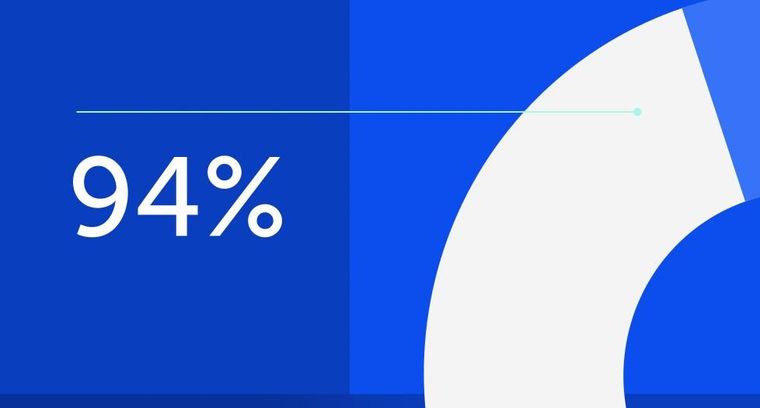
94% of researchers rate our articles as excellent or good
Learn more about the work of our research integrity team to safeguard the quality of each article we publish.
Find out more
ORIGINAL RESEARCH article
Front. Energy Res., 03 March 2025
Sec. Smart Grids
Volume 12 - 2024 | https://doi.org/10.3389/fenrg.2024.1522514
Focusing on the low-carbon economic operation of an integrated energy system (IES), this paper proposes a novel energy-carbon pricing and energy management method to promote carbon emission reductions in the IES based on the carbon emission flow theory and reinforcement learning (RL) approach. Firstly, an energy-carbon integrated pricing model is proposed. The proposed pricing method charges prosumers by tracing the embedded carbon emissions of energy usages, and establishes an energy-carbon-prices relationship between the power grid, IES and prosumers. Secondly, an energy management model considering the energy-carbon integrated pricing strategy is established based on the Markov decision processes (MDP), including prosumers energy consumption cost model and energy service provider (ESP) profit model. Then, a solving method based on the RL approach is proposed. Finally, numerical results show that the proposed method can improve operation economy and reduce carbon emissions of IES. When carbon price accompanying electricity and thermal is considered in the process of pricing and energy management, the profit of ESP can be improved and the cost of prosumers can be reduced, and the total carbon emission of IES can be reduced by 5.75% compared with not considering carbon price.
In response to the challenges of global climate changes, the world is actively promoting low-carbon and clean energy systems. China is committed to achieve the carbon peak by 2030 and carbon neutral by 2060 (Liu and Niu, 2021). IES can exploit synergies among different energy forms (Li et al., 2023), which has been extensively recognized as an efficient way of reducing carbon emissions by promoting renewable energy absorption and energy cascading utilization (Su et al., 2021; Wei et al., 2021). In order to realize the low-carbon economic operation of the IES, it is necessary to consider carbon trading, carbon quota at the level of system operation optimization to reduce carbon emissions (Huang et al., 2022a). In addition, considering carbon emission factors in the energy interaction, especially in the energy pricing, is also an effective method to reduce carbon emissions from energy production and consumption (Huang Zhang, 2018; Wang et al., 2020a).
Carbon emissions can be incorporated into the system operation as the additional cost in the objective function or maximum allowable emissions in constraints. Based on this, there have been some studies on the low-carbon economic operation and energy management of IES. Literature Wang et al. (2020a) proposes a two-stage scheduling model to investigate environmental benefits of consumers participating in both electricity and carbon emission trading markets. Literature Wang et al. (2020b) proposes a two-stage low-carbon operation planning model based on a bilateral trading mechanism to mitigate carbon emissions. In Literature Gu et al. (2020), a bi-level optimal low-carbon economic dispatch model for an industrial park is proposed to optimize energy conversion equipment, and set reasonable energy selling prices. In Literature Xiang et al. (2021), a low-carbon economic dispatch model for electricity-gas systems is proposed, in which the impacts of the different low-carbon technologies on system economy and carbon emission are investigated. In Literature Yao et al. (2012), a computational framework for integrating wind power uncertainties and carbon prices in economic dispatch model is developed to solve the revised dispatch strategy. To realize the low-carbon economic operation, a ladder-type carbon trading is introduced Cui et al. (2021a). In Literature Cui et al. (2021b), the carbon capture technology is used to the low-carbon dispatch of the IES, in which the price-based demand response is considered. Literature Chen et al. (2021) proposes a two-stage low-carbon optimal scheduling model for combined heat and power (CHP) systems considering the carbon emission flow theory and demand responses based on the carbon prices for reducing carbon emissions. Literature Shen (2024) addresses this real-world challenge by utilizing evolutionary game theory to model the strategic interactions between these stakeholders under a low-carbon trading mechanism.
In addition, the consumption-based carbon emission accounting is able to clarify the carbon emissions responsibility, and the carbon emissions responsibility of consumers can be calculated based on the energy consumption and corresponding carbon emission flows. In different energy networks, the carbon emission flows distribution may be significantly, which leads to different attributed carbon emission responsibilities of consumers (Peters, 2008; Li et al., 2013). In Literature Chen et al. (2018), the carbon responsibility of the power system is shared on the generation side and load side, and the problem is modeled as a cost sharing problem based on the cooperative game. Combining the carbon emission analysis and the power flow calculation, the theoretical architecture of carbon emission flow analysis of the power system is preliminarily formed (Zhou et al., 2012). In Literature Zhang et al. (2022), the impacts of various energy flows on the carbon emissions is explored in a case study. Literature Sun et al. (2017) presents a transmission expansion planning model considering the consumption-based carbon emission accounting. In addition, some literatures have studied the carbon emission pricing based on the calculation of the carbon emission flows. Literature Cheng et al. (2019) studies the low-carbon operation of multiple energy systems by coordinating the transmission-level and distribution-level via the energy-carbon integrated prices. Literature Moreira et al. (2010) analysis the social welfare of the Iberian electricity market considering the carbon emission prices.
From the above literatures (The comparisons of details between this article and previous research are depicted in Supplementary Appendix A), it can be concluded that most of the existing methods to account for carbon emissions in the power system are the generation-based. However, this generation-based carbon emission accounting may lead to unbalanced responsibilities and benefits between the generation units and consumers, especially in the IES that have electricity-thermal energy exchanges. In addition, although the existing research on the energy pricing and low-carbon operation of IES considers the carbon emission factors in modeling, it does not connect the transmission relationship and carbon emission responsibility in the IES with the multi-energy interaction process between ESP and users. To deal with the above-mentioned challenges, the energy-carbon flow relationship and energy-carbon pricing strategy between ESP and prosumers in the IES need to be modeled and analyzed in detail, and the solution method of this complex pricing model is proposed. To this end, we propose an energy-carbon integrated pricing and energy management method of the IES based on the RL. The main contributions are as follows:
1) An energy-carbon integrated pricing model is proposed. The carbon emission intensity (CEI) index is applied to quantify the carbon emission intensity of different energy node in the IES. The energy-carbon integrated prices model is established to study the energy-carbon-prices relationship between the power grid, CHP energy service provider (CHP-ESP) and prosumers.
2) A dynamic pricing method based on the RL of the IES composed of the CHP-ESP and prosumers is proposed. Considering the energy supply revenue of the CHP-ESP and the energy cost of prosumers, the energy transaction process is simulated by the Q-Learning algorithm based on the energy-carbon integrated pricing model. This further determines the energy pricing strategy of the CHP-ESP, optimal energy consumption strategy of prosumers and system operation strategy.
The remainder of this paper is organized as follows. Section 2 introduces the system architecture and pricing model. The energy management model is proposed in Section 3. The reinforcement learning methodology is used to solve model in Section 4. Section 5 presents case study results. Finally, conclusions are given in Section 6.
This paper focuses on the IES with CHP and prosumers, and the system structure is shown in Figure 1.
CHP-ESP is the operator of the IES, which controls CHP and can provide electric energy and thermal energy for prosumers in the system. The CHP is equipped with a CHP energy management system (CHP-EMS), which can dispatch the electricity and thermal generations. The prosumer has a certain proportion of controllable loads, which makes it have the load adjustment ability. In addition, prosumer is equipped with the photovoltaic generation (PV) and prosumer energy management system (PEMS), which can dispatch the electricity energy consumption, thermal energy consumption and electricity energy transaction traded with the IES. In order to enhance the system stability, the IES is connected with the power grid to meet the electricity demand. When there is excess electricity in the IES, the excess electricity will be sent back to the power grid.
This section mainly studies the relationship between the energy flows and carbon flows, and establishes an energy-carbon integrated model. In general, the electricity input from the power grid to IES is mixture electricity of coal-fired generators, WT, PV and hydropower. Here, it is assumed that there is no carbon emission in WT, PV and hydropower generation. Figure 2 shows the energy-carbon flow relationship among the power grid, CHP-ESP and prosumer.
Based on the energy-carbon relationship, carbon emission intensity theory and proportional sharing assumption theory (Cheng et al., 2019), this paper proposes the CEI index to reflect the carbon emission intensity of each energy node. The CEI index represents the average carbon emissions related to the injected energy flow during a certain time period, which is equal to the weighted average of the carbon intensities of all injected energy flows (Zhang et al., 2022). Given t∈ 𝒯 ≡ {t:t = 1,2,⋯,T}, where T is the number of time slots of the energy operation. The CEI index models are shown as:
here,
Therefore, the energy-carbon integrated prices of the electricity and thermal can be obtained by combining CHP-ESP energy prices and carbon price according to the method in Zhang et al. (2022), Cheng et al. (2019), and Kang et al. (2012). The prices models are shown as:
here,
The electrical loads of prosumers can be classified as critical and adjustable loads according to priorities (Yuan et al., 2021; Liu et al., 2019; Wang et al., 2021). n∈𝒩 ≡ {n:n = 1,2,⋯,N}, N is the number of prosumers. The electrical loads models are shown as:
here,
The thermal loads of prosumers can be classified as critical and adjustable loads according to priorities (Liu et al., 2019; Wang et al., 2021). The thermal loads models are shown as:
here,
The cost of prosumers consists of the satisfaction loss cost and energy cost. The satisfaction loss cost function indicates the loss value of the energy consumption utility (Wang H. et al., 2020; Cheng et al., 2021). The objective functions of prosumers are shown in Equations 1–3:
here,
In this study, the CHP-ESP is the link between the power grid and prosumers. It participates in the energy market transactions and supplies electricity and thermal energy to prosumers by scheduling energy supply units. Hence, the objective of the CHP-ESP is to perform dynamic pricing that maximizes its profits (Huang et al., 2022b). The objective functions of CHP-ESP are shown in Equations 4–6:
here,
The energy transaction process between the CHP-ESP and prosumers is similar to a price game behavior, and the benefits generated by both sides should be considered in the pricing decision-making process. In this paper, we consider both the CHP-ESP’s profit and prosumers’ cost as follows (Lu et al., 2018).
here,
In order to ensure the smooth energy interaction between the CHP-ESP and prosumers and the safe operation of the CHP-ESP, the following constraints must be met (Huang et al., 2022b; Wang et al., 2019). The constraints include the power balance constraint (Equations 7, 8), the equipment operation constraint (Equation 9), the load constraint (Equations 10, 11 (Liu et al., 2016)) and the price constraint (Equations 12, 13). The constraints are shown as:
here,
At present, the energy management method of energy system is mostly combined with optimization algorithm or heuristic algorithm in terms of algorithm. The calculation efficiency of optimization algorithm is high, but it is difficult to escape from local optimization when dealing with nonlinear, nonconvex or discontinuous problem. Heuristic algorithm can get the corresponding optimal solution or Pareto frontier under given conditions, but it has many restrictions, long calculation time and insufficient generalization learning ability (Cheng et al., 2022; Cheng et al., 2020).
Reinforcement learning based on MDP theory is an important machine learning method with strong autonomous learning ability and adaptability. RL is a method to make sequential decisions in an unknown environment. It can change the strategy in real time based on online learning from past experience. In RL approach, the agent interacts with the environment, and constantly learns adaptively through “trial and error” to find the optimal strategy. RL method does not need the distribution knowledge of uncertain factors in the system, and it is a potential method to solve the optimization problem with uncertain factors. It has been introduced into the operation optimization and energy management of smart grid, buildings and so on as a potential solution (Wang et al., 2021; Lu et al., 2021; Zhong et al., 2021).
In this paper, the energy-carbon integrated pricing and energy management problem is modeled as a discrete finite horizon MDP because it is a decision-making problem in a stochastic environment. The MDP process consists of four basic elements, namely the state, action, reward and discount rate. The reward and action taken only depend on the current energy information, but have nothing to do with the historical data. Therefore, this problem can be modeled as a finite MDP with the CHP-ESP as the agent (Lu et al., 2018; Lu et al., 2021; Zhong et al., 2021). Figure 3 shows the agent-environment interactive mode.
We formulate the energy-carbon integrated pricing as a discrete finite MDP model. The MDP models established are shown in Equations 14–18:
here,
Then this MDP problem is solved by adopting the Q-learning method, which solves the problem of sequence decision in unknown environment by mapping selection probability from the state to action. Table 1 shows the flowchart of Q-learning algorithm.
If the agent chooses strategy
Similarly, the value of action
Then, the bellman equation is introduced to represent an iterative relationship of correlation between the current state value and future state value.
And the iterative relationship of the correlation between the current action and future action can be obtained. Here,
The
Based on the Q-learning algorithm, the simulation will be run with iterations from which the optimal prices are computed, i.e., at the beginning of a day, the CHP-ESP receives the wholesale electricity price from the power grid, the load demand from prosumers and other parameters defined in the scenario. Then the CHP-ESP calculates the Q-value (CHP-ESP prices), and finally obtains the maximum Q-value.
In this section, an IES is used as an example to test the energy management and energy-carbon integrated pricing method proposed in this paper. The test system consists of 1 CHP-ESP and three prosumers, and the system structure is shown in Figure 1, and it is assumed that the electricity supply of the power grid in this case is only coal-fired power units.
The entire time cycle is divided into 24 time slots representing the 24 h of a day, thus the optimization time scale t is 1 h. The load data and PV data come from a typical day in winter (As shown in Figures 4–6). The feed-in tariff of the power grid without carbon is 0.35 CNY/kWh. Table 2 shows the wholesale electricity price and electricity elasticity coefficient, Table 3 shows the comfort parameters of three prosumers (Lu et al., 2018; Guo et al., 2020). The wholesale thermal price is 0.3 CNY/kWh, and the thermal elasticity coefficient is −0.3. The CEI index of natural gas is 1.96 kg/m3, the CEI index of coal power units is 0.85 kg/kWh, the low calorific value of natural gas is 9.78 kWh/m3, the operational efficiency of the CHP is 0.92, and the thermal-electricity ratio of the CHP is 1.35. The simulation is conducted using the software with python-programmed code, and a 2.9 GHz, i5-10400 CPU, 16 GB RAM windows PC hardware.
This section presents the simulation results to assess the performance of the proposed energy-carbon integrated pricing model according to the Q-learning algorithm.
Figure 7 shows the feed-in tariff of power grid. Figures 8, 9 show the electricity-carbon price of the power grid and CHP-ESP respectively. Figure 10 shows the electricity energy consumption of three prosumers. Figure 11 shows the thermal-carbon price of the CHP-ESP, and Figure 12 shows the thermal energy consumption of three prosumers.
It can be seen from Figure 8 that the electricity price with carbon of the power grid is exactly the same as the electricity price without carbon in some time periods, especially from 6: 00 to 13: 00. In the above time period, the thermal load demand is large, which makes CHP units a large amount of electricity while supplying thermal. At the same time, the PV power in the IES is very rich. The above two factors lead the CHP-ESP to transmit the abundant electricity to the power grid. In other time periods, the CHP-ESP needs to purchase electricity from the power grid due to insufficient PV output, and the power grid accumulates a part of carbon cost on the basis of electricity price according to the electric power delivered to the IES.
Comparing the electricity price strategies of the power grid and CHP-ESP, we can find that the electricity-carbon price of the CHP-ESP and power grid have the same trend. This is because the CHP-ESP price strategy is produced under the joint constraints of the power grid price and carbon emission intensity of the IES. For the CHP-ESP, its pricing decision shows a change trend similar to the power grid price. From 7: 00 to 13: 00, the PV power to which each prosumer belongs can meet most of the power demand, and under stimulation of electricity price, the prosumer will adjust the loads to the maximum extent, which makes the net electricity loads of prosumers relatively small in this time period. Therefore, prosumers need to bear less carbon cost, and the electricity price of the CHP-ESP with the carbon is similar to that without the carbon. On the contrary, the PV power of prosumers is insufficient in other periods, which leads to prosumers must rely on the CHP-ESP to meet the electricity demand. The large amount of electricity with the carbon purchased from the CHP-ESP eventually leads to the extra carbon cost of prosumers.
In addition, as can be seen from Figure 10, the electric load adjustment strategy of prosumers is also affected by the price with the carbon factor. The electricity load adjustment amount of prosumers in the period of the small net loads is obviously lower than that in the period of large net loads. This is the result of efforts for prosumers to minimize the electricity cost and carbon cost.
Because the CHP is the only thermal source in the system, the carbon emission intensity generated is only related to the operation state of the CHP. It can be seen from Figures 11, 12 that the CHP-ESP will reduce the thermal price in the period when the thermal load demand of prosumers is low, so as to prevent prosumers from drastically reducing the thermal load due to the thermal cost.
Figure 13 shows the optimized scheduling strategy of the IES. The CHP operates in the “thermal-lead mode,” and its operation strategy is closely related to thermal loads. As the backup power supply of the IES, the power grid can guarantee electric power balance in the IES.
This section analyzes the optimization results of the CHP-ESP and prosumers, and discusses the influence of carbon price changes on the results. Figure 14 shows the convergence of the Q-value, and Table 4 shows the optimization results under different pricing modes.
By analyzing the data in Table 4, it can be found that compared with the traditional method (Price without carbon), the pricing and energy management model considering carbon emission factors can get almost the same economic operation results, but it has obvious advantages in carbon emission reduction, and the carbon emissions are reduced by 5.75%. The simulation takes 150s with 100 iterations, and converges to the optimal value at close to 67 iterations.
In order to further study the influence of carbon price changes on the results, we simulates the changes of the average prices, objective values and carbon emissions when the carbon price increased from 0.049 CNY/kg to 0.099 CNY/kg. Figure 15 shows the average prices for the different carbon prices, Figure 16 shows the objective functions for different carbon prices.
When the carbon price is increasing, the average thermal-carbon price increases in direct proportion to carbon prices. The electricity-carbon price increased sharply at first, then slowly and gradually stabilized at the later stage. When the growth rate of the carbon price is small, prosumers can still tolerate price increase. However, with the continuous increase of the carbon price, the electricity-carbon price increase will gradually exceed the tolerance range of prosumers, and prosumers will greatly reduce electricity demand, which makes the CHP-ESP lower the electricity price to ensure the electricity sales volume to meet expected minimum profit.
As can be seen from Figure 16 that the change trend of the CHP-ESP profit with the carbon price is similar to that of the average electricity-carbon price. This is because the main profit of the CHP-ESP comes from supplying electricity and thermal energy to prosumers, and the profit of prosumers is more affected by the electricity-carbon price. In the early stage of the carbon price increase, prosumers will adjust their loads to minimize the energy cost. However, with the further increase of carbon price, the load adjustments of prosumers will reach the upper limit. At this time, prosumers will be in a weak position, unable to offset the substantial increase in energy costs caused by the increase of carbon price. At the same time, the load adjustments due to the price increase will also damage the utility of the prosumers. Therefore, the cost of prosumers shows a trend of increasing slowly and then increasing sharply.
In the RL model established in this paper, the interaction process between CHP-ESP and prosumers, in which CHP-ESP is the leader to formulate the price strategy and prosumers are the followers to respond to the price strategy and optimize the energy consumption, which conforms to the Stackelberg game mechanis. Therefore, this section simulates the energy management method and energy-carbon pricing strategy based on Stackelberg game, and compare the results from two aspects of optimization results and algorithm performance to enhance the robustness of the results. The modeling and proving process of Stackelberg game model refer to literature (Huang et al., 2022b; Wang and Hu, 2023), and the distributed solving algorithm based on genetic algorithm established in literature (Wang and Hu, 2023) is adopted for model solving, and other optimization parameters adopt the parameters set in this paper. Table 5 shows the comparison of optimization results, and Figure 17 shows the convergence curve of Stackelberg game.
From the data calculation in Table 5, compared with Stackelberg game optimization, the total operating benefit of ESP and the total cost of IES optimized by RL are increased by 86.8 CNY and 45.37 CNY respectively, which shows that the two methods have very close performance in objective function optimization. However, combined with Table 5 and Figure 17, it can be seen that the RL method established in this paper can complete the optimization iteration faster and get the optimal solution compared with the Stackelberg game. In the process of energy management numerical calculation, it can save calculation time and cost, and this advantage will be more obvious in the future when larger-scale systems are optimized.
In this paper, an energy-carbon integrated pricing and energy management method of IES based on the RL approach is proposed, the proposed method establishes an energy-carbon-prices relationship between the power grid, IES and prosumers by tracing the embedded carbon emissions of energy consumption chains. In addition, the energy-carbon integrated pricing and energy management model is solved by the Q-learning algorithm to determine the optimal energy pricing strategy, prosumers energy consumption strategy and system operation strategy. Case study based on two scenarios of with and without the carbon price shows that the proposed method has obvious advantages in the carbon emission reduction and effectively facilitates the low-carbon operation of the IES. The carbon emissions in the operation of the IES are reduced by 5.75% with the incorporation of the carbon price.
The model established in this paper can enrich the multi-agent energy interaction and pricing method of IES from the theoretical level, and support IES to participate in the energy interaction in the energy- carbon market from the application level to realize the low-carbon economic operation. However, the thermal energy supply of the IES in this paper is provided by IES itself, without considering the thermal interaction with the external thermal market. When the IES interacts with the external thermal market, it will increase the complexity of carbon emission calculation, these will be considered in future research.
The original contributions presented in the study are included in the article/Supplementary Material, further inquiries can be directed to the corresponding author.
HZ: Conceptualization, Funding acquisition, Investigation, Software, Writing–original draft, Writing–review and editing. XS: Writing–original draft, Writing–review and editing. HL: Methodology, Writing–original draft. EL: Software, Writing–original draft. YZ: Writing–original draft. KL: Writing–original draft. TL: Writing–original draft. MX: Writing–original draft.
The author(s) declare that financial support was received for the research, authorship, and/or publication of this article. This work is supported by Yunnan postdoctoral fund project, research and application demonstration of key technologies of flexible and efficient collaboration of new multi-energy systems in border and cross-border areas (YNKJXM202110177).
Authors HZ and EL were employed by Electric Power Research Institute of Yunnan Electric Power Grid Co., Ltd. Authors XS, HL, KL, TL, and MX were employed by CSG Electric Power Research Institute Co. Author YZ was employed by Yunnan Power Grid Co., Ltd.
The author(s) declare that no Generative AI was used in the creation of this manuscript.
All claims expressed in this article are solely those of the authors and do not necessarily represent those of their affiliated organizations, or those of the publisher, the editors and the reviewers. Any product that may be evaluated in this article, or claim that may be made by its manufacturer, is not guaranteed or endorsed by the publisher.
The Supplementary Material for this article can be found online at: https://www.frontiersin.org/articles/10.3389/fenrg.2024.1522514/full#supplementary-material
Chen, L., Sun, M., Zhou, Y., Ella, Z., Fang, C., and Fen, D. (2018). Method of carbon obligation allocation between generation side and demand side of power system. Automation Electr. Power Syst. 42 (19), 106–111. doi:10.7500/AEPS20171113004
Chen, H., Mao, W., Zhang, R., and Yu, W. (2021). Low-carbon optimal scheduling of a power system source-load considering coordination based on carbon emission flow theory. Power Syst. Prot. Control 49 (10), 1–11. doi:10.19783/j.cnki.pspc.200932
Cheng, Y., Zhang, N., Zhang, B., Kang, C., and Feng, M. (2019). Low-carbon operation of multiple energy systems based on energy-carbon integrated prices. IEEE Trans. Smart Grid (99), 1. doi:10.1109/TSG.2019.2935736
Cheng, L., Liu, G., Huang, H., Wang, X., Chen, Y., Zhang, J., et al. (2020). Equilibrium analysis of general N -population multi-strategy games for generation-side long-term bidding: an evolutionary game perspective. J. Clean. Prod. 276, 124123. doi:10.1016/j.jclepro.2020.124123
Cheng, L., Yin, L., Wang, J., Teng, S., Chen, Y., Liu, G., et al. (2021). Behavioral decision-making in power demand-side response management: a multi-population evolutionary game dynamics perspective. Int. J. Electr. Power and Energy Syst. 129, 106743. doi:10.1016/j.ijepes.2020.106743
Cheng, L., Chen, Y., and Liu, G. (2022). 2PnS-EG: a general two-population n-strategy evolutionary game for strategic long-term bidding in a deregulated market under different market clearing mechanisms. Int. J. Electr. Power and Energy Syst. 142, 108182. doi:10.1016/j.ijepes.2022.108182
Cui, Y., Zeng, P., Zhong, W., Cui, L., Zeng, P., and Zhao, Y. (2021a). Low-carbon economic dispatch of electricity-gas-heat integrated energy system based on ladder-type carbon trading. Electr. Power Autom. Equip. 41 (03), 10–17. doi:10.16081/j.epae.202011030
Cui, Y., Zeng, P., Wang, Z., Wang, M., Zhang, J., and Zhao, Y. (2021b). Low-carbon economic dispatch of electricity-gas-heat integrated energy system with carbon capture equipment considering price-based demand response. Power Syst. Technol. 45 (02), 447–459. doi:10.13335/j.1000-3673.pst.2020.0100a
Gu, H., Li, Y., Yu, J., Wu, C., Song, T., and Xu, J. (2020). Bi-level optimal low-carbon economic dispatch for an industrial park with consideration of multi-energy price incentives. Appl. Energy 262, 114276. doi:10.1016/j.apenergy.2019.114276
Guo, W., Liu, P., and Shu, X. (2020). Optimal dispatching of electric-thermal interconnected virtual power plant considering market trading mechanism. J. Clean. Prod. 279 (54), 123446. doi:10.1016/j.jclepro.2020.123446
Huang, Y., and Zhang, Y. (2018). Energy use and carbon emissions efficiency study of Chinese regions based on price factor. Pol. J. Environ. Stud. 27 (5), 2059–2069. doi:10.15244/pjoes/78152
Huang, Y., Wang, Y., and Liu, N. (2022a). Low-carbon economic dispatch and energy sharing method of multiple Integrated Energy Systems from the perspective of System of Systems. Energy 244 (Part A), 122717. doi:10.1016/j.energy.2021.122717
Huang, Y., Wang, Y., and Liu, N. (2022b). A two-stage energy management for heat-electricity integrated energy system considering dynamic pricing of Stackelberg game and operation strategy optimization. Energy 244, 122576. doi:10.1016/j.energy.2021.122576
Kang, C., Zhou, T., Chen, Q., Xu, Q., Xia, Q., and Ji, Z. (2012). Carbon emission flow in networks. Sci. Rep. 2, 479. doi:10.1038/srep00479
Li, B., Song, Y., and Hu, Z. (2013). Carbon flow tracing method for assessment of demand side carbon emissions obligation. IEEE Trans.4 (4), 1100–1107. doi:10.1109/tste.2013.2268642
Li, K., Mu, Y., Yang, F., Wang, H., and Zhang, C. (2023). A novel short-term multi-energy load forecasting method for integrated energy system based on feature separation-fusion technology and improved CNN. Appl. Energy 351, 121823. doi:10.1016/j.apenergy.2023.121823
Liu, Y., and Niu, D. (2021). Coupling and coordination analysis of thermal power carbon emission efficiency under the background of clean energy substitution. Sustainability 13. doi:10.3390/su132313221
Liu, N., Wang, C., and Lei, J. (2016). Power energy sharing and Demand Response model for cluster of PV prosumers under market environment. Automation Electr. Power Syst. 44 (16), 49–56. doi:10.7500/AEPS20160120002
Liu, P., Ding, T., Zou, Z., and Yang, Y. (2019). Integrated demand response for a load serving entity in multi-energy market considering network constraints. Appl. Energy 250, 512–529. doi:10.1016/j.apenergy.2019.05.003
Lu, R., Hong, S., and Zhang, X. (2018). A Dynamic pricing demand response algorithm for smart grid: reinforcement learning approach. Appl. Energy 220, 220–230. doi:10.1016/j.apenergy.2018.03.072
Lu, T., Chen, X., Mcelroy, M. B., Nielsen, C. P., Wu, Q., and Ai, Q. (2021). A reinforcement learning-based decision system for electricity pricing plan selection by smart grid end users. IEEE Trans. Smart Grid 12 (3), 2176–2187. doi:10.1109/tsg.2020.3027728
Moreira, A., Oliveira, S., and Pereira, J. (2010). Social welfare analysis of the iberian electricity market accounting for carbon emission prices. Iet Generation Transm. and Distribution 4 (2), 231–243. doi:10.1049/iet-gtd.2009.0105
Peters, G. P. (2008). From production-based to consumption-based national emission inventories. Ecol. Econ. 65 (1), 13–23. doi:10.1016/j.ecolecon.2007.10.014
Shen, T. (2024). Spontaneous Formation of evolutionary game strategies for long-term carbon emission reduction based on low-carbon trading mechanism. Mathematics 12, 12193109. doi:10.3390/math12193109
Su, J., Chiang, H., Zeng, Y., and Zhou, N. (2021). Toward complete characterization of the steady-state security region for the electricity-gas integrated energy system. IEEE Trans. Smart Grid 12 (4), 3004–3015. doi:10.1109/tsg.2021.3065501
Sun, Y., Kang, C., Xia, Q., Chen, Q., Zhang, N., and Cheng, Y. (2017). Analysis of transmission expansion planning considering consumption-based carbon emission accounting. Appl. Energy 193, 232–242. doi:10.1016/j.apenergy.2017.02.035
Wang, Y., Wang, Y., Huang, Y., Yang, J., Ma, Y., Yu, H., et al. (2019). Operation optimization of regional integrated energy system based on the modeling of electricity-thermal-natural gas network. Appl. Energy 251, 113410. doi:10.1016/j.apenergy.2019.113410
Wang, Y., Qiu, J., Tao, Y., and Zhao, J. (2020a). Carbon-Oriented operational planning in coupled electricity and emission trading markets. IEEE Trans. Power Syst. 35 (4), 3145–3157. doi:10.1109/tpwrs.2020.2966663
Wang, Y., Qiu, J., Tao, Y., Zhang, X., and Wang, G. (2020b). Low-carbon oriented optimal energy dispatch in coupled natural gas and electricity systems. Appl. Energy 280, 115948. doi:10.1016/j.apenergy.2020.115948
Wang, H., Li, K., Zhang, C., and Ma, X. (2020c). Distributed coordinative optimal operation of community integrated energy system based on Stackelberg game. Proc. CSEE 40 (17), 5435–5445. doi:10.13334/j.0258-8013.pcsee.200141
Wang, X., Wang, S., Zhang, Q., Shaomin, W., and Liwei, F. (2021). A multi-energy load prediction model based on deep multi-task learning and ensemble approach for regional integrated energy systems. Int. J. Electr. Power and Energy Syst. 126 (9), 106583. doi:10.1016/j.ijepes.2020.106583
Wang, Y., and Hu, J. (2023). Two-stage energy management method of integrated energy system considering pre-transaction behavior of energy service provider and users. Energy 271, 127065. doi:10.1016/j.energy.2023.127065
Wei, X., Zhang, X., Sun, Y., and Qiu, J. (2021). Carbon emission flow oriented optimal planning of electricity-hydrogen integrated energy system with hydrogen vehicles. IEEE Trans. Industry Appl. (99), 1. doi:10.1109/TIA.2021.3095246
Xiang, Y., Wu, G., Shen, X., Ma, Y., Gou, J., Xu, W., et al. (2021). Low-carbon economic dispatch of electricity-gas systems. Energy 226, 120267. doi:10.1016/j.energy.2021.120267
Yao, F., Dong, Z., Meng, K., Xu, Z., Iu, H. H. C., and Wong, K. P. (2012). Quantum-inspired particle swarm optimization for power system operations considering wind power uncertainty and carbon tax in Australia. IEEE Trans. Industrial Inf. 8 (4), 880–888. doi:10.1109/tii.2012.2210431
Yuan, G., Gao, Y., and Ye, B. (2021). Optimal dispatching strategy and real-time pricing for multi-regional integrated energy systems based on demand response. Renew. Energy 179, 1424–1446. doi:10.1016/j.renene.2021.07.036
Zhang, H., Sun, W., Li, W., and Ma, G. (2022). A carbon flow tracing and carbon accounting method for exploring CO2 emissions of the iron and steel industry: an integrated material-energy-carbon hub. Appl. Energy 309, 118485. doi:10.1016/j.apenergy.2021.118485
Zhong, S., Wang, X., Zhao, J., Li, W., Li, H., Wang, Y., et al. (2021). Deep reinforcement learning framework for dynamic pricing demand response of regenerative electric heating. Appl. Energy 288, 116623. doi:10.1016/j.apenergy.2021.116623
Zhou, T., Kang, C., Xu, G., and Chen, Q. (2012). Preliminary theoretical investigation on power system carbon emission flow. Automation Electr. Power Syst. 36 (07), 38–43+85. doi:10.3969/j.issn.1000-1026.2012.07.008
IES Integrated Energy System
MDP Markov decision processes
ESP Energy Service Provider
CEI Carbon Emission Intensity
CHP Combined Heat and Power
EMS Energy Management System
PEMS Prosumer Energy Management System
Keywords: integrated energy system, energy-carbon pricing, energy management, carbon emission, reinforcement learning
Citation: Zhang H, Shi X, Lu H, Luo E, Zhang Y, Li K, Liu T and Xu M (2025) Energy management method of integrated energy system based on energy and carbon pricing strategy and reinforcement learning approach. Front. Energy Res. 12:1522514. doi: 10.3389/fenrg.2024.1522514
Received: 04 November 2024; Accepted: 09 December 2024;
Published: 03 March 2025.
Edited by:
Chaojie Li, University of New South Wales, AustraliaCopyright © 2025 Zhang, Shi, Lu, Luo, Zhang, Li, Liu and Xu. This is an open-access article distributed under the terms of the Creative Commons Attribution License (CC BY). The use, distribution or reproduction in other forums is permitted, provided the original author(s) and the copyright owner(s) are credited and that the original publication in this journal is cited, in accordance with accepted academic practice. No use, distribution or reproduction is permitted which does not comply with these terms.
*Correspondence: Xuntao Shi, eHVudGFvc2hpMjAyNEAxNjMuY29t
Disclaimer: All claims expressed in this article are solely those of the authors and do not necessarily represent those of their affiliated organizations, or those of the publisher, the editors and the reviewers. Any product that may be evaluated in this article or claim that may be made by its manufacturer is not guaranteed or endorsed by the publisher.
Research integrity at Frontiers
Learn more about the work of our research integrity team to safeguard the quality of each article we publish.