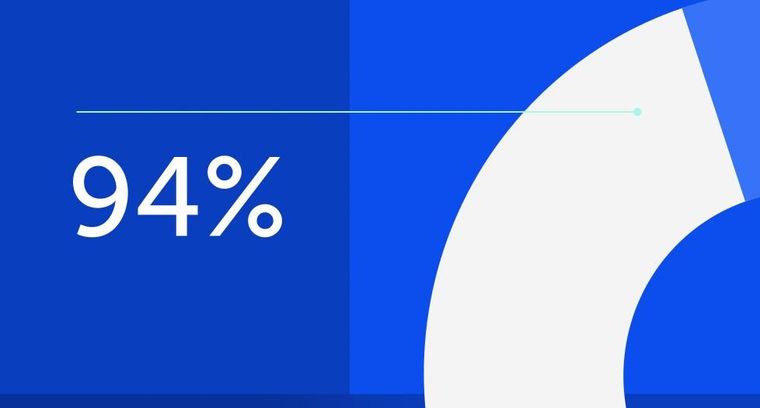
94% of researchers rate our articles as excellent or good
Learn more about the work of our research integrity team to safeguard the quality of each article we publish.
Find out more
REVIEW article
Front. Environ. Chem., 13 June 2022
Sec. Chemical Treatments
Volume 3 - 2022 | https://doi.org/10.3389/fenvc.2022.853764
This article is part of the Research TopicUrban Runoff of Pollutants and Their TreatmentView all 5 articles
Concerns about pollutants in urban stormwater were initially raised in the early 1970s. Australian investigations decades later, also found urban stormwater runoff contained elevated levels of sediment and nutrients, as well as heavy metals, that brought stormwater management to the forefront for regulators. Planning policies were implemented to integrate stormwater management into development in the form of water sensitive urban design (WSUD), also known internationally as low-impact design (LID) and Sustainable Urban Design solutions (SUDs). Since their introduction, comprehensive broad scale field research to verify their success in achieving load reduction targets (LRTs), has been limited. Paucity of field data on the performance of WSUD has prompted organisations to initiate their own locally-specific studies. Limited regulatory guidance on design of monitoring programs has resulted in various methodologies and meta-data recording. This research review collates urban stormwater data from 77 Australian studies, from geographic regions of east coast Australia. The raw dataset in this review included 2,836 events and 4,536 individual results, collected between 1993 and 2021 from local councils, research organisations and water authorities. The review examined total suspended solids (TSS), total phosphorus (TP) and total nitrogen (TN) concentrations, prior to any form of treatment measures as they are the focus of current guidelines and standards for stormwater management. Seminal research, used to inform stormwater guidelines and water quality modelling across Australia, is significantly different (p < 0.001), in this case approximately double the reviewed concentrations. International data is also >20% higher, on average. Geographic location of catchments had the greatest influence on pollutant concentrations, after accounting for the effects of land use and catchment urbanisation date (p < 0.001). Based on the findings of this review, generic load reduction targets (expressed as a percentage of annual inputs, e.g. 80% TSS reduction, 45% TN, 45% TP) typical in current Australian planning regulations, may be sub-optimal in achieving receiving water quality goals, particularly given the difficulty of removing pollutants when present in low concentrations. Alternately, place-based discharge targets which meet, or exceed, background water quality, or ecological and hydrological benchmarks may be a more appropriate tool to achieve environmental objectives.
Data which characterises urban stormwater quality and the influence of catchment-specific attributes is fundamental for cost-effective, sustainable, and strategic stormwater management investment (Reeve et al., 2015; Page et al., 2016). Extensive research was undertaken in Australia for nearly a decade from 1999 to investigate stormwater quality under varying catchment conditions and to develop effective stormwater treatment options for achieving regulatory load reduction targets (LRTs) for sediment and nutrients (Fletcher et al., 2004; Goonetilleke et al., 2005; Taylor et al., 2005). The seminal work by Duncan (1999) collated over 500 data records from international and Australian studies to characterise stormwater quality according to the influence of broad catchment characteristics, such as land use. Data from this early research was used to inform planning and environmental regulation, catchment-based risk assessment frameworks, and predictive stormwater models; including the Model for Urban Stormwater Improvement Conceptualisation (MUSIC) (Wong et al., 2002; Wong et al., 2006; Water by Design, 2019). MUSIC is widely used in Australia, and in some international locations, to predict the average annual load reduction by stormwater quality improvement devices (SQIDs) to meet water quality objectives (WQOs). Compliance with these WQOs is legislated in many planning and environmental protection regulations formulated by State and local Authorities, as part of urban development approvals (State of Queensland, 2017).
Such foundational research has been instrumental to the advancement of urban stormwater management, water sensitive urban design (WSUD) and urban planning in Australia, and more broadly (Duncan, 1999; Wong et al., 2002; Goonetilleke et al., 2005). WSUD is also known internationally as low-impact development (LID) or Sustainable urban Design Systems (SuDS). That research, however, is now over 2 decades old, was based partly on international literature, and noted the lack of Australian data available at the time (Duncan, 1999). The Australian Guidelines for Water Recycling: Managing Health and Environmental Risk–Stormwater Harvesting and Reuse (AGWR-SHR) prepared nearly a decade later, provides a risk management framework for capturing stormwater for non-potable use (National Water Quality Management Strategy, 2009). However, the AGWR-SHR also noted a lack of Australian data available to characterise summary statistics for urban stormwater runoff. Only Eighteen Australian studies were used, with summarised values for 33 of 50 tested water quality parameters, drawn from a refined group of fewer than five studies. Most data for microbial indicators were derived from a single study analysing 59 samples across four Sydney locations (AWQC, 2008). The AGWR-SHR also found reporting on catchment and sample characteristics, such as land use and type of stormwater flow, lacking across several studies. These disparities led to reduced confidence from the authors in the summary statistics provided in the AGWR-SHR (National Water Quality Management Strategy, 2009).
The lack of published stormwater quality data reflects wider challenges when combining and comparing results between and within catchments, such as for predictive modelling. Planning and modelling guidelines across Australia typically require urban development proposals to demonstrate compliance with relevant load reduction targets (LRTs) using predictive models based on generic urban stormwater quality input concentrations when suitable local data is not available (WBD, 2010; WBD, 2017; Melbourne Water, 2018). Recent publications of South-east Queensland studies suggested that urban stormwater runoff had lower concentrations than those specified by current guidelines (Nichols and Lucke, 2016; Lucke et al., 2018). Although a conservative approach to stormwater treatment can be justified when local stormwater quality is unknown, where pollutant concentrations are much lower than reported in the aforementioned guidelines, this can have substantial implications for cost-effective stormwater management. Studies of constructed wetlands, for example, have found that load reduction performance is correlated to inlet concentration (Chen et al., 2015; Land et al., 2016). Any treatment measures must therefore be significantly larger, or modified to achieve the LRTs. After 20 years of emerging evidence of a discrepancy between stormwater concentrations specified in current Australian stormwater management guidelines and measured water quality in some regions, a wider collation and analysis of contemporary data characterising stormwater pollutants across Australia was considered necessary.
A recent meta-analysis by Simpson et al. (2022) investigated the influence of landuse, climate and imperviousness on urban stormwater quality and incorporated data from 360 catchments worldwide. Their review also reported lower average concentrations for total suspended solids and total phosphorus than those adopted in current Australian stormwater guidelines.
This review had three objectives; firstly, to characterise the typical concentrations of total suspended solids (TSS), total phosphorus (TP) and total nitrogen (TN) in untreated urban stormwater across eastern Australia using an expanded database. Secondly, to analyse differences between these concentrations and those reported in current Australian and international stormwater data. Finally, to investigate the potential impacts of land use, geographic location, and catchment urbanisation date on concentrations of TSS, TP and TN in untreated urban stormwater. At the commencement of this review, it was hypothesised that the concentrations of TSS, TP and TN in untreated urban stormwater would be lower than those reported by earlier publications (Duncan, 1999; National Water Quality Management Strategy, 2009). Concentrations of TSS, TP and TN were also hypothesised to increase as urbanised catchments age.
This review did not engage in a detailed evaluation of the validity of the sample collection process, analytical variability possible between different laboratories, accuracy of the land-use classification of the monitored catchment, or other filtering of data that may have occurred by the data owners. As a result, variability was anticipated in the dataset. The review did not investigate the influence of rain intensity on TSS, TP and TN. The focus of this study was to summarise data in terms of central values (i.e. means and medians) across datasets for multiple datasets that cover a broad range of rain intensities, geographic locations and climatic regions.
Stormwater quality data was aggregated by collating the results of water monitoring programs from research institutions, government organisations and environmental consultants across Australia following a request to share available data. Snowball sampling (Patton, 2015) was used to identify potential contributors as stormwater quality data was found to be largely unavailable in published literature and held by local government or in restricted access publications. Data contributors were identified along the urban coastal regions of eastern Australia, from north Queensland to Melbourne, Victoria. A small number of studies were identified from Western Australia and South Australia, however, were excluded due to either the potential influence of groundwater or failing inclusion criteria described below. Data contributors were kept anonymous to preserve data privacy, except where included in the acknowledgements. Unless requests were made to address data privacy concerns, the research hypotheses of the review were not revealed, to minimise bias. Contributing organisations typically provided an aggregation of stormwater quality results across multiple locations and time periods.
The inclusion criteria for the study were;
• TSS, TP and TN concentrations from untreated urban stormwater; and
• Catchments with at least 85% urban land use, of which <25% is open space (parkland).
Typical sampling locations included urban road catchments, roadside gutters adjacent to stormwater collection devices, stormwater drains, concrete and natural stream channels, and culverts. Samples were collected between 1993 and 2021 from sites upstream of treatment measures (i.e. untreated). Catchments ranged from single use developments to multi-use urban areas (0.3–1120 ha). Figure 1 provides an example of one of the sampled catchments in south-east Queensland. Sites across Greater Melbourne and NSW included urban streams and disturbed waterways.
FIGURE 1. Road catchment from Southeast Queensland (Source: Lucke et al., 2018).
Water quality data for 2,836 sampling events and 4,536 individual data points were provided by various organisations. The data included dry weather samples as well as rain event samples. Supplied data also included a range of pollutant parameters and nutrient forms, however, for this review it was standardised to concentrations of TSS, TP and TN (mg/L), as these were available for all locations and >99% of events. Collection of samples occurred across various parts of the rain event, using a variety of methods including discrete grab samples and flow-weighted composites. Variation in the reporting of sampling methods meant it was not possible to comprehensively separate them. Event mean concentrations (EMC) were calculated, by the contributing organisation, where multiple samples were taken from a single location during a defined rainfall event. Where the meta-data provided clear identification between rain event and routine monitoring, dry weather samples were removed since the review was targeting event data only. Concentrations less than the limit of reporting were replaced with the minimum detection limit to avoid artificially reducing event concentration values and biasing the summary metrics.
Comprehensive predictor variables related to climate, catchment characteristics, runoff, and rainfall conditions were not able to be calculated due to significant variation in the reporting of information between data providers. However, temporal satellite imagery (Google Maps) was used in conjunction with documents provided by the data contributors to discern sampling locations (eg. roadside gutter, stormwater drain, channel, culvert), data suitability, year of sample collection, catchment urbanisation date, land use of the largest proportion of land surface in the catchment (residential, commercial, industrial), and geographic region (regional Queensland, south-east Queensland, greater Melbourne, greater Sydney). Broad regions were used in place of exact locations to preserve data privacy. The catchment urbanisation date was estimated as the number of years since the catchment was converted from non-urban land uses, such as natural vegetation or agricultural land, to urban land uses. Discrete decadal groups (>21 years, 11–20 years, <10 years) were used to categorise urbanisation because the exact year some older catchments were urbanised, was not well recorded. An analysis of relationships between predictors found collinearity between the year of sample collection and geographic location. The year of sample collection was subsequently removed from further analysis. All samples were considered valid, and outliers identified with a Grubb’s outlier test were removed only after this validation process. Following the data refinement process, a final dataset of 2,823 samples remained.
Initial inspection of the data revealed positively skewed distributions for all pollutants and landuse types, as presented in Figure 2, and is typical of environmental data (Blackwood, 1992). Datasets were subsequently log-transformed to check if the data subsequently followed a normal distribution. Residual vs. Fitted plots and Normal Q-Q plots showed that log-transformation of the parameters substantially improved model fits however, Shapiro-Wilk normality tests confirmed that all datasets were non-parametric.
Wilcoxon, Mann-Whitney, non-parametric tests were used to compare differences in the concentrations of TSS, TP and TN between our Australian dataset and those reported by other publications (Simpson et al., 2022; Duncan, 1999; Brisbane City Council, 2004; National Water Quality Management Strategy, 2009).
The influence of catchment urbanisation date, geographic location, and land use on concentrations of TN, TP and TSS was analysed using generalised linear modelling (GLM). The GLM was considered advantageous over other statistical tests as it allowed for modelling of non-linear effects from non-normal sample distributions, as is typical with stormwater quality data. Maximal models for log-transformed TN, TP and TSS data were constructed by calculating main effects for all available predictors (catchment urbanisation date, land use, geographic location). Cases with missing values were removed. Models were then simplified to derive minimum adequate models, which retain only those predictors which have the most significant predictive value without substantially reducing the model’s overall predictive accuracy. This was useful for determining which predictors most influenced concentrations of TN, TP and TSS. The minimum adequate models were derived using a stepwise algorithm to repeatedly drop model terms, and inspect the impact of model simplification on goodness of fit using Akaike’s information criterion (AIC); which ranks the quality of alternative statistical models fitted by maximum likelihood: AIC = 2log likelihood + knpar, where npar represents the number of parameters in the fitted model and k = 2 (Sakamoto and Ishiguro, 1986). Model diagnostics indicated low bias as residual variance was approximately equivalent across predicted values of TN, TP and TSS, with residual medians close to 0. The proportion of deviance in each nutrient group explained by the minimum adequate models was calculated as 1 - (model deviance/model null.deviance); which can be considered an equivalent measure of the coefficient of determination.
The final dataset comprised 2,823 samples of untreated urban stormwater collected between 1993 and 2021. Samples were collected from 77 locations along the urban coastal regions of eastern Australia, from north Queensland to Melbourne, Victoria. The median number of samples collected per site was 38. Concentrations of TSS, TP and TN were highly variable and positively skewed. The separation of data according to broad catchment characteristics found catchments urbanised >21 years ago had greater median nutrient concentrations compared to catchments urbanised 11–20 and <10 years ago. Industrial land uses had greater TSS and TN concentrations when compared to residential and commercial land uses, however, commercial catchments had higher mean TP concentrations. Summary statistics for TSS, TP and TN are presented in Table 1, and compares the current review data with historical data from Australian and international publications.
TABLE 1. Comparison of Total Suspended Solids (TSS), Total Phosphorus (TP) and Total Nitrogen (TN) Statistics for Stormwater from Urban Residential, Industrial and Commercial Land Uses for the current dataset, historical Australian and International datasets.
Mean TSS concentrations in urban residential catchments were 60% and 40% lower respectively in the current review when compared with Australian and international data. Nutrient concentrations in urban residential were also lower with mean TP 35% and 42% and mean TN 35% and 30% lower respectively than Australian and international urban data. Whilst mean concentrations of TSS, TP and TN in the current data set were still below those observed in the Australian and international data sets the variance percentage was less for commercial and industrial catchments. This may be in part related to changes in urban planning and form occurring at a different rate in residential precincts compared to commercial and industrial precincts. Table 2 presents the pollutant data in broad geographic regions, landuse and approximate date since development was completed.
TABLE 2. Concentrations of TSS, TP, TN in untreated urban stormwater across broad catchment characteristics (not log-transformed data mg/L).
Despite log-transforming the current datasets, statistical tests failed to confirm normality assumptions required for comparison with Students t tests. Wilcoxon-Mann-Whitney, non-parameteric evaluation of the datasets grouped by landuse confirmed that the TSS, TP and TN concentrations from urban residential, commercial and industrial landuses are significantly different to each other, and the international and historical Australian data (α = 0.1).
Generalised linear modelling found the geographic location of catchments had the greatest influence on concentrations of TN, TP and TSS (p < 0.001) after also accounting for the effects of landuse and catchment urbanisation date. Catchments located across the Sydney region had the greatest median concentrations of TSS, TP and TN (Figures 3–5). Both geographic location and land use were key predictors for TSS, where industrial land uses had significantly greater TSS than residential and commercial land uses (p = 0.017). Geographic locations are shown indicatively on the Box and Whisker plots with site codes to protect confidentiality of the data source. The catchment urbanisation date did not significantly influence concentrations of TN (p = 0.675), TP (p = 0.318) and TSS (p = 0.243); showing that the age of urban catchments was not a significant factor when also accounting for geographic location.
FIGURE 3. Summary urban residential landuse TSS, TP and TN concentrations for each site by geographic region, with typical values shown in red (WbD, 2018).
FIGURE 4. Summary commercial landuse TSS, TP and TN concentrations for each site by geographic region, with typical values shown in red (WbD, 2018).
FIGURE 5. Summary industrial landuse TSS, TP and TN concentrations for each site by geographic region, with typical values shown in red (WbD, 2018).
Consistent with recent localised studies (Nichols and Lucke, 2016; Lucke et al., 2018), our expanded analysis across eastern Australia found significantly lower concentrations of TSS, TP and TN in urban stormwater than those presented by earlier Australian reviews and used in predictive models such as MUSIC (Duncan, 1999; National Water Quality Management Strategy, 2009). After accounting for the effects of catchment urbanisation date, and land use, we found that the geographic location of catchments had the greatest influence on concentrations of TSS, TP and TN. Detailed interrogation of the data suggests that this is likely due to high nutrient and sediment concentrations collected across Sydney catchments during the early 1990s. The NSW State government subsequently invested AUD$60 million in stormwater improvement projects through the Stormwater Trust from 1997 (https://www.environment.nsw.gov.au/resources/stormwater/usp/uspeesfdlow.pdf). Improved urban stormwater quality is also expected when urban fertiliser use is reduced, sewerage infrastructure is upgraded, wastewater treatment plant discharges are ceased, and land use changes from intensive agriculture (Wicke et al., 2012; Panasiuk et al., 2015; Wijesiri et al., 2015; Lucke et al., 2018). These changes are likely to occur independently of the catchment urbanisation date, which was not a significant predictor of TSS, TP and TN in this review. An alternate explanation could be that the sampling methods, location, sample preparation, preservation, selected laboratory analysis or accuracy of concentration reporting/recording, tied to the data provider, could be influencing the outcome. It was not possible to determine this level of detail from the supplied datasets alone.
Concentrations of TN, TP and TSS in this review were observed to be highly variable between catchments, even those of similar landuse. The complex interplay between pollutant sources, rainfall, landuse, stormwater drainage, nature and age of wastewater infrastructure typically produces variable stormwater quality (e.g. Liu et al., 2013; Tondera et al., 2018b). For example, variability in rain intensity alters the kinetic energy of runoff between and within catchments, which leads to effects such as first flush, peak flow, interflow, and differential pollutant wash off (Taylor et al., 2005; Tondera et al., 2018a). This presents significant challenges for the generalisation of results between catchments and across temporal scales, as is desirable with predictive stormwater modelling. Such high variability of these environmental parameters also confirms observations that high pollutant concentrations occasionally occur in urban stormwater, skewing arithmetic means higher than might be truly representative. This reinforces the need for locally relevant, comprehensive, ongoing stormwater monitoring and widespread infrastructure to better manage stormwater before discharging to waterways. Harvesting and re-use projects, and projects discharging to high value ecosystems, may warrant a more conservative WQO or treatment concept that reflects frequent, lower concentrations but can accommodate rare, elevated levels.
The relatively low variability in TSS, TP and TN explained by the minimum adequate models show that factors beyond the broad characteristics analysed in this review influence stormwater quality. For example, the spatial layout of nutrient sources and impervious surfaces interact with rainfall intensity, duration, and antecedent dry days to influence stormwater volume, velocity and quality, beyond that typically observed when only considering landuse (Liu et al., 2012; Liu et al., 2013). Likewise, the millennium drought, which affected eastern Australia between 1997–2009, may have influenced stormwater quality and the results forming part of this review. This effect was not analysed due to co-linearity between the geographic location of catchments, year of sample collection and period of the millennium drought.
Differences in the reporting of climatic, catchment and sampling characteristics between data providers limited the number of detailed predictor variables which could be reliably generated from the data. However, many of the publications summarised by the foundational reviews also lacked comprehensive information regarding such factors known to influence stormwater quality (Duncan, 1999; National Water Quality Management Strategy, 2009). Recent meta-analysis of urban stormwater data concluded that impervious percentage was not a significant influence on pollutant concentration, but that climate and landuse were the most influential, and that regional location had a statistically significant influence on nutrients and metal levels (Simpson et al., 2022).
Other researchers have also noted difficulties identifying whether previous studies undertook sampling of untreated, mixed or treated stormwater (Reeve et al., 2015; Nichols and Lucke, 2016). Subsequently, data is aggregated into general categories, such as broad landuse, which could mask the impact of more detailed influences on stormwater quality. For example, stormwater quality data sets often vary insource flow (untreated, mixed and treated stormwater) and catchment types, the use of grab or automated sampling techniques; collection time intervals during stormflow, and number of locations within a catchment (Goonetilleke and Lampard, 2019). The advancement of cost-effective, sustainable, and fit-for-purpose stormwater management requires a detailed understanding of how such factors influence stormwater quality (Reeve et al., 2015). There is an evident need for standardised collection and reporting of comprehensive sampling methods, catchment landuse, and rainfall characteristics to be included in urban stormwater monitoring protocols, as well as regular updates to stormwater quality over longer climatic timeframes.
The results of this review further reinforce that nutrient and sediment concentrations in untreated urban stormwater are typically lower than reported in earlier influential studies and predictive models currently used for stormwater management, such as MUSIC. This highlights the possibility that, where in most cases default guideline values are adopted due to a lack of suitable local data, stormwater management model predictions of WSUD performance across many urban catchments in eastern Australia are over-estimating local conditions. This could be compromising the cost effectiveness of treatment and the potential for varied reuse options. For example, planning guidelines typically require development proposals to model how treatment devices will achieve WQO’s using predictive modelling. The Queensland State Planning Policy set southeast Queensland pollutant reduction targets at 80% for TSS, 60% for TP and 45% for TN (State of Queensland, 2017). Yet the achievement of these WQO’s through the implementation of SQID’s can become problematic when pollutant concentrations in urban stormwater are already low prior to treatment, since the pollutant concentration available for treatment removal is limited. Attempts to achieve generic LRT’s may lead to large footprint, and costly “treatment trains,” limiting potential reuse options for stormwater with low pollutant concentrations, where the focus could instead be on ecological or hydrological benchmarks.
A conservative approach to stormwater treatment can be justified when local concentrations are unknown. However, the outcomes of this review and other international meta-analysis, emphasise the importance of recent and locally-acquired stormwater quality data obtained from ongoing water monitoring programs (Simpson et al., 2022). Such recommendations are consistent with the MUSIC user guide (eWater, 2019) and regional modelling guidelines (Melbourne Water, 2018).
Results of this review raise questions regarding generic percent LRTs specified in planning regulations due to the difficulty of removing stormwater pollutants at low concentrations with WSUD. A re-assessment of guideline values and WQO’s would ensure data is representative of typical stormwater quality across urban catchments of eastern Australia. Discharge targets which meet, specific concentrations may be more suitable than percent load reduction targets (e.g. EPA Environment Protection Authority Victoria, 2019) to promote fit-for-purpose and cost-effective stormwater management without compromising water quality. Adopting this approach would likely require initiating or augmenting local water monitoring programs to adequately identify pollutant concentrations across landuses, catchments and corresponding waterways. To facilitate this, development of monitoring guidelines are recommended for targeted catchment sampling programs to determine concentrations for particular landuses during and between events. The authors suggest that these guidelines include as a minimum: sampling techniques, minimum number of samples/events, standard analytes, meta-data required. This meta-data should include: sampling method, site location, soil type, landuse type, impervious percentage, Limit of detection, area of upstream catchment, time and date of sample and year of development.
Extensive research undertaken in Australia in the decade from 1990 to 2000, was instrumental to the advancement of urban stormwater quality management, yet it acknowledged a lack of available Australian data. Recently published data in Southeast Queensland indicated that pollutant concentrations in urban stormwater are substantially lower than previously reported. The results presented in this review of 2,823 rainfall events captured from sites ranging from Melbourne, Victoria, to North Queensland, provide further confirmation of typically lower TSS, TP and TN concentrations in urban stormwater runoff.
The following conclusions were drawn;
1. Summary statistics of TSS (
2. For commercial catchments, summary statistics of TSS (
3. Industrial catchments were observed with summary data of TSS (
4. The evaluated dataset indicate that urban stormwater quality concentrations and LRTs used in many predictive models and regulatory policies may be over-estimating actual outcomes across the eastern Australian urban catchments considered, and emphasises the importance of up-to-date, locally relevant data.
5. Percent LRTs specified in planning regulations and policies may be a blunt tool, that may be better nuanced with local (background) water quality and ecological health or hydrological criteria.
6. Comparative understanding of urban stormwater quality across catchments is limited by variances in monitoring and meta-data collection methodologies. Initiation or augmentation of existing regional water monitoring programs to adequately identify pollutant concentrations across catchments and corresponding waterways is recommended. Guidelines should include: sampling techniques, minimum number of samples/events, standard analytes, meta-data required. Meta-data should include: sampling method, site location, soil type, landuse type, impervious percentage, limit of detection, area of upstream catchment, time and date of sample, year of development.
All authors contributed to the preparation of the manuscript. KO performed the statistical evaluations of the dataset.
Author DD is employed by Drapper Environmental Consultants. Author TM is employed by Water Technology. Author RC is employed by Melbourne Water.
The remaining authors declare that the review was conducted in the absence of any commercial or financial relationships that could be construed as a potential conflict of interest.
The handling editor PE declared a past collaboration with the author DD.
All claims expressed in this article are solely those of the authors and do not necessarily represent those of their affiliated organizations, or those of the publisher, the editors and the reviewers. Any product that may be evaluated in this article, or claim that may be made by its manufacturer, is not guaranteed or endorsed by the publisher.
The authors would like to acknowledge the CRC for Water Sensitive Cities, Drapper Environmental Consultants, Melbourne Water, Queensland Urban Utilities, SPEL Stormwater, Stockland, Sydney Water, Townsville City Council, and Water Technology for providing data used in this study.
AWQC, (2008). “Pathogens in Stormwater,” in NSW Department of Environment and Climate Change and the Sydney Metropolitan Catchment Management Authority. Editor P. MONIS.
Blackwood, L. G. (1992). The Lognormal Distribution, Environmental Data, and Radiological Monitoring. Environ. Monit. Assess. 21, 193–210. doi:10.1007/BF00399687
Environment Protection Authority (EPA) Victoria, (2019). State Environment Protection policY (SEPP Waters). Melbourne, Victoria: EPA Victoria.
Fletcher, T., Duncan, H., Poelsma, P., and Lloyd, S. (2004). Stormwater Flow and Quality and the Effectiveness of Non-proprietary Stormwater Treatment Measures: A Review and Gap Analysis. Monash University, Cooperative Research Centre for Catchment Hydrology.
Goonetilleke, A., and Lampard, J.-L. (2019). “Stormwater Quality, Pollutant Sources, Processes, and Treatment Options,” in Approaches to Water Sensitive Urban Design (Elsevier), 49–74. doi:10.1016/B978-0-12-812843-5.00003-4
Goonetilleke, A., Thomas, E., Ginn, S., and Gilbert, D. (2005). Understanding the Role of Land Use in Urban Stormwater Quality Management. J. Environ. Manag. 74, 31–42. doi:10.1016/j.jenvman.2004.08.006
Land, M., Granéli, W., Grimvall, A., Hoffmann, C. C., Mitsch, W. J., Tonderski, K. S., et al. (2016). How Effective Are Created or Restored Freshwater Wetlands for Nitrogen and Phosphorus Removal? A Systematic Review. Environ. Evid. 5, 9. doi:10.1186/s13750-016-0060-0
Liu, A., Egodawatta, P., Guan, Y., and Goonetilleke, A. (2013). Influence of Rainfall and Catchment Characteristics on Urban Stormwater Quality. Sci. Total Environ. 444, 255–262. doi:10.1016/j.scitotenv.2012.11.053
Liu, A., Goonetilleke, A., and Egodawatta, P. (2012). Inherent Errors in Pollutant Build-Up Estimation in Considering Urban Land Use as a Lumped Parameter. J. Environ. Qual. 41, 1690–1694. doi:10.2134/jeq2011.0419
Lucke, T., Drapper, D., and Hornbuckle, A. (2018). Urban Stormwater Characterisation and Nitrogen Composition from Lot-Scale Catchments - New Management Implications. Sci. Total Environ. 619-620, 65–71. doi:10.1016/j.scitotenv.2017.11.105
Melbourne Water (2018). MUSIC Guidelines: Input Parameters and Modelling Approaches for MUSIC Users in Melbroune Water's Service Area. Melbourne, Victoria: Melbourne Water.
National Water Quality Management Strategy (2009). Australian Guidelines for Water Recycling: Managing Health and Environmental Risks (Phase 2)—stormwater Harvesting and Reuse. Canberra: Australia Biotext.
Nichols, P., and Lucke, T. (2016). Field Evaluation of the Nutrient Removal Performance of a Gross Pollutant Trap (GPT) in Australia. Sustainability 8, 669. doi:10.3390/su8070669
Page, D., Vanderzalm, J., Dillon, P., Gonzalez, D., and Barry, K. (2016). Stormwater Quality Review to Evaluate Treatment for Drinking Water Supply via Managed Aquifer Recharge. Water Air Soil Pollut. 227, 322. doi:10.1007/s11270-016-3021-x
Panasiuk, O., Hedström, A., Marsalek, J., Ashley, R. M., and Viklander, M. (2015). Contamination of Stormwater by Wastewater: A Review of Detection Methods. J. Environ. Manag. 152, 241–250. doi:10.1016/j.jenvman.2015.01.050
Patton, M. Q. (2015). Qualitative Research and Evaluation Methods. 4th edn. Thousand Oaks, CA: Sage.
Reeve, P., Monis, P., Lau, M., Reid, K., Van Den Akker, B., Humpage, A., et al. (2015). Quantifying Water Quality Characteristics of Stormwater: Assessment of Untreated Stormwater for the Adelaide Airport and Barker Inlet Stormwater-Aquifer Storage and Recovery Recycled Water Schemes. Water J. Aust. Water Assoc. 42, 73.
Sakamoto, Y., and Ishiguro, M. (1986). Akaike Information Criterion Statistics. Dordrecht, Holland: Reidel.
Simpson, I. M., Winston, R. J., and Brooker, M. R. (2022). Effects of Land Use, Climate, and Imperviousness on Urban Stormwater Quality: A Meta-Analysis. Sci. Tot. Env. 809, 152206. doi:10.1016/j.scitotenv.2021.152206
State of Queensland (2017). State Planning Policy. Brisbane, Queensland: Department of Infrastructure, Local Government and Planning, Queensland Government.
Taylor, G. D., Fletcher, T. D., Wong, T. H. F., Breen, P. F., and Duncan, H. P. (2005). Nitrogen Composition in Urban Runoff-Implications for Stormwater Management. Water Res. 39, 1982–1989. doi:10.1016/j.watres.2005.03.022
Tondera, K., Blecken, G.-T., Chazarenc, F., and Tanner, C. C. (2018a). Ecotechnologies for the Treatment of Variable Stormwater and Wastewater Flows. Springer.
Tondera, K., Blecken, G.-T., Tournebize, J., Mander, Ü., and Tanner, C. C. (2018b). “Nutrient Removal from Variable Stormwater Flows,” in Ecotechnologies for the Treatment of Variable Stormwater and Wastewater Flows (Springer). doi:10.1007/978-3-319-70013-7_3
Wicke, D., Cochrane, T. A., and O'sullivan, A. (2012). Build-up Dynamics of Heavy Metals Deposited on Impermeable Urban Surfaces. J. Environ. Manag. 113, 347–354. doi:10.1016/j.jenvman.2012.09.005
Wijesiri, B., Egodawatta, P., Mcgree, J., and Goonetilleke, A. (2015). Influence of Pollutant Build-Up on Variability in Wash-Off from Urban Road Surfaces. Sci. Total Environ. 527-528, 344–350. doi:10.1016/j.scitotenv.2015.04.093
Wong, T. H. F., Fletcher, T. D., Duncan, H. P., and Jenkins, G. A. (2006). Modelling Urban Stormwater Treatment-A Unified Approach. Ecol. Eng. 27, 58–70. doi:10.1016/j.ecoleng.2005.10.014
Keywords: concentration targets, integrated stormwater management, pollutant load reduction, urban stormwater, water sensitive urban design
Citation: Drapper D, Olive K, McAlister T, Coleman R and Lampard J-L (2022) A Review of Pollutant Concentrations in Urban Stormwater Across Eastern Australia, After 20 Years. Front. Environ. Chem. 3:853764. doi: 10.3389/fenvc.2022.853764
Received: 13 January 2022; Accepted: 25 May 2022;
Published: 13 June 2022.
Edited by:
Prasanna Egodawatta, Queensland University of Technology, AustraliaCopyright © 2022 Drapper, Olive, McAlister, Coleman and Lampard. This is an open-access article distributed under the terms of the Creative Commons Attribution License (CC BY). The use, distribution or reproduction in other forums is permitted, provided the original author(s) and the copyright owner(s) are credited and that the original publication in this journal is cited, in accordance with accepted academic practice. No use, distribution or reproduction is permitted which does not comply with these terms.
*Correspondence: Darren Drapper, ZGFycmVuQGRyYXBwZXJjb25zdWx0YW50cy5jb20=
Disclaimer: All claims expressed in this article are solely those of the authors and do not necessarily represent those of their affiliated organizations, or those of the publisher, the editors and the reviewers. Any product that may be evaluated in this article or claim that may be made by its manufacturer is not guaranteed or endorsed by the publisher.
Research integrity at Frontiers
Learn more about the work of our research integrity team to safeguard the quality of each article we publish.