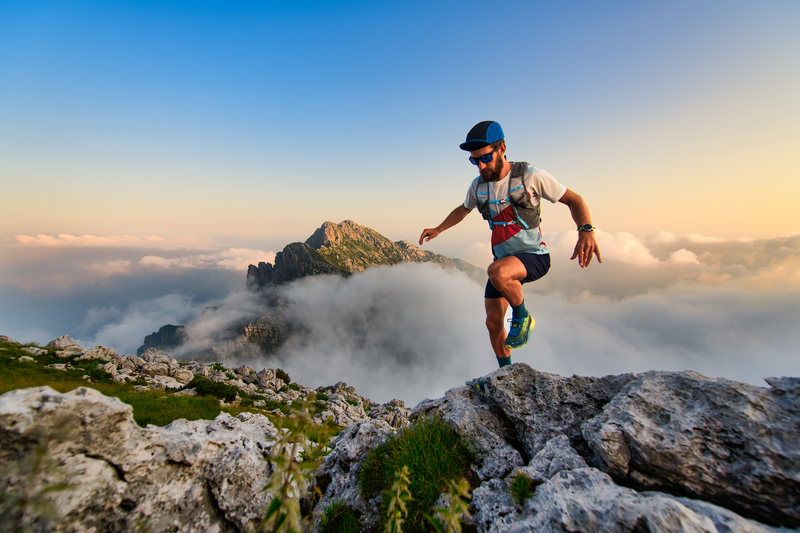
94% of researchers rate our articles as excellent or good
Learn more about the work of our research integrity team to safeguard the quality of each article we publish.
Find out more
ORIGINAL RESEARCH article
Front. Environ. Chem. , 28 August 2023
Sec. Inorganic Pollutants
Volume 4 - 2023 | https://doi.org/10.3389/fenvc.2023.1221820
Heavy metals are necessary at low concentration for biological activity, but they are often toxic for microorganisms at high concentrations. Amplicon sequencing of 16S rRNA and 18S rRNA was used to investigate changes in the bacterial and microeukaryotic communities in an activated sludge bioreactor incrementally contaminated with nickel (Ni), copper (Cu), and zinc (Zn) with an IC50 value ranging from 0% to 100%, as previously determined, while an uncontaminated bioreactor served as a control. The chemical oxygen demand (COD) was on average 90% in the uncontaminated bioreactor but dropped to 49% when the heavy metal concentration was 100% IC50. The bacterial community in the uncontaminated bioreactor was dominated by Alphaproteobacteria (mostly Agrobacterium and Brevundimonas) when the heavy metal concentrations were low and Bacteroidetes (mostly Sphingobacterium) when the highest amounts of heavy metal concentrations were applied. The members of Ciliophora, Ascomycota, and Basidiomycota alternatively dominated in the uncontaminated bioreactor, while Ascomycota (mostly Fusarium) dominated in the contaminated bioreactor. The results revealed that increased concentrations of Ni, Cu, and Zn altered the bacterial and microeukaryotic communities and some putative metabolic functions.
Industrialization, mining activities, and agricultural practices generate large amounts of contaminants that contribute to water, air, and soil pollution. These pollutants alter ecosystems, and some of them, such as heavy metals, might affect human and environment health (Ruangdech et al., 2017; Fatema et al., 2021). There is no specific definition of what a heavy metal is, but it is generally considered that they are characterized by an atomic weight between 63.5 and 200.6 and an element density of >5 g cm-3 (Saxena et al., 2020). A total of 13 heavy metals are considered important environmental pollutants, with copper (Cu), nickel (Ni), and zinc (Zn) being among the most important contaminants (Sparks, 2005). The anthropogenic sources of these heavy metals are mainly refinery, metallurgy, chemical manufacturing, paints, batteries, and electronic equipment (Gupta et al., 2020), whereas the main natural sources are sedimentary and igneous rocks (Alengebawy et al., 2021).
On the one hand, metals such as Cu and Zn are necessary in small amounts for metabolic activities in humans and plants. For instance, small amounts of Zn stimulate biological activity as a cofactor in RNA polymerase and transcriptions. On the other hand, large amounts of heavy metals are harmful to humans, plants, animals, and microorganisms (Sparks, 2005), as they affect metabolic activity and cause genetic damage (Qayyum et al., 2012). Consequently, the discharge of these heavy metals, as a result of human activity, can alter ecosystem functioning (Guo et al., 2010). They can accumulate in all levels of the food chain through biomagnification (Madgett et al., 2021), which can lead to the hyperaccumulation of these heavy metals (Mahapatra et al., 2020) in organisms. Some organisms can survive high concentrations of some heavy metals, while most cannot (Jackson et al., 2009; Kieu et al., 2011). The activity of most bacteria is inhibited when the concentrations of heavy metals increase (Bhat et al., 2020), as they alter the protein structure by inhibiting their activity. For instance, Cu can disrupt cellular functioning and inhibit enzymatic activity (Gupta et al., 2020). Cabrera et al. (2006) found that Ni at concentrations >8.5 mg L-1 inhibited the metabolic activity of Desulfovibrio vulgaris. Su et al. (2020) reported that bacterial communities in a bioreactor can be negatively affected by Ni at concentrations >10 mg L-1. Cu at high concentrations negatively affects cellulase activities and inhibits microbial communities (Guo et al., 2012). Large amounts of Zn inhibit the removal rates of organic material expressed as the chemical oxygen demand (COD) in wastewater treatment plants and inhibit the dominant functional bacteria (Wang et al., 2020).Therefore, according to the US EPA, the permissible limits of heavy metals that can be discharged in wastewater are 0.25 mg Cu L-1, 0.20 mg Ni L-1, and 0.80 mg Zn L-1 (Gunatilake, 2015).
Wastewater is normally treated prior to discharge. The objective of treating wastewater is to remove suspended solids, organic matter, and nutrients to a greater extent. The efficiency of wastewater treatment depends on microbial activity (Gao et al., 2022). Thus, it is important to know how the microbial communities are affected by pollutants, such as heavy metals, as this may reduce the efficiency of wastewater treatment while the normal microbial activity might be altered. Nevertheless, to the best of our knowledge, no study has reported the effect of the incremental application of heavy metals on microbial communities and how they respond to these changes.
In this study, two bioreactors were inoculated with activated sludge and fed continuously with synthetic wastewater for several months (Esquivel-Rios et al., 2014). One bioreactor was contaminated with increased amounts of Cu, Ni, and Zn, and the effect of the incremental increase in heavy metals on bacterial community was monitored through 16S rRNA gene sequencing and on the microeukaryotic community through 18S rRNA gene sequencing. An uncontaminated bioreactor maintained under the same operational conditions served as a control. The objective of this study was to investigate how an incremental application of Cu, Ni, and Zn affects the bacterial and microeukaryotic communities. Therefore, we investigated which groups adapted to increased heavy metal concentrations. These bacterial and microeukaryotic groups might play a significant role in the degradation of organic pollutants when wastewater is contaminated with heavy metals.
Two bioreactors were inoculated with 1 L sludge obtained from a wastewater treatment plant in Mexico City (Mexico) and maintained under steady state for several years with synthetic wastewater prior to their use in the current experiment. The details of the experimental setup can be found in Esquivel-Rios et al. (2014). Briefly, both bioreactors had a total working volume of 2.6 L each. In the first phase, i.e., from day 0 to day 22, both bioreactors were supplied with synthetic wastewater that contained (mg L-1) the following: peptone, 160; meat extract, 110; urea, 30; NaCl, 7; CaCl2•2H2O, 4; MgSO4•7H2O, 2; K2HPO4, 21.75; Na2HPO4, 33.40; and NH4Cl, 1.70. At day 22, one of the bioreactors was supplied additionally with increasing amounts of Cu (CuSO4•5H2O), Ni (NiCl2•6H2O), and Zn (ZnSO4•7H2O) (considered the heavy metal-amended bioreactor), while the other bioreactor was left uncontaminated (considered the unamended bioreactor) (Supplementary Table S1). The incremental concentration ranged from 0% to 100% of IC50 i.e., “the concentration at which a substance exerts half of its maximal inhibitory effect” (www.aatbio.com/resources/faq-frequently-asked-questions/What-is-the-ic50-value). The IC50 values used in this study were derived from Esquivel-Rios et al. (2014) for Cu and Zn and from Cokgor et al. (2007) and Hernandez-Martinez et al. (2018) for Ni. Both bioreactors were aerated with a constant flow rate of 1 VVM (air volume per bioreactor working volume), while the temperature was maintained at 21°C ± 2°C and the pH at 7.0 ± 0.5 by adding 1 M H3PO4 solution when necessary during the entire experiment. The initially activated sludge inoculum was characterized for COD, volatile suspended solids (VSSs), pH, temperature, and sludge volumetric index (SVI). At the beginning of the reactor operation, the COD concentration was 4,760 ± 345 mg COD L-1 and SSV 3,293 ± 186 mg SSV L-1, with a COD/SSV ratio of 1.44. The SVI was 110, indicating a good sedimentation of the sludge inoculum. The initial temperature of the inoculum was 22°C ± 5°C and pH 8.0 ± 0.3. The activated sludge reactors were acclimatized for 54 days prior to the application of the heavy metals. The acclimatization of the sludge allowed us to obtain a stable biomass in the bioreactor. The chemical oxygen demand was determined colorimetrically as described by the APHA (1999). The soluble COD was considered a representative of the substrate removal, and with that purpose, the COD was determined from the centrifugated (5900 g for 15 min; model 5810 R, Eppendorf, Mexico) and filtered sample (0.45-μm filter, 66601, Pall Inc., Mexico) from the influent and effluent samples of the reactors.
On each sampling day, three samples were taken from each bioreactor using a 15-mL sterile tube (Supplementary Figure S1) and maintained at −20°C until DNA extraction. DNA was extracted using two different techniques to guarantee the lysis of all cells. The first method used an enzymatic lysis of cells based on a method developed by Sambrook and Russel (2001), and the second technique used thermal shock for cell lysis (Valenzuela-Encinas et al., 2008).
The extracted DNA was used as the template DNA for polymerase chain reaction (PCR) amplification. The high-throughput sequencing primers used were the universal bacterial primers 341F (5′—CCTACGGGIGGCWGCAG—3′) and 805R (5′—GACTACHVGGGTATCTAATCC—3′) (Herlemann et al., 2011), which target the V3–V4 regions. The 18S rRNA genes were amplified using the primers SSU817F (5′—TTAGCATGGAATAATRRAATAGGA—3′) and SSU1196R (5′—ATCTGGSCCTGGTGSGTTTCC—3′) (Borneman and Hartin, 2000).
The amplicons of 16S rRNA and 18S rRNA were purified with the DNA Clean kit (Zymo Research, Irvine, CA, United States) and were quantified with the PicoGreen dsDNA Assay Kit (Invitrogen, Carlsbad, United States), using a NanoDrop 3300 Fluorospectrometer (Thermo Fisher Scientific, Waltham, MA, United States). DNA was sequenced using a MiSeq 2 × 300 PE (Illumina, San Diego, California, United States) performed by Macrogen, Inc. (DNA Sequencing Service, Seoul, Korea).
The 16S rRNA and 18S rRNA sequences were analyzed using QIIME2 (Quantitative Insights Into Microbial Ecology) v. 2020.8 software (Bolyen et al., 2019). The paired-end sequences were demultiplexing within QIIME2. Denoising and quality analysis were done using DADA2 (Callahan et al., 2016). The low-quality sequences were removed (<30 Phred score). The taxonomy was determined in the Greengenes database for 16S rRNA sequences and SILVA v. 138 for 18S rRNA sequences by using the blast consensus method.
The data were analyzed using R v. 4.0.5 (R Core Team). The data were centered log-ratio (CLR)-normalized using the ALDEx2 package for “normalizing the reads to a common sequencing depth” (Gloor et al., 2017). The barplot figures were prepared using the ggplot2 package (Wickham et al., 2022). The principal component analysis (PCA) done out using the FactoMineR package using the centered log-ratio-normalized data (Lê et al., 2008). The Hill numbers of order q = 0, q = 1, and q = 2 (Chao et al., 2014) were calculated using the hillR package (Li, 2018) and plotted using the ggplot2 package.
The putative metabolic functions were determined using the PICRUSt2 (Phylogenetic Investigation of Communities by Reconstruction of Unobserved States) (https://github.com/picrust/picrust2; v2.0.0-b) (Douglas et al., 2020) plugin within QIIME2. The feature tables and representative sequences of the samples of 16S rRNA of both bioreactors were used as input data for the functional prediction. The putative functions were determined in the “Kyoto Encyclopedia of Genes and Genomes (KEGG)” ortholog database with the PICRUSt2 results. The outputs were normalized using the ALDEx2 package. The resulted metabolic pathways were determined using STAMP (statistical analysis of taxonomic and functional profiles) v. 2.1.3 software (Parks et al., 2014).
In the unamended bioreactor, the percentage of COD removed changed slightly over time and was on average 90% (Figure 1). After the first application of heavy metals (phase II), the percentage of COD removed did not change in the heavy metal-amended bioreactor but decreased at the end of phase III. In phases IV and V, the percentage of COD removed dropped initially after the application of heavy metals but increased again toward the end of both phases. In phase VI, the percentage of COD removed decreased in the heavy metal-amended bioreactor over time but without recovery. After 141 days, the percentage of COD removed in the heavy metal-amended bioreactor was only 49%.
The Hill numbers were generally larger in the unamended bioreactor than those in the heavy metal-amended bioreactor and were larger at the onset than toward the end of the experiment (Supplementary Figure S2). The amplicon sequence variant (ASV) richness (q = 0) and typical ASV (q = 1) were larger in the unamended bioreactor than those in the heavy metal-amended bioreactor in phases IV and V and were similar or larger in the heavy metal-contaminated bioreactor than those in the unamended reactor in phases II, III, and VI (Supplementary Figure S2). The dominant (q = 2) ASVs were similar in both bioreactors, except in phases II, III, and V where they were larger in the unamended bioreactor than in the heavy metal-amended bioreactor (Supplementary Figure S2).
Proteobacteria (relative abundance 79%), mostly Alphaproteobacteria, dominated in the unamended bioreactor, with members of Bacteroidetes being the second most abundant bacterial phylum (14%) (Figure 2A). The relative frequency of the most abundant bacterial genera showed large variations in the unamended bioreactor over time so that the most dominant group often changed from phase to phase (Figure 3A). For instance, members of Shinella dominated toward the end of phase IV, whereas phylotypes belonging to Brevundimonas dominated at the end of phase VI.
FIGURE 2. Relative frequency (%) of bacterial phyla and proteobacterial classes in the (A) unamended bioreactor and (B) heavy metal-amended bioreactor.
FIGURE 3. Relative frequency (%) of bacterial genera in the (A) unamended bioreactor and (B) heavy metal-amended bioreactor.
Proteobacteria also dominated in the heavy metal-amended bioreactor (68%) until day 89, while members of Bacteroidetes dominated thereafter (Figure 2B). Members of the Alphaproteobacteria dominated in phases II and III, but their relative abundance decreased when the amount of heavy metals applied to the bioreactor increased (phase IV). They were replaced gradually by Gammaproteobacteria. The relative frequency of Bacteroidetes started to increase from day 89 onward and reached a maximum of 89% on day 109, i.e., the onset of phase VI. Thereafter, their relative frequency decreased, while that of Gammaproteobacteria increased again. Members of Agrobacterium, Brevundimonas, and Pseudochrobactrum dominated in the heavy metal-amended bioreactor in phases II and III. In phases IV and V, i.e., from day 52 until day 89, members of Stenotrophomonas dominated in the heavy metal-amended bioreactor (86%), but they were replaced by phylotypes belonging to Sphingobacterium toward the end of the experiment (Figure 3B). Consequently, a PCA clearly separated the bacterial community in the uncontaminated bioreactor from that in the heavy metal-contaminated bioreactor in each phase of the experiment (Supplementary Figure S3).
The microeukaryotic communities were different between the unamended and metal-amended bioreactors. Ciliophora dominated in the unamended bioreactor in phases I, II, III, and V, while members of Ascomycota dominated in phase VI and Basidiomycota in phase IV (Figures 4A, 5A). Ascomycota dominated in the heavy metal-amended bioreactor in phases II, III, V, and VI. The most abundant microscopic eukaryotic genus in the heavy metal-amended bioreactor was Fusarium; however, in the unamended bioreactor, other members of Chytridiomycota and Basidiomycota dominated (Figures 4B, 5B).
FIGURE 4. Relative frequency (%) of microeukaryotic phyla in the (A) unamended bioreactor and (B) heavy metal-amended bioreactor.
FIGURE 5. Relative frequency (%) of microeukaryotic genera in the (A) unamended bioreactor and (B) heavy metal-amended bioreactor.
In both bioreactors, the most abundant putative metabolic functions were related to the central metabolism, i.e., xenobiotics metabolism and biodegradation, the metabolism of cofactors and vitamins, and carbohydrate and amino acid metabolism (Figure 6). The relative abundance of putative xenobiotic biodegradation and metabolism was larger in the uncontaminated bioreactor than in the heavy metal-amended bioreactor, while that of the metabolism of cofactors and vitamins showed an opposite trend. The proportion of genetic information processing, such as replication and repair and translation and transcription processing, was higher in the heavy metal-amended bioreactor than in the unamended bioreactor.
FIGURE 6. Putative metabolic functions as predicted using the KEGG ortholog classification of the predicted functions in the heavy metal-amended (blue) and unamended bioreactors (red).
Heavy metals, such as Cu, Ni, and Zn, are known to inhibit COD removal in a bioreactor. In this study, increased applications of Cu, Ni, and Zn reduced COD removal compared to the uncontaminated bioreactor, indicating that the heavy metals applied inhibited microbial activity. For instance, Liu et al. (2020) found that Ni(II) at a concentration of 30 mg L-1 inhibited the COD removal as it inhibited dehydrogenase activity. Wang et al. (2018) reported that the percentage of COD removal dropped to 85% when the concentration of Cu (II) was 10 mg L-1 in a bioreactor, while Sun et al. (2016) found that the COD removal percentage was higher in a bioreactor fed with activated sludge and contaminated with 20 mg Cu L-1 (54%) than in the bioreactor contaminated with 40 mg Cu L-1 (14%); the more contaminated the system was, the less the COD removal percentage was. Wang et al. (2009) reported that Cu binds mostly to enzymes, such as urease and phosphatase, reducing their activity and thereby affecting the P and N cycle. Wang et al. (2020) found that the COD removal decreased by 5% in a bioreactor fed with activated sludge in the presence of Zn(II) compared to a non-contaminated bioreactor. In this study, increased applications of Cu, Ni, and Zn reduced the COD removal in the heavy-metal-contaminated bioreactor compared to the unamended bioreactor, indicating that the heavy metals applied inhibited the microbial metabolic activity.
Bhat et al. (2020) reported that the alpha diversity in an uncontaminated bioreactor inoculated with activated sludge was higher when 100 mg Cu L-1 was added after 30 days. In this study, the alpha diversity was higher in the metal-amended bioreactor than in the unamended bioreactor in phase II. However, in the last three phases, the effective number of ASVs was lower in the heavy metal-amended bioreactor than in the unamended bioreactor. Therefore, the bacteria were well adapted to lower heavy metal concentrations in the bioreactor (phase II) but not to higher concentrations (phases IV, V, and VI).
For comparing the microbial community, two bioreactors were set up: a heavy metal-amended bioreactor polluted with incremental concentrations of Ni, Cu, and Zn and an unamended bioreactor that served as a control. Proteobacteria was the most abundant phylum followed by Bacteroidetes in both the heavy metal-amended and unamended bioreactors. Proteobacteria contain a large number of versatile strains and often dominate not only in different ecosystems, e.g., in fresh and salt water (Zhou et al., 2020), but also in the gastrointestinal tract of humans. Sharma et al. (2021) stated that Proteobacteria play an important role in the detoxification of organic and inorganic pollutants in wastewater. Bacteroidetes are known to degrade polymeric organic matter and also to colonize diverse environments, such as soils, oceans, freshwater, and the gastrointestinal tract of mammals (Thomas et al., 2011). Bacteroidetes dominate in gut microbiota, where they are essential in digesting food in the intestinal tract (Rinninella et al., 2019). Niestępski et al. (2020) found that Proteobacteria, Firmicutes, and Bacteroidetes are the most abundant phyla in wastewater treatment plants and activated sludge. The inoculum used in the bioreactors was taken from wastewater, mostly from households. This might explain why the members of Bacteroidetes were so abundant in the bioreactor studied.
Alphaproteobacteria was the most abundant class in phases II and III, and Agrobacterium was the dominant genus in the heavy metal-amended bioreactor in the first 45 days, i.e., phase II, III, and part of IV, which suggests that these groups can thrive in a bioreactor with low concentrations of Cu, Ni, and Zn (10% and 20% IC50). Alphaproteobacteria, often phototrophs, can be found in very different habitats that include soil, oceans, freshwater, lichens, and in activated sludge of wastewater treatment plants (Imhoff, 2006). Phylotypes belonging to Agrobacterium are Gram-negative heterotrophic bacteria and are usually found in soils, which cause tumors in plants (Nester, 2015). Some members of Agrobacterium are heavy metal-resistant bacteria, such as Agrobacterium tumefaciens CCNWGS0286 isolated from a zinc–lead mine tailing (Hao et al., 2012) and the copper-resistant Agrobacterium tumefaciens strain type IAM 13129 isolated from root nodules of Lespedeza cuneata growing in a gold mining tailing region in the northwest of China (Yu et al., 2009). As such, Agrobacterium can survive the heavy metal concentrations applied in this experiment.
It has been found that Gammaproteobacteria and Alphaproteobacteria are the most abundant Proteobacteria class in activated sludge (Sun et al., 2021). In phase V, members of Gammaproteobacteria replaced Alphaproteobacteria as the more dominant proteobacterial class. Sun et al. (2016) found that the relative abundance of Gammaproteobacteria increased gradually compared to that of the other members of Proteobacteria when the Cu (II) concentration increased from 10 mg L-1 to 40 mg L-1 in a bioreactor inoculated with activated sludge of domestic and industrial origin. Di Cesare et al. (2020) found that Gammaproteobacteria was the dominant class (31%) on the surface of the marine sediment with a concentration of 14 mg Ni kg-1, followed by Alphaproteobacteria (10%). Members of Gammaproteobacteria have a higher relative abundance of the CzcA gene (gene for Cd, Co, and Zn resistance) than Alphaproteobacteria when Zn(II) was found in the environment (Wang et al., 2020). These characteristics might explain why Gammaproteobacteria replaced Alphaproteobacteria when the heavy metal concentration increased.
It has been reported that members of Stenotrophomonas removed >90% of COD in treated wastewater (Al-Dhabi et al., 2021). Additionally, Stenotrophomonas can survive high concentrations of heavy metals, as it expresses genes responsible for resistance to metals, such as Cu (copLAB gene), Hg (ohrAB gene), and As (arsC1HRC2B gene) (Ryan et al., 2009). Stenotrophomonas can survive other adverse conditions, such as high salinity and extreme pH, and it was the dominant genus in a polycyclic aromatic hydrocarbon (PAH)-contaminated soil (Kuppusamy et al., 2016). This might explain why members of Stenotrophomonas were so abundant in the heavy metal-contaminated bioreactor in the last phases of this study.
Sphingobacterium is a Gram-negative bacterium and has been found in activated sludge of synthetic wastewater polluted with 4-chlorophenol (Zhao et al., 2016) and in soil contaminated with PAHs (Smułek et al., 2020). Nam et al. (2015) reported that the strain Sphingobacterium sp. KM-02, isolated from a polycyclic aromatic hydrocarbon-contaminated soil near a mine-impacted area in Korea, can degrade fluorene in the presence of heavy metals. They found that concentrations of 10 mg Cd and Cu L-1 inhibited their activity, Zn and Pb had a small negative effect at concentrations between 10 and 100 mg L-1, and As had no effect on the growth or fluorene degradation even at 100 mg L-1. This suggests that members of Sphingobacterium can survive in polluted environments and can be active when heavy metal concentrations are high. This might explain why Sphingobacterium was the most abundant genus in the last two phases in the heavy metal-amended bioreactor used in this study when IC50 was at 40% (phase V) and 100% (phase VI). Additionally, Arbianti et al. (2018) found that Gram-negative bacteria removed more COD from wastewater than Gram-positive bacteria. The high relative abundance of the Gram-negative genera Azospirillum, Agrobacterium, Sphingobacterium, and Stenotrophomonas correlated with a large COD removal (%) (Supplementary Figure S4), and they are identified as Gram-negative bacteria.
In this study, the diversity of the microeukaryotic communities in both bioreactors was lower than that of bacteria, and three phyla accounted for >80% of all microeukaryotes. Fungi can easily adapt to extreme environmental conditions. Ascomycota (fungi) dominated in the heavy metal-amended bioreactor, while Ciliophora and Basidiomycota (fungi) dominated in the unamended bioreactor used in this study. Basidiomycota and Ascomycota were the most abundant phyla in activated sludge samples (Niu et al., 2017; Zhang et al., 2018). Lin et al. (2019) reported that Ascomycota were well adapted to high heavy metal concentrations in paddy soil; the reason could be that Ascomycota can adapt well to diverse environmental stress and nutritional options (Hu et al., 2019; Li et al., 2022). The relative abundance of Basidiomycota was higher in the paddy soil with low amounts of heavy metals than those with higher concentrations (Lin et al., 2019). Members of Ciliophora (protists) are sensitive to heavy metals (Vilas–Boas et al., 2020). These studies indicate why Ascomycota dominated in the heavy metal-amended bioreactor and Ciliophora and Basidiomycota in the unamended bioreactor used in this study.
The genus Fusarium dominated in the heavy metal-amended bioreactor. It is a filamentous fungal phytopathogen that belongs to the Ascomycota phylum. Fusarium has been isolated from soil contaminated with different concentrations of Cu, Ni, and Zn (Zafar et al., 2007) and can survive large amounts of Ag (I) (El Sayed and El-Sayed, 2020). Raspanti et al. (2009) found that Fusarium strains cultivated in solid potato-dextrose agar were not inhibited by 10 mg Zn L-1 and 10 mg Pb L-1. Therefore, Fusarium can survive high heavy metal concentrations, as found in this study, where the maximum zinc concentration was 40 mg Zn L-1.
The most abundant putative metabolic functions in both bioreactors were essential metabolic pathways, as previously found in biological sludge (More et al., 2014; Ou et al., 2018; Yang et al., 2020). Li et al. (2020) found that the bacterial membrane transport pathway was enriched in river sediment polluted with 69.2 mg Cr kg-1 and suggested that this would allow bacteria to transport more metal ions across their membrane as a mechanism for survival. They also reported that genetic information processing, such as replication and repair and translation and transcription, was reduced in river sediment that contained 30.3 mg Cu kg-1 as this metal ion can pass through the membrane and inhibit enzyme activity. In this study, however, the proportion of membrane transport putative functions was similar in both the heavy metal-amended and unamended bioreactors. This suggests that heavy metal ions did not negatively affect genetic information processing in the bioreactor used in this study. Nevertheless, the xenobiotic biodegradation metabolism was negatively affected by heavy metal addition in the heavy metal-amended bioreactor, which could signify that the microbial community did not detoxify the system. More in-depth studies, such as shotgun metagenomic sequencing, are needed to recognize, in more detail, genes involved in metabolic pathways.
Heavy metals at 40% IC50, i.e., 4 mg Cu L-1, 16 mg Zn L-1, and 13.2 mg Ni L-1, are harmful to most microorganisms. However, the activity of some microorganisms is not affected even at high heavy metal concentrations. Therefore, they continue to contribute to the removal of COD in wastewater treatments. In this study, Stenotrophomonas, a member of Gammaproteobacteria, Sphingobacterium that belongs to Bacteroidetes, and Fusarium (Ascomycota) were enriched at the highest heavy metal concentration. More in-depth studies, such as shotgun metagenomic sequencing, are needed to further increase our knowledge of how heavy metals, such as Ni, Cu, and Zn, might affect other members of the microbial community, such as protists, fungi, archaea, and viruses, and their metabolic functioning.
Supplementary Material of this work can be found in the online version of this paper.
The datasets presented in this study can be found in online repositories. The names of the repository/repositories and accession number(s) can be found at: https://www.ncbi.nlm.nih.gov/bioproject/PRJNA972121 and PRJNA972121.
CA-S: conceptualization, methodology, software, formal analysis, investigation, data curation, and writing—original draft. NM-C: resources and data curation. MH-G: resources and data curation. GH-M: resources and data curation. YN-N: conceptualization, methodology, and writing—review and editing. FT: supervision, validation, writing—review and editing, project administration, and funding acquisition. LD: methodology, visualization, supervision, validation, writing—review and editing, project administration, and funding acquisition. All authors contributed to the article and approved the submitted version.
This work was funded by “Centro de Investigación y de Estudios Avanzados del IPN” (Cinvestav) and “Consejo Nacional de Ciencia y Tecnología” (CONACYT).
The authors gratefully acknowledge the grant-aided support from CONACYT for CA-S (grant no. 754492).
The authors declare that the research was conducted in the absence of any commercial or financial relationships that could be construed as a potential conflict of interest.
All claims expressed in this article are solely those of the authors and do not necessarily represent those of their affiliated organizations, or those of the publisher, the editors, and the reviewers. Any product that may be evaluated in this article, or claim that may be made by its manufacturer, is not guaranteed or endorsed by the publisher.
The Supplementary Material for this article can be found online at: https://www.frontiersin.org/articles/10.3389/fenvc.2023.1221820/full#supplementary-material
APHA (1999). Standard methods for the examination of water and wastewater. 20th edn. Washington DC: American Publishers Health Association.
Al-Dhabi, N. A., Esmail, G. A., Alzeer, A. F., and Arasu, M. V. (2021). Removal of nitrogen from wastewater of date processing industries using a Saudi Arabian mesophilic bacterium, Stenotrophomonas maltophilia Al-Dhabi-17 in sequencing batch reactor. Chemosphere 268, 128636. doi:10.1016/j.chemosphere.2020.128636
Alengebawy, A., Abdelkhalek, S. T., Qureshi, S. R., and Wang, M. Q. (2021). Heavy metals and pesticides toxicity in agricultural soil and plants: ecological risks and human health implications. Toxics 9 (3), 42–34. doi:10.3390/toxics9030042
Arbianti, R., Utami, T. S., Leondo, V., ElisabethPutri, S. A., and Hermansyah, H. (2018). Effect of biofilm and selective mixed culture on microbial fuel cell for the treatment of tempeh industrial wastewater. IOP Conf. Ser. Mater. Sci. Eng. 316 (1), 012073. doi:10.1088/1757-899X/316/1/012073
Bhat, S. A., Cui, G., Li, W., Wei, Y., and Li, F. (2020). Effect of heavy metals on the performance and bacterial profiles of activated sludge in a semi-continuous reactor. Chemosphere 241, 125035. doi:10.1016/j.chemosphere.2019.125035
Bolyen, E., Rideout, J. R., Dillon, M. R., Bokulich, N. A., Abnet, C. C., Al-Ghalith, G. A., et al. (2019). Reproducible, interactive, scalable and extensible microbiome data science using QIIME 2. Nat. Biotechnol. 37 (8), 852–857. doi:10.1038/s41587-019-0209-9
Borneman, J., and Hartin, R. J. (2000). PCR primers that amplify fungal rRNA genes from environmental samples. Appl. Environ. Microbiol. 66 (10), 4356–4360. doi:10.1128/AEM.66.10.4356-4360.2000
Cabrera, G., Pérez, R., Gómez, J. M., Ábalos, A., and Cantero, D. (2006). Toxic effects of dissolved heavy metals on Desulfovibrio vulgaris and Desulfovibrio sp. strains. J. Hazard. Mater. 135 (1–3), 40–46. doi:10.1016/j.jhazmat.2005.11.058
Callahan, B. J., McMurdie, P. J., Rosen, M. J., Han, A. W., Johnson, A. J. A., and Holmes, S. P. (2016). DADA2: high-resolution sample inference from illumina amplicon data. Nat. Methods 13 (7), 581–583. doi:10.1038/nmeth.3869
Chao, A., Chiu, C. H., and Jost, L. (2014). Unifying species diversity, phylogenetic diversity, functional diversity, and related similarity and differentiation measures through hill numbers. Annu. Rev. Ecol. Evol. Syst. 45, 297–324. doi:10.1146/annurev-ecolsys-120213-091540
Cokgor, E. U., Ozdemir, S., Karahan, O., Insel, G., and Orhon, D. (2007). Critical appraisal of respirometric methods for metal inhibition on activated sludge. J. Hazard. Mater. 139 (2), 332–339. doi:10.1016/j.jhazmat.2006.06.038
Di Cesare, A., Pjevac, P., Eckert, E., Curkov, N., Miko Šparica, M., Corno, G., et al. (2020). The role of metal contamination in shaping microbial communities in heavily polluted marine sediments. Environ. Pollut. 265, 114823. doi:10.1016/j.envpol.2020.114823
Douglas, G. M., Maffei, V. J., Zaneveld, J. R., Yurgel, S. N., Brown, J. R., Taylor, C. M., et al. (2020). PICRUSt2 for prediction of metagenome functions. Nat. Biotechnol. 38 (6), 685–688. doi:10.1038/s41587-020-0548-6
El Sayed, M. T., and El-Sayed, A. S. A. (2020). Tolerance and mycoremediation of silver ions by Fusarium solani. Heliyon 6 (5), e03866. doi:10.1016/j.heliyon.2020.e03866
Esquivel-Rios, I., González, I., and Thalasso, F. (2014). Microrespirometric characterization of activated sludge inhibition by copper and zinc. Biodegradation 25 (6), 867–879. doi:10.1007/s10532-014-9706-1
Fatema, K., Shoily, S. S., Ahsan, T., Haidar, Z., Sumit, A. F., and Sajib, A. A. (2021). Effects of arsenic and heavy metals on metabolic pathways in cells of human origin: similarities and differences. Toxicol. Rep. 8 (December 2020), 1109–1120. doi:10.1016/j.toxrep.2021.05.015
Gao, J., Yang, N., Liao, H., Yuan, H., and Zhu, J. (2022). Activated sludge microbial communities influencing the performance of a full-scale wastewater treatment plant treating optoelectronic wastewater. J. Environ. Chem. Eng. 10 (1), 106833. doi:10.1016/j.jece.2021.106833
Gloor, G. B., Macklaim, J. M., Pawlowsky-glahn, V., and Egozcue, J. J. (2017). Microbiome datasets are compositional: and this is not optional. Front. Microbiol. 8 (November), 2224–2226. doi:10.3389/fmicb.2017.02224
Guo, W., Liu, X., Liu, Z., and Li, G. (2010). Pollution and potential ecological risk evaluation of heavy metals in the sediments around Dongjiang Harbor, Tianjin. Procedia Environ. Sci. 2 (5), 729–736. doi:10.1016/j.proenv.2010.10.084
Guo, X., Gu, J., Gao, H., Qin, Q., Chen, Z., Shao, L., et al. (2012). Effects of Cu on metabolisms and enzyme activities of microbial communities in the process of composting. Bioresour. Technol. 108, 140–148. doi:10.1016/j.biortech.2011.12.087
Gupta, G. S., Yadav, G., and Tiwari, S. (2020). Bioremediation of heavy metals: a new approach to sustainable agriculture. Restor. Wetl. Ecosyst. A Trajectory Towards a Sustain. Environ., 195–226. doi:10.1007/978-981-13-7665-8_13
Hao, X., Xie, P., Johnstone, L., Miller, S. J., Rensing, C., and Wei, G. (2012). Genome sequence and mutational analysis of plant-growth-promoting bacterium Agrobacterium tumefaciens CCNWGS0286 isolated from a zinc-lead mine tailing. Appl. Environ. Microbiol. 78 (15), 5384–5394. doi:10.1128/AEM.01200-12
Herlemann, D., Labrenz, M., Jü Rgens, K., Bertilsson, S., Waniek, J. J., and Andersson, A. F. (2011). Transitions in bacterial communities along the 2000 km salinity gradient of the Baltic Sea. ISME J. 5, 1571–1579. doi:10.1038/ismej.2011.41
Hernandez-Martinez, G. R., Ortiz-Alvarez, D., Perez-Roa, M., Urbina-Suarez, N. A., and Thalasso, F. (2018). Multiparameter analysis of activated sludge inhibition by nickel, cadmium, and cobalt. J. Hazard. Mater. 351 (October), 63–70. doi:10.1016/j.jhazmat.2018.02.032
Hu, T., Wang, X., Zhen, L., Gu, J., Zhang, K., Wang, Q., et al. (2019). Effects of inoculating with lignocellulose-degrading consortium on cellulose-degrading genes and fungal community during co-composting of spent mushroom substrate with swine manure. Bioresour. Technol. 291 (May), 121876. doi:10.1016/j.biortech.2019.121876
Imhoff, J. (2006). “The phototrophic alpha-proteobacteria,” in The prokaryotes. 5th ed. (Springer), 41–64.
Jackson, V. A., Paulse, A. N., Bester, A. A., Neethling, J. H., Khan, S., and Khan, W. (2009). Bioremediation of metal contamination in the plankenburg river, western cape, South Africa. Int. Biodeterior. Biodegrad. 63 (5), 559–568. doi:10.1016/j.ibiod.2009.03.007
Kieu, H. T. Q., Mu, E., and Horn, H. (2011). Heavy metal removal in anaerobic semi-continuous stirred tank reactors by a consortium of sulfate-reducing bacteria. Water Res. 5, 4–11. doi:10.1016/j.watres.2011.04.043
Kuppusamy, S., Thavamani, P., Megharaj, M., Venkateswarlu, K., Lee, Y. B., and Naidu, R. (2016). Pyrosequencing analysis of bacterial diversity in soils contaminated long-term with PAHs and heavy metals: implications to bioremediation. J. Hazard. Mater. 317, 169–179. doi:10.1016/j.jhazmat.2016.05.066
Lê, S., Josse, J., and Husson, F. (2008). FactoMineR: an r package for multivariate analysis. J. Stat. Softw. 25 (1), 1–18. doi:10.18637/jss.v025.i01
Li, C., Quan, Q., Gan, Y., Dong, J., Fang, J., Wang, L., et al. (2020). Effects of heavy metals on microbial communities in sediments and establishment of bioindicators based on microbial taxa and function for environmental monitoring and management. Sci. Total Environ. 749, 141555. doi:10.1016/j.scitotenv.2020.141555
Li, D. (2018). hillR: taxonomic, functional, and phylogenetic diversity and similarity through hill numbers. J. Open Source Softw. 3 (13), 1041. doi:10.21105/joss.01041
Li, S., Chen, W., Liu, D., Tao, Y. Y., Ma, H., Feng, Z., et al. (2022). Effect of superphosphate addition on heavy metals speciation and microbial communities during composting. Bioresour. Technol. 359 (June), 127478. doi:10.1016/j.biortech.2022.127478
Lin, Y., Ye, Y., Hu, Y., and Shi, H. (2019). The variation in microbial community structure under different heavy metal contamination levels in paddy soils. Ecotoxicol. Environ. Saf. 180 (April), 557–564. doi:10.1016/j.ecoenv.2019.05.057
Liu, R., Li, S., Yu, N., Zhao, C., Gao, X., and Gao, C. (2020). Performance evaluation and microbial community shift of sequencing batch reactors under different nickel (Ni(II)) concentrations. Environ. Technol. Innovation 19 (238), 100991. doi:10.1016/j.eti.2020.100991
Madgett, A. S., Yates, K., Webster, L., McKenzie, C., and Moffat, C. F. (2021). The concentration and biomagnification of trace metals and metalloids across four trophic levels in a marine food web. Mar. Pollut. Bull. 173 (PA), 112929. doi:10.1016/j.marpolbul.2021.112929
Mahapatra, B., Dhal, N. K., Pradhan, A., and Panda, B. P. (2020). Application of bacterial extracellular polymeric substances for detoxification of heavy metals from contaminated environment: a mini-review. Materials Today: Proceedings, 30, 283–288. doi:10.1016/j.matpr.2020.01.490
More, R. P., Mitra, S., Raju, S. C., Kapley, A., and Purohit, H. J. (2014). Mining and assessment of catabolic pathways in the metagenome of a common effluent treatment plant to induce the degradative capacity of biomass. Bioresour. Technol. 153, 137–146. doi:10.1016/j.biortech.2013.11.065
Nam, I. H., Kim, Y., Cho, D., Kim, J. G., Song, H., and Chon, C. M. (2015). Effects of heavy metals on biodegradation of fluorene by a Sphingobacterium sp strain (KM-02) isolated from polycyclic aromatic hydrocarbon-contaminated mine soil. Environ. Eng. Sci. 32 (10), 891–898. doi:10.1089/ees.2015.0037
Nester, E. W. (2015). Agrobacterium: nature's genetic engineer. Front. Plant Sci. 5 (JAN), 730–816. doi:10.3389/fpls.2014.00730
Niestępski, S., Harnisz, M., Ciesielski, S., Korzeniewska, E., and Osińska, A. (2020). Environmental fate of Bacteroidetes, with particular emphasis on Bacteroides fragilis group bacteria and their specific antibiotic resistance genes, in activated sludge wastewater treatment plants. J. Hazard. Mater. 394 (March), 122544. doi:10.1016/j.jhazmat.2020.122544
Niu, L., Li, Y., Xu, L., Wang, P., Zhang, W., Wang, C., et al. (2017). Ignored fungal community in activated sludge wastewater treatment plants: diversity and altitudinal characteristics. Environ. Sci. Pollut. Res. 24 (4), 4185–4193. doi:10.1007/s11356-016-8137-4
Ou, D., Li, W., Li, H., Wu, X., Li, C., Zhuge, Y., et al. (2018). Enhancement of the removal and settling performance for aerobic granular sludge under hypersaline stress. Chemosphere 212, 400–407. doi:10.1016/j.chemosphere.2018.08.096
Parks, D. H., Tyson, G. W., Hugenholtz, P., and Beiko, R. G. (2014). Stamp: statistical analysis of taxonomic and functional profiles. Bioinformatics 30 (21), 3123–3124. doi:10.1093/bioinformatics/btu494
Qayyum, S., Ara, A., and Usmani, J. A. (2012). Effect of nickel and chromium exposure on buccal cells of electroplaters. Toxicol. Industrial Health 28 (1), 74–82. doi:10.1177/0748233711407237
Raspanti, E., Cacciola, S. O., Gotor, C., Romero, L. C., and García, I. (2009). Implications of cysteine metabolism in the heavy metal response in Trichoderma harzianum and in three Fusarium species. Chemosphere 76 (1), 48–54. doi:10.1016/j.chemosphere.2009.02.030
Rinninella, E., Raoul, P., Cintoni, M., Franceschi, F., Miggiano, G. A. D., Gasbarrini, A., et al. (2019). What is the healthy gut microbiota composition? A changing ecosystem across age, environment, diet, and diseases. Microorganisms 7 (1), 14. doi:10.3390/microorganisms7010014
Ruangdech, T., Wongphatcharachai, M., Staley, C., Sadowsky, M. J., and Sajjaphan, K. (2017). Influence of heavy metals on rhizosphere microbial communities of Siam weed (Chromolaena odorata (L)) using a 16S rRNA gene amplicon sequencing approach. Agric. Nat. Resour. 51 (3), 137–141. doi:10.1016/j.anres.2016.05.005
Ryan, R. P., Monchy, S., Cardinale, M., Taghavi, S., Crossman, L., Avison, M. B., et al. (2009). The versatility and adaptation of bacteria from the genus Stenotrophomonas. Nat. Rev. Microbiol. 7 (7), 514–525. doi:10.1038/nrmicro2163
Sambrook, J., Russel, D., and Lai, R. (2001). Molecular cloning: a laboratory manual. Dongwuxue Yanjiu 33 (1), 75–78. doi:10.3724/sp.j.1141.2012.01075
Saxena, G., Purchase, D., Mulla, S. I., Saratale, G. D., and Bharagava, R. N. (2020). Phytoremediation of heavy metal-contaminated sites: eco-environmental concerns, field studies, sustainability issues, and future prospects. Rev. Environ. Contam. Toxicol. 249, 71–131. doi:10.1007/398_2019_24
Sharma, P., Tripathi, S., and Chandra, R. (2021). Metagenomic analysis for profiling of microbial communities and tolerance in metal-polluted pulp and paper industry wastewater. Bioresour. Technol. 324 (January), 124681. doi:10.1016/j.biortech.2021.124681
Smułek, W., Sydow, M., Zabielska-Matejuk, J., and Kaczorek, E. (2020). Bacteria involved in biodegradation of creosote PAH – a case study of long-term contaminated industrial area. Ecotoxicol. Environ. Saf. 187, 109843. doi:10.1016/j.ecoenv.2019.109843
Sparks, D. L. (2005). Toxic metals in the environment: the role of surfaces. Plant Soil 1, 193–197. doi:10.2113/gselements.1.4.193
Su, J. F., Gao, J., lin Huang, T., and ming Zhang, Y. (2020). Simultaneous nitrate, nickel ions and phosphorus removal in a bioreactor containing a novel composite material. Bioresour. Technol. 305, 123081. doi:10.1016/j.biortech.2020.123081 (
Sun, F. L., Fan, L. L., and Xie, G. J. (2016). Effect of copper on the performance and bacterial communities of activated sludge using Illumina MiSeq platforms. Chemosphere 156, 212–219. doi:10.1016/j.chemosphere.2016.04.117
Sun, F., Xu, Z., and Fan, L. (2021). Response of heavy metal and antibiotic resistance genes and related microorganisms to different heavy metals in activated sludge. J. Environ. Manag. 300 (August), 113754. doi:10.1016/j.jenvman.2021.113754
Thomas, F., Hehemann, J. H., Rebuffet, E., Czjzek, M., and Michel, G. (2011). Environmental and gut Bacteroidetes: the food connection. Front. Microbiol. 2 (MAY), 93–16. doi:10.3389/fmicb.2011.00093
Valenzuela-Encinas, C., Neria-González, I., Alcántara-Hernández, R. J., Enríquez-Aragón, J. A., Estrada-Alvarado, I., Hernández-Rodríguez, C., et al. (2008). Phylogenetic analysis of the archaeal community in an alkaline-saline soil of the former lake Texcoco (Mexico). Extremophiles 12 (2), 247–254. doi:10.1007/s00792-007-0121-y
Vilas–Boas, J. A., Cardoso, S. J., Senra, M. V. X., Rico, A., and Dias, R. J. P. (2020). Ciliates as model organisms for the ecotoxicological risk assessment of heavy metals: a meta–analysis. Ecotoxicol. Environ. Saf. 199 (May), 110669. doi:10.1016/j.ecoenv.2020.110669
Wang, Q., Lv, R., Rene, E. R., Qi, X., Hao, Q., Du, Y., et al. (2020). Characterization of microbial community and resistance gene (CzcA) shifts in up-flow constructed wetlands-microbial fuel cell treating Zn (II) contaminated wastewater. Bioresour. Technol. 302 (November), 122867. doi:10.1016/j.biortech.2020.122867
Wang, Q. Y., Zhou, D., and Long, C. (2009). Microbial and enzyme properties of apple orchard soil as affected by long-term application of copper fungicide. Soil Biol. Biochem. 41, 1504–1509. doi:10.1016/j.soilbio.2009.04.010
Wang, Z., Xia, P., Gao, M., Ma, K., Deng, Z., Wei, J., et al. (2018). Long-term effects of combined divalent copper and tetracycline on the performance, microbial activity and community in a sequencing batch reactor. Bioresour. Technol. 249 (10), 916–923. doi:10.1016/j.biortech.2017.11.006
Wickham, H., Chang, W., Henry, L., Pedersen, T. L., Takahashi, K., Wilke, C., et al. (2022). Create elegant data visualisations using the grammar of graphics. R package, version 3.4. 0.
Yang, Y., Wang, L., Xiang, F., Zhao, L., and Qiao, Z. (2020). Activated sludge microbial community and treatment performance of wastewater treatment plants in industrial and municipal zones. Int. J. Environ. Res. Public Health 17 (2), 436. doi:10.3390/ijerph17020436
Yu, J., Fan, L., Yang, S., Tang, M., Yang, W., Li, H., et al. (2009). Characterization of copper-resistant Agrobacterium isolated from legume nodule in mining tailings. Bull. Environ. Contam. Toxicol. 82 (3), 354–357. doi:10.1007/s00128-008-9598-z
Zafar, S., Aqil, F., and Ahmad, I. (2007). Metal tolerance and biosorption potential of filamentous fungi isolated from metal contaminated agricultural soil. Bioresour. Technol. 98 (13), 2557–2561. doi:10.1016/j.biortech.2006.09.051
Zhang, H., Feng, J., Chen, S., Li, B., Sekar, R., Zhao, Z., et al. (2018). Disentangling the drivers of diversity and distribution of fungal community composition in wastewater treatment plants across spatial scales. Front. Microbiol. 9, 1291. doi:10.3389/fmicb.2018.01291
Zhao, J., Chen, X., Bao, L., Bao, Z., He, Y., Zhang, Y., et al. (2016). Correlation between microbial diversity and toxicity of sludge treating synthetic wastewater containing 4-chlorophenol in sequencing batch reactors. Chemosphere 153, 138–145. doi:10.1016/j.chemosphere.2016.01.086
Keywords: 16S rRNA, 18S rRNA, heavy metals, bioreactor, activated sludge
Citation: Aceves-Suriano CE, Montoya-Ciriaco N, Hernández-Guzmán M, Hernández-Martínez GR, Navarro-Noya YE, Thalasso F and Dendooven L (2023) Changes in the bacterial and microeukaryotic communities in the bioreactor upon increasing heavy metal concentrations. Front. Environ. Chem. 4:1221820. doi: 10.3389/fenvc.2023.1221820
Received: 12 May 2023; Accepted: 07 August 2023;
Published: 28 August 2023.
Edited by:
Sapana Jadoun, Universidad de Concepción, ChileReviewed by:
Shagufta Jabin, Manav Rachna International Institute of Research and Studies (MRIIRS), IndiaCopyright © 2023 Aceves-Suriano, Montoya-Ciriaco, Hernández-Guzmán, Hernández-Martínez, Navarro-Noya, Thalasso and Dendooven. This is an open-access article distributed under the terms of the Creative Commons Attribution License (CC BY). The use, distribution or reproduction in other forums is permitted, provided the original author(s) and the copyright owner(s) are credited and that the original publication in this journal is cited, in accordance with accepted academic practice. No use, distribution or reproduction is permitted which does not comply with these terms.
*Correspondence: Luc Dendooven, ZGVuZG9vdmVuQG1lLmNvbQ==
Disclaimer: All claims expressed in this article are solely those of the authors and do not necessarily represent those of their affiliated organizations, or those of the publisher, the editors and the reviewers. Any product that may be evaluated in this article or claim that may be made by its manufacturer is not guaranteed or endorsed by the publisher.
Research integrity at Frontiers
Learn more about the work of our research integrity team to safeguard the quality of each article we publish.