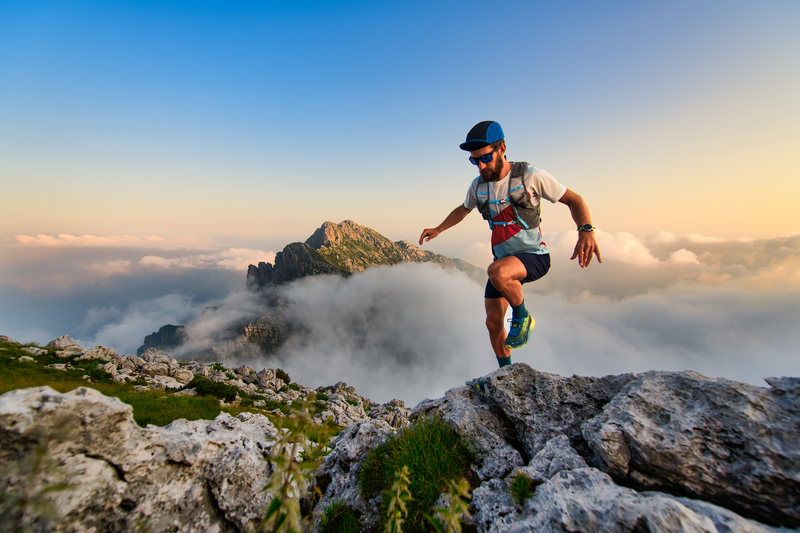
94% of researchers rate our articles as excellent or good
Learn more about the work of our research integrity team to safeguard the quality of each article we publish.
Find out more
ORIGINAL RESEARCH article
Front. Energy Res.
Sec. Smart Grids
Volume 13 - 2025 | doi: 10.3389/fenrg.2025.1504972
This article is part of the Research Topic Advancing Demand Response in Renewable Smart Grid for a Sustainable Future View all 7 articles
The final, formatted version of the article will be published soon.
You have multiple emails registered with Frontiers:
Please enter your email address:
If you already have an account, please login
You don't have a Frontiers account ? You can register here
This research investigates the economic and environmental viability of a combined renewable energy system that incorporates solar photovoltaic, wind, and biomass power production, with diesel generators and battery storage serving as backup options. The system is designed to optimize energy costs while ensuring high reliability, lower emissions, and greater renewable energy utilization. Various advanced optimization methods, including Genetic Algorithm, Particle Swarm Optimization, Artificial Bee Colony Optimization, and Teaching-Learning-Based Optimization, are utilized to determine the most efficient system configuration. The combined energy system is evaluated under two operational strategies, load-following and cycle-charging, with a maximum power supply loss probability of 2% to maintain system dependability. The findings indicate that the Teaching-Learning-Based Optimization approach surpasses other methods in identifying the most cost-effective and environmentally friendly solution. This investigation centers on Barwani, a rural district in India, to evaluate the technical and economic feasibility of implementing such a combined energy system. Through comprehensive comparative analyses, the study emphasizes the superior performance of Teaching-Learning-Based Optimization in achieving optimal outcomes, showcasing its potential for practical applications in remote and energy-scarce regions.
Keywords: Diesel dispatch strategies, LPSP, Penalty cost, emission, Particulate matter (PM) emission factors, Evolutionary techniques
Received: 01 Oct 2024; Accepted: 10 Mar 2025.
Copyright: © 2025 Narvey, Sawle and Kumar. This is an open-access article distributed under the terms of the Creative Commons Attribution License (CC BY). The use, distribution or reproduction in other forums is permitted, provided the original author(s) or licensor are credited and that the original publication in this journal is cited, in accordance with accepted academic practice. No use, distribution or reproduction is permitted which does not comply with these terms.
* Correspondence:
Yashwant Sawle, Madhav Institute of Technology & Science Gwalior, Gwalior, India
Disclaimer: All claims expressed in this article are solely those of the authors and do not necessarily represent those of their affiliated organizations, or those of the publisher, the editors and the reviewers. Any product that may be evaluated in this article or claim that may be made by its manufacturer is not guaranteed or endorsed by the publisher.
Research integrity at Frontiers
Learn more about the work of our research integrity team to safeguard the quality of each article we publish.