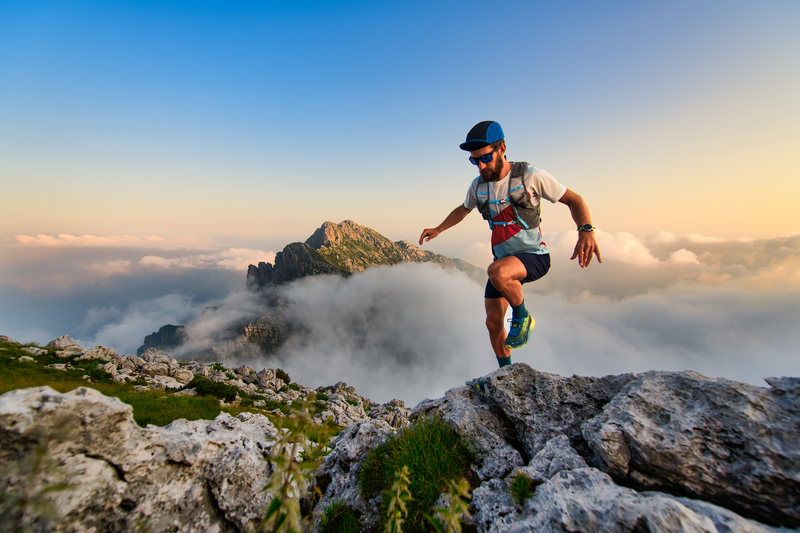
95% of researchers rate our articles as excellent or good
Learn more about the work of our research integrity team to safeguard the quality of each article we publish.
Find out more
ORIGINAL RESEARCH article
Front. Energy Res.
Sec. Smart Grids
Volume 13 - 2025 | doi: 10.3389/fenrg.2025.1445522
The final, formatted version of the article will be published soon.
You have multiple emails registered with Frontiers:
Please enter your email address:
If you already have an account, please login
You don't have a Frontiers account ? You can register here
The rapid and effective islanding detection and disconnection of the microgrid are significant for preventing equipment from failure and safeguarding humanity's safety. To address the drawbacks of active methods and passive methods, an intelligent islanding detection strategy based on parameteroptimized variational mode decomposition (VMD) and deep learning was developed. Firstly, the proposed adaptive variational mode decomposition (AVMD) strategy improves the optimal mode number and penalty term of VMD by utilizing the relative entropy between the original signal and the intrinsic mode functions (IMFs). Then, the Teager energy operator (TEO) further extracts sequence features to track the instantaneous energy of the IMFs. Finally, the AVMD-TEO-MPEbased features are used to train the one-dimensional convolutional neural network (1D-CNN) as a deep learning classifier. Simulation results indicate that the proposed method can effectively differentiate the islanding state under different working conditions with a testing accuracy level of 100% within a maximal detection time of 46.402ms. It is also noise resistant to a degree. Comparative analysis confirms that the proposed method outperforms the existing method in distinguishing between islanding and non-islanding events.
Keywords: Islanding detection, relative entropy, Variational mode decomposition, Teager energy operator, multi-scale permutation entropy, Convolutional Neural Network
Received: 15 Jun 2024; Accepted: 31 Mar 2025.
Copyright: © 2025 Xia, Lv, Chen, Yu, Li, Yang and Yang. This is an open-access article distributed under the terms of the Creative Commons Attribution License (CC BY). The use, distribution or reproduction in other forums is permitted, provided the original author(s) or licensor are credited and that the original publication in this journal is cited, in accordance with accepted academic practice. No use, distribution or reproduction is permitted which does not comply with these terms.
* Correspondence:
Yuli Lv, Sichuan University of Science and Engineering, Zigong, China
Disclaimer: All claims expressed in this article are solely those of the authors and do not necessarily represent those of their affiliated organizations, or those of the publisher, the editors and the reviewers. Any product that may be evaluated in this article or claim that may be made by its manufacturer is not guaranteed or endorsed by the publisher.
Research integrity at Frontiers
Learn more about the work of our research integrity team to safeguard the quality of each article we publish.