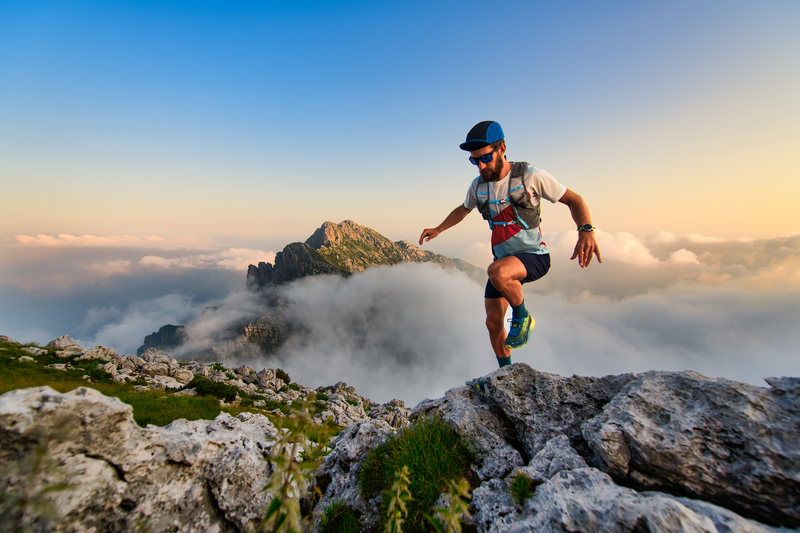
95% of researchers rate our articles as excellent or good
Learn more about the work of our research integrity team to safeguard the quality of each article we publish.
Find out more
EDITORIAL article
Front. Energy Res. , 07 February 2025
Sec. Energy Storage
Volume 13 - 2025 | https://doi.org/10.3389/fenrg.2025.1558766
This article is part of the Research Topic Full Lifecycle Management of Battery Energy Storage Systems View all 6 articles
Editorial on the Research Topic
Full lifecycle management of battery energy storage systems
Rechargeable battery systems have seen tremendous growth in recent years, driven due to rapid uptake of hybrid and electric vehicles (EV) as well as other portable electronic applications. This is set to continue with the electrification of transportation in general. Owing to the tremendous volumes of battery systems being produced and projected, rigorous life cycle management techniques are becoming more important. Stationary energy storage systems are seen as probable second use of retired automotive battery backs. For safe and effective re-use of batteries new technologies need to be implemented to ensure accurate understanding of important parameters such as residual energy capacity and state of health (SOH) to indicate duration to complete end of life. In re-use, thermal runaway is also an important challenge and the ability to accurately determine this risk is important. In addition to such diagnostic methods, the environmental impact of the battery through the different phases of its life cycle needs to be carefully evaluated.
To capture the latest developments in this important area the Research Topic entitled “Full Lifecycle Management of Battery Energy Storage Systems” was selected. Lifecycle management of batteries is a multi-disciplinary field, and hence a broad range of areas are relevant such as artificial intelligence, design, materials, electrochemistry, power electronics, thermal and mechanical technologies, and recycling. For this Research Topic, five original research articles covering some aspects of these topics were selected for publication. Four of the five papers utilize a range of data-driven approaches highlighting the importance of this rapidly growing field to the full life cycle management of battery energy storage systems.
In Melzack et al.’s work they explored the environmental impact of different dual energy-storage systems (DESS) in the cradle to gate part (from raw materials to leaving the production line) of the electric vehicle batteries life cycle. Instead of using a single lithium-ion battery pack, the study explored the use of hybrid solutions including supercapacitors and newer technologies such as aqueous Al-ion cells to deliver the high-power component required for electric vehicles. Such dual systems are expected to increase the lifetime of the battery backs. Lifecycle assessments (LCA) for lithium-ion batteries have been undertaken in the past but few studies have been completed for these dual systems. Additionally, this investigation wanted to explore the environmental impact during the production stage of the DESS rather than over the operating lifetime. The study found that DESS can extend the lifetime of battery packs as well providing beneficial the environmental impacts using supercapacitors, as compared to pure lithium ion-based packs. This was not the case for aqueous Al-ion technology used in DESS, which was partially due to these cells being lab-based and commercial data is not yet available for this early technology. Such LCA studies are important as they provide a holistic view taking into consideration sustainability into all aspects of the battery’s life.
Batteries can no longer be designed for single use in electric vehicles, rather future re-use needs to be considered to cover all aspects of its actual lifecycle. Thermal runaway can cause catastrophic failure in EVs and particularly in the secondary use of retired batteries, where it has a high probability of onset. In this collection Chen et al., devised an early warning data-driven approach for the thermal runaway. This study adopted an unsupervised learning approach employing ensemble learning and a reconstruction error calculation, which avoided the challenges of limited data sets which the supervised learning techniques face. In addition, a bidirectional long short-term memory (Bi-LSTM) network with an attention mechanism (AM) is used to extract the time-varying characteristics of lithium battery data. The technique was successfully implemented on data from retired batteries.
SOH estimation is crucial for the full lifecycle management of batteries, particularly in the re-use scenarios. Data-driven approaches are becoming increasingly important and two papers in this collection outline novel data-driven approaches for SOH estimation. Wang et al. develop a SOH estimation method that integrates data-driven model and signal analysis method. Specifically, the differential thermal voltammetry (DTV) analysis method is used to analyze aging characteristics to obtain features strongly related to battery aging, and solve the problem of poor interpretability of data-driven methods. A data-driven model is constructed with the Bi-LSTM as the core, and the AM is added to focus on important parts of the sequence to further improve the accuracy of the model. The proposed method is validated via the Oxford battery degradation dataset, and the results show that the proposed method achieves high accuracy in SOH estimation with a root mean square error (RMSE) maintained at about 0.4%. Zou et al. describe a data-driven method for SOH estimation leveraging the Bi-LSTM that uses the DTV analysis to extract features, and incorporates AM at both temporal and spatial scales to enable the model focusing on important information in the features. The validation using the Oxford Battery degradation Dataset shows that the proposed method achieves high accuracy in SOH estimation, with the RMSE of around 0.4%.
Another important pillar of battery life cycle management is to be able to accurately determine remaining capacity. In this collection Guo et al. propose a novel approach using multi-voltage-interval incremental capacity (IC) peaks combined with a back-propagation neural network (BPNN) for battery capacity estimation. Multiple voltage intervals covering relatively narrow and wide values are applied for computing IC curves to enhance the estimation robustness, removing the need to employ smoothing algorithms. A BPNN is then applied to approximate the correlation between multi-voltage-interval IC peak and capacity. Besides, a five-point moving window technique is proposed to capture multi-voltage-interval IC peaks online effectively.
This Research Topic brings together pioneering research that integrates emerging techniques, such as artificial intelligence, and battery physics to enhance the SOH estimation, capacity evaluation and thermal runaway early warning techniques for lithium-ion batteries. These data-driven approaches advance battery health understanding and degradation/failure mitigation strategies, enabling an intelligent full lifecycle management of batteries, ultimately contributing to a sustainable, zero pollution future.
AM: Supervision, Writing–original draft, Writing–review and editing. XL: Writing–review and editing. BS: Writing–review and editing. EH: Writing–review and editing. HR: Writing–original draft, Writing–review and editing.
The author(s) declare that no financial support was received for the research, authorship, and/or publication of this article.
The authors declare that the research was conducted in the absence of any commercial or financial relationships that could be construed as a potential conflict of interest.
The author(s) declare that no Generative AI was used in the creation of this manuscript.
All claims expressed in this article are solely those of the authors and do not necessarily represent those of their affiliated organizations, or those of the publisher, the editors and the reviewers. Any product that may be evaluated in this article, or claim that may be made by its manufacturer, is not guaranteed or endorsed by the publisher.
Keywords: battery management, artificial intelligence, operation optimizations, re-utilization, energy storage systems, data-driven methods
Citation: Mumtaz A, Liu X, Sun B, Hosseinzadeh E and Ruan H (2025) Editorial: Full lifecycle management of battery energy storage systems. Front. Energy Res. 13:1558766. doi: 10.3389/fenrg.2025.1558766
Received: 10 January 2025; Accepted: 17 January 2025;
Published: 07 February 2025.
Edited and reviewed by:
Kui Jiao, Tianjin University, ChinaCopyright © 2025 Mumtaz, Liu, Sun, Hosseinzadeh and Ruan. This is an open-access article distributed under the terms of the Creative Commons Attribution License (CC BY). The use, distribution or reproduction in other forums is permitted, provided the original author(s) and the copyright owner(s) are credited and that the original publication in this journal is cited, in accordance with accepted academic practice. No use, distribution or reproduction is permitted which does not comply with these terms.
*Correspondence: Haijun Ruan, YWUxNjcyQGNvdmVudHJ5LmFjLnVr
Disclaimer: All claims expressed in this article are solely those of the authors and do not necessarily represent those of their affiliated organizations, or those of the publisher, the editors and the reviewers. Any product that may be evaluated in this article or claim that may be made by its manufacturer is not guaranteed or endorsed by the publisher.
Research integrity at Frontiers
Learn more about the work of our research integrity team to safeguard the quality of each article we publish.