.jclepro.2018.04.003
He, F., and Liu, H. (2019). Performance enhancement evaluation of the digital transformation of physical enterprises from the perspective of the digital economy. Reform. 4, 137–148.
Hou, J., Li, W., and Zhang, X. (2024). Research on the impacts of digital economy on carbon emission efficiency at China's City level. PLoS ONE 19:e0308001. doi: 10.1371/journal.pone.0308001
Jin, X., and Liu, Q. (2024). Spatiotemporal evolution and obstacle analysis of the coupling coordination between the digital economy and green development. East China Econ. Manag. 38, 77–90. doi: 10.19629/j.cnki.34-1014/f.231016014
Kazemzadeh, E., Fuinhas, J. A., Salehnia, N., Koengkan, M., and Silva, N. (2023). Exploring necessary and sufficient conditions for carbon emission intensity: a comparative analysis. Environ. Sci. Pollut. Res. Int. 30, 97319–97338. doi: 10.1007/s11356-023-29260-8
Kazemzadeh, E., Koengkan, M., Fuinhas, J. A., Teixeira, M., and Mejdalani, A. (2022). Heterogeneous impact of electrification of road transport on premature deaths from outdoor air pollution: a macroeconomic evidence from 29 European Countries. World Electr. Vehicl. J. 13:80155. doi: 10.3390/wevj13080155
Lazzaretto, A., and Toffolo, A. (2004). Energy, economy and environment as objectives in multi-criterion optimization of thermal systems design. Energy 29, 1139–1157. doi: 10.1016/j.energy.2004.02.022
Lee, C. C., Chen, M. P., Wu, W., and Xing, W. (2021). The impacts of ICTs on tourism development: international evidence based on a panel quantile approach. Informat. Technol. Tour. 23, 509–547. doi: 10.1007/s40558-021-00215-4
Lenzen, M., and Dey, C. J. (2002). Energy and greenhouse emissions impacts of some consumer choice, technology and government outlay options. Energy Econ. 24, 377–403. doi: 10.1016/S0140-9883(02)00007-5
Li, X., Wang, L., and Wang, L. (2024). The coupling coordination evaluation and influencing factors analysis of the development of china's digital economy and the construction of an ecological civilization. Pol. J. Environ. Stud. 33, 3747–3758. doi: 10.15244/pjoes/178531
Lian, Y., Dong, H., and Cao, H. (2024). The effect of digital economy and environmental regulation on green total factor productivity: evidence from China. Glob. Fin. J. 62:101010. doi: 10.1016/j.gfj.2024.101010
Liao, Z. (1999). Quantitative evaluation and classification system of coordinated development between environment and economy: a case study of the Pearl River Delta urban agglomeration. Trop. Geogr. 2, 76–82.
Liu, Y., Yang, Y., Li, H., and Zhong, K. (2022). Digital economy development, industrial structure upgrading and green total factor productivity: empirical evidence from China's cities. Int. J. Environ. Res. Publ. Health 19:42414. doi: 10.3390/ijerph19042414
Long, H. (2024). Digital economy, carbon emission reduction constraints, and corporate green technology innovation. Technol. Econ. Manag. Res. 3, 107–112.
Lu, F., Hou, X., and Liu, Y. (2008). Coordinated development model of the economy-resource-environment system based on system coupling theory. China Popul. Resour. Environ. 18, 123–127.
Ozturk, I., and Ullah, S. (2022). Does digital financial inclusion matter for economic growth and environmental sustainability in OBRI economies? an empirical analysis. Resour. Conserv. Recycl. 185:106489. doi: 10.1016/j.resconrec.2022.106489
Parzen, E. (1962). On estimation of probability density function and model. Ann. Math. Statist. 33, 1065–1076. doi: 10.1214/aoms/1177704472
Porter, M. (1991). America's green strategy. Sci. Am. 264:168. doi: 10.1038/scientificamerican0491-168
Porter, M., and van der Linde, C. (1995). Toward a new conception of the environment competitiveness relationship. J. Econ. Perspect. 9, 97–118. doi: 10.1257/jep.9.4.97
Ren, S., Hao, Y., Xu, L., Wu, H., and Ba, N. (2021). Digitalization and energy: how does internet development affect China's energyconsumption? Energy Econ. 98:105220. doi: 10.1016/j.eneco.2021.105220
Rosenblatt, M. (1956). Remarks on some nonparametric estimates of a density function. Ann. Math. Statist. 27, 832–837. doi: 10.1214/aoms/1177728190
Shahbaz, M., Wang, J., Dong, K., and Zhao, J. (2022). The impact of digital economy on energy transition across the globe: the mediating role of government governance. Renew. Sustain. Energy Rev. 166:112620. Article 112620. doi: 10.1016/j.rser.2022.112620
Shannon, C. E. (1948). A mathematical theory of communication. Bell Syst. Tech. J. 27, 379–423. doi: 10.1002/j.1538-7305.1948.tb01338.x
Shao, S., and Razzaq, A. (2022). Does composite fiscal decentralization reduce tradeadjusted resource consumption through institutional governance, human capital, and infrastructure development? Resour. Pol. 79:103034. doi: 10.1016/j.resourpol.2022.103034
Silva, N., Fuinhas, J. A., Koengkan, M., and Kazemzadeh, E. (2024). What are the causal conditions that lead to high or low environmental performance? a worldwide assessment. Environ. Impact Assess. Rev. 104:107342. doi: 10.1016/j.eiar.2023.107342
Song, X., Tian, Z., Ding, C., Liu, C., Wang, W., Zhao, R., et al. (2022). Digital economy, environmental regulation, and ecological well-being performance: a provincial panel data analysis from China. Int. J. Environ. Res. Publ. Health 19:1811801. doi: 10.3390/ijerph191811801
Soytas, U., and Sari, R. (2009). Energy consumption, economic growth, and carbon emissions: challenges faced by an EU candidate member. Ecol. Econ. 68:1667e1675. doi: 10.1016/j.ecolecon.2007.06.014
Tang, J., and Qin, F. (2022). Analyzing the impact of local government competition on green total factor productivity from the factor market distortion perspective: based on the three stage DEA model. Environ. Dev. Sustainabil. 24, 14298–14326. doi: 10.1007/s10668-021-02032-5
Tao, M., Sheng, M. S., and Wen, L. (2023). How does financial development influence carbon emission intensity in the OECD countries: some insights from the information and communication technology perspective. J. Environ. Manag. 335:117553. doi: 10.1016/j.jenvman.2023.117553
Tapscott, D. (1996). The Digital Economy: Promise and Peril in the Age of Networked Intelligence. New York, NY: McGraw-Hill.
Ulucak, R., Khan, S. U. D., Baloch, M. A., and Li, N. (2020). Mitigation pathways toward sustainable development: is there any trade-off between environmental regulation and carbon emissions reduction? Sustain. Dev. 28, 813–822. doi: 10.1002/sd.2032
Wang, C., Engels, A., and Wang, Z. (2018). Overview of research on China's transition to low-carbon development: the role of cities, technologies, industries and the energy system. Renew. Sustain. Energy Rev. 81, 1350–1364. doi: 10.1016/j.rser.2017.05.099
Wang, H., Wu, Y., and Zhu, N. (2024). Measurement and spatio-temporal heterogeneity analysis of coupling coordination between development of digital economy and agricultural carbon emission performance. PLoS ONE 19:305231. doi: 10.1371/journal.pone.0305231
Wang, J., Zhu, J., and Luo, Q. (2021). Measurement of the development level and evolution of China's digital economy. J. Quant. Technol. Econ. 38, 26–42. doi: 10.13653/j.cnki.jqte.2021.07.002
Wang, L., and Shao, J. (2023). Digital economy, entrepreneurship and energy efficiency. Energy 269:126801. doi: 10.1016/j.energy.2023.126801
Wang, S., Kong, W., Ren, L., Zhi, D., and Dai, B. (2021). Misconceptions and corrections of the coupling coordination degree model in China. J. Nat. Resour. 36, 793–810. doi: 10.31497/zrzyxb.20210319
Wang, S., Wang, A., Liu, S., Zhang, C., Qiao, L., and Li, X. (2024). Research on the coupling coordination relationship between the digital economy and high-quality energy development: evidence from China. Heliyon 10:e24637. doi: 10.1016/j.heliyon.2024.e24637
Xu, Q., Zhong, M. R., and Li, X. (2022). How does digitalization affect energy? Int. Evid. Energy Econ. 107:105879. doi: 10.1016/j.eneco.2022.105879
Yang, J., and Cheng, Y. (2024). Research on the spatiotemporal differentiation and influencing factors of the coupling coordination degree of the digital economy, environmental regulation, and sustainable development. Ecol. Econ. 40, 154–163.
Yu, Z., Liu, S., and Zhu, Z. (2022). Has the digital economy reduced carbon emissions? analysis based on panel data of 278 cities in China. Int. J. Environ. Res. Publ. Health 19:1811814. doi: 10.3390/ijerph191811814
Yuan, S., Oluwaseyi, M., Yilmaz, G., Shaheen, R., Ameen, A., and Tan, Z. (2021). Digitalization of economy is the key factor behind fourth industrial revolution: how G7 countries are overcoming with the financing issues? Technol. Forecast. Soc. Chang. 165:120533. doi: 10.1016/j.techfore.2020.120533
Zhang, W., Li, G., Uddin, M. K., and Guo, S. (2020). Environmental regulation, Foreign investment behavior, and carbon emissions for 30 provinces in China. J. Clean. Prod. 248:119208. doi: 10.1016/j.jclepro.2019.119208
Zhang, W., Zhou, H., Chen, J., and Fan, Z. (2022). An empirical analysis of the impact of digital economy on manufacturing green and low-carbon transformation under the dual-carbon background in China. Int. J. Environ. Res. Publ. Health 19:2013192. doi: 10.3390/ijerph192013192
Keywords: digital economy, environmental regulation, carbon emission intensity, coupling coordination measurement, spatiotemporal evolution
Citation: Tang S (2024) Coupling coordination measurement and spatiotemporal evolution characteristics of digital economy, environmental regulation, and carbon emission intensity—Empirical evidence from Chinese provinces. Front. Environ. Econ. 3:1511080. doi: 10.3389/frevc.2024.1511080
Received: 14 October 2024; Accepted: 19 November 2024;
Published: 10 December 2024.
Edited by:
Murad Harasheh, University of Bologna, ItalyReviewed by:
Emad Kazemzadeh, Ferdowsi University of Mashhad, IranCopyright © 2024 Tang. This is an open-access article distributed under the terms of the Creative Commons Attribution License (CC BY). The use, distribution or reproduction in other forums is permitted, provided the original author(s) and the copyright owner(s) are credited and that the original publication in this journal is cited, in accordance with accepted academic practice. No use, distribution or reproduction is permitted which does not comply with these terms.
*Correspondence: Shibo Tang, dGFuZ3hqdV8yMDAzQDE2My5jb20=
Disclaimer: All claims expressed in this article are solely those of the authors and do not necessarily represent those of their affiliated organizations, or those of the publisher, the editors and the reviewers. Any product that may be evaluated in this article or claim that may be made by its manufacturer is not guaranteed or endorsed by the publisher.
Research integrity at Frontiers
Learn more about the work of our research integrity team to safeguard the quality of each article we publish.