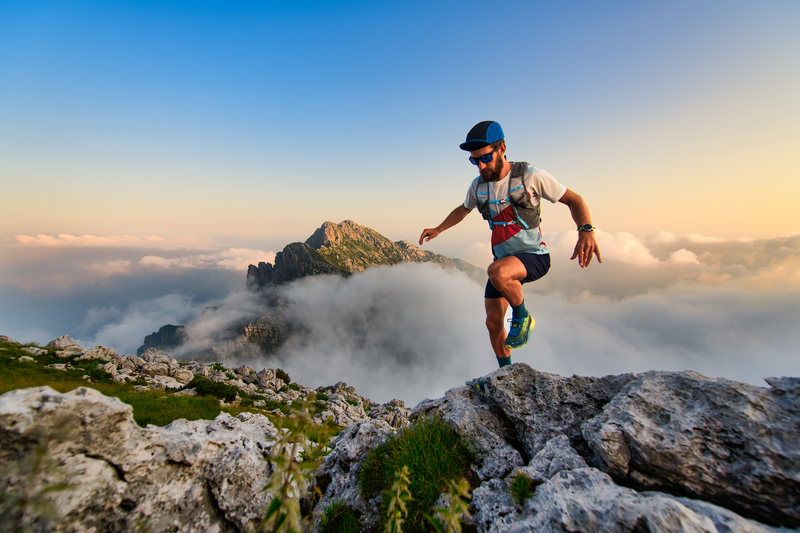
94% of researchers rate our articles as excellent or good
Learn more about the work of our research integrity team to safeguard the quality of each article we publish.
Find out more
METHODS article
Front. Energy Res.
Sec. Energy Efficiency
Volume 13 - 2025 | doi: 10.3389/fenrg.2025.1472768
The final, formatted version of the article will be published soon.
You have multiple emails registered with Frontiers:
Please enter your email address:
If you already have an account, please login
You don't have a Frontiers account ? You can register here
This study introduces a new technique called Pinnacle Multipoint Optimization (PMO) for Maximum Power Point Tracking (MPPT). The approach is specifically developed to improve the performance of photovoltaic (PV) systems in both regular and partially shaded settings. The PMO algorithm adaptively modifies three crucial tracking points to effectively traverse the power-voltage (P-V) curve, guaranteeing precise monitoring (GMPP). The PMO algorithm has been proven to surpass the classic Perturb and Observe (P&O) approach regarding efficiency, tracking speed, and resilience through comprehensive simulations conducted under diverse irradiation settings. More precisely, when there is partial shadowing, the PMO approach demonstrates significant enhancements compared to the P&O method, which only achieves an average efficiency of 54.71%. The PMO algorithm is proficient in optimizing energy output and minimizing power dissipation in photovoltaic systems.
Keywords: Maximum Power Point Tracking (MPPT), Pinnacle Multi-Point Optimization (PMO), Photovoltaic (PV) systems, Partial shading conditions, GMPP tracking
Received: 30 Jul 2024; Accepted: 07 Feb 2025.
Copyright: © 2025 Mhanni and Lagmich. This is an open-access article distributed under the terms of the Creative Commons Attribution License (CC BY). The use, distribution or reproduction in other forums is permitted, provided the original author(s) or licensor are credited and that the original publication in this journal is cited, in accordance with accepted academic practice. No use, distribution or reproduction is permitted which does not comply with these terms.
* Correspondence:
Youssef Mhanni, Faculty of Sciences Tetouan, Abdelmalek Essaadi University, Tetouane, Morocco
Disclaimer: All claims expressed in this article are solely those of the authors and do not necessarily represent those of their affiliated organizations, or those of the publisher, the editors and the reviewers. Any product that may be evaluated in this article or claim that may be made by its manufacturer is not guaranteed or endorsed by the publisher.
Research integrity at Frontiers
Learn more about the work of our research integrity team to safeguard the quality of each article we publish.