- 1Department of Electrical Engineering, Mizoram University, Aizawl, Mizoram, India
- 2Fukushima Renewable Energy Institute, AIST (FREA), Koriyama, Japan
- 3Faculty of Health, Engineering and Science, Victoria University, Melbourne, VIC, Australia
In this paper, the effect of electric vehicles (EVs) on load frequency control (LFC) in the context of a deregulated market within an asymmetric three-area system featuring a novel combination of hybrid power plants is presented. The paper discusses load frequency control within a deregulated market in an unequal three-area system using a new combination of hybrid power plants. All the areas have one renewable energy source and a thermal power plant (TPP), and each area incorporates electric vehicles. Area 1 contains a combination of a wind turbine system (WTS) and thermal, Area 2 has a geothermal power plant (GTPP) and thermal, and Area 3 has a biogas power plant (BPP) and thermal. This proposed system is investigated. Conventional PID, PI, and I controllers are used because they are simple, cheap, and easily available. Their performance is observed and compared. The controller parameters undergo optimization by applying an innovative optimization method called the Mine Blasting algorithm, which utilizes an integral square error (ISE)-based fitness function. The analysis is done under bilateral and contract violation cases with and without generation rate constraints. Moreover, the state of charge (SoC) estimation concept under a deregulated environment and the significance of EVs in the proposed system, especially in the case of contract violation, is presented.
1 Introduction
Frequency control is one of the most important parameters in a power system as it maintains the stability of the system (Ulutas et al., 2020). Although supplying electric energy to consumers, it is imperative to maintain voltage and frequency at their rated values (Hussain I. et al., 2020; Das et al., 2022a). Any mismatch between the demand and supply will lead to deviation in frequency; that is, frequency may rise or fall. If frequency falls, the generation needs to be increased, and if frequency rises, the generation needs to be decreased. These can be interpreted so that if demand changes, the system deviates from the initial or normal values, causing small, unpredictable changes. So, an automated control system is introduced to identify deviations and trigger a series of counter-control measures aimed at rapidly and efficiently eliminating and neutralizing these discrepancies. This is called automatic load frequency control (ALFC) (Kundur, 2018).
There is much literature on the LFC of power systems, and many scholars are doing research in this area. Initially, Elgerd (2007) introduced the concept of power system modeling of a thermal unit, which is expanded by researchers on different types of generating units (Elgerd and Fosha, 1970). The increase in electrical power demand results in increased pollution and decreased fossil fuel stock; thereby, researchers are now concentrating on renewable energy sources (RESs) for power generation because they are free from pollution and running costs (Abdolrasol et al., 2022; Abdolrasol et al., 2023; Mazumdar et al., 2024). Wang, Y. et al. discuss the deregulation system and transfer function of different renewable sources (Wang et al., 1994).
At the same time, studies on the effect of renewable energy in LFC are needed. Das et al. presented the risk of high renewable energy (Das et al., 2022b). Singh et al. mentioned the high penetration level of renewable energy sources (Singh N. J. et al., 2021). Moreover, the type of renewable energy sources chosen is also an important parameter in multi-source power systems. Wind, hydropower, and solar PV are chosen by Ali et al. (2024), wind and solar PV are chosen by Fathy and Alharbi (2021), and hydropower and wind are chosen by (Singh N. K. et al., 2021). In this work, renewable energy sources of wind, geothermal, and BPPs are chosen for investigation because India has good resources for those energy sources. Moreover, the proposed system has not yet been investigated in the case where those power plants are combined with a thermal power plant in each area in a three-area power system.
As pollution is increasing due to the burning of petrol or diesel, many countries are developing an EV policy. Many current studies are investigating EVs to support a green environment. Therefore, vehicle-to-grid (V2G) integration is an important topic, and hence, many researchers are working on the integration of EVs into the grid. Gaur et al. (2019), Vachirasricirikul and Ngamroo (2014), Gaur et al. (2018), and Izadkhast et al. (2015) discuss V2G integration. However, there is still a wide scope of research in incorporating EVs into the LFC of multi-area systems. A study of the state of charge estimation (SoC) of EV batteries is also essential, but the SoC in LFC studies is very rare in literature, especially in the case of a deregulated environment. The SoC concept was mentioned by Deddarma and Dutta (2017), Tappeta et al. (2022), and Hussain et al. (2020b), and it is used in interconnected power systems under conventional environments (Deddarma and Dutta, 2017). However, this concept is not yet used in multi-area multi-source systems under a deregulated market.
1.1 Limitations and motivation
From the above-mentioned literature review, it is observed that the incorporation of three unequal areas under a deregulated environment with the integration of renewable sources and EVs has not yet been investigated. Comparative studies of dynamic responses integrating EVs with SoC and MBA-optimized controllers by considering a deregulated environment under different scenarios need to be explored in LFC. On the basis of the above-mentioned limitations, the motivation and inspiration for this work are based on the points listed below:
1. To achieve a stable and dependable power supply, it is crucial to research and analyze the performance of a multi-area power system in a combination of RESs and EVs with SoC estimation.
2. To achieve better dynamic performance of the considered power system by considering a deregulated environment under different scenarios, it is required to optimize the gain values of the controllers using robust optimization techniques.
3. When integrating a diversified group of RESs with the grid, it becomes more important to review the impact of RESs with EVs on system performance under different sets of operating conditions.
1.2 Novelty and contribution
Considering the above-mentioned motivations, the following novelties are captured:
1. Performance evaluation of the power system considering integration of renewable sources (wind turbine system, geothermal power plant, and biogas power plant) and EVs with the SoC concept in each area under a deregulated scenario.
2. Included the SoC estimation concept in each area of the study system.
3. Address the impact of a combination of RESs and EVs with SoC estimation in LFC under a deregulated environment under different scenarios.
Based on the above novelties, the contributions of the present work are as follows.
(a) Develop a new system with three unequal areas with a combination of renewable sources and EVs with SoC.
(b) Analyses the impact of incorporating EVs in the deregulated environment under different scenarios.
(c) Optimize the gains and other parameters of controllers and compare their performance.
(d) Study the SoC estimation concept under a deregulated environment.
(e) Study the significance of EVs in the case of contract violation.
(f) The dynamic responses are studied by considering a deregulated environment under different scenarios and also comparing the results, including and excluding EVs.
The arrangement of the rest of the paper will be as follows: descriptions of the system are presented in Section 2, simulation results and analysis/discussion are presented in Section 3, and the conclusion is in Section 4.
2 Description
Figure 1 presents the power system transfer function model under study incorporating RESs. The proposed model area capacity is assumed to be 1:2:3. Each area in the proposed system contains different types of energy sources. All areas comprise two energy sources, one of which is a reheat thermal plant: a wind turbine system (WTS) in Area 1, a geothermal plant (GTPP) in Area 2, and a biogas power plant in Area 3. Then, EVs are incorporated in each area to examine the effect of EVs on LFC. All the required nominal system data are given in Appendix A. The MBA algorithm is utilized to tune the gain and other parameters of the controllers. Optimization techniques use the cost function to converge to its optimum value. Cost functions, also known as loss functions or objective functions, are fundamental concepts in machine learning and optimization. The four types of cost functions are integral squared error (ISE), integral absolute error (IAE), integral time-weighted squared error (ITSE), and integral of time multiplied absolute error (ITAE). Equations 1–4 expressed the cost function for ISE, ITAE, ITSE, and IAE, where c and d stand for area numbers (c = 1, 2, 3; d = 2, 3; assuming c ≠ d) (Babu and Saikia, 2021).
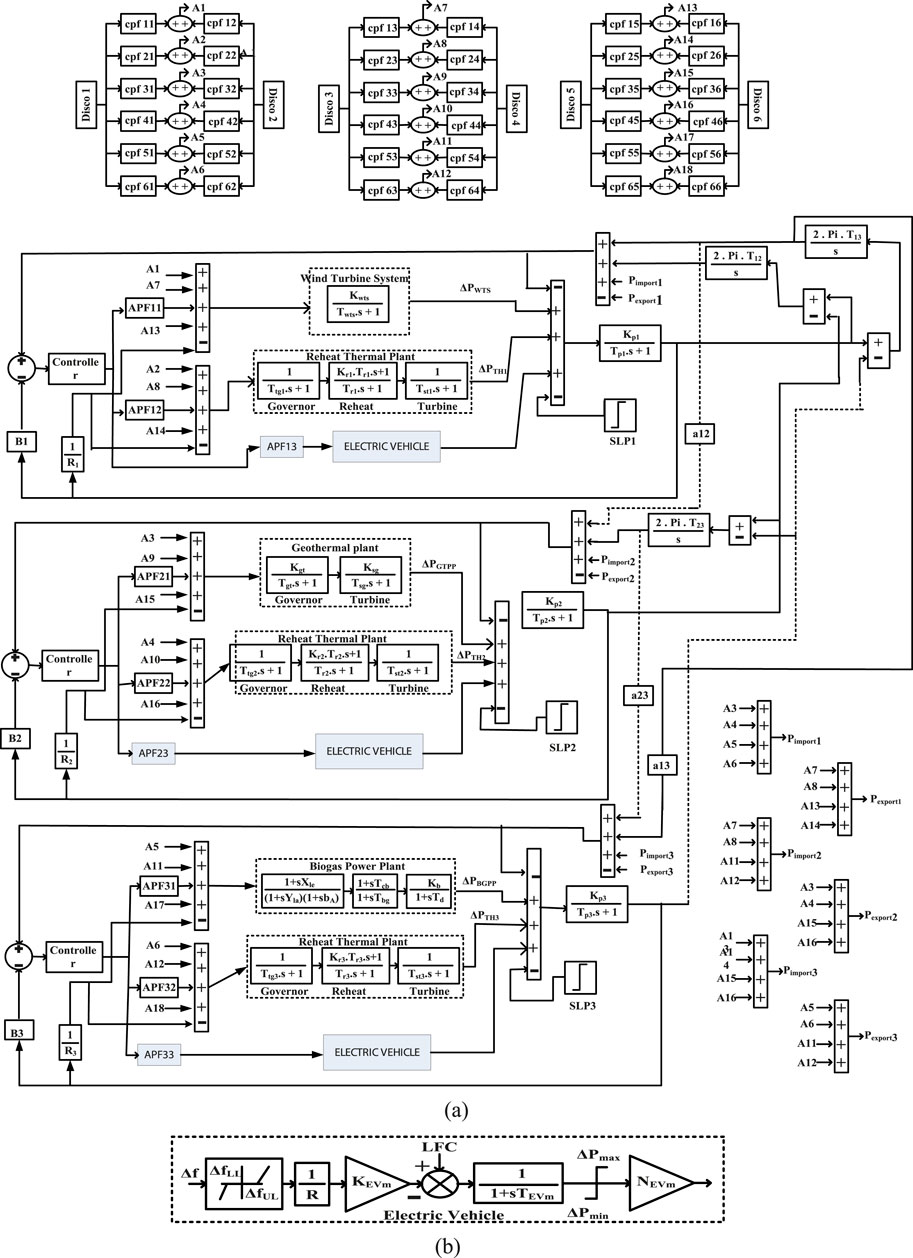
Figure 1. (A) Transfer function model of the three-area hybrid source system. (B) Transfer function model of the electric vehicle.
2.1 Wind turbine system
Wind turbines (WTs), also known as wind generators or windmills, are machines designed to convert wind kinetic energy into electrical power. The amount of power generated by a wind turbine is reliant on the speed of the wind. The pitch angle of the wind turbine blades, which can be adjusted per requirements, plays a vital role in controlling and optimizing power generation in varying wind conditions. The mechanical output power (Pwts) is calculated as Equation 5.
where Cp is the power coefficient, ρ is the density of air, A is the blade-swept area, and υ is the wind speed (Tasnin and Saikia, 2018).
2.2 Geothermal power plant
Geothermal power is one potential source of renewable energy that can be used to generate electric power. Geothermal power plants operate based on the principles of thermodynamics, harnessing heat from the interior of the Earth to produce electricity. The operation of a geothermal power plant (GTPP) involves several key thermodynamic processes, and these processes often include phase changes of the working fluid (Chatuanramtharnghaka et al., 2024).
2.3 Biogas power plant
A biogas power plant (BGPP) utilizes animal droppings and biodegradable wastes to produce biogas used to generate power. They are considered environmentally friendly because they not only produce electricity but also help in waste management by converting biodegradable waste into energy and reducing greenhouse gas emissions compared to letting organic waste decay naturally (Latif et al., 2021). The transfer function model of all these sources is shown in Figure 1A.
2.4 Electric vehicle model
The EV transfer function model is depicted in Figure 1B (Das et al., 2022b). It consists of a battery charger, LFC, and primary frequency control (PFC). The battery charger manages the power exchange between the grid and the battery. The main reason for connecting the EV fleet with the grid is to facilitate participation in LFC during sudden mismatches in load and power generation. Each EV is equipped with a dead band function featuring droop characteristics to mitigate undesired frequency responses upon sudden disconnection from the grid. The dead band value is set as +10 mHz and −10 mHz for the upper limit (∆fUL) and the lower limit (∆fLL), respectively. The value for the droop coefficient of an aggregate model (R) is 2.4 Hz/p.u. MW, which is the same as conventional units. The gain (KEVm) of each EV depends on its SoC, maintained at 1 within the SoC range of 50%–70%. TEVm denotes the battery’s time constant. ∆Pmax and ∆Pmin represent the maximum and minimum power output of EV fleets, respectively. NEVm signifies the number of EVs connected to the grid, set at 2,000, 4,000, and 1,500 for Areas 1, 2, and 3, respectively (Dang et al., 2017).
2.5 State of charge (SoC)
“The SoC describes the remaining discharge capacity of a battery relative to its total discharge capacity. It plays a crucial role in battery management systems (BMS) as many of its functionalities depend on the SoC of individual cells. Accurate SoC measurement is essential to ensure smooth BMS operation and to maximize energy extraction from the battery pack. However, directly measuring SoC is challenging due to its internal nature, relying heavily on the accuracy of the cell model used in the estimation algorithm” (Das S. et al., 2022). SoC can be formulated by its conventional definition as given in Equation 6 below:
where SoC0 is the initial value of the SoC, Qact is the actual capacity of the battery, and i is the current of the battery. i is positive in the discharging mode and negative in the charging mode.
“The SoC of a battery is also defined as the ratio of its current capacity (Q(t)) to the nominal capacity (Qn). The nominal capacity is given by the manufacturer and represents the maximum amount of charge that can be stored in the battery. So, the SoC can be defined as follows (Equation 7):” (Tappeta et al., 2022):
However, in the case of EVs, SoC is co-related with the gain (KEVm), which influences the EVs’ participation in LFC when they are connected to the grid. The KEVm value varies between 0 and 1. An experiment examined the impact of KEVm variation in this range (i.e., 0–1). As KEVm approaches 1, EVs become more active in LFC helping in regulation of the system frequency during load fluctuations. SoC is between 50% and 70% when KEVm is 1; KEVm varies in the range of 0 ≤ KEVm < 1 when SoC is below 50% or above 70% (Deddarma and Dutta, 2017). Therefore, the value of KEVm is taken as 1 in our case study, which means the SoC is estimated as 50%–70%.
2.6 An overview of the proposed system under a deregulated market
In this case study, the proposed system contains six GENCOS and six distribution companies (DISCOs), considering two DISCOs in each area. These generation companies (GENCOs) and DISCOs depend on the cost participation factor (cpfkl) in the DISCO participation matrix as shown in Equation 6; the DISCOs and GENCOs will be communicated to each other similarly to the design by Farooq et al. (2022). In Equation 8, “k” and “l” signify the GENCO and DISCO numbers, respectively.
The cpfkl is expressed in Equation 9 (Babu and Saikia, 2021).
and Equation 10 holds true.
The schedule tie-line power in the areas is given in Equation 11 (Babu and Saikia, 2021):
where
Tieactualpower deviation is expressed in Equation 12:
Tieerrorpower is as in Equation 13.
The area control error (ACE) is given in Equation 14, which is the addition of frequency change and tie-line power error (Gaur et al., 2019).
DISCOs can engage in communication and transactions with GENCOs from any geographical area (bilateral type of power transaction). A contracted demand of 0.01 per unit (pu) megawatts is taken into account for each DISCO, and they can collaborate with various GENCOs across regions. The DISCO participation matrix (DPM) for this specific case study is described by Matrix (Equation 15), which encapsulates the bilateral transactions between each DISCO and GENCO.
3 Results and analysis
Simulations were performed on four different sources, three non-identical and area-based interconnecting power systems under various conditions as shown in Figures 2–12. The capacities of each power source are considered as follows: the WTS and thermal in Area 1 are 10 MW and 90 MW, respectively; the GTPP and thermal in Area 2 are 20 MW and 180 MW, respectively; and the BGPP and thermal in Area 3 are 30 MW and 270 MW, respectively. The scheduled tie-line power within interconnected areas is determined by utilizing matrix Equations 16–21. From the DPM (Equation 15), active power generated by GENCOs under bilateral conditions in a steady state is as follows:
where ∆Pwts, ΔPth1, ∆Pgtpp, ΔPth2, ∆Pbgpp, and ΔPth3 are the incremental generation of the GENCOs in Areas 1, 2, and 3, respectively (Gaur et al., 2019).
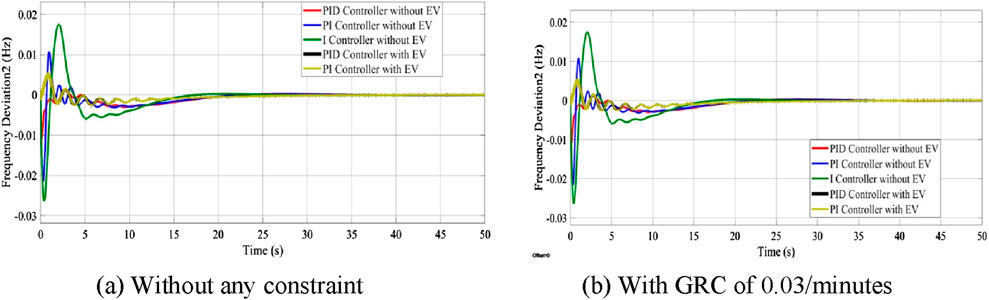
Figure 2. Frequency variation in Area 2 under the bilateral condition. (A) Without any constraint. (B) With a GRC of 0.03/min.
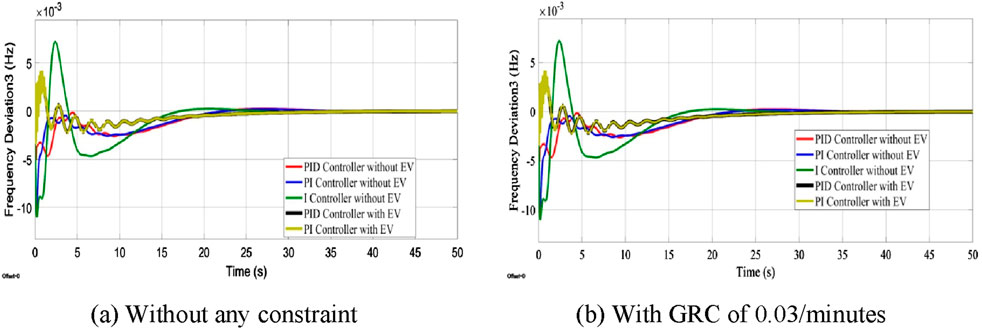
Figure 3. Frequency variation in Area 3 under the bilateral condition. (A) Without any constraint. (B) With a GRC of 0.03/min.
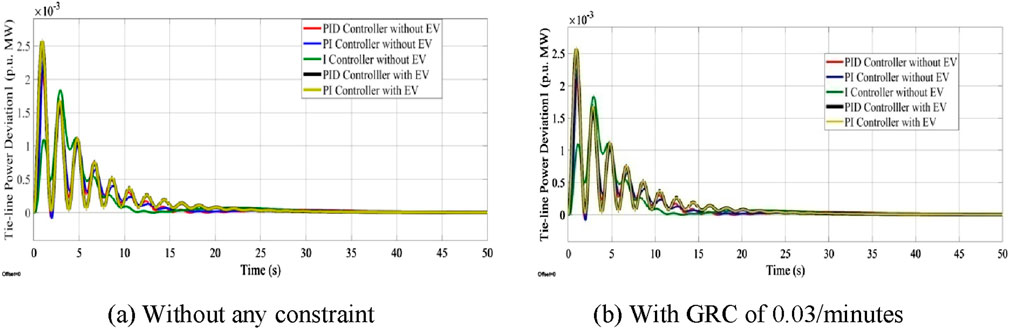
Figure 4. Tie-line power variation between Areas 1 and 2 under the bilateral condition. (A) Without any constraint. (B) With a GRC of 0.03/min.
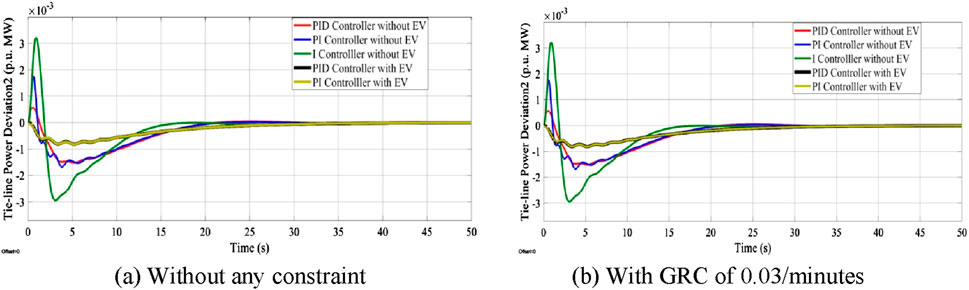
Figure 5. Tie-line power variation between Areas 2 and 3 under the bilateral condition. (A) Without any constraint. (B) With a GRC of 0.03/min.
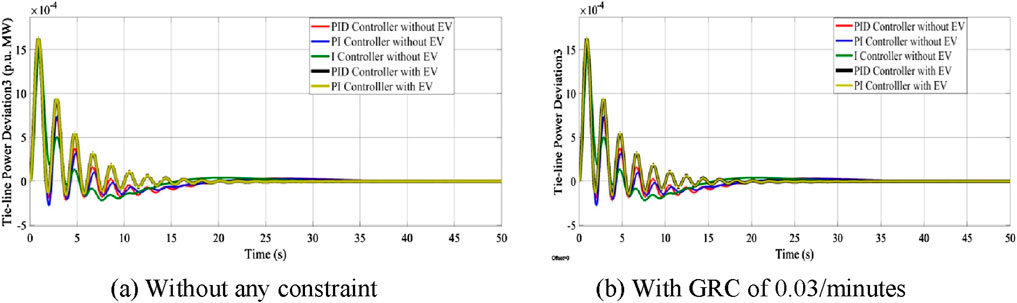
Figure 6. Tie-line power variation between Areas 1 and 3 under the bilateral condition. (A) Without any constraint (B) With a GRC of 0.03/min.
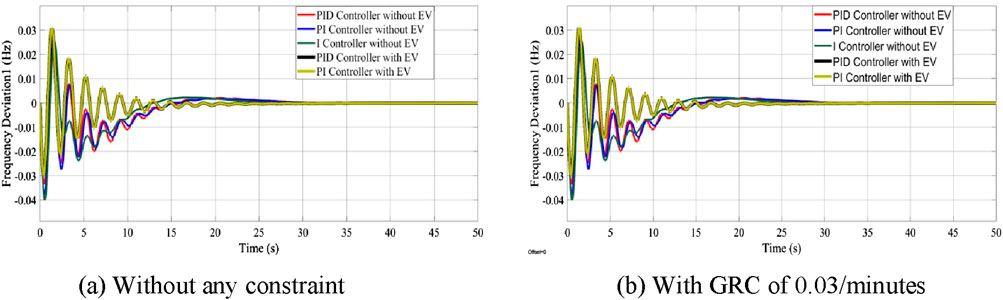
Figure 7. Frequency variation in Area 1 under a contract violation. (A) Without any constraint. (B) With a GRC of 0.03/min.
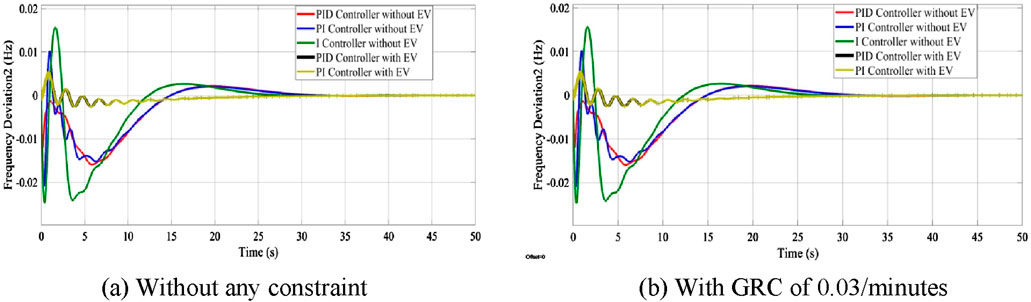
Figure 8. Frequency variation in Area 2 under a contract violation. (A) Without any constraint. (B) With a GRC of 0.03/min.
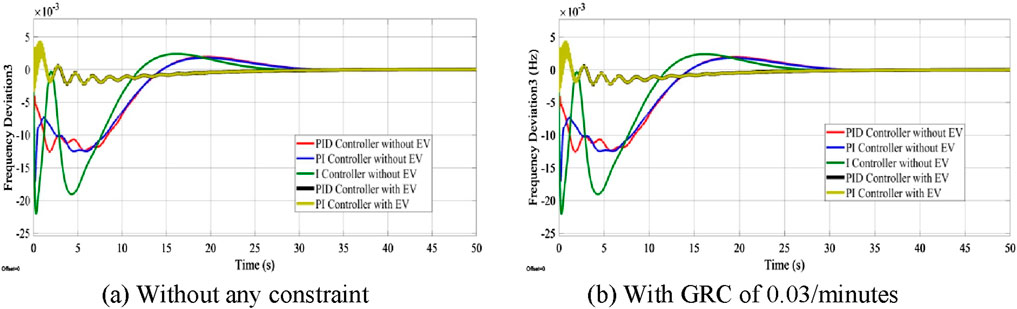
Figure 9. Frequency variation in Area 3 under a contract violation. (A) Without any constraint. (B) With a GRC of 0.03/min.
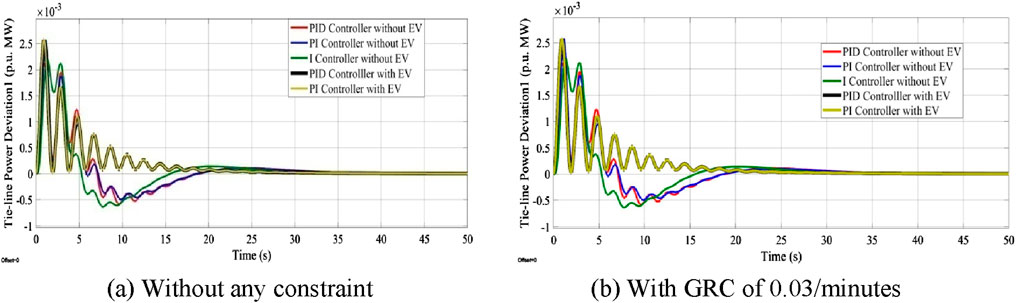
Figure 10. Tie-line power variation between Areas 1 and 2 under a contract violation. (A) Without any constraint. (B) With a GRC of 0.03/min.
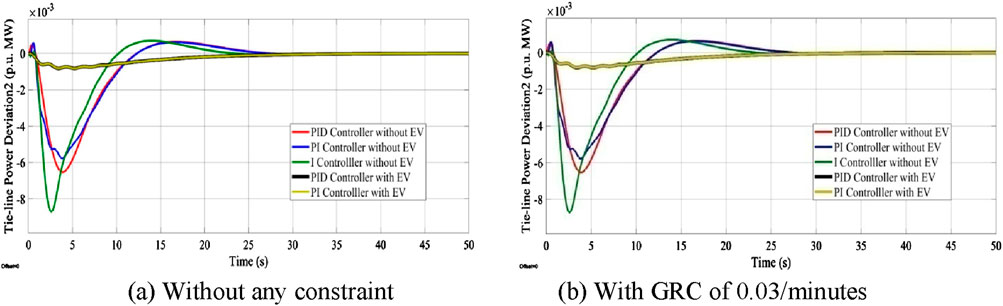
Figure 11. Tie-line power variation between Areas 2 and 3 under a contract violation. (A) Without any constraint. (B) With a GRC of 0.03/min.
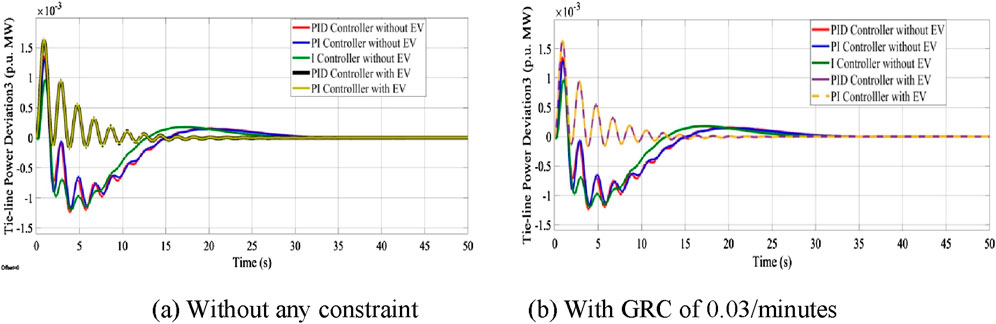
Figure 12. Tie-line power variation between Areas 1 and 3 under a contract violation. (A) Without any constraint. (B) With a GRC of 0.03/min.
In this case study, the PID, PI, and I controllers are used to monitor the dynamic responses of the system. The MBA algorithm is utilized to tune gain values and other parameters of the controllers. The results obtained are performed under (i) a bilateral condition with no non-linearity, (ii) a bilateral condition with a 3% Generation Rate Constraint (GRC), (iii) a contract violation in Area 3 with no non-linearity, and (iv) a contract violation in Area 3 with a 3% GRC. The SoC of each EV is 50%–70%.
3.1 Case 1: bilateral condition
In this case, simulation is performed as per the contract agreement (a) with no constraint or non-linearity and (b) with a GRC. Optimum values of PID, PI, and I controllers shown in Table 1 are used.
As per the results obtained above, the proposed system gives a stable response. There is no large difference in the responses when EVs are included or not in the case of bilateral conditions. However, it can be observed that the responses are a little better when EVs are included in the system except in frequency variation in Area 1 (Figure 13). Regardless of the controller used, the EVs themselves help to improve the system responses. As per simulation results, the PID controller gives better dynamic responses than the PI and I controllers as shown in Table 2.
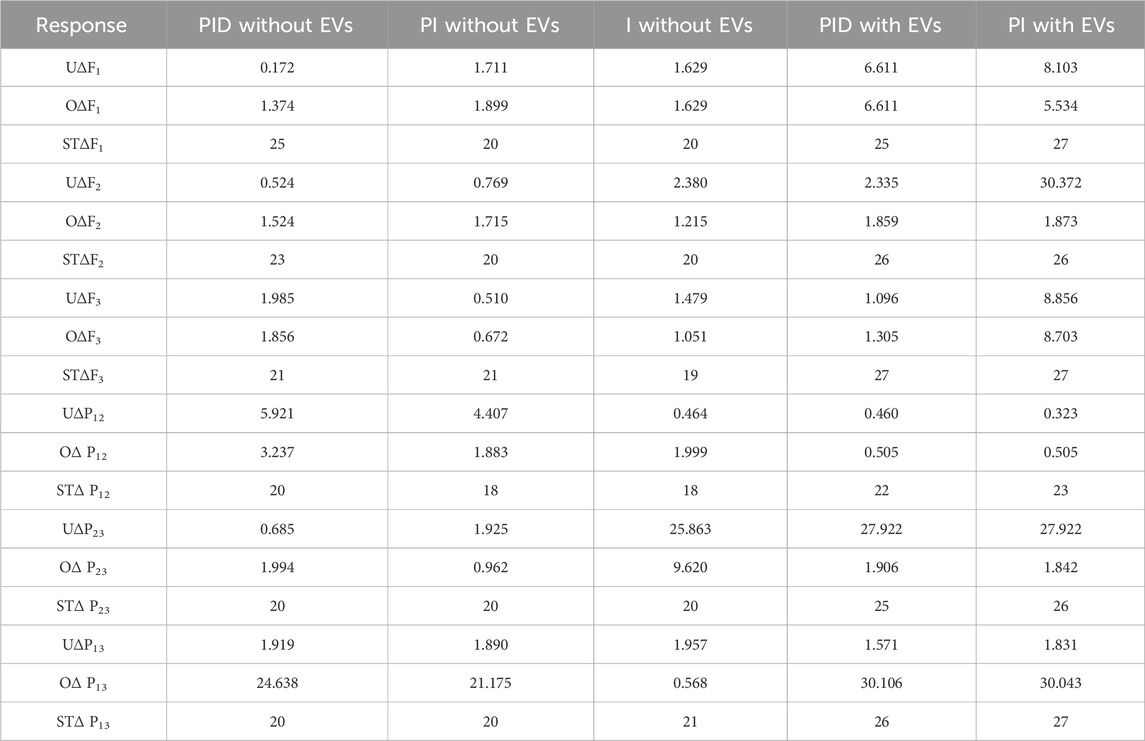
Table 2. Undershoot (U−), peak overshoot (O+), and settling time (ST) of responses considering different controllers under the bilateral condition.
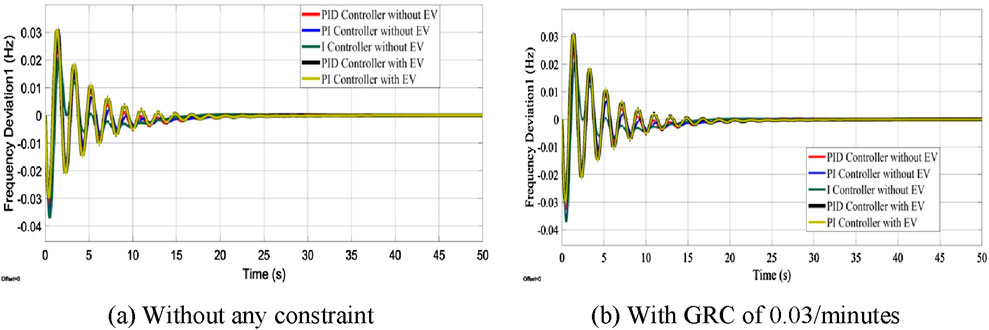
Figure 13. Frequency variation in Area 1 under the bilateral condition. (A) Without any constraint. (B) With a GRC of 0.03/min.
3.2 Case 2: contract violation
In this case, simulation is performed under contract violation. (DISCO exceeds its contracted power demand; the additional power must be supplied by local power sources). The DISCO in Area 3 demands extra power of 0.01 p.u. MW, violating the contract. This additional load request will be managed by power sources in Area 3.
(a) Without incorporating EVs, the biogas power plant and thermal power plant-3 will manage power requirements, and their power generation can be calculated as in Equations 22, 23.
(b) When EVs are incorporated, the biogas power plant, thermal power plant-3, and EVs will manage the power requirements, and their power generation can be calculated as in Equations 24, 25.
This means each EV will produce 0.0010 pu MW power. The simulation results obtained are given below.
In this case, the proposed system also gives a stable response with the Optimum values of PID, PI, and I controllers shown in Table 3. As per the results obtained, it can be seen that the responses are much better when EVs are included in the system. Regardless of the controller used, the EVs themselves help to improve the system responses. As shown in Table 4, the responses are more or less the same when using a PID or PI controller when EVs are included in the system. EVs also make significant contributions in the case of contract violation by giving sufficient power to meet the extra demand from that area, as shown in Figures 14, 15. As per simulation results, the PID controller gives better dynamic responses than the PI and I controllers.
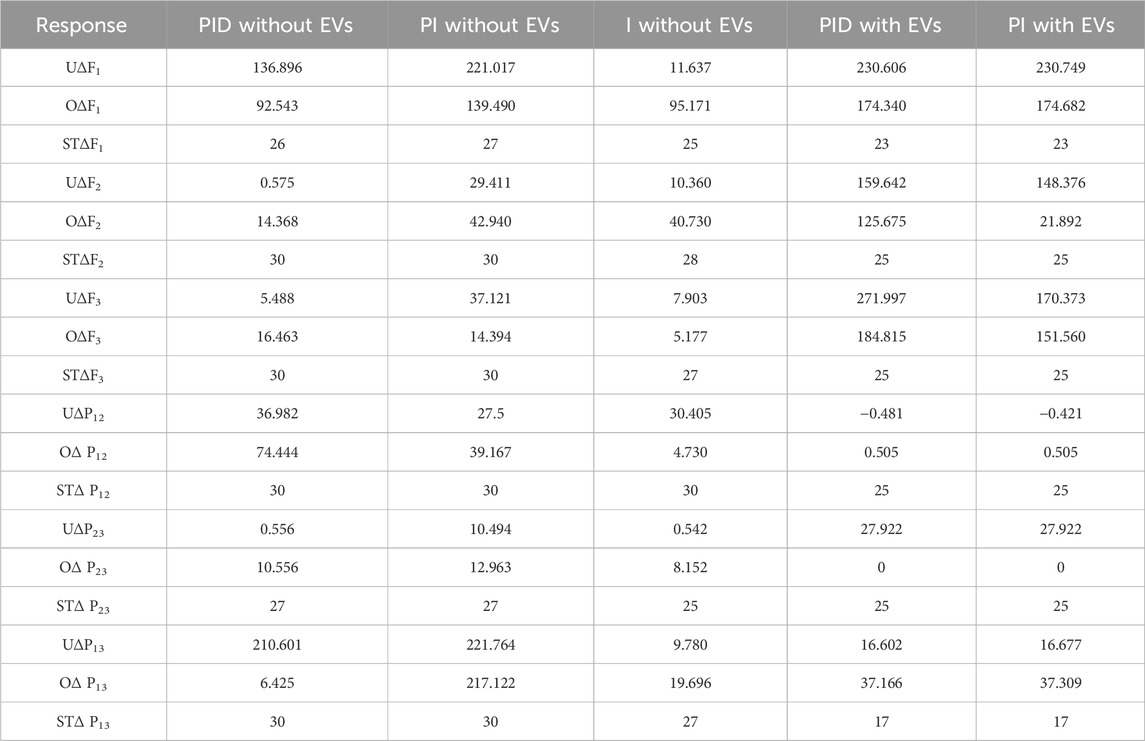
Table 4. Undershoot (U−), peak overshoot (O+), and settling time (ST) of responses considering different controllers under a contract violation.
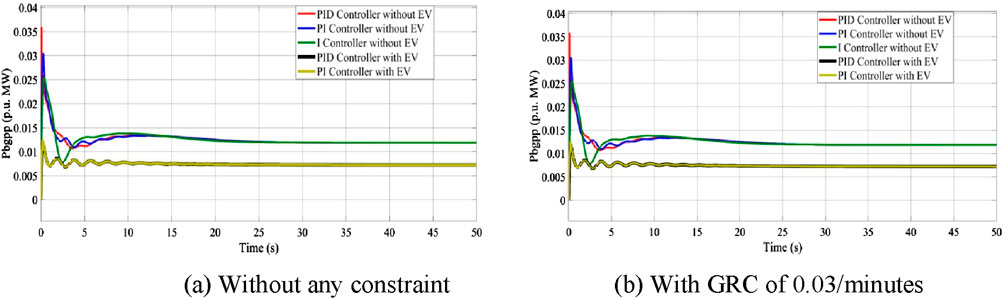
Figure 14. Power generated by a biogas power plant under a contract violation. (A) Without any constraint (B) With a GRC of 0.03/min.
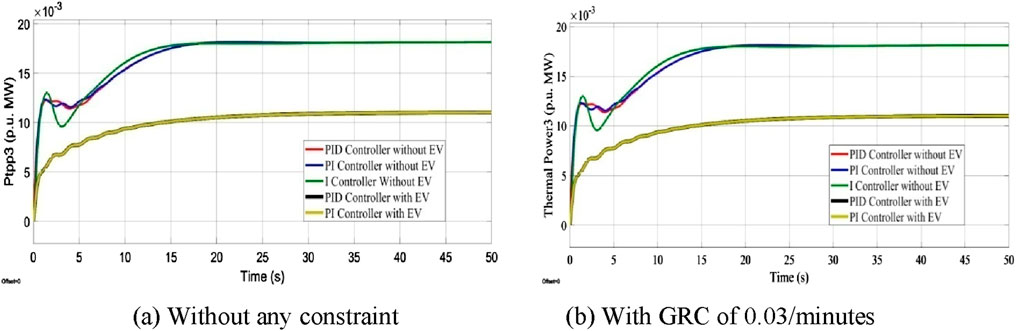
Figure 15. Power produced by a thermal power plant in Area 3 under a contract violation. (A) Without any constraint. (B) With a GRC of 0.03/min.
4 Conclusion
In this work, an ALFC in a multi-area system incorporating EVs along with renewable sources under a deregulated market is presented. The system has three areas with two power sources in each area. Each area comprises one renewable source along with a thermal unit; this combination is new in the literature. The proposed system is tested successfully under bilateral and contract violation types of power transactions using classical controllers with and without a GRC. The effect of EVs under a deregulated environment is studied successfully by estimating the SoC of each EV at 50%–70%. The findings show that the EVs play a vital role in controlling tie-line power deviation and frequency fluctuation under both the bilateral condition and contract violation cases. Moreover, EVs also make significant contributions in the case of contract violation by giving sufficient power to meet the extra demand from that area. Hence, the conclusion can be made that incorporating EVs may play a vital role in LFC under the deregulated environment in the near future. In this work, few RESs are considered in the multi-area multi-source power system to study the dynamic performance of the system; that is, limited RESs like wind, biogas, and geothermal are considered. Therefore, this work can extend to large traditional and deregulated multi-area power systems while integrating other RESs such as wave energy, tidal, solar PV, etc.
Data availability statement
The original contributions presented in the study are included in the article/supplementary material; further inquiries can be directed to the corresponding authors.
Author contributions
FL: writing–original draft and writing–review and editing. SD: writing–original draft and writing–review and editing. SL: writing–original draft and writing–review and editing. TU: writing–original draft and writing–review and editing. AK: writing–original draft and writing–review and editing.
Funding
The author(s) declare that no financial support was received for the research, authorship, and/or publication of this article.
Conflict of interest
The authors declare that the research was conducted in the absence of any commercial or financial relationships that could be construed as a potential conflict of interest.
Generative AI statement
The author(s) declare that no Generative AI was used in the creation of this manuscript.
Publisher’s note
All claims expressed in this article are solely those of the authors and do not necessarily represent those of their affiliated organizations, or those of the publisher, the editors and the reviewers. Any product that may be evaluated in this article, or claim that may be made by its manufacturer, is not guaranteed or endorsed by the publisher.
References
Abdolrasol, M. G. M., Ayob, A., Mutlag, A. H., and Ustun, T. S. (2023). Optimal fuzzy logic controller based PSO for photovoltaic system. Energy Rep. 9, 427–434. doi:10.1016/j.egyr.2022.11.039
Abdolrasol, M. G. M., Hannan, M., Hussain, S. S., and Ustun, T. S. (2022). Optimal PI controller based PSO optimization for PV inverter using SPWM techniques. Energy Rep. 8, 1003–1011. doi:10.1016/j.egyr.2021.11.180
Ali, T., Muhammad, A., Touti, E., Graba, B. B., Aoudia, M., Abbas, G., et al. (2024). Terminal voltage and load frequency control in a real four-area multi-source interconnected power system with nonlinearities via OOBO algorithm. IEEE Access 12, 123782–123803.
Babu, N. R., and Saikia, L. C. (2021). Load frequency control of a multi-area system incorporating realistic high-voltage direct current and dish-Stirling solar thermal system models under deregulated scenario. IET Renew. Power Gener. 15, 1116–1132. doi:10.1049/rpg2.12093
Chatuanramtharnghaka, B., Deb, S., Singh, K. R., Ustun, T. S., and Kalam, A. (2024). Reviewing demand response for energy management with consideration of renewable energy sources and electric vehicles. World Electr. Veh. J. 15, 412. doi:10.3390/wevj15090412
Dang, X. J., Yan, L., Jiang, H., Wu, X. R., and Sun, H. X. (2017). Open-circuit voltage-based state of charge estimation of lithium-ion power battery by combining controlled auto-regressive and moving average modeling with feedforward-feedback compensation method. Int. J. Electr. Power and Energy Syst. 90, 27–36. doi:10.1016/j.ijepes.2017.01.013
Das, A., Dawn, S., Gope, S., and Ustun, T. S. (2022a). A strategy for system risk mitigation using FACTS devices in a wind incorporated competitive power system. Sustainability 14, 8069. doi:10.3390/su14138069
Das, A., Dawn, S., Gope, S., and Ustun, T. S. (2022b). A risk curtailment strategy for solar PV-battery integrated competitive power system. Electronics 11, 1251. doi:10.3390/electronics11081251
Das, S., Saikia, L. C., and Datta, S. (2022c). Effect of PI-TI based virtual inertia controller with virtual damping on a renewable energy based multi-area power system under deregulation. Arabian J. Sci. Eng. 48, 6431–6452. doi:10.1007/s13369-022-07376-2
Deddarma, S., and Dutta, A. (2017). Utilizing electric vehicles for LFC in restructured power systems using fractional order controller. IEEE Trans. Smart Grid 8, 2554–2564. doi:10.1109/tsg.2016.2527821
Elgerd, O. I., and Fosha, C. (1970). Optimum megawatt frequency control of multi-area electric energy systems. IEEE Trans. Power Appl. Syst. 89 (4), 556–563. doi:10.1109/tpas.1970.292602
Farooq, Z., Rahman, A., Hussain, S., and Ustun, T. S. (2022). Power generation control of renewable energy based hybrid deregulated power system. Energies 15, 517. doi:10.3390/en15020517
Fathy, A., and Alharbi, A. G. (2021). Recent approach based movable damped wave algorithm for designing fractional-order PID load frequency control installed in multi-interconnected plants with renewable energy. IEEE Access 9, 71072–71089. doi:10.1109/access.2021.3078825
Gaur, P., Bhowmik, D., and Soren, N. (2019). Utilisation of plug-in electric vehicles for frequency regulation of multi-area thermal interconnected power system. IET Energy Syst. Integr. 1 (2), 88–96. doi:10.1049/iet-esi.2018.0028
Gaur, P., Soren, N., and Bhowmik, D. (2018). Impact assessment of vehicle-to-grid technology in LFC of multi-area solar-thermal power system. Int. J. Renew. Energy Res. 8 (3), 1580–1590.
Hussain, I., Das, D. C., Sinha, N., Latif, A., Hussain, S. M. S., and Ustun, T. S. (2020a). Performance assessment of an islanded hybrid power system with different storage combinations using an FPA-tuned two-degree-of-freedom (2DOF) controller. Energies 13, 5610. doi:10.3390/en13215610
Hussain, S. M. S., Aftab, M. A., Ali, I., and Ustun, T. S. (2020b). IEC 61850 based energy management system using plug-in electric vehicles and distributed generators during emergencies. Int. J. Electr. Power and Energy Syst. 119, 105873. doi:10.1016/j.ijepes.2020.105873
Izadkhast, S., Garcia-Gonzalez, P., and Frías, P. (2015). An aggregate model of plug-in electric vehicles for primary frequency control. IEEE Trans. Power Syst. 30 (3), 1475–1482. doi:10.1109/tpwrs.2014.2337373
Latif, A., Hussain, S. M. S., Das, D. C., and Ustun, T. S. (2021). Optimization of two-stage IPD-(1+I) controllers for frequency regulation of sustainable energy based hybrid microgrid network. Electronics 10, 919. doi:10.3390/electronics10080919
Mazumdar, D., Biswas, P. K., Sain, C., Ahmad, F., Ustun, T. S., and Kalam, A. (2024). Performance analysis of drone sqadron optimisation based MPPT controller for grid implemented PV battery system under partially shaded conditions. Renew. Energy Focus 49 (2024), 100577. doi:10.1016/j.ref.2024.100577
Singh, N. J., Singh, S., Chopra, V., Aftab, M. A., Hussain, S. M. S., and Ustun, T. S. (2021a). Chaotic evolutionary programming for an engineering optimization problem. Appl. Sci. 11, 2717. doi:10.3390/app11062717
Singh, N. K., Koley, C., Gope, S., Dawn, S., and Ustun, T. S. (2021b). An economic risk analysis in wind and pumped Hydro energy storage integrated power system using meta-heuristic algorithm. Sustainability 13, 13542. doi:10.3390/su132413542
Tappeta, V. S. R., Appasani, B., Patnaik, S., and Ustun, T. S. (2022). A review on emerging communication and computational technologies for increased use of plug-in electric vehicles. Energies 15, 6580. doi:10.3390/en15186580
Tasnin, W., and Saikia, L. C. (2018). Comparative performance of different energy storage devices in AGC of multi-source system including geothermal power plant. J. Renew. Sustain. Energy 10, 0241015. doi:10.1063/1.5016596
Ulutas, A., Altas, I. H., Onen, A., and Ustun, T. S. (2020). Neuro-Fuzzy-based model predictive energy management for grid connected microgrids. Electronics 9, 900. doi:10.3390/electronics9060900
Vachirasricirikul, S., and Ngamroo, I. (2014). Robust LFC in a smart grid with wind power penetration by coordinated V2G control and frequency controller. IEEE Trans. Smart Grid 5 (1), 371–380. doi:10.1109/tsg.2013.2264921
Wang, Y., Zhou, R., and Wen, C. (1994). New robust adaptive load-frequency control with system parametric uncertainties. IET Proc. - Generation, Transm. Distribution 141 (3), 184–190.
Appendix A
Nominal system parameters are as follows:
1. Power system: f = 60 Hz; Rc = 2.4 Hz/p.u. MW; βc = 425 × 10−3 p.u. MW/Hz; Kpc = 120 Hz/p.u. MW; Tpc = 20 s; Tcd = 0.086 s; Suffix c,d = Areas 1, 2, and 3.
2. WTS: Kwts = 1; Twts = 1.5 s.
3. GTPP: Kgt = 1; Ksg = 1; Tgt = 0.1299 s; Tsg = 0.1 s
4. BGPP: Yla = 0.041 s; bA = 0.05 s; Xle = 1; Tcb = 0.5 s; Tbg = 0.5 s; Kb = 0.8; Td = 0.5 s.
5. Reheat thermal plant: Tsg = 0.08 s; Tt = 0.3 s; Tr = 10 s; Kr = 0.5.
6. Electric vehicle: KEVm = 1; NEV1 = 2,000; NEV2 = 4,000; NEV3 = 1,500;
7. Charging and discharging = ±50 KW; ∆fUL and ∆fLL = ±10 mHz.
Keywords: LFC, multi area system, renewable source, electric vehicle, SoC, deregulation
Citation: Lalhmangaihzuala F, Datta S, Lalngaihawma S, Ustun TS and Kalam A (2025) A renewable integrated multi-area system for LFC incorporating electrical vehicle with SoC estimation. Front. Energy Res. 12:1508391. doi: 10.3389/fenrg.2024.1508391
Received: 09 October 2024; Accepted: 18 December 2024;
Published: 20 January 2025.
Edited by:
Yirui Wang, Ningbo University, ChinaReviewed by:
Youcef Belkhier, Université de Bretagne Occidentale, FranceZahid Farooq, National Institute of Technology, Srinagar, India
Copyright © 2025 Lalhmangaihzuala, Datta, Lalngaihawma, Ustun and Kalam. This is an open-access article distributed under the terms of the Creative Commons Attribution License (CC BY). The use, distribution or reproduction in other forums is permitted, provided the original author(s) and the copyright owner(s) are credited and that the original publication in this journal is cited, in accordance with accepted academic practice. No use, distribution or reproduction is permitted which does not comply with these terms.
*Correspondence: Subir Datta, c3ViaXJuZXJpc3RAZ21haWwuY29t; Taha Selim Ustun, c2VsaW0udXN0dW5AYWlzdC5nby5qcA==; Akhtar Kalam, YWtodGFyLmthbGFtQHZ1LmVkdS5hdQ==