- Division of Food Production and Society, Norwegian Institute of Bioeconomy Research (NIBIO), Ås, Norway
Eco-efficiency is gaining popularity to measure the agricultural system's economic and environmental performance. The dynamic eco-efficiency of the agricultural system is assessed in this study using a parametric frontier framework that considers the inter-temporal nature of production decisions and methane emissions. We also estimated the static eco-efficiency model for comparison. The empirical analysis is based on 30 years of unbalanced panel data from 692 dairy farms (1991–2020). The generalized method of moment estimation is used to compute dynamic models. Both dynamic and static models show that dairy farms in the study area used available technology inefficiently, which means that some farmers produced lower outputs per input than the best-performing farmers. According to the dynamic eco-efficiency score, dairy farms only generate 94% of the maximum viable output for the input used. If all dairy farms became eco-efficient, an average dairy farm could raise its output by about 6% using the existing technology. According to the projected scores, farmers might improve their eco-efficiency by 10% on average without using more inputs in a static condition. Policymakers should encourage dairy farms to share information with the best-performing dairy farms on how to improve production while considering environmental concerns.
1. Introduction
Growing concern for the sustainability of current agricultural systems and livestock farming has triggered intensive research in the field. Policymakers encourage farm businesses to adopt sustainable decisions and behaviors to maintain long-term food security while protecting the environment (Alem, 2023). Evaluating food production system performance while considering environmental issues is critical for designing policies and practices that sustain long-term agricultural growth (Pacini et al., 2003; Alem, 2021). Eco-efficiency, also known as economic-ecological development, became popular in the 1990s to attain long-term agricultural growth (Gołaś et al., 2020). Eco-efficiency is a management strategy that focuses on increasing agricultural output while utilizing fewer resources, decreasing waste, and cutting emissions (Peças et al., 2019).
The issue of eco-efficiency in the dairy industry is addressed by several frameworks in the literature, such as those from Pelletier et al. (2008), Picazo-Tadeo et al. (2011), Shortall and Barnes (2013), Madau et al. (2017), Wettemann and Latacz-Lohmann (2017), Adenuga et al. (2018), Cecchini et al. (2018), Le et al. (2020), Le et al. (2020), and BaleŽentis et al. (2022). Different methods were employed in earlier studies to calculate eco-efficiency scores. Both the ratio and frontier approaches can be used to calculate overall eco-efficiency, and each has advantages and disadvantages (see for detail Song and Chen, 2019). According to Kuosmanen and Kortelainen (2005), eco-efficiency is the proportion of economic value acquired to the environmental effect generated. Cost-benefit assessments commonly use life cycle costs to determine the economic value contributed (Huppes and Ishikawa, 2005; Shortall and Barnes, 2013). The most common environmental impact is an aggregation figure of material use, energy use, pollutants, and wastes (Shortall and Barnes, 2013). Environmental impacts are challenging to classify and combine using ratio techniques (see Huppes and Ishikawa, 2005; Song and Chen, 2019 for detail). On the other hand, the parametric approach using stochastic frontier analysis (SFA) bases its definition of eco-efficiency on the ratio of actual production to frontier output (both desirable and undesirable) for a certain farm (see Orea and Wall, 2017; Song and Chen, 2019; Stetter and Sauer, 2022). Frontier-based techniques can be classified into three categories: parametric, semi-parametric, and non-parametric methods. If a farm is on the frontier, its real production point is entirely eco-efficient; if it is below the frontier, it is eco-inefficient. The pros and cons of each estimating strategy have been a topic of discussion in the economics literature. Given that it can consider measurement errors, stochastic effects, and single-step estimations of inefficiency effects, an SFA appears to be the best methodology for agricultural research to account for the whole farming system (see for details Cabrera et al., 2010; Alem, 2018).
To assess the dynamic eco-efficiency of resource use, a non-parametric approach such as the Data Envelopment Analysis framework has been widely used in the economics literature (see for example Gómez-Calvet et al., 2016; Pishgar-Komleh et al., 2021; Jiang et al., 2022). This paper contributes to the literature in the following ways. We estimated the dynamic model using the parametric approach, but unlike Alem (2020), we evaluated eco-efficiency by the including both desired (main output) and undesirable (environmental effect) outputs. Since environmental outputs like CH4 are a cost to society rather than a cost to the dairy farm, the output should be adjusted to reflect this. To the best of our knowledge, no previous research has investigated the dynamic of eco-efficiency using a parametric framework approach that accounts for the entire farming system. We estimated a static model for comparison. In addition, we had the advantage of working with a large panel dataset of the dairy farm system from 1991 to 2020.
The rest of this article is organized as follows. The characteristics of Norwegian agriculture and greenhouse gas emissions are discussed in Section 2. Section 3 explains the theoretical framework for modeling environmental production technology and its dynamic applications. The empirical model and estimation procedures are described in Section 4, and the data are presented in Section 5. In Section 6, the findings are highlighted and addressed. Concluding remarks are included in Section 7.
2. Norwegian agriculture system and greenhouse gas emissions
In Norway, agriculture is typically small and run by family members who are primarily concerned with keeping the business running. Farmland accounts for only 3% of Norway's total land area with adequate climatic conditions for agriculture (Alem, 2023). These farmlands are often scattered, small, and steep. Rogaland (in southern Norway) is the principal farming region of the nation (SSB, 2022). In many regions of Norway, the single crop is the production of fodder, which is primarily made of grass, and the basis of Norwegian agriculture is grass-based animal husbandry. This explains why animal production has grown to be Norway's most important agricultural product, with the dairy farming system employing close to a quarter of all farmers (Alem et al., 2019; Alem, 2023). Agriculture in Norway has been and continues to be heavily regulated and subsidized because of unfavorable conditions, and farmers are subject to complex agricultural legislation that have a substantial impact on their output choices (Bonesmo et al., 2013). Norway's agricultural sector contributes to global climate change by emitting greenhouse gases into the environment and contributes to the environmental problem by emitting 4,5 million tons of equivalent CO2 in 2020 (SSB, 2022). Livestock farming has substantial environmental consequences, including GHG emissions (BaleŽentis et al., 2022). Methane emissions were the largest among all sources of greenhouse gases emitted into the atmosphere during the previous 40 years (SSB, 2022). Norwegian agriculture released 2.51 million tons of methane in 2018, more than any other industry (CAIT Climate Data Explorer, 2022). As a result, the purpose of this research is to estimate the performance of dairy farms while accounting for environmental output (CH4).
3. Conceptual model
We used Alem (2020) approach but modified the output to be more environmentally friendly. That is using the following production function.
where is the difference between desirable outputs (Q) and undesirable outputs (b) such as methane (CH4) emissions. The function form used, such as Translog or quadratic is denoted by f(xit; β); t is the time (year); β is an estimation of technological parameters; i signifies the farm unit. Consider be the real output generated after deducting environmental expenses or undesirable output (b). We consider also θ to be the rate at which outputs are adjusted.
If the adjustment speed is <1, the total performance will be lower than the expected output (Alem, 2021). The actual output (qit) for the first period of production is just θ of the expected output . The fraction of potential output for upcoming periods of production is just θ as important as the proportion of Q it* for past periods of production. Therefore, using Alem (2020), the dynamic output generation technique may be described:
Substituting Equations (4)–(8),
The current environmental adjusted output depends on the current and previous inputs, as shown in Equation (6). We estimated CH4 emissions (b) using the Tier 2 approach which includes country-specific estimates from the Norwegian Environment Agency (NIR, 2020) and follows the Intergovernmental Panel on Climate Change technique (IPCC, 2006). According to IPCC (2006) and Alem (2023), the basic equation for estimating the emission factor for enteric fermentation is as follows
GED =137.9 + 0.0249 * Milk 305 * 0.2806 × Concentrate proportion; and Ym = 7.38 – 0.00003 × Milk 305 – 0.01758 × Concentrate proportion. GED = gross energy intake for dairy farms, MJ/day; Ym = methane conversion rate, %. The factor 55.65 (MJ/kg CH4) is the energy content of methane. Milk305 = 305 days lactation yield of energy-corrected milk (ECM).
The concentration proportion is the percentage of concentrates in the diet calculated using net energy, and Equation (7) assumes a year-long emission factor. Moreover, we estimated Dairy farm CH4 emissions Kg/year using Alem's (2023) approach.
4. Empirical static and dynamic models
4.1. Model specifications
We choose a translog (TL) specification and following Alem (2020) method, Equation (3) is expressed in TR and log form as:
where is a vector of outputs adjusted for environmental output (market value of methane), xkjt denotes the inputs used; Dt denotes the variable for years, and the Greek letters are variables that can be calculated. εit is the sum of two error terms (εit = vit−uit). vit is the error term and we assumed . uit is eco-efficiency while ωi captures unobserved heterogeneity. The model was estimated following Greene (2005) procedures.
The sluggish adjustment of inputs is incorporated into the dynamic stochastic production frontier as:
4.2. Estimation of the dynamic model
The generalized method of moments (GMM) estimator was used for the analysis because the dependent and the lagged dependent variables are both a function of the error component (Arellano and Bond, 1991; see for detail Blundell and Bond, 1998; Alem, 2020). A one-step GMM estimator is used to calculate Equation (9). We ran several tests to ensure that the model was valid. The Arellano and Bond (1991) test is used to determine the residual in the difference of second-order autocorrelation. Furthermore, the validity of the over-identifying limitations is tested using Sargan's J. We used Battese and Tim (1988) procedure to calculate the eco-efficiency score for both dynamic and static models. We used data from Norwegian dairy farms for our empirical application.
5. Data
The dataset used in this study is based on a farm-level survey conducted by the Norwegian Institute of Bioeconomy Research (NIBIO). For the case study and empirical analysis, we used an unbalanced panel of 6,229 observations from 692 dairy farms from 1991 to 2020. To guarantee that milk production is the primary agricultural output, we select farms where milk sales represent at least 80% of total farm income. To represent environmental production technology, two outputs (desirable and undesired) and four inputs (land, labor, materials, and capital) are employed. Desirable output (dairy output) consists of dairy output, which reflects overall farm revenue in dairy farming (Q). Undesirable output (CH4 emission) is estimated following Alem (2023) and the Intergovernmental Panel on Climate Change (IPCC, 2006) methodology incorporating the country-specific estimates from the Norwegian Environment Agency (NIR, 2020). The value of farm-level CH4 emissions was calculated using data from Statistics Norway (SSB, 2022). The empirical model (9) specifies the Translog function, which has four inputs that follow the literature (Lien et al., 2018; Alem et al., 2019). Agricultural land (x1) is measured in hectares, and labor (x2) is defined as the total work hours spent on the farm. Materials (x3), which includes feed, energy, and power expenditures; and Capital assets (x4) which comprises the implicit quantity index value of machinery, buildings, and cattle. All figures were calculated in NOK and adjusted for 2015 prices. We only considered farms with at least 3 years of data in the analysis to contain panel characteristics. Table 1 provides a summary of the input and output variables.
Norway's dairy farms are small. The average annual dairy revenue was roughly 1.5 million NOK and has increased over time, resulting in an average annual CH4 emission of 0.99 tons. However, because of price differences over time, the average annual CH4 emission value fluctuates. All production inputs used in the dairy farm increase over time (Figure 1).
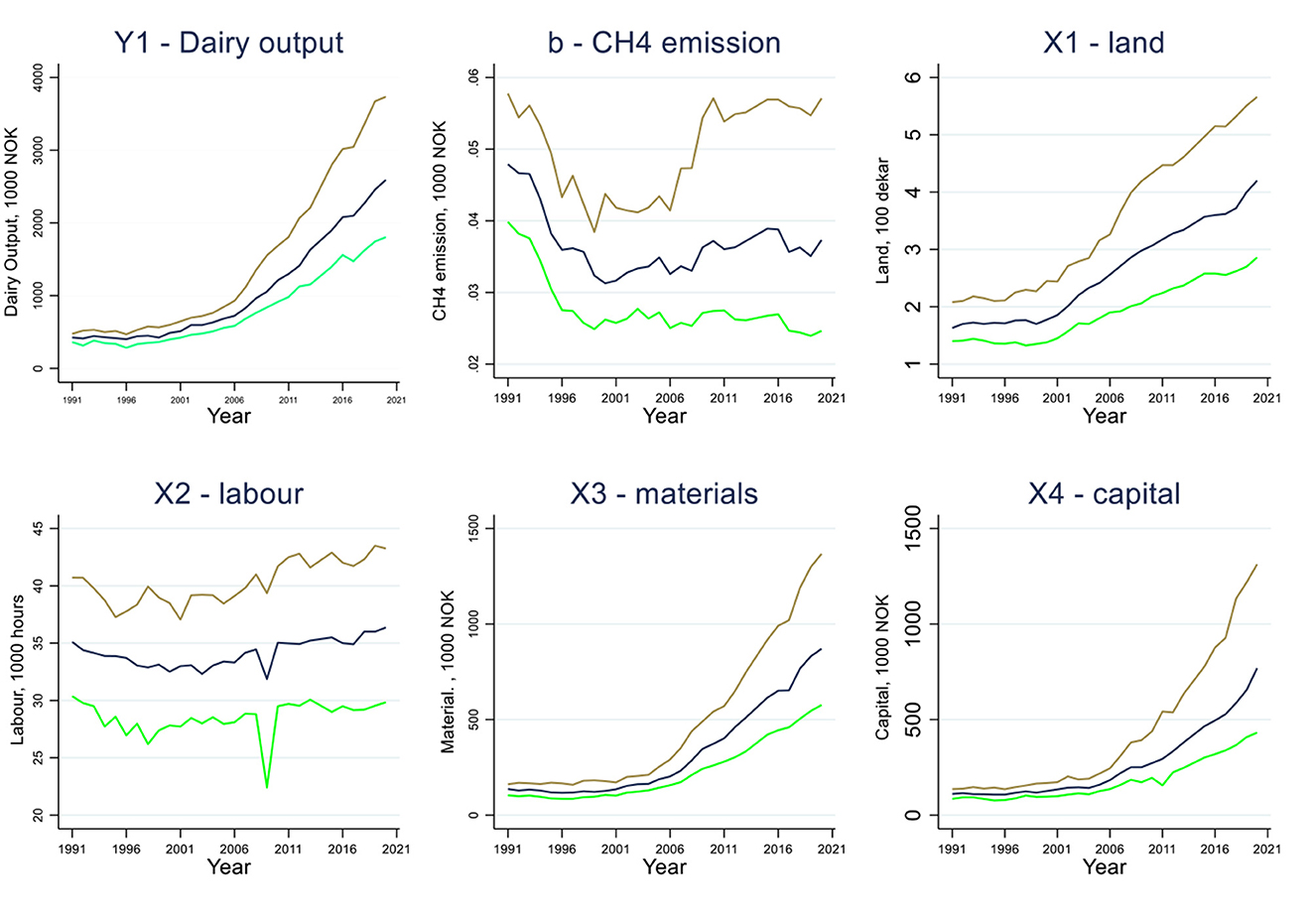
Figure 1. The median, first, and third quantile values of outputs and inputs are shown in the center, bottom, and top lines, respectively.
6. Main results and findings
The parameter values for the stochastic frontier dynamic analysis are shown in Table 2. We did many specification tests to decide on the proper function and empirical model for the survey data. The test showed that most of the total error variation is because of technological inefficiencies. The testing of a translog simplification of the Cobb-Douglas functional form for stochastic models was rejected. As a result, for this analysis, the functional form of the translog was used.
The Arellano-Bond test statistic for AR (1) (p-value = 0.42) represents that the regression does not have a first-order autocorrelation problem (Table 1). As a result, white noise to the sectors is not related sequentially, resulting in consistent estimates and no significant evidence of serial correlation in first-differenced errors at order 2. The J-statistic validates the validity of the instruments (p-value = 0.167) (see for details Sargan, 1958). Because the GMM estimate relies on internal instruments, there may be numerous valid instrumental variables. The parameter estimates for the stochastic frontier static model are shown in Table 3. The Table shows that all parameter estimates are statistically significant. All variables are divided by their sample mean before estimation, so the parameters in Tables 2, 3 can be directly interpreted as elasticities. Both models have the highest partial elasticities of material use, which are statistically significant (P < 0.001). Material inputs had an elasticity of 0.51 to 0.34, which implies that a 1% increase in material inputs, ceteris paribus, would lead to a 0.34–0.51% increase in dairy output. According to the findings, focusing on technology to increase material input productivity is critical for improving the dairy industry. The time-trend variable's first-order coefficient is an estimate of the average annual rate of technological change (Wang and Ho, 2010; Alem et al., 2019). The time trend coefficient, which had values of 0.011 for the dynamic model and 0.022 for the static model, was statistically significant at the 1% level, implying that technological progress occurred because of the adoption of new environmental production technology in the dairy industry at a rate ranging from 2.2 to 1.1% per year from 1991 to 2020. Alem (2021) reported on technological advancements in Norwegian dairy farms from 2000 to 2018.
Table 4 shows the projected eco-efficiency scores. Both dynamic and static models show that dairy farms in the study area used available technology inefficiently, which means that some farmers produced lower outputs per input than the best-performing farmers. The dynamic model average is 0.94, while the static eco-efficiency score is 0.90. The dynamic and static eco-efficiency scores differ considerably according to the Welch test (see Table 4). The static model understates the efficiency since the dynamic eco-efficiency ratings are higher.
Dairy farms only produce 94% of the highest feasible production for the input utilized, according to the dynamic eco-efficiency score. For example, if every dairy farm became more ecologically friendly, production could increase by around 6%. Farmers may improve their eco-efficiency by 10% on average with the existing input quantity, according to the projected scores in the static model. Table 2 also displays the distribution of the sample farms based on their eco-efficiency score. In dynamic and static models, for example, 25% of farms are 0.92 and 0.87% eco-efficient, respectively, whereas 75% of sample farms are 0.96 and 0.94% eco-efficient. Figure 2 also shows that the static model's eco-efficiency estimations are more dispersed than the dynamic models.
7. Conclusion and policy implications
This study calculates dynamic eco-efficiency on dairy farms while accounting for intertemporal production decisions and CH4 emissions. We estimate a static model for comparison. Farm-level panel data from 1991 to 2020 was used to examine the Norwegian dairy system. Both dynamic and static models show that dairy farms used available technology in the area inefficiently, i.e., some farmers produced lower outputs from the inputs they used or used more inputs to produce the same output compared to the best-performing farmers. The mean eco-efficiency score for the dynamic model was 0.94, compared to 0.90 for the static model. According to the dynamic eco-efficiency score, dairy farms only generate 94% of the maximum viable output for the input used. If all dairy farms became eco-efficient, an average dairy farm could raise its output by about 6%. According to the projected scores, in a static condition, farmers might improve their eco-efficiency by 10 % on average without using more inputs. The findings in both models indicate that Norwegian dairy farms show positive technological progress.
The analysis in this paper has important policy implications. First, both models show that resources are being used inefficiently; therefore, policymakers should encourage dairy farms to share experience and information with the best-performing dairy farms on how to improve production while taking environmental concerns into account. Second, both models have the highest partial elasticities of the production material. Policy intervention and dairy sector improvement focusing on technology related to material input are critical. Furthermore, we assessed the dairy farm's performance by adjusting the societal cost of Ch4 emissions. As a result, emission-reduction policies and technology development, such as dairy feed that emits less CH4, improve the overall performance of dairy farms.
The study looked at the environmental impact of livestock methane (CH4) emissions in both dynamic and static models. As a result, future research may look into other environmental issues like biodiversity, fertilizer consumption, and waste.
Data availability statement
The datasets presented in this article are not readily available because the data collected at farm is confidential and the responsibility of NIBIO. Requests to access the datasets should be directed to aGFidGFtdS5hbGVtQG5pYmlvLm5v.
Author contributions
The author confirms being the sole contributor of this work and has approved it for publication.
Funding
The Norwegian Research Council supported this work (Grant Number: 333502, 2022) for a project of multi-criteria assessment, decision support, and management tools for sustainable circular mixed farming systems for dairy production.
Acknowledgments
The author acknowledges the financial support through the partners of the Joint Call of the Cofund ERA-Nets SusCrop (Grant No. 771134), FACCE ERA-GAS (Grant No. 696356), ICT-AGRI-FOOD (Grant No. 862665), and SusAn (Grant No. 696231).
Conflict of interest
The author declares that the research was conducted in the absence of any commercial or financial relationships that could be construed as a potential conflict of interest.
Publisher's note
All claims expressed in this article are solely those of the authors and do not necessarily represent those of their affiliated organizations, or those of the publisher, the editors and the reviewers. Any product that may be evaluated in this article, or claim that may be made by its manufacturer, is not guaranteed or endorsed by the publisher.
References
Adenuga, A. H., Davis, J., Hutchinson, G., Donnellan, T., and Patton, M. (2018). Modeling regional environmental efficiency differentials of dairy farms on the island of Ireland. Ecol. Indic. 95, 851–861. doi: 10.1016/j.ecolind.2018.08.040
Alem, H. (2018). Effects of model specification, short-run, and long-run inefficiency: an empirical analysis of stochastic frontier models. Agricul. Econ. 64, 508–516. doi: 10.17221/341/2017-AGRICECON
Alem, H. (2020). Performance of the Norwegian dairy farms: a dynamic stochastic approach. Res. Econ. 74, 263–271 doi: 10.1016/j.rie.2020.07.006
Alem, H. (2021). The role of technical efficiency in achieving sustainable development: a dynamic analysis of Norwegian dairy farms. Sustainability 13, 1841. doi: 10.3390/su13041841
Alem, H. (2023). Accounting for heterogeneity in performance evaluation of Norwegian dairy and crop producing farms. Economies 11, 9. doi: 10.3390/economies11010009
Alem, H., Lien, G., Hardaker, J. B., and Guttormsen, A. (2019). Regional differences in technical efficiency and technological gap of Norwegian dairy farms: a stochastic meta-frontier model. Appl. Econ. 51, 409–421. doi: 10.1080/00036846.2018.1502867
Arellano, M., and Bond, S. R. (1991). Some tests of specification for panel data: monte carlo evidence and an application to employment equations. Rev. Econ. Stud. 58, 277–297. doi: 10.2307/2297968
BaleŽentis, T., Dabkiene, V., and Štreimikiene, D. (2022). Eco-efficiency and shadow price of greenhouse gas emissions in Lithuanian dairy farms: an application of the slacks-based measure. J. Clean. Prod. 356, 131857. doi: 10.1016/j.jclepro.2022.131857
Battese, G.. E., and Tim, J. C. (1988). Prediction of firm-level technical inefficiencies with a generalized frontier production function. J. Econometr. 38, 387–399. doi: 10.1016/0304-4076(88)90053-X
Blundell, R., and Bond, S. (1998). Initial conditions and moment restrictions in dynamic panel data models. J. Econometr. 87, 115–143 doi: 10.1016/S0304-4076(98)00009-8
Bonesmo, H., Beauchemin, K. A., Harstad, O. M., and Skjelvåg, A. O. (2013). Greenhouse gas emission intensities of grass silage based dairy and beef production: a systems analysis of Norwegian farms. Livestock Sci. 152, 239–252. doi: 10.1016/j.livsci.2012.12.016
Cabrera, V. E., Solis, D., and Del Corral, J. (2010). Determinants of technical efficiency among dairy farms in Wisconsin. J. Dairy Sci. 93, 387–393. doi: 10.3168/jds.2009-2307
CAIT Climate Data Explorer (2022). Historical Emissions Data. Available online at: https://www.climatewatchdata.org/countries/NOR?end_year=2020&start_year=1990
Cecchini, L., Venanzi, S., Pierri, A., and Chiorri, M. (2018). Environmental efficiency analysis and estimation of CO2 abatement costs in dairy cattle farms in Umbria (Italy): A SBM-DEA model with undesirable output. J. Clean. Prod. 197, 895–907. doi: 10.1016/j.jclepro.2018.06.165
Gołaś, M., Sulewski, P., Wąs, A., Kłoczko-Gajewska, A., and Pogodzińska, K. (2020). On the way to sustainable agriculture—Eco-efficiency of Polish commercial farms. Agriculture 10, 438.
Gómez-Calvet, R., Conesa, D., Gómez-Calvet, A. R., and Tortosa-Ausina, E. (2016). On the dynamics of eco-efficiency performance in the European Union. Comp. Operat. Res. 66, 336–350. doi: 10.1016/j.cor.2015.07.018
Greene, W. (2005). Fixed and random effects in stochastic frontier models. J. Product. Anal. 23, 7–32. doi: 10.1007/s11123-004-8545-1
Huppes, G., and Ishikawa, M. (2005). A framework for quantified eco-efficiency analysis. J. Ind. Ecol. 9, 25–41.
IPCC. (2006). Guidelines for National Greenhouse Gas Inventories, eds H. S. Eggleston, L. Buendia, K. Miwa, T. Ngara, and K. Tanabe (National Greenhouse Gas Inventories Programme).
Jiang, P. P., Wang, Y., Luo, J., Zhu, L., Shi, R., Hu, S., et al. (2022). Measuring static and dynamic industrial eco-efficiency in China based on the MinDS–Malmquist–Luenberger model. Environ. Dev. Sustain, 25, 1–21. doi: 10.1007/s10668-022-02263-0
Kuosmanen, T., and Kortelainen, M. (2005). Measuring eco-efficiency of production with data envelopment analysis. J. Ind. Ecol. 9, 59–72.
Le, S., Jeffrey, S., and An, H. (2020). Greenhouse gas emissions and technical efficiency in Alberta dairy production: what are the trade-offs? J. Agricul. Appl. Econ. 52, 177–193. doi: 10.1017/aae.2019.41
Lien, G., Kumbhakar, S. C., and Alem, H. (2018). Endogeneity, heterogeneity, and determinants of inefficiency in Norwegian crop-producing farms. Int. J. Prod. Econ. 201, 53–61. doi: 10.1016/j.ijpe.2018.04.023
Madau, F. A., Furesi, R., and Pulina, P. (2017). Technical eco-efficiency and total factor productivity changes in European dairy farm sectors. Agricul. Food Econ. 5, 1–14. doi: 10.1186/s40100-017-0085-x
NIR (2020). Norwegian Environment Agency 2020. Greenhouse Gas Emissions 1990–2018. National Inventory Report (NIR 2020). Available online at: https://www.norskeutslipp.no/en/Agriculture/?SectorID=9211
Orea, L., and Wall, A. (2017). A parametric approach to estimating eco-efficiency. J. Agric. Econ. 68, 901–907.
Pacini, C., Wossink, A., Giesen, G., Vazzana, C., and Huirne, R. (2003). Evaluation of sustainability of organic, integrated, and conventional farming systems: a farm and field-scale analysis. Agriculture, Ecosyst. Environ. 95, 273–288. doi: 10.1016/S0167-8809(02)00091-9
Peças, P., Götze, U., Bravo, R., Richter, F., and Ribeiro, I. (2019). Methodology for selection and application of eco-efficiency indicators fostering decision-making and communication at product level—the case of molds for injection molding. Adv. Appl. Manuf. Eng. 1–52. doi: 10.1016/B978-0-08-102414-0.00001-X
Pelletier, N., Arsenault, N., and Tyedmers, P. (2008). Scenario modeling potential eco-efficiency gains from a transition to organic agriculture: life cycle perspectives on Canadian canola, corn, soy, and wheat production. Environ. Manag. 42, 989–1001. doi: 10.1007/s00267-008-9155-x
Picazo-Tadeo, A. J., Gómez-Limón, J. A., and Reig-Martínez, E. (2011). Assessing farming eco-efficiency: a data envelopment analysis approach. J. Environ. Manag. 92, 1154–1164. doi: 10.1016/j.jenvman.2010.11.025
Pishgar-Komleh, S. H., Cechura, L., and Kuzmenko, E. (2021). Investigating the dynamic eco-efficiency in the agriculture sector of the European Union countries. Environ. Sci. Pollut. Res. 28, 48942–48954. doi: 10.1007/s11356-021-13948-w
Sargan, J. D. (1958). The estimation of economic relationships using instrumental variables. Econom. J. Econom. Soc. 26, 393–415. doi: 10.2307/1907619
Shortall, O. K., and Barnes, A. P. (2013). Greenhouse gas emissions and the technical efficiency of dairy farmers. Ecol. Indic. 29, 478-488.
Song, J., and Chen, X. (2019). Eco-efficiency of grain production in China based on water footprints: A stochastic frontier approach. J. Clean. Prod. 236, 117685.
SSB (2022). Environmental Accounting. Emissions from Norwegian Economic Activity. Statistics-Norway. Available online at: https://www.ssb.no/statbank/table/09298/
Stetter, C., and Sauer, J. (2022). Greenhouse gas emissions and eco-performance at farm level: A parametric approach. Environ. Resour. Econ. 81, 617–647.
Wang, H.-J., and Ho, C.-W. (2010). Estimating fixed-effect panel stochastic frontier models by a model transformation. J. Econ. 157, 286–296. doi: 10.1016/j.jeconom.2009.12.006
Keywords: technology, emissions, dynamic eco-efficiency, dairy farm, panel data analysis
Citation: Alem H (2023) Measuring dynamic and static eco-efficiency in Norwegian dairy farms: a parametric approach. Front. Environ. Econ. 2:1182236. doi: 10.3389/frevc.2023.1182236
Received: 08 March 2023; Accepted: 23 June 2023;
Published: 18 July 2023.
Edited by:
Gideon Danso-Abbeam, University for Development Studies, GhanaReviewed by:
Fikru Alemayehu, Inland Norway University of Applied Sciences, NorwayCopyright © 2023 Alem. This is an open-access article distributed under the terms of the Creative Commons Attribution License (CC BY). The use, distribution or reproduction in other forums is permitted, provided the original author(s) and the copyright owner(s) are credited and that the original publication in this journal is cited, in accordance with accepted academic practice. No use, distribution or reproduction is permitted which does not comply with these terms.
*Correspondence: Habtamu Alem, aGFidGFtdS5hbGVtQG5pYmlvLm5v