- Instituto de Pesquisas Energéticas e Nucleares, IPEN-CNEN, São Paulo, Brazil
Sorption technologies have been proposed for the treatment of water containing methylene blue (MB), a toxic and persistent pollutant. Despite its environmental risks, the role of process variables in MB removal has not been fully explored through experimental design. The objective of this study is to assess the potential of bone meal powder (BMP), an underexplored agricultural byproduct, as an affordable adsorbent for the removal of MB from water. BMP was subjected to a series of analytical characterization techniques, and its adsorption capacity was evaluated through a comprehensive factorial design, which investigated the effects of biosorbent dosage, solution pH, and initial MB concentration. The study revealed that the highest adsorption level was 14.49 mg g−1, attained under the following conditions: 1 g L−1 BMP, pH 11, and 100 mg L−1 MB. The adsorption equilibrium was reached within 60 min, with a measured capacity (qexp) of 18 mg g−1. Theoretical adsorption isotherms indicated a capacity of 63 mg g−1, which aligned well with the Langmuir model. To predict adsorption outcomes, machine learning models were applied, with multiple linear regression performing best. Optimization of decision trees and neural networks improved accuracy but risked overfitting. FT-IR, XRD, and ICP analyses indicated ion exchange as a significant mechanism of adsorption. In desorption studies, H2SO4 was the most effective agent, achieving 68.72% desorption efficiency. BMP exhibited optimal recyclability for up to four cycles before efficiency declined.
1 Introduction
Dyes find extensive applications across diverse industries, encompassing cosmetics, textiles, food, paints and varnish, leather, pharmaceuticals, and pulp and paper. As a result, they are significant contributors to industrial pollutants (Benkhaya et al., 2020; Chen et al., 2020; Hynes et al., 2020). The direct discharge of untreated wastewater containing dyes into natural water bodies has adverse effects on aquatic ecosystems, endagering aquatic organisms and posing risks to human health (Nasar and Mashkoor, 2019; Deering et al., 2020; Lau et al., 2014; Lellis et al., 2019).
Finding effective methods for removing dyes from wastewater is of utmost importance. Among common techniques employed for the removal of dyes from industrial effluents include surfactants (Shah et al., 2022; Shah et al., 2021), coagulation/flocculation (Raj et al., 2023), electrocoagulation (Nippatla and Philip, 2019), filtration (Suresh et al., 2023), adsorption (Agarwala and Mulky, 2023), ion-exchange (Barman et al., 2023), advanced oxidation processes (AOPs) (Zahrani and Ayati, 2020; Prado et al., 2022), activated sludge processes (Meerbergen et al., 2017), sequencing batch reactors (Lawal et al., 2023), membrane bioreactors (Mojiri et al., 2023), moving bed biofilm reactors (Maurya et al., 2023), and constructed wetlands (Jayalakshmi et al., 2023).
Among the afore-mentioned techniques, adsorption stands out as a crucial and valuable method for decontamination. It is recognized for its effectiveness, afordability, lack of sludge generation, high removal efficiency and/or selectivity, mechanical stability, and recyclability (Xiong et al., 2018; Tong et al., 2019; Zhou S. et al., 2019; Dutta et al., 2020), Additionally, in certain instances, the dye can be recovered without altering its chemical identity, as adsorption is a non-destructive process (da Fontoura et al., 2017; Toor and Jin, 2012; Azari et al., 2020; Guilhen et al., 2022).
Methylene blue (MB) is a widely recognized cationic dye belonging to the primary thiazine class, with a chemical composition expressed by the molecular formula C16H18N3ClS (Khan et al., 2022). This dye finds many applications across industries, such as textiles, pharmaceuticals, paper production, dyeing, printing, paints, medicine, and food (Koyuncu and Kul, 2020; Mijinyawa, Durga, and Mishra, 2019; Parakala et al., 2019; Balarak et al., 2020). Above certain concentration, MB dye is harmful to human health due to its considerable toxicity (Cheng et al., 2020). Human health and the environment are affected by MB for its toxicity, carcinogenicity, and non-biodegradability (Contreras et al., 2019; Sun et al., 2019; Guilhen et al., 2022), being also highly water-soluble. The ocurrence of MB in water bodies, even at minute concentrations, may reduce the transmission of sunlight, which, in turn, diminishes oxygen solubility and, consequently, disrupts the photosynthetic processes of aquatic organisms (Zhou Y. et al., 2019; Lawagon and Amon, 2019; Ahmad Zaini and Sudi, 2018; Kosswattaarachchi and Cook, 2018).
In this context, various types of biosorbents, including yeast (de Araujo et al., 2020; Chen and Wang, 2016; Yu et al., 2017), algae (de Araujo et al., 2022; Vieira et al., 2019), alginate (de Araujo et al., 2020; Yu et al., 2017), and agricultural crop residues (Sah et al., 2022), such as sugarcane bagasse ash and husks (de Padua Ferreira et al., 2020), can be used as low-grade biosorbents (Sfair, 2021). The application of bone meal powder (BMP) for treating aqueous solutions containing MB has not been thoroughly studied considering an experimental design approach. It is an inexpensive, abundant and natural material with considerable adsorptive capacity, being obtained from industrial meat processing residues and consists of 65%–70% inorganic matter, mainly hydroxyapatite (Ca10(PO4)6(OH)2) (Chojnacka, 2005; Mattar et al., 2014; Watanabe et al., 2021).
The aim of this study was to assess BMP’s effectiveness as an adsorbent material in eliminating MB from water matrices. Although BMP has demonstrated potential in other applications, its use as an adsorbent for water purification remains understudied, particularly for the removal of synthetic dyes like MB. Existing studies have predominantly focused on more conventional or modified adsorbents, thereby leaving a gap in understanding the capabilities of BMP as a cost-effective and sustainable alternative. In order to achieve this, biosorption treatment using BMP was applied to synthetic aqueous solutions containing MB, under various experimental conditions. An experimental factorial design was employed to identify optimal conditions by altering key parameters such as the initial concentration of MB, pH of the solution, and BMP biosorbent dosage. Additionally, aspects such as equilibrium time and kinetics, equilibrium adsorption isotherms, were explored to offer a comprehensive analysis (Ali Ahmed et al., 2024; Jain et al., 2022; Al-Asadi et al., 2023; Holliday et al., 2024; Farch et al., 2023; Mussa et al., 2023). By investigating these factors, this research provides valuable insights into BMP’s potential as a promising adsorbent for the removal of MB from water solutions.
2 Materials and methods
2.1 Biosorbent
The bone meal (manufactured by Fênix - Indústria e Comércio de Fertilizantes LTDA-ME, located in Cidade Cedral, Brazil) was ground and sieved, resulting in particle sizes ranging from 0.297 to 0.125 mm. Subsequently, the samples were stored in sealed polyethylene bottles, properly labeled, within the laboratory for future use.
2.2 Synthetic solutions
The solutions were formulated by employing analytical grade reagents and distilled water. The MB solutions utilized in the adsorption assays were derived from the respective stock solutions of the MB dye (C.I.52015, Merck, Darmstadt, Germany; Composition: Dye, ≥82% spectrophotometric assay).
2.3 Adsorption essays
The experiments were conducted using a batch approach, in which predetermined concentrations of MB were brought into contact with the biosorbent. Erlenmeyer flasks containing MB solutions and the biosorbent were placed in a BT 400 orbital shaking incubator (Biothec, Piracicaba, Brazil) and shaken at 130 rpm, maintaining a controlled temperature of 25°C ± 2°C. Appropriate amounts of BMP were suspended in 50 mL of the MB-containing solution, and the contact time was set at 24 h. After shaking, the biosorbent was separated from the solution by centrifugation at 6,000 rpm for 15 min. Initial exploratory experiments were carried out with an adsorbent mass/solution (M) of 2 g L⁻1, initial MB concentration ([MB]0) of 50 mg L⁻1, and pH 8. Posteriorly, a factorial experimental design was implemented to further investigate M, [MB]0, and pH. Using the most appropriate experimental conditions, provided by the experimental design, the equilibrium time and adsorption isotherms were evaluated through kinetic and isotherm modeling. Equilibrium time was evaluated by varying time within 0–24 h using pre-determined time intervals, whereas the isothermal study involved varying the initial MB concentrations from 5 to 1,500 mg L⁻1. All experiments were performed in triplicate.
The quantitative determination of MB was performed employing a Pharmacia Biotech Ultrospec 3000 UV UV-VIS spectrophotometer (Uppsala, Sweden). The absorption spectrum was obtained by measuring the absorbance at λmax = 668 nm, representing the peak absorption wavelength of MB detected by UV-VIS spectroscopy. The MB calibration curve was obtained using solutions of different concentrations of MB, in the range of 0–10 mg L⁻1 (five points: 0/1/2.5/5 and 10 mg L⁻1; Supplementary Figure S1). The equations for computing the adsorption capacity (qt or qexp) (Equation 1) and the removal percentage (R (%)) (Equation 2) are as follows:
where q is the adsorption capacity of MB (mg g⁻1), C0 is the initial concentration of MB in solution (mg L⁻1), Ct is the equilibrium concentration in solution (mg L⁻1) at a given time t, V is the volume of the solution (L) and M is the mass of the biosorbent (g).
where R is the extraction efficiency or percentage retention, C0 (mg L⁻1) is the initial concentration of MB and Ct (mg L⁻1) represents the concentration of MB at time t.
The equations related to the equilibrium kinetics, error functions with their deviation estimates, and isotherm models are presented in the Tables 1, 2 and in Supplementary Table S1.
2.4 Characterization methods
Nitrogen adsorption/desorption isotherms were utilized to analyze the Brunauer–Emmett–Teller (BET) surface area (Quanta Chrome NOVA, ACIL and Weber, Brazil). The total phosphorous concentration in the aqueous solutions before and after adsorption studies was determined using a Spectro ARCOS (Spectro Analytical Instruments, Germany) inductively coupled plasma optical emission spectrometer (ICP OES) with a linear calibration curve ranging from 0 to 1.25 mg L⁻1 at 177.495 nm (R2 = 0.9999).
The biosorbents were subjected to imaging analysis using a Hitachi TM4000Plus II tabletop Scanning Electron Microscopy (SEM) manufactured in Tokyo, Japan. In order Energy-dispersive X-ray spectroscopy (EDS) data were collected alongside SEM imaging to gather detailed information about the elemental composition of the biosorbent. The EDS measurements were conducted using a tungsten source and acceleration voltages of 5 and 15 kV, with an electron beam resolution of 30 nm. SEM images were acquired at magnifications ranging from 500 to 1,500 times. With micrographs of the BMP biosorbent material captured using particles ranging in size from 0.125 mm to 0.297 mm.
Fourier Transform InfraRed (FT-IR) spectroscopy was carried out using a Nicolet 6,700 FT-IR spectrometer (Thermo Fisher, United States) equipped with a liquid N2 cooled MCT detector and an ATR accessory (MIRacle with a Pike® diamond/ZnSe crystal plate). The X-ray diffraction (XRD) analysis was conducted on a Miniflex II X-ray diffractometer (Rigaku Co., Japan) using a CuKα radiation source with a wavelength of 1.5406Å. The analysis was carried out in 20°–90° and 2° min⁻1 as the scan rate.
The zeta potential of the BMP was measured using a Zetasizer NANO-Z instrument (Malvern Instruments Limited, United Kingdom) equipped with a helium-neon laser (633 nm), and a scattering angle of 173° for the measurement of the scattered light intensity. Particles were suspended in ultrapure water at a concentration of 1 mg mL⁻1 in an ultrasonic bath for 10 min. The experiment was carried out in triplicate at each point and the pH of the potentiometric titration was adjusted with 0.40 M HCl and 0.10 M NaOH solutions, with the pH evaluated from 1 to 9. The Smoluchowski approximation was the theoretical model used to calculate the value of the zeta potential (Hunter, 1988).
2.5 2k factorial experimental design and mathematical modeling
Factorial experimental design is a robust approach commonly used in modeling experiments for adsorption of dye (Salman et al., 2024; Khalfaoui et al., 2024). By systematically varying the factors of interest, factorial design allows for the efficient exploration of the entire experimental parameters, helping to identify significant factors and interactions affecting the response variable.
The equation for the adsorption experiments is n = 2k, where ‘n’ denotes the total number of experiments (24, performed in triplicate) and ‘k’ represents the number of variables (three in total). The experimental design chosen is the full factorial design, which was implemented in order to maximize MB removal. For this purpose, three parameters were selected: initial MB concentration ([MB]0), solution pH, and BMP biosorbent dosage (M). Table 3 lists the real and coded values considered for each test. The lower and upper bounds of each parameter were denoted by (−1) and (+1), respectively. Both the experimental design and statistical analyses studies were performed in RStudio (R Core Team, 2023). The most used R package was the “Process Improvement using Data”, named PID (Dunn, 2018). Analysis of Variance (ANOVA) was employed to facilitate comparison, with a confidence level of 95% utilized to assess both main and interaction effects concerning the percentage of MB removal and qexp.
Equation 3 was used to calculate the coded values of the variables, where var is the selected variable.
The equations generated by applying the multiple linear model were submitted to the stepwise algorithm based on the Akaike Information Criterion (AIC) for a significance level of 0.05. Therefore, only the statistically significant variables and their interactions were included in the equations. The stepwise algorithm was used to automatically select the most relevant variables in the applied model, simplifying the interpretation of the resulting equations and ensuring an efficient and accurate analysis of the relationships between the factors studied.
In the mathematical modeling study, Python code was employed to evaluate various machine learning (ML) techniques for analyzing experimental data, similarly to previous studies (Kumari et al., 2023a; Kumari et al. 2024; Kumari et al. 2023b; Kooh et al., 2022; Musa et al., 2024; Aghilesh et al., 2023; Mijwel et al., 2023). Initially, linear regression, decision tree (DT) regression, random forest (RF) regression, support vector machine (SVM), and neural network (NN) models were applied to predict the adsorption capacity based on experimental factors A, B, and C. Each model underwent training and evaluation for mean absolute error (MAE), with a grid search approach used to optimize hyperparameters for the SVM model. Further analysis involved incorporating interactions between variables A, B, and C, followed by modeling using the same ML techniques. Lastly, the study explored NN models with different configurations using grid search. The packages used were “pandas,” “numpy,” “matplotlib,” “sklearn,” and “seaborn.”
2.6 Desorption and regeneration experiments
Three different chemical reagents, including hydrochloric acid (HCl 37%, Supelco), sulfuric acid (H2SO4 98%, Synth) and sodium hydroxide (NaOH 99%, Isofar) were tested as eluents. A concentration of 1% (w/v for NaOH; v/v for HCl and H2SO4) of each eluent was used in the desorption experiments, which were carried out in 125 mL capped Erlenmeyer flasks, each containing 50 mL solution of the eluent solution. For these studies, BMP was loaded with MB at the concentration at which the isotherm reached its maximum point (300 mg L⁻1). This material was placed in contact with the desorption medium under constant agitation at 130 rpm for 1 h. Analyses were carried out three times at intervals of 15, 30, and 60 min. After selecting the best desorption medium, 1% and 3% H2SO4 were used for the recycling experiments, in which BMP was used again to remove MB in aqueous solution ([MB]0 = 100 mg L⁻1). The adsorbent was washed with distilled water after each experiment. The loss of efficiency was recorded for each cycle. The study of the cycles was completed when the % removal decreased by half, reaching its maximum reuse capacity.
2.7 Effect of coexisting ions
Four common coexisting ions: PO43⁻, CO32⁻, Cl−, and K+ (Cco-existing ions = 10 mg L⁻1) were added into the aqueous solutions with MB ([MB]0 = 100 mg L⁻1) to investigate their effect on the adsorption performance.
3 Results and discussion
3.1 Characterization of bone meal powder
BMP has a BET surface area of 48 m2 g⁻1 and showed no significant changes after the adsorption process. Compared to other bone materials, this value is significantly higher than that of Bali cow bone hydrochar powder, which presented 15 m2 g⁻1, and was used for the adsorption of methyl red from aqueous solution (Neolaka et al., 2023). Nevertheless, CO2 physically activated bone chars have been employed for dye adsorption and presented high BET surface area values of 137 m2 g⁻1 (Bedin et al., 2017).
Micrographs of BMP are presented in Figure 1A, illustrating its irregular surface morphology. For instance, SEM imaging of comparable biosorbents such as hydroxyapatite and tricalcium phosphate/chitosan, also showed irregular surface morphology, with clusters and agglomerates, surface roughness and intricate structures (Fijoł et al., 2021; Viswanathan et al., 2019). These observations strengthen the validity of the micrographs obtained for BMP, indicating that its irregular surface is a common feature among comparable biosorbent materials. Furthermore, as shown in Figure 1A, a measurement of the particles’ sizes was done, indicating sizes between 54.4 μm and 129 μm, which agree with (Deydier et al., 2005) who found particles between 50 and 245 μm by laser diffraction analysis. Figures 1B, C shows BMP after in contact with MB. After this contact, the BMP was larger in size, although deagglomerated, showing a broken structure in thin sheets, with spheres on its surface and homogeneously scattered, with a clear presence of pores, indicating a strong change in its structure after contact with the dye.
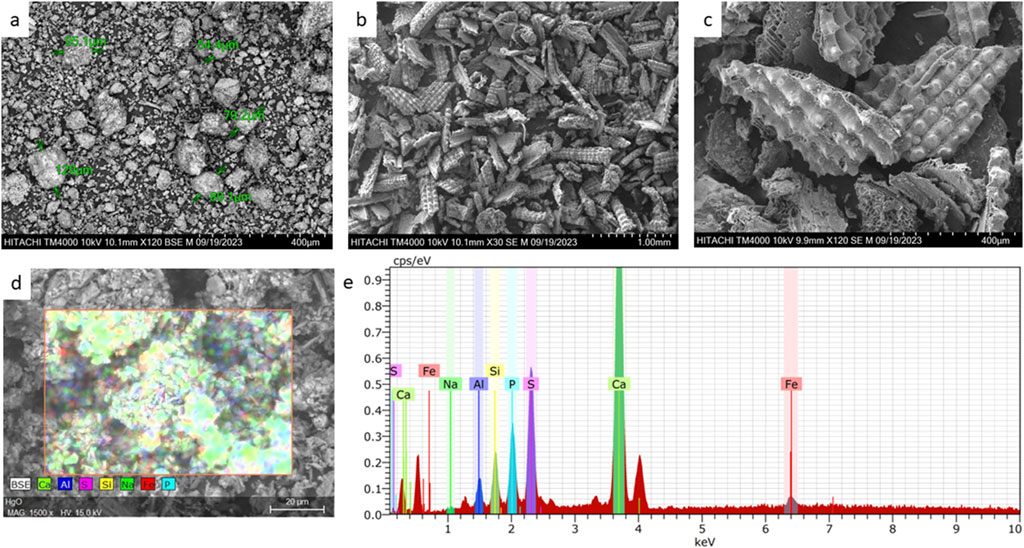
Figure 1. Scanning Electron Microscopy (SEM) Imaging and Energy Dispersive X-ray Spectroscopy (EDS) analysis of bone meal. (A) Micrograph of raw bone meal at 120× magnification with powder size measurement; (B) micrograph of bone meal after contact with MB ([MB]0 = 100 mg L−1, adsorbent dosage = 1 g L−1, time of contact = 1 h, pH = 11) at 30× magnification; (C) micrograph of bone meal after contact with MB at ×120 magnification; (D) raw bone meal micrograph with EDS scanning; (E) EDS analysis of raw bone meal.
Additionally, EDS analysis was conducted on the raw material, revealing the presence of calcium, phosphorus, and sulfur, as demonstrated in Figures 1D, E. EDS analyses of analogous raw materials have consistently detected the presence of calcium and phosphorus (Biedrzycka et al., 2021; Viswanathan et al., 2019), similar to what was observed in the analysis of BMP. This shared elemental composition across different biosorbents suggests a common chemical composition and potentially similar properties.
Phosphate constitutes a vital element within hydroxyapatite (Ca10(PO4)6(OH)2), a primary component of BMP. This is evident from the mid-IR spectrum of BMP (Figure 2), showcasing pronounced absorption bands at 1,000–1,100 cm⁻1 associated with the antisymmetric stretching bands of apatite PO43⁻ groups (Paschalis et al., 2011; Vassalo et al., 2016). Additionally, the peak at 668 cm⁻1 corresponds to the out-of-plane bending vibrations of the PO43⁻- ions (Vassalo et al., 2016).
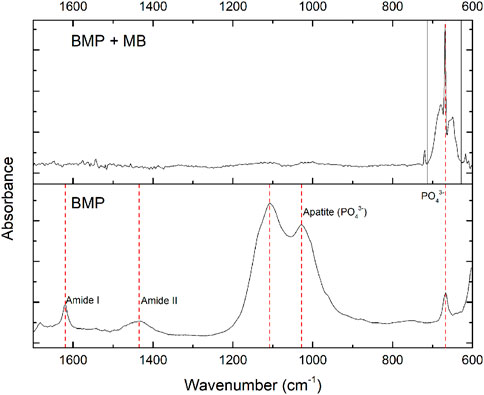
Figure 2. Mid-IR spectra of the (bottom) bone meal powder and (top) bone meal powder with methylene blue ([MB]0 = 100 mg L−1, adsorbent dosage = 1 g L−1, time of contact = 1 h, pH = 11).
The observed peak at 1,620 cm⁻1 could be ascribed to two types of vibrations: in plane H-O-H bending (water) and carbonyl stretching (carbon dioxide) (Mendes et al., 2012; Kesmez, 2020). CO32⁻ peak is observed at 1,435 cm-1 and can be explained by carbonate substitution for hydroxyl and phosphate groups in hydroxyapatite (Sahana et al., 2013; Chappard et al., 2016). The mid-IR spectrum indicates deep involvement of PO43⁻ groups (650–680 cm⁻1) in MB adsorption and suggests that the interaction of MB with these groups may elevate the HPO42⁻ species in the solution. Quantifying the phosphate mass in the aqueous solution pre and post the adsorption process revealed noteworthy changes. Initially, the MB solutions exhibited a phosphate concentration of 0.073 ± 0.003 (mg L⁻1), surging to 0.569 ± 0.009 (mg L⁻1) post-mixture with BMP, marking an eightfold increase. This substantial increment strongly indicates a significant participation of the ion exchange mechanism in the MB adsorption by BMP. It is important to note that the concentration of phosphate released during the adsorption process is far below its presence in typical sewage effluents, which is commonly between 4 and 15 mg L⁻1 (Dantas et al., 2021). Therefore, the release of phosphate from BMP to the environment is within acceptable levels and does not pose a significant risk of secondary pollution.
XRD analysis was conducted to investigate any structural changes induced by the adsorption process. The diffractogram of the samples are shown in Figure 3. Crystalline phases found in the sample prior to adsorption were potassium hydrogen phosphate hydrate (PDF#26-0905), calcium phosphate (PDF#52-1538), hydroxyapatite (PDF#89-4405) and phosphorus oxide (PDF#870-0952). After dye adsorption, it can be seen that the crystalline structure has become amorphous.
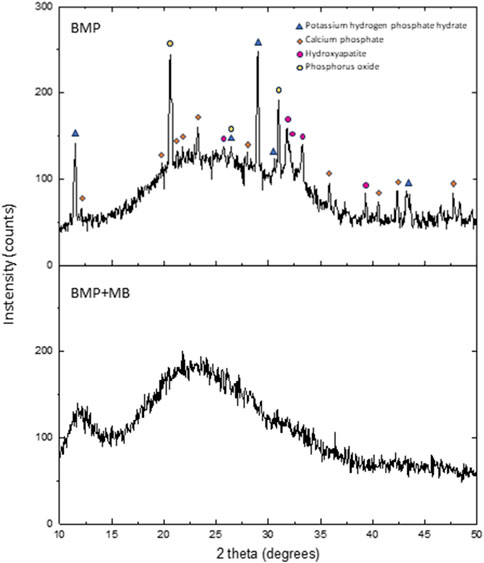
Figure 3. XRD of the BMP material before: BMP; after in contact with MB: BMP + MB. ([MB]0 = 100 mg L−1, adsorbent dosage = 1 g L−1, time of contact = 1 h, pH = 11).
The zeta potential study of the BMP as a function of pH was done and it is presented in Supplementary Figure S2. MB is cationic, indicating a higher affinity for the adsorbent when it the latter exhibits a negative zeta potential (Zhang et al., 2012; Ebadollahzadeh and Zabihi, 2020; Li et al., 2022), i.e. at pH values above the isoelectric point (IEP). Below the IEP (1.85), the BMP surface has a positive charge balance. As all pH values tested in this study were above the IEP, the affinity of MB for the BMP surface remained unaffected.
3.2 Experimental design
The exploratory results indicated a qexp of 7.5 ± 0.04 mg g⁻1, with a removal of 32% ± 0.002% (M = 2 g L⁻1; pH = 8; [MB]0 = 50 mg L⁻1). To search for higher qexp and removal rates, further experiments were conducted and a factorial experimental design was employed. The response (qexp) obtained for each experiment is shown in Table 4. The model equations represent the role of each variable process and estimates variations in the experimental range. Equations 4, 5 show the estimated model for qexp and for the removal percentage, respectively. The whole design matrix with the predicted data and the statistical parameters and their values are provided in the (Supplementary Tables S2, S3). The estimated values, ŷ1 and ŷ2, shown in Supplementary Table S2, represent the experimental data well, being consistent with R2 values (Supplementary Table S3) of 99.50% for y1 and 99.95% for y2.
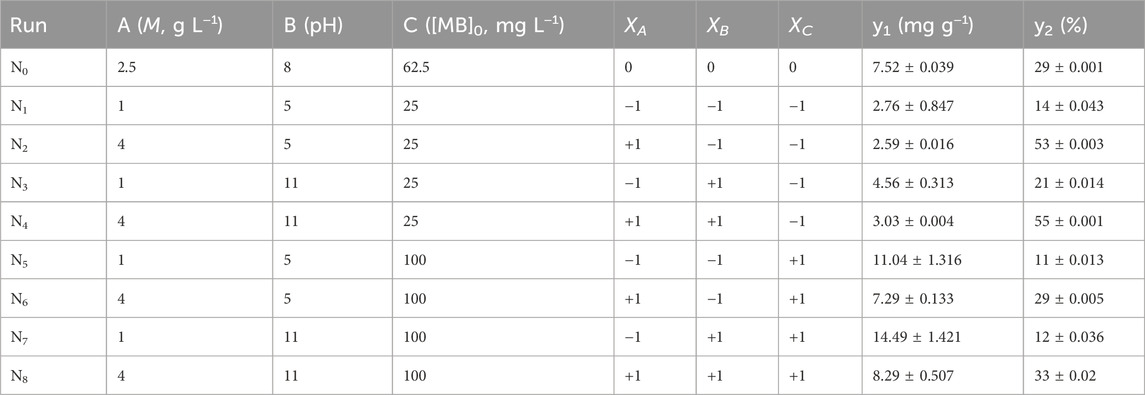
Table 4. Design matrix for MB removal by BMP. y1 and y2 are the adsorption capacity (mg g−1) and percentage removals, respectively.
3.3 Statistical analysis
The Pareto plot, depicted in Figure 4A, provides insights into the most influential factors affecting qexp. Notably, the variables C (initial MB concentration) and A (M, g L⁻1) exhibited the most significant effects, followed by the A:C interaction, with variable B (pH) and the A:B interaction having a comparatively lower impact. The analysis reveals that variable C plays a predominant role in the process, positively influencing qexp. Conversely, the effect of variable A negatively influences this response, ranking second in importance.
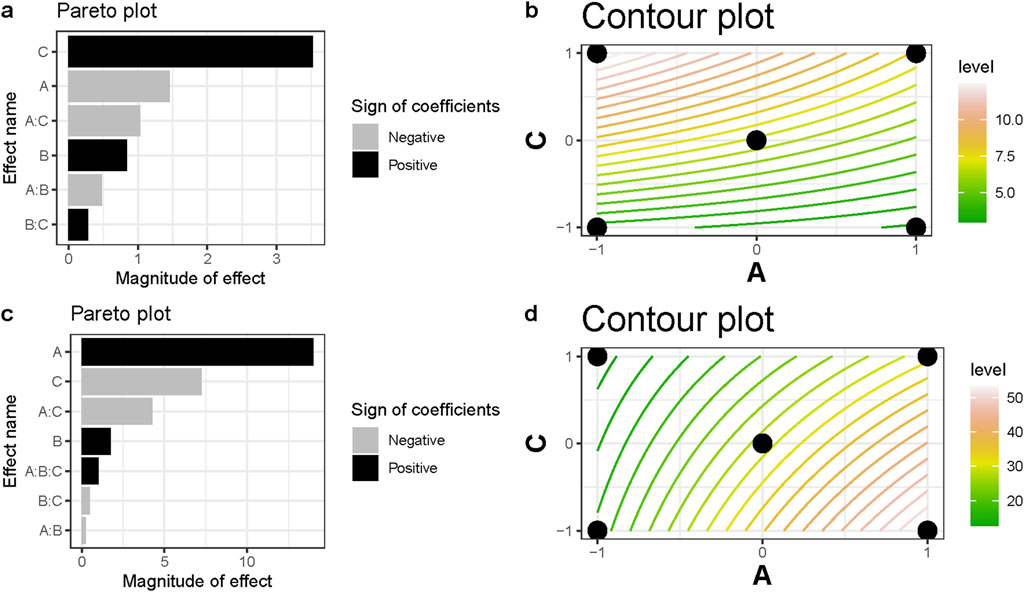
Figure 4. (A) Pareto plot from qexp,BMP on the removal of MB (most significant effects labeled); (B) Contour plot of the main interaction (A:C) regarding BMP adsorption capacity of MB. (C) Pareto plot from the MB removal percentage by BMP (most significant effects labeled); (D) Contour plot of the main interaction (A:C) regarding MB removal percentage by the BMP.
Figure 4B presents a contour plot that visually represents these effects while highlighting the relatively weak interaction between the terms. It is evident that increasing the initial MB concentration (variable C) and decreasing the adsorbent dosage (variable A) are key strategies for enhancing qexp values. However, the impact of variable B (pH) on performance appears to be statistically insignificant, potentially overshadowed by the more pronounced effect of variable C, which has a significantly higher magnitude.
The contour plot further supports this behavior, with higher values concentrated in the upper left corner of Figure 4B. The observed curvatures imply that the interactions between these variables play an important role in the adsorption process and must be considered to provide optimized treatment systems. According to this experimental range, design experiments with lower values of M (<1 g L⁻1) and higher values of [MB]0 (>75 mg L⁻1) are recommended to optimize the most relevant adsorption conditions. These findings emphasize the importance of careful consideration and manipulation of the initial MB concentration and adsorbent dosage to maximize qexp values and support scaling-up in an optimized manner. Further experimentation within the suggested parameter ranges can yield even more optimized conditions for the adsorption process.
When considering the removal percentages, the adsorbent dosage (variable A) emerged as the most influential factor (Figures 4C, D). However, unlike qexp values, an interesting contrast was observed: variable A exhibited a positive effect on MB removal. Following this, the initial MB concentration (variable C) was identified as the second most significant variable affecting MB removal. Intriguingly, its effect on the response was also opposite to that observed for qexp values, with a negative impact.
These distinct responses underscore the importance of carefully selecting appropriate response variables when making informed decisions regarding the system conditions. Depending on the desired outcome (e.g., maximizing qexp values or achieving high removal percentages), different variables may exert opposing effects. It becomes crucial to consider the specific objectives and desired outcomes of the system in order to make optimal choices.
Understanding the varying effects of different variables on different response variables enables informed decision-making, allowing for the selection of system conditions that align with the desired goals. By carefully considering and selecting the appropriate response variables, researchers and practitioners can navigate these complexities and make well-informed choices to achieve the desired outcomes in the system.
3.4 Machine learning study
While linear regression modeling yielded high correlation values and offered valuable insights into MB adsorption by BMP, the application of additional mathematical tools is crucial to enhance our understanding of the process. Indeed, multiple linear regression comes with certain limitations, including multicollinearity when independent variables are highly correlated, and the risk of overfitting or overly complex models when the number of independent factors is large relative to the amount of observations. In addressing these challenges, several measures were implemented in this study, such as excluding non-statistically significant factors and ensuring an appropriate ratio of independent variables to the number of observations. However, to further address these issues and offer alternative mathematical formulations, the incorporation of regularization techniques such as Ridge Regression and Lasso Regression is essential. These methods penalize unnecessary factors, thus helping to prevent overfitting and simplifying model complexity. Figure 5 presents a comparison between these linear models, both considering and not considering interactions between independent variables, respectively.
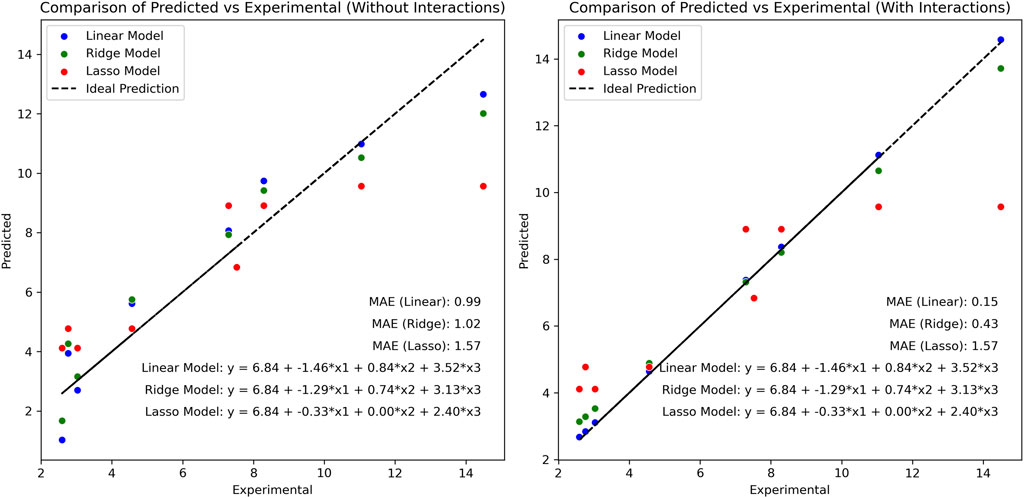
Figure 5. Comparison between linear, Ridge and Lasso models. The stepwise was not employed for these comparisons. (left side) the effects of the interactions were not included in the evaluation; (right side) the effect of the pair and triple interactions were considered into the models but are not given in the graph equations.
Clearly, multiple linear regression yielded the most accurate fittings, with Mean Absolute Errors (MAEs) of 0.99 and 0.15, depending on whether the interaction of independent variables was included. Without interactions, Ridge outperformed the linear model for lower values but exhibited decreased performance as values exceeded approximately seven. The Lasso model appeared to overly penalize linear equations, resulting in significantly poorer performance for both scenarios and indicating insensitivity to the addition of interactions. However, when interactions were considered, the linear model demonstrated exceptional fitting, achieving a MAE of 0.15, with predicted values closely aligned with experimental ones. For extrapolation beyond the study’s data range, the Ridge model could prove advantageous, as it exhibited sensitivity to added interaction values without achieving near-perfect fitting.
Alternative mathematical approaches offer intriguing methods for data prediction and can be particularly useful for guiding further experimentation. For instance, the DT model provides a systematic decision-making process based on parameter selection, facilitating the identification of optimal values for the dependent variable. As depicted in Supplementary Figure S3, this figure highlights that to enhance adsorption capacity, one should initiate with a MB concentration exceeding 43.75 mg L⁻1, followed by a lower adsorbent dosage of less than 1.75 g L⁻1, and subsequently, a pH level higher than 8. By adhering to this sequence, adsorption capacity can significantly increase from 3.23 to 14.49 mg g⁻1.
The linear regression model remains the most effective, outperforming DT, RF, SVM, and NN (Figure 6). Notably, augmenting linear regression with interactions resulted in superior accuracy, surpassing even high-performance models like NN.
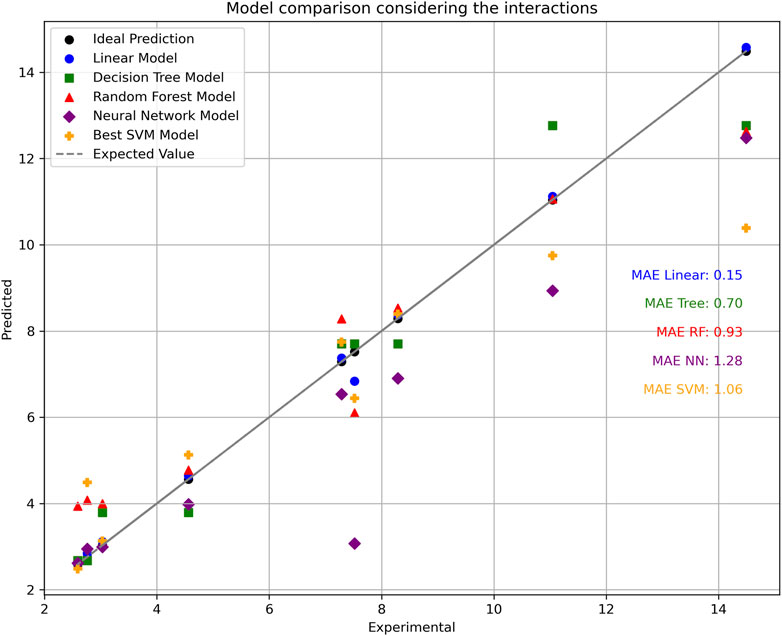
Figure 6. Predictions by different ML models and their MAE calculations. The SVM model included was selected by working with different values for the hyperparameters (see Supplementary Figure S4 for the grid search configuration study).
However, these models operate with distinct parameter values, including hyperparameters. Consequently, optimizing these parameters may yield a perfect yet unrealistic fit. For instance, when varying the maximum depth parameter for DT, perfect fitting was achieved by increasing the value from one to two (see SI, Supplementary Figure S5). Conversely, with NN, significantly improved predictions were observed by adjusting hidden layer sizes, culminating in a MAE of 0.47 (see SI, Supplementary Figures S6–S8). However, achieving a perfect fit may require incorporating interactions between independent variables, while suboptimal parameter values may lead to poor fitting.
3.5 Effect of contact time
The adsorption of MB over time under the specified conditions (M = 1 g L⁻1; pH = 11; [MB]0 = 100 mg L⁻1) is presented in Figure 7. The data reveals that the adsorption is rapid and that most MB is adsorbed within the initial 30 min. Subsequently, the rate of adsorption slows as equilibrium is approached, likely due to the gradual occupancy of vacant sites initially available.
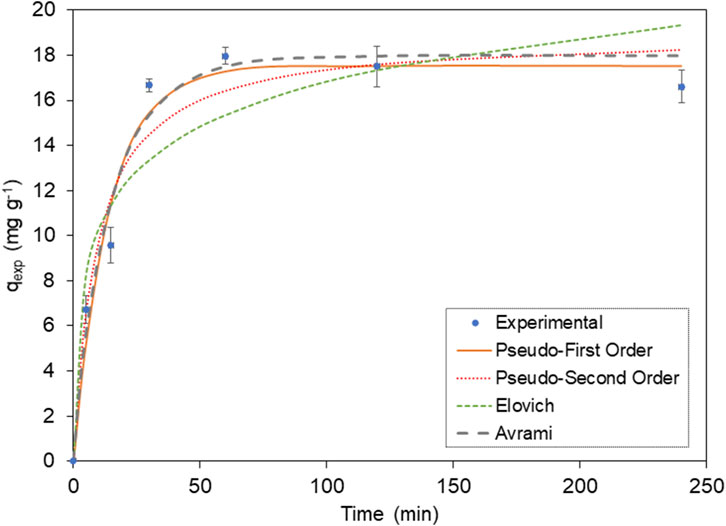
Figure 7. Kinetic models of MB adsorption onto BMP. For the equations, see Table 1.
To gain further insights into the adsorption process, we conducted kinetic studies utilizing six distinct models: Avrami, Elovich, pseudo-first order (PFO), and pseudo-second order (PSO) (refer to Table 1, for the equations). These kinetic models offer valuable insights into the kinetic parameters and the underlying mechanism of MB adsorption onto BMP. The parameter values are presented in Table 5, along with the corresponding residual standard errors, enabling the assessment of the models’ accuracy. Additionally, the Relative Mean Square Error (RMSE) was calculated to provide a comprehensive evaluation of the model’s performance.
The calculated values of qexp for PFO and PSO exhibited slightly discrepancies, with PFO being closer to the experimentally observed value (qexp). Furthermore, the RMSE was lower for the PFO model, 1.11, indicating a superior fit compared to the PSO model, which yielded an RMSE of 1.44. In this manner, the experimental data aligns better with the non-linear PFO model, suggesting that adsorption follows a pseudo-first-order chemical reaction and that the rate of the adsorption can be elucidated by the first-order reaction rate equation (Yang and Al-Duri, 2005). In simpler terms, PFO kinetics imply that the adsorption governs the rate-limiting step in the process (Ahmad et al., 2005).
The data also exhibited a good fit with the Avrami fractional kinetic equation, concurring with the PFO model, since it displayed lower error function values than PSO and Elovich for the studied MB concentration (Passos et al., 2008). Upon analyzing the kinetic parameter values presented in Table 5, it is evident that the values of KAV are much more suitable for evaluating the kinetics of the process than the pseudo-second-order rate constant (k2). This is due to the fact that the Avrami constant values are independent of the initial concentration of the adsorbate, having min-1 as their units. Conversely, the values of k2 are highly dependent on initial pollutant concentrations, given that their units are g mg−1 min−1 (Oladoja, 2016).
3.6 Isotherm studies: Langmuir and Freundlich models
Langmuir and Freundlich isotherms were employed and fitted to the experimental data (Figure 8). The parameters obtained by linear and non-linear regression for each isotherm model are presented in Table 6. At equilibrium, our findings demonstrate an augmentation in the amount of MB adsorbed. Notably, with an increase in the initial concentration of MB, there is a corresponding decrease in the efficiency of MB removal. The Langmuir isotherm, which assumes monolayer adsorption on a surface with a finite number of identical sites, provided the highest correlation coefficient (R2 = 0.935), suggesting that the adsorption process is predominantly occurring on homogeneous sites on the BMP surface. The calculated maximum adsorption capacity (qmax) of 63 mg g⁻1 aligns well with the experimental data, indicating that BMP has a substantial number of active sites for MB adsorption.
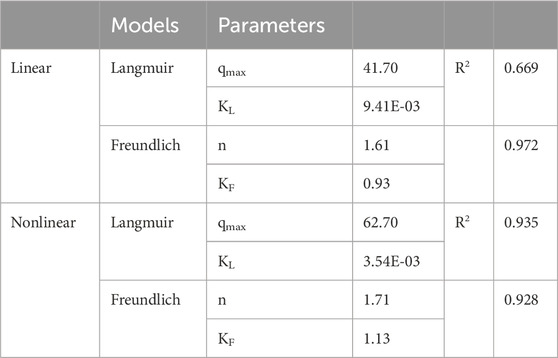
Table 6. Parameters calculated by using the Langmuir and Freundlich adsorption isotherm models for MB adsorption on BMP, along with the corresponding correlation coefficients obtained for each model.
In contrast, the Freundlich isotherm, which is an empirical model describing adsorption on heterogeneous surfaces, also demonstrated a satisfactory fit with a slightly lower R2 value of 0.928. This indicates that while the BMP surface exhibits some degree of heterogeneity, the prevalence of homogeneous adsorption sites is more pronounced, as evidenced by the superior fit of the Langmuir model. The Freundlich constant (KF = 0.93), which is related to adsorption capacity, and the heterogeneity factor (n = 1.71) were also consistent with moderate adsorption intensity, reflecting the complex nature of the adsorption process.
The higher correlation observed with the Langmuir model in comparison to the Freundlich model suggests that the adsorption of MB on BMP may occur primarily on uniform sites until saturation is reached. Once saturation is achieved, multilayer adsorption (as suggested by the Freundlich model) could potentially take place within the pores or at more heterogeneous sites.
In this study, a brief investigation was conducted into the qpred values of various adsorbent materials for MB removal. To facilitate a thorough comparison, performance of BMP in relation to other commonly studied adsorbents was carefully evaluated, including olive pomace, charcoal, silicas, and several zeolites. The detailed results are accessible in Supplementary Table S4 of the Supplementary Information, offering significant insights into the adsorption properties of these materials.
The findings from this study unequivocally demonstrate that BMP exhibits superior qpred values for MB when compared to olive pomace, charcoal, silicas, and the majority of the investigated zeolites. This underscores the remarkable potential of BMP as a proficient adsorbent for removing MB in water and wastewater treatment applications. However, it is important to note that certain modified adsorbents, such as the zwitterion composite chitosan-epichlorohydrin/zeolite, as well as peels derived from banana, potato, and jackfruit, displayed even higher qpred values for MB. These modified materials exhibited exceptional performance, suggesting promising avenues for further research and development with modified BMP in the field of pollutant removal.
3.7 Desorption and regeneration
As shown in Figure 9A, at a time span of 1 h, a maximum of 68.72% and 63.14% of desorption were achieved using H2SO4 1% and HCl 1% as desorption solutions, respectively, while in the alkaline media (NaOH 1%), a 12.91% desorption efficiency was observed.
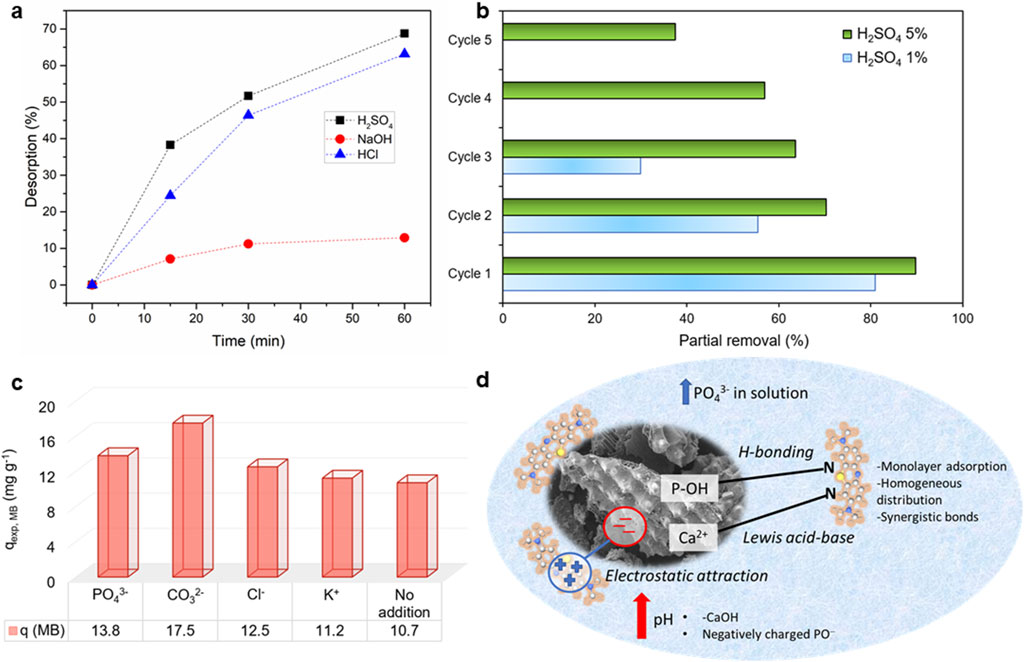
Figure 9. (A) Desorption efficiencies of MB under various desorption conditions, (black square) 1% H2SO4; (red circle) 1% NaOH, (blue triangle) 1% HCl; (B) Regeneration cycles of BMP with H2SO4 as the desorption agent; (C) Effect of co-existing ions in the aqueous solutions; (D) Proposed mechanism of the adsorption process between bone meal powder and methylene blue. The material used was obtained after the experiments with the following conditions: [MB]0 = 100 mg L−1, adsorbent dosage = 1 g L−1, time of contact = 1 h, pH = 11, Cco-existing ions = 10 mg L−1.
This difference suggests that the desorption efficiency of adsorbed MB is strongly pH-sensitive and can be attributed to the fact that, BMP was prone to protonation in acidic media and, hence, not a favorable surface for MB adsorption. The recovery of BMP was evaluated and is critical for reducing the cost of MB removal process, enabling the potential reuse of the adsorbent.
The MB adsorption-desorption cycles are shown in Figure 9B. H2SO4 was chosen as the desorption agent due to its superior efficiency. Two concentrations were used to assess regeneration capacity (1% and 5%, v/v). H2SO4 5% exhibited superior recycling capability. The BMP regeneration efficiency decreased from 89.74% to 37.54% after five consecutive cycles, suggesting effective recycling (>50% removal efficiency) over four cycles. After the fourth cycle, the reduction in MB removal indicates that a significant number of chemical reactions have occurred on BMP’s surface, resulting in the collapse of its porous structure and decreased adsorption capacity during subsequent regeneration cycles. Conversely, using H2SO4 1% (v/v) resulted in considerably lower recycling, with BMP regeneration efficiency decreasing from 80.94% to 29.91% after three consecutive cycles, allowing for only two cycles of BMP regeneration.
With regard to desorption agents and their efficacy in recycling, direct comparisons with previous investigations have proven challenging. The current literature lacks comprehensive studies utilizing BMP and BMP-derived materials specifically for contaminant adsorption, creating a gap in the field. As highlighted by (Adewuyi, 2020), this is a common problem across different applications using biosorbents, highlighting the need to prioritize regeneration studies to thoroughly evaluate the value of these affordable biosorbents.
3.8 Effect of coexisting ions
Figure 9C shows that the presence of coexisting ions had minimal impact MB adsorption onto BMP when the MB concentration was 10 mg L⁻1. The adsorption capacities of MB after the addition of coexisting ions ranged from 11.2 to 17.5 mg g⁻1, higher than that observed in the absence of co-ions (10.7 mg g⁻1). These findings suggest that the process was not negatively affected by the presence of coexisting ions. However, there was an important increase in adsorption capacity, particularly when anions were added into the solution, which may be ascribed to enhanced dimerization reactions of MB in solution due to the forces: ion-dipole interactions, dipole-dipole interactions, and van der Waals forces among MB molecules (Lin et al., 2017; Kuang et al., 2020). Notably, the presence of PO43- had a more pronounced effect on MB adsorption by BMP.
3.9 Mechanisms of adsorption between bone meal powder and methylene blue
The adsorption of MB molecules onto BMP is primarily driven by a combination of electrostatic interactions, ion exchange, and surface chemistry. The BMP, with its BET surface area of 48 m2 g⁻1, provides a substantial and irregular surface morphology that facilitates the adsorption process. The interaction between the phosphate groups (PO43⁻) in BMP and the nitrogen atoms in the MB molecules is crucial, as depicted in Figure 9D. Additionally, these nitrogen atoms are likely to interact with Ca2+ ions present in hydroxyapatite through Lewis acid-base interactions, as suggested elsewhere (Sharma et al., 2021).
As the pH value increases, the surface of BMP becomes increasingly negatively charged due to the prevalence of neutral -CaOH groups and negatively charged PO- species. This results in a stronger electrostatic attraction between the negatively charged BMP surface and the positively charged groups of the MB dye. Furthermore, the release of phosphate ions during the adsorption process indicates that ion exchange is also a significant mechanism, whereby phosphate groups on BMP are replaced by MB molecules in solution. The observed structural changes in BMP after adsorption, such as increased porosity and the appearance of thin, broken sheets, provide further evidence that these interactions lead to a strong and efficient adsorption process.
4 Conclusion
This study demonstrated the effectiveness of BMP as an adsorbent for removing MB from aqueous solutions. Among the experimental conditions tested, N4 (M = 4 g L⁻1; pH = 11; [MB]0 = 25 mg L−1) achieved the highest removal efficiency (R = 55%). However, the highest adsorption capacity (qexp = 14.49 mg g⁻1) was observed under the N7 condition (M = 1 g L−1; pH = 11; [MB]0 = 100 mg L−1). Equilibrium was reached within 30 min, with a final qexp value of 18 mg g-1. The kinetic analysis revealed that the pseudo-first order model provided the best fit to the experimental data, yielding a qexp value of 17.51 mg g−1 and a rate constant (k1) of 0.0714 min−1. This indicates that the adsorption process is primarily governed by the rate of MB removal over time. The adsorption isotherms followed the Langmuir model, predicting a maximum adsorption capacity (qpred) of 63 mg g⁻1, suggesting monolayer adsorption on a homogeneous surface. Desorption studies indicated that the efficiency of MB desorption from BMP is highly pH-dependent, with the highest desorption (68.72%) occurring in acidic conditions using H2SO4 as the desorbing agent. This highlights the importance of pH in the regeneration process, where acidic conditions reduce BMP’s effectiveness for re-adsorption due to surface protonation. The findings of this research underscore the potential of BMP as a viable adsorbent for MB removal, particularly in the context of utilizing agricultural byproducts in wastewater treatment. The experimental data provide valuable insights into the adsorption kinetics, equilibrium behavior, and the impact of operational parameters, which can inform the development of practical applications for dye-containing wastewater treatment. Future work will focus on translating these theoretical results into scalable and efficient treatment processes for real-world wastewater applications.
Data availability statement
The original contributions presented in the study are included in the article/Supplementary Material, further inquiries can be directed to the corresponding author.
Author contributions
LA: Conceptualization, Data curation, Formal Analysis, Funding acquisition, Investigation, Methodology, Visualization, Writing–original draft, Writing–review and editing. GM: Data curation, Investigation, Writing–original draft. AC: Data curation, Investigation, Writing–original draft. JM: Resources, Supervision, Writing–review and editing. SG: Conceptualization, Formal Analysis, Funding acquisition, Methodology, Supervision, Writing–review and editing.
Funding
The author(s) declare that financial support was received for the research, authorship, and/or publication of this article. The authors acknowledge the fellowship awarded by the Brazilian National Council for Scientific and Technological Development to GFM. Additionally, we sincerely appreciate the financial support provided by the Brazilian Nuclear Energy Commission (CNEN).
Acknowledgments
We are also thankful for the collaboration of Dr. Rodrigo Fernando Brambilla de Souza (IPEN-CNEN/SP), Dr. Maciel Santos Luz (IPT-USP), M. Sc. Gilmar Alves de Almeida (IPT-USP), Dr. Edson Pereira Soares (IPEN-CNEN/SP), Dr. Rafael Henrique Lazzari Garcia (IPEN-CNEN/SP), and B.Sc. Deiwid Ferreira Gomes (IPEN/CNEN-SP) in the chemical characterization of the samples.
Conflict of interest
The authors declare that the research was conducted in the absence of any commercial or financial relationships that could be construed as a potential conflict of interest.
Publisher’s note
All claims expressed in this article are solely those of the authors and do not necessarily represent those of their affiliated organizations, or those of the publisher, the editors and the reviewers. Any product that may be evaluated in this article, or claim that may be made by its manufacturer, is not guaranteed or endorsed by the publisher.
Supplementary material
The Supplementary Material for this article can be found online at: https://www.frontiersin.org/articles/10.3389/fenvc.2024.1451051/full#supplementary-material
References
Adewuyi, A. (2020). Chemically modified biosorbents and their role in the removal of emerging pharmaceutical waste in the water system. Water 12 (6), 1551. doi:10.3390/w12061551
Agarwala, R., and Mulky, L. (2023). Adsorption of dyes from wastewater: a comprehensive review. ChemBioEng Rev. 10 (3), 326–335. doi:10.1002/cben.202200011
Aghilesh, K., Kumar, A., Agarwal, S., Garg, M. C., and Joshi, H. (2023). Use of artificial intelligence for optimizing biosorption of textile wastewater using agricultural waste. Environ. Technol. 44 (1), 22–34. doi:10.1080/09593330.2021.1961874
Ahmad, A., Sumathi, S., and Hameed, B. (2005). Adsorption of residue oil from palm oil mill effluent using powder and flake chitosan: equilibrium and kinetic studies. Water Res. 39 (12), 2483–2494. doi:10.1016/j.watres.2005.03.035
Ahmad Zaini, M. A., and Sudi, R. M. (2018). Valorization of human hair as methylene blue dye adsorbents. Green Process. Synthesis 7 (4), 344–352. doi:10.1515/gps-2017-0021
Al-Asadi, S. T., Al-Qaim, F. F., Al-Saedi, H. F. S., Deyab, I. F., Kamyab, H., and Chelliapan, S. (2023). Adsorption of methylene blue dye from aqueous solution using low-cost adsorbent: kinetic, isotherm adsorption, and thermodynamic studies. Environ. Monit. Assess. 195 (6), 676. doi:10.1007/s10661-023-11334-2
Ali Ahmed, A., Zhour, H., Berredjem, Y., Souaad, H., and Djellabi, R. (2024). Valorization of silybum marianum seed shells waste as biosorbent for basic fuchsin dye removal from water: kinetics, isotherms, and thermodynamic studies. Desalination Water Treat. 317 (January), 100278. doi:10.1016/j.dwt.2024.100278
Azari, A., Nabizadeh, R., Nasseri, S., Mahvi, A. H., and Ali, R. M. (2020). Comprehensive systematic review and meta-analysis of dyes adsorption by carbon-based adsorbent materials: classification and analysis of last decade studies. Chemosphere 250, 126238. doi:10.1016/j.chemosphere.2020.126238
Balarak, D., Bazzi, M., Shehu, Z., and Chandrika, K. (2020). Application of surfactant-modified bentonite for methylene blue adsorption from aqueous solution. Orient. J. Chem. 36 (02), 293–299. doi:10.13005/ojc/360212
Barman, M. K., Bhattarai, A., and Saha, B. (2023). Applications of ion exchange resins in environmental remediation. Vietnam J. Chem. 61 (5), 533–550. doi:10.1002/vjch.202300027
Bedin, K. C., Stéfani, P. D. A., Leandro, P. K. T., Cazetta, A. L., and Almeida, V. C. (2017). Bone char prepared by CO2 atmosphere: preparation optimization and adsorption studies of remazol brilliant blue R. J. Clean. Prod. 161 (September), 288–298. doi:10.1016/j.jclepro.2017.05.093
Benkhaya, S., Souad, M., Ahmed, El H., M’rabet, S., and Ahmed, El H. (2020). A review on classifications, recent synthesis and applications of textile dyes. Inorg. Chem. Commun. 115 (May), 107891. doi:10.1016/j.inoche.2020.107891
Biedrzycka, A., Skwarek, E., and Hanna, U. M. (2021). Hydroxyapatite with magnetic Core: synthesis methods, properties, adsorption and medical applications. Adv. Colloid Interface Sci. 291 (May), 102401. doi:10.1016/j.cis.2021.102401
Chappard, C., André, G., Daudon, M., and Bazin, D. (2016). Analysis of hydroxyapatite crystallites in subchondral bone by fourier Transform infrared spectroscopy and powder neutron diffraction methods. Comptes Rendus Chim. 19 (11–12), 1625–1630. doi:10.1016/j.crci.2015.03.015
Chen, C., and Wang, J. (2016). Uranium removal by novel graphene oxide-immobilized Saccharomyces cerevisiae gel beads. J. Environ. Radioact. 162–163 (October), 134–145. doi:10.1016/j.jenvrad.2016.05.012
Chen, J., Xiong, Y., Duan, M., Xiang, L., Li, J., Fang, S., et al. (2020). Insight into the synergistic effect of adsorption–photocatalysis for the removal of organic dye pollutants by Cr-doped ZnO. Langmuir 36 (2), 520–533. doi:10.1021/acs.langmuir.9b02879
Cheng, J., Zhan, C., Wu, J., Cui, Z., Si, J., Wang, Q., et al. (2020). Highly efficient removal of methylene blue dye from an aqueous solution using cellulose acetate nanofibrous membranes modified by polydopamine. ACS Omega 5 (10), 5389–5400. doi:10.1021/acsomega.9b04425
Chojnacka, K. (2005). Equilibrium and kinetic modelling of chromium(III) sorption by animal bones. Chemosphere 59 (3), 315–320. doi:10.1016/j.chemosphere.2004.10.052
Contreras, M., Grande-Tovar, C. D., Vallejo, W., and Chaves-López, C. (2019). Bio-removal of methylene blue from aqueous solution by galactomyces geotrichum KL20A. Water 11 (2), 282. doi:10.3390/w11020282
da Fontoura, J. T., Sebastião Rolim, G., Mella, B., Farenzena, M., and Gutterres, M. (2017). Defatted microalgal biomass as biosorbent for the removal of acid blue 161 dye from tannery effluent. J. Environ. Chem. Eng. 5 (5), 5076–5084. doi:10.1016/j.jece.2017.09.051
Dantas, M. S., Barroso, G. R., and Oliveira, S. C. (2021). Performance of sewage treatment plants and impact of effluent discharge on receiving water quality within an urbanized area. Environ. Monit. Assess. 193 (5), 289. doi:10.1007/s10661-021-09075-1
de Araujo, L. G., de Borba, T. R., de Pádua Ferreira, R. V., Canevesi, R. L. S., da Silva, E. A., Dellamano, J. C., et al. (2020). Use of calcium alginate beads and Saccharomyces cerevisiae for biosorption of 241Am. J. Environ. Radioact. 223–224 (November), 106399. doi:10.1016/j.jenvrad.2020.106399
de Araujo, L. G., Vieira, L. C., Canevesi, R. L. S., da Silva, E. A., Watanabe, T., de Padua Ferreira, R. V., et al. (2022). Biosorption of uranium from aqueous solutions by Azolla sp. and limnobium laevigatum. Environ. Sci. Pollut. Res. 29 (30), 45221–45229. doi:10.1007/s11356-022-19128-8
Deering, K., Spiegel, E., Quaisser, C., Nowak, D., Rakete, S., Garí, M., et al. (2020). Exposure assessment of toxic metals and organochlorine pesticides among employees of a natural history museum. Environ. Res. 184 (May), 109271. doi:10.1016/j.envres.2020.109271
de Padua Ferreira, R. V., de Araujo, L. G., Canevesi, R. L. S., da Silva, E. A., Ferreira, E. G. A., Palmieri, M. C., et al. (2020). The use of rice and coffee husks for biosorption of U (total), 241Am, and 137Cs in radioactive liquid organic waste. Environ. Sci. Pollut. Res. 27 (29), 36651–36663. doi:10.1007/s11356-020-09727-8
Deydier, E., Guilet, R., Sarda, S., and Sharrock, P. (2005). Physical and chemical characterisation of crude meat and bone meal combustion residue: ‘waste or raw material? J. Hazard. Mater. 121 (1–3), 141–148. doi:10.1016/j.jhazmat.2005.02.003
Dunn, K. (2018). Pid: process improvement using data. Available at: https://cran.r-project.org/package=pid.
Dutta, S., Manna, K., Srivastava, S. K., Gupta, A. K., and Kumar Yadav, M. (2020). Hollow polyaniline microsphere/Fe3O4 nanocomposite as an effective adsorbent for removal of arsenic from water. Sci. Rep. 10 (1), 4982. doi:10.1038/s41598-020-61763-z
Ebadollahzadeh, H., and Zabihi, M. (2020). Competitive adsorption of methylene blue and Pb (II) ions on the nano-magnetic activated carbon and alumina. Mater. Chem. Phys. 248 (July), 122893. doi:10.1016/j.matchemphys.2020.122893
Farch, S., Yahoum, M. M., Toumi, S., Tahraoui, H., Lefnaoui, S., Kebir, M., et al. (2023). Application of walnut shell biowaste as an inexpensive adsorbent for methylene blue dye: isotherms, kinetics, thermodynamics, and modeling. Separations 10 (1), 60. doi:10.3390/separations10010060
Fijoł, N., Abdelhamid, H. N., Pillai, B., Hall, S. A., Thomas, N., and Mathew, A. P. (2021). 3D-Printed monolithic biofilters based on a polylactic acid (PLA) – hydroxyapatite (HAp) composite for heavy metal removal from an aqueous medium. RSC Adv. 11 (51), 32408–32418. doi:10.1039/D1RA05202K
Guilhen, S. N., Watanabe, T., Silva, T. T., Rovani, S., Marumo, J. T., Tenório, J. A. S., et al. (2022). Role of point of zero charge in the adsorption of cationic textile dye on standard biochars from aqueous solutions: selection criteria and performance assessment. Recent Prog. Mater. 4 (2), 1. doi:10.21926/rpm.2202010
Ho, Y. S., and McKay, G. (1999). Pseudo-second order model for sorption processes. Process Biochem. 34 (5), 451–465. doi:10.1016/S0032-9592(98)00112-5
Ho, Y. S., and McKay, G. (2002). Application of kinetic models to the sorption of copper(II) on to peat. Adsorpt. Sci. and Technol. 20 (8), 797–815. doi:10.1260/026361702321104282
Holliday, M. C., Parsons, D. R., and Zein, S. H. (2024). Agricultural pea waste as a low-cost pollutant biosorbent for methylene blue removal: adsorption kinetics, isotherm and thermodynamic studies. Biomass Convers. Biorefinery 14 (5), 6671–6685. doi:10.1007/s13399-022-02865-8
Hunter, R. J. (1988). Zeta potential in colloid science: principles and applications. London: Academic Press.
Hynes, N. R. J., Senthil Kumar, J., Kamyab, H., Angela Jennifa Sujana, J., Ali Al-Khashman, O., Kuslu, Y., et al. (2020). Modern enabling techniques and adsorbents based dye removal with sustainability concerns in textile industrial sector -A comprehensive review. J. Clean. Prod. 272 (November), 122636. doi:10.1016/j.jclepro.2020.122636
Jain, H., Yadav, V., Rajput, V. D., Minkina, T., Agarwal, S., and Garg, M. C. (2022). An eco-sustainable green approach for biosorption of methylene blue dye from textile industry wastewater by sugarcane bagasse, peanut hull, and orange peel: a comparative study through response surface methodology, isotherms, kinetic, and thermodynamics. Water, Air, and Soil Pollut. 233 (6), 187. doi:10.1007/s11270-022-05655-0
Jayalakshmi, R., Soundaranayaki, K., and Subhash Kannan, M. (2023). Removal of methylene blue dye from textile wastewater using vertical flow constructed wetland. Mater. Today Proc. 77, 365–370. doi:10.1016/j.matpr.2022.12.030
Kesmez, Ö. (2020). Preparation of anti-bacterial biocomposite nanofibers fabricated by electrospinning method. J. Turkish Chem. Soc. Sect. A Chem. 7 (1), 125–142. doi:10.18596/jotcsa.590621
Khalfaoui, A., Benalia, A., Laggoun, Z., Bouchareb, R., Zaamta, I., Melloul, R., et al. (2024). Effective synthesis and application of artichoke and orange peels-based bio-sorbents for ketoprofen removal from wastewater: process optimization using factorial methodology. Desalination Water Treat. 317 (January), 100197. doi:10.1016/j.dwt.2024.100197
Khan, I., Saeed, K., Zekker, I., Zhang, B., Hendi, A. H., Ahmad, A., et al. (2022). Review on methylene blue: its properties, uses, toxicity and photodegradation. Water 14 (2), 242. doi:10.3390/w14020242
Kooh, M. R. R., Thotagamuge, R., Chou Chau, Y.-F., Mahadi, A. H., and Lim, C. M. (2022). Machine learning approaches to predict adsorption capacity of Azolla pinnata in the removal of methylene blue. J. Taiwan Inst. Chem. Eng. 132 (March), 104134. doi:10.1016/j.jtice.2021.11.001
Kosswattaarachchi, A. M., and Cook, T. R. (2018). Repurposing the industrial dye methylene blue as an active component for redox flow batteries. ChemElectroChem 5 (22), 3437–3442. doi:10.1002/celc.201801097
Koyuncu, H., and Kul, A. R. (2020). Removal of methylene blue dye from aqueous solution by nonliving lichen (pseudevernia furfuracea (L.) zopf.), as a novel biosorbent. Appl. Water Sci. 10 (2), 72. doi:10.1007/s13201-020-1156-9
Kuang, Y., Zhang, X., and Zhou, S. (2020). Adsorption of methylene blue in water onto activated carbon by surfactant modification. Water 12 (2), 587. doi:10.3390/w12020587
Kumari, S., Chowdhry, J., Sharma, P., Agarwal, S., and Garg, M. C. (2023a). Integrating artificial neural networks and response surface methodology for predictive modeling and mechanistic insights into the detoxification of hazardous MB and CV dyes using saccharum officinarum L. Biomass. Chemosphere 344 (December), 140262. doi:10.1016/j.chemosphere.2023.140262
Kumari, S., Singh, S., Lo, S.-L., Sharma, P., Agarwal, S., and Garg, M. C. (2024). Machine learning and modelling approach for removing methylene blue from aqueous solutions: optimization, kinetics and thermodynamics studies. J. Taiwan Inst. Chem. Eng., 105361. doi:10.1016/j.jtice.2024.105361
Kumari, S., Verma, A., Sharma, P., Agarwal, S., Rajput, V. D., Minkina, T., et al. (2023b). Introducing machine learning model to response surface methodology for biosorption of methylene blue dye using Triticum aestivum biomass. Sci. Rep. 13 (1), 8574. doi:10.1038/s41598-023-35645-z
Lagergren, S. (1898). Zur theorie der sogenannten adsorption geloster stoffe. K. Sven. Vetenskapsakad. Handl. 24, 1–39.
Largitte, L., and Pasquier, R. (2016). A review of the kinetics adsorption models and their application to the adsorption of lead by an activated carbon. Chem. Eng. Res. Des. 109 (May), 495–504. doi:10.1016/j.cherd.2016.02.006
Lau, Ee V., Gan, S., Ng, H. K., and Poh, P. E. (2014). Extraction agents for the removal of polycyclic aromatic hydrocarbons (PAHs) from soil in soil washing technologies. Environ. Pollut. 184 (January), 640–649. doi:10.1016/j.envpol.2013.09.010
Lawagon, C. P., and Amon, R. E. C. (2019). Magnetic rice husk ash ‘cleanser’ as efficient methylene blue adsorbent. Environ. Eng. Res. 25 (5), 685–692. doi:10.4491/eer.2019.287
Lawal, I. M., Soja, U. B., Hussaini, A., Saleh, D., Aliyu, M., Noor, A., et al. (2023). Sequential batch reactors for aerobic and anaerobic dye removal: a mini-review. Case Stud. Chem. Environ. Eng. 8 (December), 100547. doi:10.1016/j.cscee.2023.100547
Lellis, B., Fávaro-Polonio, C. Z., Pamphile, J. A., and Polonio, J. C. (2019). Effects of textile dyes on health and the environment and bioremediation potential of living organisms. Biotechnol. Res. Innovation 3 (2), 275–290. doi:10.1016/j.biori.2019.09.001
Li, H., Budarin, V. L., Clark, J. H., North, M., and Wu, X. (2022). Rapid and efficient adsorption of methylene blue dye from aqueous solution by hierarchically porous, activated starbons®: mechanism and porosity dependence. J. Hazard. Mater. 436 (August), 129174. doi:10.1016/j.jhazmat.2022.129174
Lin, L., Jiang, W., and Xu, P. (2017). Comparative study on pharmaceuticals adsorption in reclaimed water desalination concentrate using biochar: impact of salts and organic matter. Sci. Total Environ. 601–602 (December), 857–864. doi:10.1016/j.scitotenv.2017.05.203
Lopes, E. C. N., dos Anjos, F. S. C., Vieira, E. F. S., and Cestari, A. R. (2003). An alternative Avrami equation to evaluate kinetic parameters of the interaction of Hg(II) with thin chitosan membranes. J. Colloid Interface Sci. 263 (2), 542–547. doi:10.1016/S0021-9797(03)00326-6
Mattar, E. P. L., Júnior, E. F. F., and de Oliveira, E. (2014). Caracterização Físico-Química de Cinza de Osso Bovino Para Avaliação Do Seu Potencial Uso Agrícola. Pesqui. Agropecuária Trop. 44 (1), 65–70. doi:10.1590/S1983-40632014000100003
Maurya, K. L., Swain, G., Kumar, M., Kumar Sonwani, R., Verma, A., and Singh, R. S. (2023). Biodegradation of Congo red dye using lysinibacillus species in a moving bed biofilm reactor: continuous study and kinetic evaluation. Appl. Biochem. Biotechnol. 195 (9), 5267–5279. doi:10.1007/s12010-023-04425-w
Meerbergen, K., Crauwels, S., Willems, K. A., Dewil, R., Van Impe, J., Appels, L., et al. (2017). Decolorization of reactive azo dyes using a sequential chemical and activated sludge treatment. J. Biosci. Bioeng. 124 (6), 668–673. doi:10.1016/j.jbiosc.2017.07.005
Mendes, L. C., Ribeiro, G. L., and Marques, R. C. (2012). In situ hydroxyapatite synthesis: influence of collagen on its structural and morphological characteristic. Mater. Sci. Appl. 03 (08), 580–586. doi:10.4236/msa.2012.38083
Mijinyawa, A. H., Durga, G., and Mishra, A. (2019). A sustainable process for adsorptive removal of methylene blue onto a food grade mucilage: kinetics, thermodynamics, and equilibrium evaluation. Int. J. Phytoremediation 21 (11), 1122–1129. doi:10.1080/15226514.2019.1606785
Mijwel, A.-A. S., Ali, N. A., Afan, H. A., Alayan, H. M., Sherif, M., and Ahmed, E. (2023). Artificial intelligence models for methylene blue removal using functionalized carbon nanotubes. Sci. Rep. 13 (1), 18260. doi:10.1038/s41598-023-45032-3
Mojiri, A., Zhou, J. L., KarimiDermani, B., Razmi, E., and Kasmuri, N. (2023). Anaerobic membrane bioreactor (AnMBR) for the removal of dyes from water and wastewater: progress, challenges, and future perspectives. Processes 11 (3), 855. doi:10.3390/pr11030855
Musa, M. A., Chowdhury, S., Biswas, S., Nur Alam, S. M., Parvin, S., and Sattar, M. A. (2024). Removal of aqueous methylene blue dye over vallisneria natans biosorbent using artificial neural network and statistical response surface methodology analysis. J. Mol. Liq. 393 (January), 123624. doi:10.1016/j.molliq.2023.123624
Mussa, Z. H., Al-Ameer, L. R., Al-Qaim, F. F., Deyab, I. F., Kamyab, H., and Chelliapan, S. (2023). A comprehensive review on adsorption of methylene blue dye using leaf waste as a bio-sorbent: isotherm adsorption, kinetics, and thermodynamics studies. Environ. Monit. Assess. 195 (8), 940. doi:10.1007/s10661-023-11432-1
Nasar, A., and Mashkoor, F. (2019). Application of polyaniline-based adsorbents for dye removal from water and wastewater—a review. Environ. Sci. Pollut. Res. 26 (6), 5333–5356. doi:10.1007/s11356-018-3990-y
Neolaka, Y. A. B., Lawa, Y., Naat, J., Lalang, A. C., Widyaningrum, B. A., Ngasu, G. F., et al. (2023). Adsorption of methyl red from aqueous solution using Bali cow bones (Bos javanicus domesticus) hydrochar powder. Results Eng. 17 (March), 100824. doi:10.1016/j.rineng.2022.100824
Nippatla, N., and Philip, L. (2019). Electrocoagulation-floatation assisted pulsed power plasma technology for the complete mineralization of potentially toxic dyes and real textile wastewater. Process Saf. Environ. Prot. 125 (May), 143–156. doi:10.1016/j.psep.2019.03.012
Oladoja, N. A. (2016). A critical review of the applicability of Avrami fractional kinetic equation in adsorption-based water treatment studies. Desalination Water Treat. 57 (34), 15813–15825. doi:10.1080/19443994.2015.1076355
Parakala, S., Moulik, S., and Sridhar, S. (2019). Effective separation of methylene blue dye from aqueous solutions by integration of micellar enhanced ultrafiltration with vacuum membrane distillation. Chem. Eng. J. 375 (November), 122015. doi:10.1016/j.cej.2019.122015
Paschalis, E. P., Mendelsohn, R., and Boskey, A. L. (2011). Infrared assessment of bone quality: a review. Clin. Orthop. and Relat. Res. 469 (8), 2170–2178. doi:10.1007/s11999-010-1751-4
Passos, C. G., Lima, E. C., Arenas, L. T., Simon, N. M., da Cunha, B. M., Brasil, J. L., et al. (2008). Use of 7-amine-4-azahepthylsilica and 10-amine-4-azadecylsilica xerogels as adsorbent for Pb(II). Colloids Surfaces A Physicochem. Eng. Aspects 316 (1–3), 297–306. doi:10.1016/j.colsurfa.2007.09.025
Plazinski, W., Rudzinski, W., and Plazinska, A. (2009). Theoretical models of sorption kinetics including a surface reaction mechanism: a review. Adv. Colloid Interface Sci. 152 (1–2), 2–13. doi:10.1016/j.cis.2009.07.009
Prado, E. S. P., Miranda, F. S., de Araujo, L. G., Fernandes, G. L., J Pereira, A. L., Gomes, M. C., et al. (2022). Physicochemical modifications and decolorization of textile wastewater by ozonation: performance evaluation of a batch system. Ozone Sci. and Eng. 45, 276–290. doi:10.1080/01919512.2022.2088470
Raj, S., Singh, H., and Bhattacharya, J. (2023). Treatment of textile industry wastewater based on coagulation-flocculation aided sedimentation followed by adsorption: process studies in an industrial ecology concept. Sci. Total Environ. 857 (January), 159464. doi:10.1016/j.scitotenv.2022.159464
R Core Team (2023). R: a language and environment for statistical computing. Vienna, Austria: R Foundation for Statistical Computing. Available at: https://www.r-project.org/.
Sah, M. K., Edbey, K., El-Hashani, A., Almshety, S., Mauro, L., Alomar, T. S., et al. (2022). Exploring the biosorption of methylene blue dye onto agricultural products: a critical review. Separations 9 (9), 256. doi:10.3390/separations9090256
Sahana, H., Kumar Khajuria, D., Razdan, R., Roy Mahapatra, D., Bhat, M. R., Suresh, S., et al. (2013). Improvement in bone properties by using risedronate adsorbed hydroxyapatite novel nanoparticle based formulation in a rat model of osteoporosis. J. Biomed. Nanotechnol. 9 (2), 193–201. doi:10.1166/jbn.2013.1482
Salman, S. D., Zair, Z. R., and Alismaeel, Z. T. (2024). Bio-adsorption of cationic-cnionic dyes from synthetic effluents using an experimental design approach. Desalination Water Treat. 317 (January), 100246. doi:10.1016/j.dwt.2024.100246
Sfair, I. C. A. (2021). Avaliação Da Utilização de Cinzas de Queima de Bagaço de Cana-de-Açúcar Na Biossorção de Corantes Presentes Em Águas Residuárias Têxteis. Available at: https://repositorio.ufscar.br/handle/ufscar/14895.
Shah, P., Bhattarai, A., and Kumar, D. (2022). Interaction of methylene blue with SDS in the premicellar solution of CPC in the aqueous and methanol-water system. Colloids Surfaces A Physicochem. Eng. Aspects 648 (September), 129091. doi:10.1016/j.colsurfa.2022.129091
Shah, P., Jha, S. K., and Bhattarai, A. (2021). Spectrophotometric study of the sodium dodecyl sulfate in the presence of methylene blue in the methanol–water mixed solvent system. J. Mol. Liq. 340 (October), 117200. doi:10.1016/j.molliq.2021.117200
Sharma, K., Sharma, S., Sharma, V., Mishra, P. K., Ekielski, A., Sharma, V., et al. (2021). Methylene blue dye adsorption from wastewater using hydroxyapatite/gold nanocomposite: kinetic and thermodynamics studies. Nanomaterials 11 (6), 1403. doi:10.3390/nano11061403
Sun, L., Hu, D., Zhang, Z., and Deng, X. (2019). Oxidative degradation of methylene blue via PDS-based advanced oxidation process using natural pyrite. Int. J. Environ. Res. Public Health 16 (23), 4773. doi:10.3390/ijerph16234773
Suresh, R., Rajendran, S., Gnanasekaran, L., Show, P. L., Chen, W.-H., and Soto-Moscoso, M. (2023). Modified poly(vinylidene fluoride) nanomembranes for dye removal from water – a review. Chemosphere 322 (May), 138152. doi:10.1016/j.chemosphere.2023.138152
Tong, Y., McNamara, P. J., and Mayer, B. K. (2019). Adsorption of organic micropollutants onto biochar: a review of relevant kinetics, mechanisms and equilibrium. Environ. Sci. Water Res. and Technol. 5 (5), 821–838. doi:10.1039/C8EW00938D
Toor, M., and Jin, B. (2012). Adsorption characteristics, isotherm, kinetics, and diffusion of modified natural bentonite for removing diazo dye. Chem. Eng. J. 187, 79–88. doi:10.1016/j.cej.2012.01.089
Vassalo, A. R., Eugénia, C., Batista De Carvalho, L. A. E., and Gonçalves, D. (2016). Rather yield than break: assessing the influence of human bone collagen content on heat-induced warping through vibrational spectroscopy. Int. J. Leg. Med. 130 (6), 1647–1656. doi:10.1007/s00414-016-1400-x
Vieira, L. C., de Araujo, L. G., de Padua Ferreira, R. V., da Silva, E. A., Canevesi, R. L. S., and Marumo, J. T. (2019). Uranium biosorption by lemna sp. and Pistia stratiotes. J. Environ. Radioact. 203 (February), 179–186. doi:10.1016/j.jenvrad.2019.03.019
Viswanathan, N., Kumar, I. A., and Meenakshi, S. (2019). Development of chitosan encapsulated tricalcium phosphate biocomposite for fluoride retention. Int. J. Biol. Macromol. 133 (July), 811–816. doi:10.1016/j.ijbiomac.2019.04.076
Watanabe, T., Guilhen, S. N., Marumo, J. T., Papai de Souza, R., and de Araujo, L. G. (2021). Uranium biosorption by hydroxyapatite and bone meal: evaluation of process variables through experimental design. Environ. Sci. Pollut. Res. 29, 79816–79829. doi:10.1007/s11356-021-17551-x
Xiong, Y., Wang, Q., Duan, M., Tan, J., Fang, S., and Wu, J. (2018). Real-time monitoring of azo dye interfacial adsorption at silica–water interface by total internal reflection-induced surface evanescent wave. Langmuir 34 (26), 7612–7623. doi:10.1021/acs.langmuir.8b00722
Yang, X., and Al-Duri, B. (2005). Kinetic modeling of liquid-phase adsorption of reactive dyes on activated carbon. J. Colloid Interface Sci. 287 (1), 25–34. doi:10.1016/j.jcis.2005.01.093
Yu, J., Wang, J., and Jiang, Y. (2017). Removal of uranium from aqueous solution by alginate beads. Nucl. Eng. Technol. 49 (3), 534–540. doi:10.1016/j.net.2016.09.004
Zahrani, A. A., and Ayati, B. (2020). Using heterogeneous Fe-ZSM-5 nanocatalyst to improve the electro fenton process for acid blue 25 removal in a novel reactor with orbiting electrodes. J. Electroanal. Chem. 873 (September), 114456. doi:10.1016/j.jelechem.2020.114456
Zhang, L., Zhang, H., Tian, Y., Chen, Z., and Lu, H. (2012). Adsorption of methylene blue from aqueous solutions onto sintering process red mud. Desalination Water Treat. 47 (1–3), 31–41. doi:10.1080/19443994.2012.696412
Zhou, S., Du, Z., Li, X., Zhang, Y., He, Y., and Zhang, Y. (2019a). Degradation of methylene blue by natural manganese oxides: kinetics and transformation products. R. Soc. Open Sci. 6 (7), 190351. doi:10.1098/rsos.190351
Keywords: adsorption, dyes, experimental design, low-cost biosorbents, machine learning
Citation: de Araujo LG, Martins GF, Campera AAA, Marumo JT and Guilhen SN (2024) Biosorption of methylene blue by bone meal: experimental and modeling with machine learning and full factorial design. Front. Environ. Chem. 5:1451051. doi: 10.3389/fenvc.2024.1451051
Received: 18 June 2024; Accepted: 29 August 2024;
Published: 18 September 2024.
Edited by:
Jun Li, University of Waterloo, CanadaReviewed by:
Bulgariu Laura, Gheorghe Asachi Technical University of Iași, RomaniaAjaya Bhattarai, Tribhuvan University, Nepal
Copyright © 2024 de Araujo, Martins, Campera, Marumo and Guilhen. This is an open-access article distributed under the terms of the Creative Commons Attribution License (CC BY). The use, distribution or reproduction in other forums is permitted, provided the original author(s) and the copyright owner(s) are credited and that the original publication in this journal is cited, in accordance with accepted academic practice. No use, distribution or reproduction is permitted which does not comply with these terms.
*Correspondence: Leandro Goulart de Araujo, bGdvdWxhcnRAYWx1bW5pLnVzcC5icg==
†Present address: Leandro Goulart de Araujo, Université de Lyon, Université Claude Bernard Lyon 1, CNRS, IRCELYON UMR 5256Villeurbanne Cedex, France