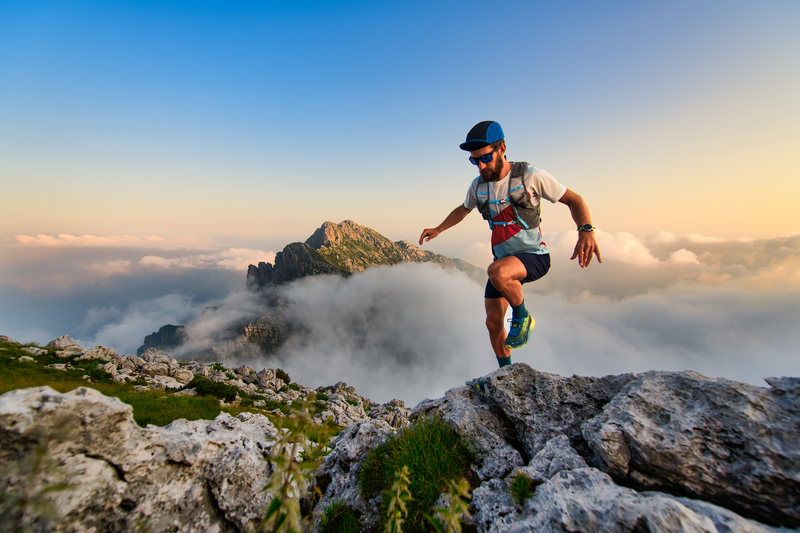
94% of researchers rate our articles as excellent or good
Learn more about the work of our research integrity team to safeguard the quality of each article we publish.
Find out more
ORIGINAL RESEARCH article
Front. Energy Res.
Sec. Energy Efficiency
Volume 13 - 2025 | doi: 10.3389/fenrg.2025.1473946
The final, formatted version of the article will be published soon.
You have multiple emails registered with Frontiers:
Please enter your email address:
If you already have an account, please login
You don't have a Frontiers account ? You can register here
United Nations Sustainable Development Goal 7 is about ensuring access to clean and affordable energy, which is a key factor in the development of society. The power generation sector majorly consists of thermal power plants. Cooling towers are a significant part of any power plant to cool steam to be reused again. Hence, the efficiency of power plants can be increased by optimizing the performance of cooling towers. This research paper aims to increase the efficiency of cooling towers by investigating the effect of ambient parameters (changing with climate) on the efficiency of cooling towers for the best site selection. Ambient parameters cannot be controlled after the installation of power plants. Therefore, proper site selection, keeping ambient parameters and their expected change before the installation of power plants, effectively increases the efficiency of the cooling tower and, ultimately, the power plant. For this purpose, data is collected from the 1140 MW combined cycle power plant in Sheikhupura, Pakistan district. A machine learning (Ada boost regressor) model has been used to quantify the effect of ambient parameters on cooling tower efficiency. After tuning the hyperparameters, an R-square score of 0.983 and a root mean squared error of 0.57 are achieved. Afterwards, a sensitivity analysis of relative humidity (%), turned out to be the most important feature, with a contribution of 12 %. The novelty of this research lies in its mathematical model for power plant site selection, which optimizes cooling tower efficiency, reduces pollution, and promotes environmental sustainability.
Keywords: Power Plants, cooling towers, machine learning, ambient parameters, Site selecting
Received: 30 Aug 2024; Accepted: 14 Feb 2025.
Copyright: © 2025 Mujtaba, Munir, Akhtar, Mahmood, Ansar, Khawar, Khalid, Basit, Jamil, Kalam, Hussain and Bhowmik. This is an open-access article distributed under the terms of the Creative Commons Attribution License (CC BY). The use, distribution or reproduction in other forums is permitted, provided the original author(s) or licensor are credited and that the original publication in this journal is cited, in accordance with accepted academic practice. No use, distribution or reproduction is permitted which does not comply with these terms.
* Correspondence:
M.A. Mujtaba, University of Engineering and Technology, Lahore, Lahore, Pakistan
Disclaimer: All claims expressed in this article are solely those of the authors and do not necessarily represent those of their affiliated organizations, or those of the publisher, the editors and the reviewers. Any product that may be evaluated in this article or claim that may be made by its manufacturer is not guaranteed or endorsed by the publisher.
Research integrity at Frontiers
Learn more about the work of our research integrity team to safeguard the quality of each article we publish.