- 1Department of Resource and Environmental Engineering, Technical University of Denmark, Kongens Lyngby, Denmark
- 2Tomas Ekvall Research, Review and Assessment, Gothenburg, Sweden
- 3Department of Sustainability and Planning, Aalborg University, Aalborg, Denmark
- 4A.P. Møller-Mærsk, Copenhagen, Denmark
Various regional and international standards have been developed to measure the environmental impacts of transportation fuels and minimize greenwashing and misinformation regarding their sustainability. These frameworks offer standardized methods and calculation guidelines for fuel producers to be able to verify compliance with predefined sustainability criteria and to achieve greenhouse gas emission reduction targets. However, significant inconsistencies exist among these standards in terms of methods, calculation rules, and default values assigned to specific fuels. This study reviews and analyses five fuel standards, namely the European Renewable Energy Directive, the United Nation’s Carbon Offsetting and Reduction Scheme for International Aviation, the California Low Carbon Fuel Standard, the United States Renewable Fuel Standard, and the UK Renewable Transport Fuel Obligation. A qualitative analysis of the different schemes’ methods identified several discrepancies. These were found to be primarily related to the modelling approach used, the burdens and credits arising from different feedstock types and co-products, and the modelling of electricity and land use changes. An example of this is that different standards provide credits for certain waste types, such as animal manure in the RED and RTFO, or municipal solid waste in CORSIA. In addition to the qualitative analysis, the carbon intensity was calculated – according to the rules set out by these frameworks – for case studies of eight fuel types, including biofuels and electrolysis-based fuels. These calculations further highlighted how the use of different fuel standards can lead to conflicting assessments of a fuel’s environmental impact. Overall, our findings demonstrate substantial variations in the methods and calculation rules prescribed by the five standards, often resulting in markedly different carbon intensity scores for the same fuel. Based on this analysis, we propose specific changes to the calculation rules to enhance harmonization and improve the accuracy in reflecting the environmental consequences of fuel production and use. These recommendations include that indirect land use changes are always included, and more transparency regarding the methods for calculating the fuel carbon footprint.
1 Introduction
The transportation sector is responsible for a fifth of global annual greenhouse gas (GHG) emissions, and is only expected to grow in the coming years, with increased demand for goods (distributed via road, rail and sea), and personal transport like aviation (IEA, 2023; Tjandra et al., 2024). At the same time, countries and international organizations are beginning to set ambitious GHG reduction targets, such as the International Maritime Organization’s (IMO) target of 70% reduction in annual GHG emissions from the international shipping fleet by 2040 (IMO, 2023a). Considering that 95% of the global transportation fleet is still running on fossil fuels (DNV, 2023), and that the combustion of these fuels contributes most to the sector’s climate change impacts, the transition away from fossil fuels for transportation is imminent. However, it is necessary to comprehensively assess the impacts of alternative transportation fuels to avoid burden-shifting.
The environmental impacts of products, like transportation fuels, can be assessed using life cycle assessment (LCA). LCA aims to assess the impacts of a product or process over its entire “life cycle” (i.e. from raw material extraction up until end-of-life management) and includes multiple environmental impact categories. Similarly, carbon footprint calculations consider only climate change impacts of a product, but still in a life cycle perspective. Carbon footprint calculations are often used in regulatory frameworks and standards, to be able to compare the climate change impacts of various products to fulfil a purpose. However, these calculations can be made with different methods that generate widely diverging results (Brandão et al., 2022; Konradsen et al., 2024). While standardized LCAs follow ISO 14040 and 14,044 guidance (International Organization for Standardization, 2006a; International Organization for Standardization, 2006b; International Organization for Standardization, 2018) on how to deal with multi-functionality and impacts in the broader system, most regulatory schemes make arbitrary choices, often geared towards incentivizing a targeted behavior in the jurisdiction in question.
The outcomes of a carbon footprint calculation of a transportation fuel can vary depending on the methodological choices and assumptions made in the calculation. For biofuels, the modelling of the impacts associated with the land that the biomass is cultivated on – also known as land use changes (LUC) – can drastically change the GHG performance of the fuel (Barnabe et al., 2013; Creutzig et al., 2015; Plevin et al., 2014; Repo et al., 2015; Searchinger et al., 2022; Valin et al., 2015). For other fuel types, the type of electricity or heat used in the fuel production can be of particular relevance. In other cases, the feedstock type used for the fuel can be particularly influential on the results. For example, a study by Brandão et al. (2022) assessed eight different production pathways for fuels produced from biomass and/or waste, using methods and guidelines from the Renewable Energy Directive (RED). The study found that the approach for modelling waste management can be crucial for waste-based fuels, and the approach to allocation among co-products can be very important for many kinds of fuels.
When explaining such differences, it is useful to distinguish between attributional and consequential life cycle studies, because they are associated with different calculation methods. An attributional life cycle assessment (ALCA) or carbon footprint estimates the share of the global environmental impact that can be defined as “belonging” to the product under study. It does so by modelling the supply chain or the value chain of the product (Weidema et al., 2018), or a mix thereof, and the potential environmental impacts of this system. The system boundaries and calculation rules of an ALCA are defined according to normative rules (UNEP/SETAC Life Cycle Initiative, 2011). A consequential LCA (CLCA), in contrast, estimates how the global environmental impacts are affected as a consequence of a change in demand for the functional unit (UNEP/SETAC Life Cycle Initiative, 2011). Thereby, many regulatory schemes that include GHG accounting often have elements from both consequential and attributional LCAs.
This study reviews the calculation rules in five different transportation fuel standards: the RED, the United Kingdom’s Renewable Transport Fuel Obligation (RTFO), the California Low Carbon Fuel Standard (LCFS) the United States’ Renewable Fuel Standard (RFS) and the Carbon Offsetting and Reduction Scheme for International Aviation (CORSIA). These standards each hold their own embedded aims that drive the guidelines for calculating the carbon footprint of a fuel.
In addition, this study demonstrates how these different guidelines can result in varied carbon footprint scores for the same fuel type and discusses what this means for fuel producers and the transportation sector. The advantages, disadvantages, and implications of the different methodological choices in the fuel standards are discussed. On this basis, recommendations are made with the standpoint that the fuel carbon intensity score should ideally reflect the foreseeable consequences of producing and using the fuel.
The study is organized into four main sections. In Section 2, we provide an overview of the different approaches to LCA modelling, introduce the fuel standards, and present the methods used in the different fuel standards. In Section 3, we compare the carbon intensity scores of eight fuel pathways provided by each fuel standard. In Section 4, we discuss the implications of differences between the fuel standards with regards to methodological approaches and carbon intensity scores, and specific recommendations for improvements to the fuel standards are provided in Section 5.
2 Methods and approaches in the renewable fuel standards
First, the concepts of consequential and attributional modelling approaches are introduced (Section 2.1). Then, the fuel standards and their respective overarching aims are presented (Section 2.2). The five standards are then discussed according to i) how they include direct and indirect LUC (Section 2.3), ii) how they manage distribution of impacts between residue and waste feedstock types, and allocation (Section 2.4), and iii) the data used for electricity and emissions (Section 2.5 and 2.6).
2.1 Consequential vs. attributional LCA approaches
Generally, two different approaches to modelling in life cycle assessment exist: consequential (CLCA) and attributional (ALCA) modelling (Sonnemann and Vigon, 2011). According to Sonnemann and Vigon (2011), consequential modelling is defined as a “system modelling approach in which activities in a product system are linked so that activities are included in the product system to the extent that they are expected to change as a consequence of a change in demand for the functional unit.” and attributional modelling is defined as a “system modelling approach in which inputs and outputs are attributed to the functional unit of a product system by linking and/or partitioning the unit processes of the system according to a normative rule”. These definitions are used when discussing “consequential” and “attributional” approaches in this work.
In practice this means that multi-functionality, i.e. when one activity supplies more than one product, is dealt with by applying substitution (synonym to system expansion) in a consequential approach and mainly partitioning/allocation in an attributional approach. For CLCA, this means that demanding by-products, which are constrained by the demand for the main product, will not affect the supplying activity, but instead the marginal/flexible supplier. And when demanding wastes, this will avoid the marginal (most likely alternative) waste treatment, e.g. demand of municipal solid waste will have the effect that landfill or incineration is avoided (Weidema et al., 2009). For ALCA, when demanding by-products, this means that a share (allocated) of the supplying activity (and its upstream) is included. Furthermore, CLCA applies market supply mixes that represent the suppliers, which are expected to change their production as a consequence of a change in demand, whereas ALCA applies market average supply for a given year. Other relevant differences between CLCA and ALCA with regard to the modelling of biofuels are the way to account for biogenic CO2 and land use changes.
2.1.1 Land use changes
Land use changes can be direct (dLUC) or indirect (iLUC). iLUC are accounted for in CLCA, but not in ALCA (Brandão et al., 2021). In ALCA, dLUC in most cases refers to historical land use changes on the actual used land. In CLCA, land use changes are the difference between the land use (dLUC and iLUC) with the demand and production of the functional unit, and its counterfactual, i.e. without the functional unit. In CLCA, dLUC refers to changes on the same land, where the crops for e.g. biofuel feedstock are grown, while iLUC are the changes in land use at other locations caused by the missing crop supply from the land where the crops of interest are grown. iLUC includes expansion and intensification of agricultural land, and potentially also reduced consumption caused by changes in crop prices (Schmidt et al., 2015).
2.1.2 Waste and residue use
In an attributional approach, wastes and residues often enter the system boundary burden free, i.e. there is an assumed impact of zero for their production. At times, attributional approaches assign burdens to residues depending on their perceived use, i.e. straw is a useful product with a market value, which is used to partition burdens between straw and grain. In a consequential approach, waste and residues are modeled by using counterfactual situations, e.g. if straw is not collected, it will decay on agricultural fields. If the counterfactual is not considered when modelling the impacts of wastes and residues, and assigning no burdens to waste and residues, this may underestimate their impact because current beneficial effects of uses of the waste are not accounted for, when the waste is diverted from this beneficial use. An example is used cooking oil (UCO), a limited feedstock, which is not able to react to changes in demand. The counterfactual situation would likely be the induced increased use of vegetable oil as a biofuel feedstock because the current “treatment” of used cooking oil is for biodiesel feedstock. When a specific buyer or country source used cooking oil, the current users will have to switch to alternative biodiesel feedstock, because the supply of it is constrained.
2.1.3 Co-products and multi-functionality
Modelling processes with multiple product outputs by allocation is inadequate from a consequential perspective because allocated processes do not exist in reality. Instead, the modelled system should include the induced substitutions caused by the by-products. Here it is useful to distinguish between determining and dependent co-products or flows. Determining flows are the ones that determine the production volume of an activity; for example, the demand for soybean meal determines the production volume of soybean crushing and cultivation. Dependent flows are constrained because their quantities are determined by the determining flows. Therefore, a change in demand for a dependent flow (e.g., soybean oil) will not affect the soybean system, but the marginal supply of vegetable oil – probably palm oil – is likely to be affected instead (Schmidt, 2015).
2.1.4 Electricity data
In ALCA, the national average supply mix of electricity is most often used. In some cases, a distinction is made between renewable electricity and a residual, still based on annual averages. In CLCA, a marginal mix is applied. The marginal mix is composed to best reflect which electricity sources will be affected by a change in demand for electricity. The marginal mix is composed based on increase rates of supply, and on lifetimes of installed capacity (Muñoz et al., 2015; Muñoz and Weidema, 2023).
2.2 Overview of the fuel standards
The EU established the RED in 2009 to promote the use of energy from renewable sources. It has been revised twice (EC, EC, 2018; EC, 2023c) and complemented by Delegated regulations on renewable fuel of non-biological origin (RFNBOs) and recycled carbon fuels (RCFs) (EC, 2023a; EC2023b).
The current RED requires that 42.5% of the gross final energy use in the EU be renewable in the year 2030 (EC, 2023c). To count as renewable energy, a transportation fuel must meet several sustainability criteria. For example, the life cycle GHG emissions must be 50%–70% lower compared to the competing fossil fuel – the exact level depends on when the fuel-production plant was installed and the type of fuel (EC, 2018).
The RTFO scheme was developed by the UK government under the RED to support the development of renewable transport fuels and reduce emissions. The scheme covers fossil and renewable fuels used in road and certain non-road transport applications, such as non-road mobile machinery and tractors. The RTFO requires from each supplier of such fuel that a percentage of the fuel in excess of 450,000 sold liters/year is certified. This percentage increases gradually from 10.1% in 2021 to 17.4% from the year 2032 (UKDfT, 2023). Third party certification is required. Fuel producers that are registered in the RTFO scheme receive a Renewable Transport Fuel Certificate (RTFC) for each unit of fuel that meets the RTFO requirements. A unit is a liter for most liquid fuels and a corresponding quantity for other fuels (UKDfT, 2023). The certificates can either be used by the producer to meet their own compliance or traded on the market. If suppliers fail to produce enough biofuel to generate RTFCs or fail to purchase adequate RTFCs to meet their obligation, they will instead pay a buy-out price which is 80 pence per unit for lack of development fuel and 50 pence per unit for other RTFC (UKDfT, 2023).
The California LCFS is designed to decrease the climate impact per MJ (denoted carbon intensity) of transportation energy carriers (denoted fuels but also including electricity). The LCFS was originally adopted by the California Air Resources Board (CARB) in 2009. It was re-adopted in 2015 and amended in 2018. The reduction in climate impact is upheld through a system of cap-and-trade, where energy carriers with less climate impact than the required average generate credits that can be traded and used to compensate for energy use with more climate impact. However, credits can be generated also by other means: through carbon capture and storage, and through investments in infrastructure for hydrogen refueling or fast charging of electric vehicles (CARB, 2020).
The RFS program is a national policy in the US that sets targets for the use of renewable fuels for transports (USEPA, 2023c). It was originally established in the year 2005 and expanded in 2007. The program requires that the quantity of renewable fuels increases gradually up to 36 billion gallons in 2022. Refiners or importers of gasoline or diesel fuel are obliged to reach this target by blending renewable fuels into their transport fuel, or by obtaining credits called “Renewable Identification Numbers” (RINs). The US Environmental Protection Agency (EPA) carries through life cycle calculations to investigate what fuel pathways meet the GHG requirements (USEPA, 2023d). The detailed calculation rules used by the EPA have not been published. A summary of the methodology is on the EPA website (USEPA, 2023a).
The CORSIA is an emission mitigation instrument to address the climate impact of aviation. It has been developed by the International Civil Aviation Organization (ICAO); a UN agency created by governments to support their diplomacy on international air transport matters. The CORSIA initiative aims to offsetting the amount of international aviation CO2 emissions that cannot be reduced through aircraft technologies, operational improvements, and sustainable aviation fuels.
2.3 Land use changes
The RED and the RTFO have similar rules for modelling the dLUC (EC, 2018; UKDfT, 2023). The emissions are calculated from the carbon stock in soil and vegetation. The reference value is the carbon stock in January 2008 or 20 years before the raw material, whichever was the later. The difference is divided by the biofuel production in the area during 20 years. A credit, or bonus, of 29 g CO2eq/MJ biofuel is subtracted if the biomass is obtained from restored land that was previously severely degraded and not in use for agriculture or any other activity in January 2008.
Calculations of the carbon footprint of individual fuels according to the RED and the RTFO do not account for iLUC. The EC (2018) argues that the precision in iLUC impacts is not sufficient for this purpose. Instead, the RED and the RTFO includes a cap on fuels produced from crops with a high iLUC risk (EC, 2018; EC2023c; UKDfT, 2023). The RTFO in addition gives double RTFCs to the development of fuels that cannot be produced from crops.
The LCFS, RFS and CORSIA account for iLUC and use different existing models to estimate these induced effects. The LCFS (CARB, 2020), use the term ‘land-use change’ when discussing induced or indirect LUC. In the LCFS, LUC is estimated by an updated version of the economic equilibrium model Global Trade Analysis Project (GTAP) model, which accounts for estimated elasticities of supply and demand of various goods. The climate impact of various types of land conversions is calculated with a new model: the Agro-Ecological Zone Emissions Factor (AEZ-EF). While the LCFS includes emissions from the soil, the fertilizer production and the farming processes involved in the cultivation of the fuel feedstock, it does not include induced changes in the corresponding emissions in other parts of the agricultural system (Malins, 2021). Instead, the calculated iLUC emissions includes only impacts of the indirect land conversion on the carbon storage.
In the RFS, iLUC and associated emissions from farming, fertilizer production etc., are estimated using two coupled partial equilibrium models (Malins, 2021):
• The FAPRI Modelling System (University of Missouri, 2023) is used for modelling impacts on the land use in the US.
• The Forest and Agricultural Sector Optimization Model (Adams et al., 1996) is used for modelling impacts on the land use in the rest of the world.
The carbon emissions of dLUC in the CORSIA methodology are based on the difference between the carbon content of soil and vegetation in the year 2008 and the current carbon content where the feedstock is cultivated. This is divided by the total production in the area over 25 years (ICAO, 2022a). The CORSIA framework also accounts for iLUC. ICAO (2022c) presents default values for the climate impact of iLUC, which should be added to the core life cycle emissions to calculate the total impact of the fuel (ICAO, 2022a). The default iLUC, and related emissions, are calculated using two global economic models (ICAO, 2022b):
⁃ GTAP-BIO, where GTAP stands for the Global Trade Analysis Project at Purdue University, is a computable general equilibrium model. It has been used to assess, e.g., policies on biofuels in the US (Baldos, 2017).
⁃ Global Biosphere Management Model (GLOBIOM) is a partial equilibrium model with constrained optimization (Havlik and Frank, 2023). It was developed at the International Institute for Applied Systems Analysis (IIASA) and has been used to assess, e.g., policies in the EU and land use change scenarios for the Intergovernmental Panel on Climate Change’s (IPCC’s) Shared Socioeconomic Pathways (Riahi et al., 2017).
Comparing the results from these two models led to harmonization of assumptions etc. (ICAO, 2022b), and converging results for most of the starch and sugar crops; however, the results for vegetable oils still diverge significantly. When the difference in iLUC climate impact between the models is 8.9 g CO2e/MJ or less, CORSIA applies the average of the results from the models. For crops where the difference is greater, they apply the lower iLUC value and add 8.9/2 = 4.45 g CO2e/MJ to this value (ICAO, 2022b).
If the feedstock is palm oil or corn grain, the resulting iLUC values can be high: 30–40 g CO2e/MJ. The iLUC value is zero if the feedstock is waste, residues, and by-products, if the feedstock production does not expand global agricultural land use, and/or if certain land management practices are applied (ICAO, 2022a). The iLUC values are negative, indicating a climate benefit, for several fuel feedstocks: Brassica carinata oil, camelina oil, switch grass etc. (ICAO, 2022b). The climate benefit of iLUC can be great (−40 or −50 g CO2e/MJ) when the feedstock is miscanthus or jatropha oil. When the fuel is produced from miscanthus, the beneficial iLUC can more than compensate for emissions from the fuel production and use. This means that the total climate impact of the fuel is beneficial (ICAO, 2022c). The models generate greatly beneficial iLUC value for miscanthus because the use of miscanthus for fuel production in the models increases the area used for growing miscanthus mainly by converting cropland pasture (i.e. former cropland that is pastured, but can be converted back to cropland), and this increases the carbon stock in the area (Malins, 2019).
The used iLUC models (GTAP-BIO and GLOBIOM) are both economic equilibrium models, which are based on the relation between elasticities of supply and demand of various goods.
The iLUC values used by the standards for three fuels are shown in Figure 1. The standards for which there are multiple values are due to low, medium and high values provided, as for the RED and RFS, or for different geographies, as for CORSIA. The values can be found in Table 1 in the Supplementary Material.
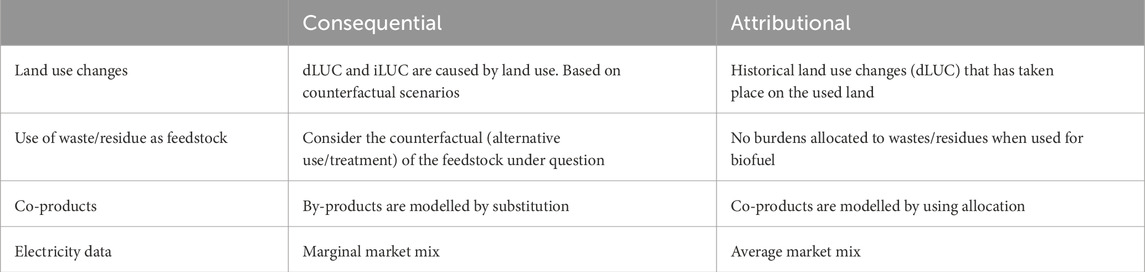
Table 1. A summary of how consequential and attributional approaches to LCA would include the elements of land use changes, waste/residue feedstock, co-products and electricity.
Land use change values vary greatly between standards as seen in Figure 1. The changes can partially be explained by choice of model, yield productivities of the land in question, and location where iLUC is assumed to take place. It is also noted that there is a greater spread of values for rapeseed and soybean biodiesel than there is for sugarcane ethanol.
2.4 Residues, wastes and co-product allocation
2.4.1 Modelling of wastes and residues used for fuel feedstock
With a few inconsistent exceptions, the standards discussed here assign zero emissions to the use of wastes/residues as biofuel feedstock and thereby do not reflect the change in GHG emissions that is induced by a change in the demand for the fuel under consideration. All wastes/residues would be used for other purposes, treated in the waste sector, or left to decay on land, if not used as biofuel feedstock. Hence, increasing the use of a waste/residue as biofuel feedstock will reduce the alternative use, treatment, or decay of this residue. Accounting for this would require the modelling of a counterfactual situation, which is a consequential approach.
In RED, RTFO, LCFS and CORSIA, fuels produced from waste, such as used cooking oil, food waste, treetops and branches, straw, and residues from processing, such as bagasse, etc. do not carry the burden of any emissions from the production and use of the product generating the waste (CARB, 2020; EC, 2018; ICAO, 2022a; UKDfT, 2023). The calculations do, however, include emissions from the collection, transport and processing of the waste and residues. This includes, for example, emissions from digestion of waste to produce biogas.
On the other hand, the RFS does not explicitly describe how the use of waste and residues as feedstock is modelled. In the case of biodiesel produced from yellow grease, the disaggregated RFS results indicate that the yellow grease enters the fuel production pathway free of burdens (USEPA, 2023b). This means that it carries no share of the impacts of the primary production of the grease and no burden associated with less grease being available for other purposes. It also is not assigned a credit for avoided waste management of yellow grease. Instead, a cut-off approach is applied that excludes any processes beyond the value chain.
In contrast, the RED Delegated Regulation (EU) 2023/1,185 on RFNBOs and RCFs considers waste and residues to be constrained inputs and requires that the calculations account for the foregone alternative fate of waste and residues used in the fuel production (EC, 2023b). In this Delegated Regulation, a key consideration of whether a co- or by-product is considered a waste is made by determining if the input is considered elastic, i.e. if it represents more than 10% of the economic value from the process where it is generated (EC, 2023b). Hence, waste and low-value by-products are considered rigid (i.e., constrained) inputs. The terms ‘elastic’ and ‘rigid’ in the Delegated Regulation are comparable with the terms ‘determining’ and ‘dependent’ co-products in consequential modelling (see Section 2.2). It is worth noting that the Delegated Regulation applies only to RFNBOs and RCFs. The definitions of rigid or elastic do not apply e.g. for common bio-oil production where there may be by- and/or co-products.
Additionally, captured fossil CO2 that is used for RFNBO or RCF production is also considered to have zero GHG emissions by both the Delegated Regulation (until 2038 or 2040) and by the UK’s RTFO. If the CO2 is waste and not generated for the purpose of RFNBO production, and if the facility that generates the fossil CO2 does not claim an emission reduction from the recycling of the CO2, it may count as 0 emission. Otherwise, each kg of CO2 carries the burden of 1 kg CO2 (UKDfT, 2024b).
2.4.2 Exceptions: animal manure and municipal solid waste
If biogas is produced from animal manure, RED and the RTFO give a credit for avoided emissions from alternative manure management (UKDfT, 2024a). The credits range from −97.6 to −108.5 gCO2eq/MJ and include a credit of −45 gCO2eq/MJ for improved agricultural management practices, for gas produced from manure used in anaerobic digestion, but varies depending on digestate storage conditions and share of manure in the feedstock. Similarly, in the LCFS, a credit for avoided emissions is given to fuel produced through anaerobic digestion of manure from milk cows and pigs (CARB, 2018). This credit appears to be large enough to make the net total impact strongly beneficial for biogas produced from manure (−150 g CO2e/MJ) and for hydrogen produced from dairy manure (−100 to −300 CO2e/MJ) (CARB, 2023). The exception for manure used for biogas production is a step towards a consequential approach. However, since it is an exception, it also makes the RTFO approach and RED (and LCFS) less consistent. It should also be noted that in RED and RTFO, this exception only applies to biogas from manure and not from other waste sources, like the organic fraction of municipal solid waste (OFMSW).
In CORSIA, if the fuel is produced from MSW diverted from landfills, the fuel can get a credit for reduced methane emissions from landfills. The size of this credit depends on the composition of the MSW, the landfill conditions, and the use of methane collected from the landfill (ICAO, 2022a). In addition, the fuel can get a credit for material recycling, if the preparation of fuel feedstock from MSW means that additional recyclable plastics (PET, HDPE, LDPE or PP), steel and/or aluminum are recovered for recycling (ICAO, 2022a). However, the recycling credits for fuel produced from MSW assumes that recovered recyclable plastics, steel and aluminum are actually recycled and substitute virgin materials of the same type. The calculations also assign all climate benefit of the recycling to the supply of the recyclable material, and no part of it to the use of recycled material. This approach is fairly well established for metals recycling. However, it is not certain that recyclable plastics will actually be recycled and substitute virgin plastics. It might also be reasonable to assign part of the climate benefit of recycling to the products produced from the recycled plastics. The credit given for substituting virgin plastics is multiplied by a factor 0.75 to account for quality losses (ICAO, 2022a). No credit is given for recycling beyond the four types of plastics, steel and aluminum.
The credits for avoided landfill and for recycling are consequential elements in the CORSIA methodology, but it only applies to fuel produced from MSW. Until additional requirements and guidance have been developed to resolve concerns regarding double counting, the credits also cannot be greater than the emissions from the production and use of the fuel. In other words, the net climate emissions from the MSW-based fuel cannot be below zero (ICAO, 2022a).
2.4.3 Energy-based co-product allocation
Both the RED and the RTFO state that emissions of co-production processes are to be partitioned (i.e., allocated) between all co-products based on their respective share of the total energy content, which is determined by their lower heating value (LHV). Emissions from the extraction and cultivation of raw materials are allocated to the fuel and co-products in proportion to their energy content (EC, 2018; UKDfT, 2023).
When more than one co-product is elastic, the Delegated Regulation prescribes allocation between these co-products; when a process yields multiple co-products where the volume can be independently varied, the Delegated Regulation states the allocation should be based on underlying physical relationships, if possible. In joint production, i.e., when the ratio produced of different co-products is fixed, allocation should be based on the energy content of the co-products (EC, 2023b).
A notable departure from a fully attributional methodology in the RED is mentioned in the Delegated regulation 2023/1,185, where it is specified that if a previously used feedstock to RFNBO or RCF production has an energy purpose, such as CO or steam, which originate from elastic production of the feedstock, then their use in RFNBO or RCF production must be included via substitution to account for the foregone energy production.
When fuels are co-produced with, for example, other fuels, chemicals, electricity, steam, hydrogen, and/or animal feed, CORSIA (ICAO, 2022b) stipulates that the climate impacts of the production shall be allocated to the products based on their energy content, and further specifies (ICAO, 2022a) that the energy content is measured as the lower heating value.
2.4.4 Other types of allocation and substitution
The LCFS makes use of substitution (CARB, 2020), and illustrates this in an example of ethanol produced from corn, where drying distiller’s grains and solubles from the biorefinery are sold as animal feed and substitutes corn and other types of feed. The use of substitution is in line with the consequential approach in LCA because it represents a cause-effect relationship. Likewise, the RED rules for RFNBOs distinguish between rigid and elastic inputs to inputs from co-production processes, and apply substitution for rigid inputs (EC, 2023b).
Similarly, the RFS (USEPA, 2023a) applies substitution to model some of the processes with multiple products. This means that the fuel gets a credit for GHG emissions avoided when co-products from the fuel production pathway displace the production of competing goods. The credit can be given for displaced feed production processes, energy supply processes, and so on. As a result of such credits, a couple of the cultivation processes have a net positive impact on the climate, when LUC impacts are excluded: the cultivation of barley for ethanol production, and the cultivation of soy for oil production. The same holds for a few of the ethanol production processes: ethanol production based on corn-stover cellulose, on sugarcane (with marginal electricity data), and on enzymatic processing of switchgrass. The net total impact on the climate can be beneficial for the full production pathway of ethanol and gasoline from corn-stover cellulose and the pathway for ethanol from enzymatic processing of switchgrass (USEPA, 2023b).
It is not clear from the RFS documents or results whether the methodology distinguishes between:
1. The use of main products that determine the volume produced of the main product and also the volume produced of by-products, and
2. The use of dependent by-products that do not affect the production process, but only the alternative fate of these by-products.
However, in other cases in the RFS, processes with multiple products are modelled through allocation, i.e., partitioning of the environmental burdens between the different products of the process. In the calculations on biodiesel from distillers’ sorghum oil via a transesterification process (USEPA, 2018), for example, the burdens of the hydrotreating process are allocated across all co-products in proportion to their lower heating values. The argument for not accounting for substituted products is that partitioning is a conservative approach. Hence, the RFS uses a mix of substitution and allocation.
2.5 Electricity data
In the RED methodology for calculating the climate impacts of biofuels, electricity bought from the grid should be modelled to reflect the average emissions from “the production and distribution of electricity in a defined region” (EC, 2018). The only exception from this rule is when the electricity is produced at a power plant that is not connected to the grid. The RED does not specify how the region should be defined, however delegated regulations related to RED include more information on this matter.
The Delegated regulation (EU) 2023/1,184 establishes a set of rules for producing RFNBOs and calculating their GHG impacts (EC, 2023a). Here, the electricity use is important because fuels of non-biological origin are produced with electricity as the main energy input. In this case, the average grid mix is calculated based on the national production mix, measured 2 years before the year for which the energy is used for RFNBO production. Several exceptions from the use of average data are given, which allow renewable electricity to count as zero GHG impact. These rules are established in two RED Delegated Regulations: Delegated regulation (EU) 2023/1,184, which defines hydrogen and hydrogen-based fuels and when they can be considered renewable and Delegated regulation (EU) 2023/1,185 which establishes the GHG methodology to calculate the full GHG lifecycle emissions of these fuels. Through these rules, the Directive establishes five situations where the GHG emissions of the electricity supplying the RFNBO producing facility can be set to zero (EC, 2023b; EC, 2024). These are:
1. Direct connection between the RFNBO and renewable electricity facility.
2. The bidding zone from which electricity is sourced has a portion of renewables other than biomass higher than 90% and the full load hours used by the RFNBO facility do not exceed the number of renewable hours in a calendar year.
3. The electricity grid has a footprint lower than 18 gCO2e/MJ and power purchase agreements (PPAs) with temporal and geographical correlation (explained below) have been concluded with renewable electricity providers for the hours used by the RFNBO facility.
4. The electricity consumed by the RFNBO facility happens during an imbalance settlement period and thus prevents downward re-dispatching of renewable electricity sources (i.e. when the system produces an excess of renewable electricity).
5. The electricity consumed by the RFNBO facility is sourced from grid electricity where PPAs for additional renewable electricity have been concluded with renewable electricity providers and temporal and geographical correlation are upheld.
Temporal correlation requirements between the production of RFNBO and renewable electricity are on an hourly basis starting from year 2030, and geographically the facilities must be either in the same or adjacent bidding zones. Renewable facilities must be no older than 3 years compared to the RFNBO facility in order to be considered additional.
The RTFO rules for calculating the actual emissions of fuels, electricity bought from the grid shall be modelled to reflect the average emissions from “the production and distribution of electricity in a defined region” (UKDfT, 2023). The RTFO does not specify how the region should be defined.
An exception from the use of regional average electricity data is given when the electricity used is fully additional renewable electricity. This holds, very much as for RED, when, for example (UKDfT, 2023):
⁃ there is a direct line between the power plant and the fuel-production plant, and it can be shown that the latter did not import electricity from the wider grid;
⁃ a power plant is explicitly built, upgraded, life-extended or brought back into service to providing electricity to a specific fuel-production site; or
⁃ the electricity used for fuel production would otherwise have been lost.
However, it’s worth noting that the RTFO has stricter rules for temporal correlation between renewable electricity assets and RFNBO producing plants, which must have a 30-min temporal correlation.
In the California LCFS framework, the electricity supply is modelled with data representing the regional or national grid average (CARB, 2018; CARB, 2023). The electricity mix in California has little climate impact, because it is dominated by natural gas (37%) and by wind, solar, and large-scale hydropower (11%–13% each (California Energy Commission, 2021)). No specific rules were found for the production of e-fuels.
In the US RFS, electricity supply is generally modelled with average grid data (USEPA, 2023a). However, the production of ethanol from sugarcanes is modelled both with average data and with data reflecting the marginal electricity supply (USEPA, 2023b).
The CORSIA framework does not prescribe in detail how the electricity supply should be modelled. However, when the calculation rules talk about the electricity supply, they mention the generation mix for grid electricity (ICAO, 2022b), or the average carbon intensity of grid electricity in the region where the electricity is used (ICAO, 2022a; ICAO, 2022b). This indicates that regional average data is at least an uncontroversial option in the CORSIA calculations. The documents do not specify how the region should be defined.
In summary, the approach for modelling electricity production varies greatly between RED documents and RED applications. While most of the standards use average data when it comes to electricity mixes there are a few exceptions when marginal data are used, notably for production of RFNBOs (RED and RTFO, sugarcane ethanol US RFS) when a few conditions are met. This is particularly important in assessments of renewable fuels produced from non-biological sources or recycled carbon, because the electricity typically dominates the energy input to such production chains.
2.6 Emissions and characterization factors
The emissions coverage and characterization factors used differ slightly between the standards. Table 2 summarizes these, along with the data sources used for the characterization factors.
None of the fuel standards account for short-lived climate pollutants like black carbon, O3 and H2, nor do they account for the cooling impacts of SOX emissions, or the (positive or negative) climate impact of changes in albedo resulting from, for example, changes in land use. Only the LCFS includes emissions other than CO2, CH4 and N2O [by including volatile organic compounds (VOC) and carbon monoxide (CO)]. Additionally, in all of the standards, biogenic CO2 is given a characterization factor of zero.
Further, none of the fuel standards considers the timing of emissions. This is relevant, for example, when forest products are used as fuels, which cause immediate emissions, while the regrowth of trees takes decades. Hereby, the use of wood as a fuel feedstock has the net effect that there will be more CO2 present in a period of time until new trees have captured the emitted CO2, which cause radiation forcing and thereby global warming (Hansen et al., 2024; Schmidt et al., 2015). Another example is when slow decaying residues are diverted as fuels, which then cause immediate emissions, while a slow decay and thereby intermediate carbon storage is lost.
Notably, however, the Delegated Regulation (EU) 2023/1,185 for RED (EC, 2023b) will allow for assigning zero climate impact to emissions of fossil CO2 that have been captured in industry until 2035 if captured from the electricity sector and until 2040 if captured in other sectors.
3 Case studies
In the following, case studies on eight fuel pathways are presented. The purpose of the case studies is to showcase how differences in modelling requirements in the standards affect the calculated carbon intensities of fuels in practice.
The five GHG standards investigated in this study contain default carbon intensity (CI) values for a selected number of fuels, feedstock types and fuel pathways. These default values are provided in a variety of ways. In certain schemes, such as the RFS, RED and RTFO, disaggregated values are provided – meaning that values are provided for different stages of the fuel supply chain (e.g. cultivation of feedstock, fuel production, transport, etc.) – while others, such as CORSIA, only provide a single value for each fuel. Disaggregated default values are useful in identifying hotspots in the fuel supply chain and investigating methodological assumptions for the calculations.
In addition to default values, the RED and RTFO provide clear calculation rules and equations to obtain a CI value for the fuels for which default values are not provided, while the other standards do not. Considering the variety of alternative fuels and the emergence of non-biomass-based alternative fuels, otherwise called RFNBOs, as alternative transportation fuels, calculation guidelines for a variety of fuels are useful.
3.1 Fuel coverage in default values
The different frameworks all consider different fuel pathways. In order to assess a variety of feedstock and fuel types, which were included in more than two standards, the following nine different fuel pathways were examined, and are summarized in Table 3.
3.2 Geographical coverage in default values
Some of the standards provide different default values for different regions. For example, RED has different values for rapeseed biodiesel produced in the EU and globally, with values of 45.5 and 50.1 kgCO2e/MJ fuel respectively. Similarly, CORSIA has different LUC values for different geographies, including USA, Europe, Brazil and globally. An example of this is that wood in the US and globally have vastly different LUC factors of −5.2 and 8.9 kgCO2e/MJ respectively (see Section 2.3 on how the standards calculate LUC). The LCFS operates differently in that the geographical location of the fuel production is stated along with the default value. Only the RFS and RTFO do not explicitly differentiate between geographical locations, but these are implied to be the USA and Europe respectively. For this study, the global values were used, when given multiple values.
3.3 Carbon intensity scores
Figure 2 shows the CI scores for the fuels assessed for each of the different standards.
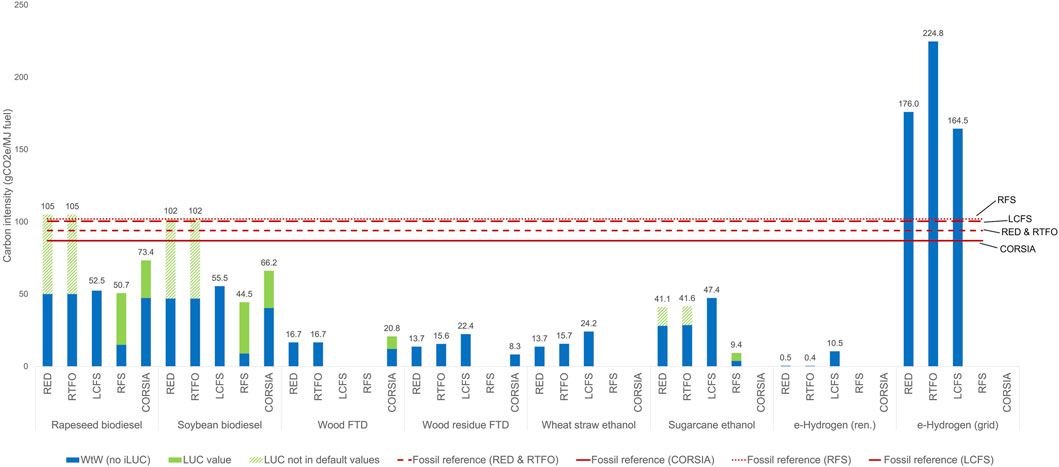
Figure 2. Carbon intensity scores for the eight fuel pathways and for the five fuel standards. The red lines indicate the fossil fuel reference values provided by each standard. FTD = Fischer-Tropsch Diesel.
As seen in Figure 2, biodiesel fuels generally perform worse than other fuels, for all of the fuel standards assessed. However, the results between the standards differ quite vastly, with a difference of 32 gCO2e/MJ fuel between CORSIA and RED for rapeseed biodiesel, and 58 gCO2e/MJ fuel difference between the RFS and RED for soybean biodiesel. These differences mean that when using RED and RTFO default values for these biodiesel fuels, the CI of these fuels is worse than the fossil fuel reference values for all standards, but only if one includes the iLUC default values, which are generally not included when biodiesel gets certified. This is mostly due to the difference in LUC values, which is high for oil crops in RED (55 gCO2e/MJ) but only 26 gCO2e/MJ in CORSIA and 36 gCO2e/MJ in the RFS (and completely omitted in LCFS). While these same fuels perform better when using other standards, it is worth noting the difference in processing emissions when comparing soybean oil in the RFS to the other standards.
Fischer-Tropsch Diesel (FTD) produced from wood and wood residues yielded lower CIs than biodiesels, even when LUC are considered, such as in CORSIA for wood FTD. The LUC value used was 8.6 gCO2e/MJ, which is the “global” value. However, the regionalized value for wood from the USA in CORSIA is given as a negative value, at −5.2 gCO2e/MJ. Using this would result in a value of 7 gCO2e/MJ for wood-based FTD, which is drastically lower than if the global value is used. None of the standards include LUC values for waste wood-based FTD, and the CI scores range between 8 and 22 gCO2e/MJ. This is likely because residues are considered burden-free in all of the standards, as discussed in Section 2.4.
Bioethanol produced from wheat straw shows generally lower CI scores than when produced from sugarcane, with scores ranging between 14 and 24 gCO2e/MJ for the former and 8–47 gCO2e/MJ for the latter. As with waste wood, LUC are not included for wheat straw, likely due to its characteristic of being a residue. However, as with waste wood, the effects of removing the residue should in some way be accounted for.
Bioethanol produced from sugarcane, which is not a residue, yields higher carbon footprint scores than for bioethanol for wheat straw, and LUC are often included. The US RFS provides many different CI scores for sugarcane ethanol, ranging between 9.5 and 58 gCO2e/MJ. This wide range is due to assumptions that can be made when calculating the score, such as the use of average or marginal electricity. The lowest value of sugarcane ethanol when calculated in the RFS (9.5 gCO2e/MJ) is vastly different to the other standards’ scores for the same fuel, which either signals an extremely efficient processing or an underestimation/exclusion of key parameters in the lifecycle (these could for example be exclusion of capital goods, an extremely good electricity mix, the exclusion of use of chemicals in processing or credits given for the production of electricity from sugarcane bagasse via substitution). It is, however, not possible to understand where the difference lies due to lack of transparency in how the values are derived.
For hydrogen, the LCFS provides default values for hydrogen produced via electrolysis with renewable and grid electricity, as 11 and 164 gCO2e/MJ respectively. For RED and RTFO, the values in Figure 2 were calculated as default values are not yet available. For hydrogen with renewable electricity, the scores were calculated to be 0.5 and 0.43 gCO2e/MJ for RED and RTFO respectively. The minor difference is due to the fact that in RED there is an emission factor for pipeline transportation, but for RTFO this is zero. It worth noting that for both RED and RTFO, if renewable electricity meets hourly and geographical correlation conditions it can be counted as zero emissions electricity. For hydrogen with grid electricity, these scores were 176 and 225 gCO2e/MJ for RED and RTFO respectively. This slightly larger difference is due to the difference in emission factors for grid electricity, which is the main contributor to the impact in this case. For RED, the European grid mix has an emission factor of 106 gCO2e/MJ (EC, 2018) but for RTFO this is stated to be 136 gCO2e/MJ (UKDfT, 2024a). In any case, regardless of the emission factor or standard used, e-hydrogen using grid electricity is always the worst scenario and is even higher than the fossil reference values.
For renewable electricity, the emission factor is zero for both RED and RTFO (EC, 2018; UKDfT, 2024a). Considering the large amount of electricity required for the production of this fuel, the emission factor associated with electricity use can have a large impact (as demonstrated for hydrogen produced via grid electricity). While the standards have additional requirements for the electricity, such as additionality, temporal and geographical correlation, the production and maintenance of renewable electricity still has an impact which should be considered.
3.4 Reduction targets based on fossil fuel baseline values
All of these schemes relate the GHG intensities to a baseline fuel, or a reference fuel, which is fossil-based. In RED and RTFO, a single baseline value is given as 94 gCO2e/MJ, and biofuels are required to meet a reduction target of 65% (i.e. have a CI of 33 gCO2e/MJ) (EC, 2018). For CORSIA, the reference fuel is conventional jet fuel, with a CI of 89 gCO2e/MJ. The reduction target for SAF is only 10%, meaning that alternative fuels need to have a CI lower than 80.1 gCO2e/MJ (ICAO, 2022a). For the US RFS, baseline values for petroleum-based gasoline and diesel are given, with values of 98.2 and 97 kgCO2e/mmBTU (or 104 and 102 gCO2e/MJ fuel) (USEPA, 2023c). Reduction requirements differ depending on feedstock and fuel type. Biodiesel and advanced biofuels from renewable feedstock except corn starch must meet a 50% reduction, whereas cellulosic biofuels must meet a 60% reduction. Bioethanol made from corn starch has a much lower reduction requirement of 20%. For the LCFS, the default value for diesel is 100 gCO2e/MJ (CARB, 2020). It is stated that the aim of the LCFS is to reduce the CI of transportation fuels by 20%, but there are no specific reduction targets for the different fuel pathways, rather the reduction target is a combined target for transport taking place in California.
Figure 3 shows the CI scores of the fuels assessed, and whether or not they comply with the reduction targets set out by each standard.
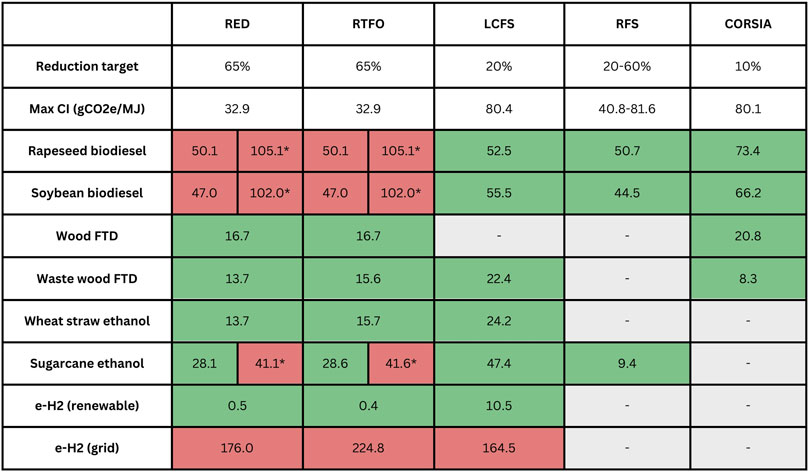
Figure 3. Heat map showing whether or not the calculated GHG scores meet each standard’s reduction targets. Green indicates that the target is met, and red indicates not. For RED and RTFO, the boxes are split to show the values without and *with iLUC.
As seen, the different standards have quite different reduction requirements, with maximum CI scores ranging from 33 gCO2e/MJ fuel according to RED and RTFO, to up to 82 gCO2e/MJ fuel according to RFS. This means that the same fuel type could be compliant with one standard but not compliant with another.
4 Discussion
Generally, the standards reviewed in this report approach the following issues differently: LUC, use of waste/residues, co-product allocation, electricity input and emission coverage and characterization differently (see Table 4). One exception is the EU RED and the UK RTFO, which are quite similar. The differences between the fuel standard methodologies are important for the calculation of results, as illustrated by Figure 2. The contradictions between the different calculation rules and results in the frameworks can cause confusion and lead to misleading decision support.
4.1 iLUC
All standards, except RED and RTFO, include iLUC, which represents a consequential approach that estimates the implications of land use changes caused by an extra demand for biofuel feedstock. The emphasis in the RED and the RTFO on dLUC calculated empirically and based on historical carbon stocks of the land from which biofuel/biomass is harvested represents a clear lack of cause-effect relationships: firstly, already cleared forests (historical high carbon stocks) cannot be saved by not sourcing feedstock from this land, and secondly the used historical approach does not account for impacts on the remaining forest, which is in fact the ultimate area of protection and the reason for avoiding LUC. Hence, the RED and the RTFO are missing an essential causal approach to link the demand for fuels with the resulting land use changes (direct or indirect).
CORSIA, the LCFS and RFS all apply different economic equilibrium models to estimate the climate impact of iLUC. This approach aims to capture a comprehensive set of causal relationships. However, the estimated climate impact can vary greatly depending on the model used, the yield of the land in question, allocation of emissions to co-products, location of the iLUC, aggregation of land types and their specific emission factors, boundary conditions and other assumptions made in the calculations. The variation is even greater when looking at scientific literature (Creutzig et al., 2015; Daioglou et al., 2020) and varies across feedstocks and methodology choices. Due to the difference in background models, the range in iLUC values reported in Figure 1 is wide. The implications of the wide range are low acceptance of iLUC values in the political landscape and low harmonization of life cycle iLUC values between different regions of the world (IMO, 2023c; IMO, 2023b).
Although the standards disagree on whether or not to use iLUC values in the calculated climate impacts of specific fuels, they all agree that iLUC impacts can be important for policy decisions. Greater harmonization of this values backed by scientific consensus could enhance uptake of iLUC values to biofuel standards and would even out the playing field.
4.2 The use of waste and residues
With regards to the use of waste/residues as a feedstock for fuel production, all standards largely follow an attributional approach, by stating that most waste and residues enter the production path with no burdens. However, they disagree on where to make exceptions from this rule by accounting for the alternative fate of the feedstock. The RED, RTFO and LCFS account for methane emissions that are avoided when manure is used to produce biogas. CORSIA instead accounts for reduced landfill emissions and increased recycling when the feedstock is MSW diverted from landfills.
The rule to disregard most counterfactual situations in the calculations risk leading to unintended consequences for the climate from fuel production, making the transition towards a carbon-efficient society less efficient and more expensive. This is notably the case for biofuels produced from constrained resources that are highly utilized, such as used cooking oil (UCO). Extra demand for UCO cannot meet the demands for energy in the transport sector, since it cannot be scaled even with deployment of optimized collection, likely leading to an increase in production of virgin vegetable oils (e.g. palm oil). Use of such feedstocks has recently been criticized by non-governmental organizations as it is prone to fraud, which has been shown in trade statistics, and will ultimately contribute to ongoing deforestation (Stratas Advisors, 2024).
The use of treetops and branches for fuel production can reduce the quantity of wood available for direct combustion to produce heat and, possibly, electricity, which then have to be substituted with the marginal electricity or heat source. For part of the treetops and branches, the alternative is instead to decompose in the forest causing temporary carbon storage, if not used for biofuel production. These impacts are also not accounted for in the standards.
The inconsistent use of counterfactual situations for some feedstock types (e.g. manure) but not others (e.g. forest residues), leads to certain fuels being incentivized over others. This is useful when there is certainty of the GHG reductions that can be accomplished by the incentive, as is the case when avoiding organic waste from being landfilled. On the other hand, it misses the point when the lack of counterfactual results in an increase in GHG emissions or induced deforestation, as can be the case by the reported fraud in the UCO market. This is a significant limitation of the standards as the alternative use of waste/residue as biofuel feedstock may have significant climate impact.
Some progress has been noted, with more consistent inclusion of counterfactual situations. Namely, the calculation rules in the Delegated Regulation on the GHG methodology for RFNBOs and RCFs, which includes cause-effect relationships of using rigid, a.k.a. constrained, vs. elastic inputs to the productions of these fuels.
4.3 Co-product allocation
Another example of the use of consequential modelling in the standards is the use of substitution to deal with by-products arising from fuel production. Only the LCFS and certain cases in the RFS stipulate or allow the use of substitution, with all other standards instead stipulating allocation. The allocation rules in RED differ between RED documents, as they depend on the multi-function of the process, and also on the purpose of the calculations. The distinction between elastic and rigid flows in the Delegated Regulation on RFNBOs and RCFs is a significant step towards a holistic methodology that will not oversee impacts.
4.4 Marginal or average electricity data
Consequential climate assessments of individual fuels ideally apply marginal electricity data. In contrast, all of the standards in our review stipulate or apply average data for electricity as the general rule. The RFS applies average and marginal data in parallel for ethanol from sugarcanes without explaining why this exception from the general rule is made.
The RED and RTFO are more explicit in stipulating when average data should be replaced by data on specific power plants or electricity technologies. The RTFO makes an exception from the use of average data when the electricity used is fully additional renewable electricity (UKDfT, 2023). This exception reflects the foreseeable consequences of using electricity. It also gives fuel producers an incentive to invest in renewable-energy production.
The RED Delegated Regulations on RFNBOs and RCFs makes several exceptions from the use of average data. The choice of electricity data is particularly important in assessments of RFNBOs and RCFs, because electricity typically dominates the energy input to the production of these fuels. Exceptions from average data are made when excess renewable electricity is produced (EC, 2023b) or when the short-term marginal electricity supply is renewable or nuclear (EC, 2023c). In such cases, the electricity is to be regarded as fully renewable (EC, 2023b) and assigned zero climate impact (EC, 2023c), respectively. This reflects the foreseeable short-term consequences of using electricity; similar results would be obtained if short-term marginal data were used in the calculations. The exception for electricity used when the short-term marginal electricity is renewable, or fossil also gives an incentive to increase the utilization of existing wind and solar power.
The RED also makes exceptions from the use of average electricity data when the electricity is produced in renewable-power plants at or directly connected to the fuel-production plants, if these power plants did not exist more than 36 months before fuel production started. In these cases, the electricity is regarded as fully renewable (EC, 2023b). This does not necessarily reflect foreseeable consequences of using the electricity, because electricity from these power plants could be used elsewhere if not in the fuel production; however, it has the benefit of giving fuel producers an incentive to make direct investments in renewable energy. Likewise, the requirements of additionality for RFNBOs and RCFs on concluding PPAs incentivizes additional renewable electricity sources which will become part of the long-term marginal electricity supply (EC, 2023b; EC, 2024).
The use of average data does not reflect the foreseeable consequences of consuming electricity. The use of national average data, in addition, overestimates the significance of national borders between countries with interconnected grids, because national electricity markets are increasingly integrated into international markets (Ekvall et al., 2023). None of the standards account for long-term marginal electricity, i.e., for foreseeable consequences of electricity use on the production capacity in the electricity supply. In the long run, such consequences are likely to be much greater and, hence, more important than short-term impacts on the utilization of existing power plants.
4.5 Emission and characterization factors
Most of the standards account for emissions of (fossil) CO2, CH4, and N2O only. The LCFS is slightly more comprehensive by accounting also for emissions of CO and VOC. This still means that none of the standards account for impacts of most short-lived climate pollutants, the cooling impacts of SOX, or changes in albedo.
An aspect of alternative transportation fuels that is emerging in literature is the concept of carbon capture and utilization (CCU) or storage (CCS) (Facchino et al., 2022; Gabrielli et al., 2020; Li, 2023; Shu et al., 2023; Von Der Assen et al., 2013). CCS may be incorporated as part of the production of alternative fuels (Shu et al., 2023), on hard to abate sectors, or as an on-board capture solution for shipping (Oh et al., 2024; Tavakoli et al., 2024). The RED and RTFO assign a credit for permanent CCS in the calculation guidelines (see the Supplementary Material). And, as mentioned in Section 2.6, the Delegated Regulation EC 2023/1,185 for RED allows fossil CO2 emissions that have been captured from industrial processes to be assigned zero climate impact (until 2035), as long as these emissions have “been taken into account upstream in an effective carbon pricing mechanism”. This is not commonly practiced in the IPCC guidelines and does not align with projections to reach global warming below 1.5 degrees Celcius, in which carbon capture and storage is promoted, but to a much lesser extent carbon capture and utilization (Gabrielli et al., 2020; IPCC, 2023). It can furthermore create confusion in sectoral GHG accounting if the sectors with overlapping CO2 emissions e.g. cement CO2 captured for shipping fuel production, do not communicate or where there is no traceability of captured CO2. Thus, ideally the nature of CO2 used in fuel production should always be stated transparently in certificates.
Moreover, the warming potential of hydrogen has not been included by any of the standards which might be an important oversight for a future transport system that relies more heavily on renewable hydrogen. The GWP100 impact of hydrogen has been estimated to around 11–13 kg CO2e/kg of hydrogen (Hauglustaine et al., 2022; Warwick et al., 2022). The values are 3 times higher if GWP20 is considered.
None of the standards consider the timing of emissions. Thereby any loss or gain of temporal carbon storage are not accounted. The omission of such effects can potentially lead to significant changes in results (Schmidt and Brandão, 2013).
4.6 General reflections
Additional standards exist beyond what we covered in this study. This means that international fuel users, as well as fuel suppliers with international markets, must relate to many diverging standards. Calculations and reporting must be made according to several different sets of rules, which makes the combined system of regulations cumbersome and expensive to adhere to. The multitude of standards on transport fuels also makes them less effective as policy tools for sifting out the best fuels. Fuels that do not qualify as renewable or low-carbon fuels in one standard can be shifted to another sector or geographical area where they do qualify. This can also contribute to an increase in global fuel transport emissions, with related increases in environmental impact.
Generally, the standards will not account for the climate cost or benefit of using waste and residues as feedstock for fuel. Hence, the system remains inconsistent. It also remains misleading for residues that cannot be used as food or feed, such as UCO (Stratas Advisors, 2024).
The standards lack transparency in the calculations of the default values. In many cases it is difficult to discern how the default values have been derived or which choices were made for the background data sources. Only RTFO and RED provide calculations equations, while the other standards do not. The differences are clear when observing the results in Figure 2. The lack of transparency makes it difficult to explain, for example, the large difference between sugarcane ethanol according to the RFS in comparison to the other standards. The difference in processing, transport and distribution of this fuel can only, presumedly, be explained by modelling choices, but these are not easily available to the standard users. Lastly, capital goods are excluded for all standards, but have been shown to be of importance for systems relying on renewable electricity like wind and solar, as well as for e-fuels considering electrolyzers’ lifetimes (Zhao et al., 2020).
While the RFS attempts to include consequential approaches more than the other standards, it is also the most inconsistent. Additionally, the lack of transparency in the RFS methodology makes it difficult to know to what extent the results obtained do reflect foreseeable consequences for the climate of the fuel -production pathways investigated. The credibility of the RFS system might increase if a uniform methodology was established, and with an increase in transparency. If this methodology includes a consistent application of marginal data and substitution that distinguishes between determining and dependent (or elastic and rigid) co-products, it will more accurately reflect the foreseeable consequences of producing and using the fuels. The latter applies to all standards reviewed herein, as they all present lack of transparency and inconsistencies which limit their effectiveness in lowering GHG emissions from transport fuel production and use. The various incentives given by the schemes result in biofuels being certified at different GHG values, even though, essentially, the fuel producing pathways are the same. For example, an organic fraction MSW-based fuel certified to the LCFS will yield a negative CI fuel, while the same pathway certified to the RED or RTFO will yield a positive CI fuel. As discussed previously, this is because, with the exception of manure-based fuels, the RED and RTFO do not include emissions credits or burdens from the counterfactual of wastes and residues i.e. avoided or induced emissions from the alternative use of these feedstock. This could inadvertently result in fuel production shifting jurisdictions in order to obtain higher GHG emission savings.
The carbon footprint values for transportation biofuels have been shown to vary widely in literature (Bouter et al., 2024; Creutzig et al., 2015; Jeswani et al., 2020; Roux et al., 2024). For example, a review of bioethanol and biodiesel LCA studies demonstrated that the carbon footprint of second generation bioethanol was reported between −115 and 173 gCO2e/MJ fuel, and between −88 and 150 gCO2e/MJ fuel for second generation biodiesel (Jeswani et al., 2020). This spread of values is due to the differences in approaches and methodological choices, but also due to stochastic (relating to the data) variabilities. Considering these, a range of results is expected as opposed to a point value. However, the five standards only provide point values as default values, and the calculation guidelines in the RED and RTFO do not provide room for incorporating ranges of input data values. The values provided by the standards fit within the ranges found in literature, but it is relevant to note that, depending on the technological parameters and process configurations, and related stochastic variability, the carbon footprint of a fuel will not yield a single value.
5 Recommendations
As it has been widely recognized that land use changes have potential large effects on the potential climate impact of biofuels, it is recommended that it should always be included. The chosen iLUC model should – as far as possible – establish a cause-effect relationship between changes in demand for biofuels and the induced land use changes. Further, the used iLUC model should include all relevant mechanisms for capturing relevant physical relationships that influence the resulting land use changes, such as crop yields, and substitutability to identify the relevant induced indirect effects, e.g. that a change in demand for land can be met both by expanding cultivated area and by intensifying existing cropland, and that transformation of 1 ha land in one country does not yield the same potential crop yields as in other countries, as well as not all land is suitable for all purposes.
The risk for unintended negative climate impacts of fuel use is reduced if counterfactual situations are consistently applied when modelling the use of waste and residues as feedstock for transportation fuels. Given the scale of the energy required for transport, which is in the order of 149 EJ in 2020, it is important to include cause-effect relationships that span outside of fuel supply chains, such as land use change, and displacement effects from fully utilized constrained feedstock. A consistent use of counterfactuals reduces the risk for negative climate impacts from the use of input that is co-produced with other products. Here it is useful to distinguish between determining and dependent (or between elastic and rigid) co-products or flows.
To ensure a comprehensive climate impact assessment within the standards, they should account for, in addition to long-term impacts, short-lived climate forcers and albedo effects, as well as temporal effects of emissions. In addition, for these assessments to be robust, they should only account for emissions for which reliable data can be found. It is also recommended that as new characterization factors are developed by the IPCC, and new IPCC reports are published, these updated values should be adopted by the standards.
The provision of clear calculation guidelines, as with the RED and RTFO, allows for the inclusion of many types of fuels, and increases the transparency regarding the methodological choices made in determining a fuel’s CI score. It is recommended that this practice is adopted for all the fuel standards, with the inclusion of suggested emission factors for parameters such as transportation, land use changes, inputs relating to cultivation and fuel processing, etc. Additionally, when default values are provided, documentation of the data and assumptions used in generating these values should be provided by the fuel standards.
6 Conclusion
In this study, five standards for GHG accounting of alternative (non-fossil) transport fuels were reviewed, using case studies to illustrate their application. We found significant inconsistencies in the calculation rules among these standards, particularly regarding co-product allocation, the treatment of waste and residues and inclusion of land use changes. Some standards, such as the RFS, LCFS, and CORSIA, lack explicit calculation rules and equations, which hinders their harmonized adoption. Conversely, the RTFO and RED offer clear calculation rules and default values, promoting uniform GHG calculations.
Each of the standards includes a mix of consequential and attributional elements, but the balance differs between the standards. To calculate environmental impact scores that reflect foreseeable consequences for the climate, counterfactual scenarios can be consistently applied when considering co-products, the use of waste and residues, and land use changes. This approach would lead to a more comprehensive assessment of the GHG impacts of transport fuels and help avoid shifting environmental burdens to other sectors.
Given the substantial energy demands of the transport sector, it is essential to account for land use changes, as deforestation can negate the environmental benefits of biofuels. Internationally recognized values for iLUC are needed to ensure that these impacts are globally acknowledged and to prevent further deforestation. The used iLUC model should include all relevant mechanisms for capturing relevant physical relationships that influence the resulting land use changes. We found that, as a result of the differences in the methodological choices outlined in this study, the same fuel produced from the same feedstock can yield different CI scores, depending on which fuel standard is used. Additionally, the GHG reduction targets differ widely between the standards, from a 10% reduction prescribed by CORSIA, to a 65% reduction according to the RED and RTFO. The GHG reduction targets of most standards, except for RED and RTFO, are insufficient to drive significant reductions. These targets permit the indefinite use of crop-based biofuels, with associated biodiversity and GHG emissions impacts. It is crucial to reduce the GHG impacts of alternative transport fuels to below 80% (18.8 g CO2e/MJ) and to completely avoid any increase in biodiversity impacts to ensure meaningful progress. Furthermore, achieving global warming targets below 1.5°C will require substantial emissions reductions from transport activities and additional CO2 removal efforts to meet global climate goals.
The variation in standards can lead to distorted production and trade patterns, as certain fuels and feedstocks may be directed towards regions offering financial incentives or higher GHG reductions due to differences in calculation rules. Further harmonization of standards is necessary to prevent such distortions. We recommend the development and adoption of a common, scientifically grounded standard. This standard should be crafted by a neutral organization, such as the ISO, to prevent bias towards particular feedstocks and encourage consensus on contentious issues like land use changes. Consistency with the general carbon-footprint standard provided by the ISO (International Organization for Standardization, 2018) should be ensured. Transparency between sectors is crucial to prevent double counting of emissions reductions that are reported in national inventories (since this is where production of the fuels occurs), while consumed elsewhere (like international aviation and shipping).
Author contributions
MR: Visualization, Writing–original draft, Writing–review and editing. TE: Conceptualization, Writing–original draft, Writing–review and editing. JS: Conceptualization, Writing–original draft, Writing–review and editing. GCV: Conceptualization, Writing–original draft, Writing–review and editing.
Funding
The author(s) declare that no financial support was received for the research, authorship, and/or publication of this article.
Conflict of interest
GCV was employed by A.P. Møller-Mærsk.
The remaining authors declare that the research was conducted in the absence of any commercial or financial relationships that could be construed as a potential conflict of interest.
Publisher’s note
All claims expressed in this article are solely those of the authors and do not necessarily represent those of their affiliated organizations, or those of the publisher, the editors and the reviewers. Any product that may be evaluated in this article, or claim that may be made by its manufacturer, is not guaranteed or endorsed by the publisher.
Supplementary material
The Supplementary Material for this article can be found online at: https://www.frontiersin.org/articles/10.3389/fenrg.2024.1490137/full#supplementary-material
Abbreviations
CARB, California Air Resources Board; CI, Carbon intensity; EC, European Commission; GHG, Greenhouse gas; ICAO, International Civil Aviation Organization; IMO, International Maritime Organization; ISO, Internation Organization of Standardization; IPCC, Intergovernmental Panel on Climate Change; LCA, Life cycle assessment; LCFS, Low Carbon Fuel Standard; LUC, Land use changes; MSW, Municipal solid waste; RCF, Recycled carbon fuel; RED, Renewable Energy Directive; RFNBO, Renewable fuels of non-biologic origin; RFS, Renewable Fuel Standard; RTFC, Renewable Transport Fuel Certificate; RTFO, Renewable Transport Fuel Obligation; UKDfT, United Kingdom Department for Transport; USEPA, United States Environmental Protection Agency.
References
Adams, D. M., Alig, R. J., Callaway, J. M., Mccarl, B. A., and Winnett, S. M. (1996). The forest and agricultural sector optimization model (FASOM): model structure and policy applications.
Baldos, U. L. C. (2017). Development of GTAP version 9: land use and land cover database for years 2004, 2007 and 2011.
Barnabe, D., Bucchi, R., Rispoli, A., Chiavetta, C., Porta, P. L., Bianchi, C. L., et al. (2013). “Land use change impacts of biofuels: a methodology to evaluate biofuel sustainability,” in Biofuels - economy, environment and sustainability (London, United Kingdom: InTech). doi:10.5772/52255
Bouter, A., Duval-Dachary, S., and Besseau, R. (2024). Life cycle assessment of liquid biofuels: what does the scientific literature tell us? A statistical environmental review on climate change. Biomass Bioenergy 190, 107418. doi:10.1016/j.biombioe.2024.107418
Brandão, M., Azzi, E., Novaes, R. M. L., and Cowie, A. (2021). The modelling approach determines the carbon footprint of biofuels: the role of LCA in informing decision makers in government and industry. Clean. Environ. Syst. 2, 100027. doi:10.1016/j.cesys.2021.100027
Brandão, M., Ekvall, T., Poulikidou, S., Johansson, K., Nilsson, J., Nojpanya, P., et al. (2022). RED, PEF, and EPD: conflicting rules for determining the carbon footprint of biofuels give unclear signals to fuel producers and customers. Front. Clim. 4. doi:10.3389/fclim.2022.988769
California Energy Commission (2021). 2020 total system electric generation. Available at: https://www.energy.ca.gov/data-reports/energy-almanac/california-electricity-data/2021-total-system-electric-generation/2020.
CARB (2018). Tier 1 simplified CI calculator instruction manual biomethane from anaerobic digestion of dairy and swine manure. Available at: https://www.arb.ca.gov/regact/2014/capandtrade14/ctlivestockprotocol.pdf.
CARB (2020). LCFS Basics with notes. Available at: https://ww2.arb.ca.gov/sites/default/files/2020-09/basics-notes.pdf.
CARB (2023). Current fuel pathways. Available at: https://ww2.arb.ca.gov/resources/documents/lcfs-pathway-certified-carbon-intensities.
Creutzig, F., Ravindranath, N. H., Berndes, G., Bolwig, S., Bright, R., Cherubini, F., et al. (2015). Bioenergy and climate change mitigation: an assessment. GCB Bioenergy 7 (5), 916–944. doi:10.1111/gcbb.12205
Daioglou, V., Woltjer, G., Strengers, B., Elbersen, B., Barberena Ibañez, G., Sánchez Gonzalez, D., et al. (2020). Progress and barriers in understanding and preventing indirect land-use change. Biofuels, Bioprod. Biorefining 14 (5), 924–934. doi:10.1002/bbb.2124
EC. (2018). DIrective (eu) 2018/2001 of the european parliament and of the council of 11 December 2018 on the promotion of the use of energy from renewable sources.
EC. (2023c). Directive (EU) 2023/2413 of the European parliament and of the council of 18 october 2023.
EC (2024). Q&A implementation of hydrogen delegated acts. Available at: https://energy.ec.europa.eu/document/download/21fb4725-7b32-4264-9f36-96cd54cff148_en?filename=2024%2003%2014%20Document%20on%20Certification.pdf.
Ekvall, T., Lorentzon, K., Lindvall, E. E., and Palander, S. (2023). Modelling electricity in environmental footprints.
Facchino, M., Popielak, P., Panowski, M., Wawrzyńczak, D., Majchrzak-Kucęba, I., and De Falco, M. (2022). The environmental impacts of carbon capture utilization and storage on the electricity sector: a life cycle assessment comparison between Italy and Poland. Energies 15 (18), 6809. doi:10.3390/en15186809
Gabrielli, P., Gazzani, M., and Mazzotti, M. (2020). The role of carbon capture and utilization, carbon capture and storage, and biomass to enable a net-zero-CO2 emissions chemical industry. Industrial Eng. Chem. Res. 59 (15), 7033–7045. doi:10.1021/acs.iecr.9b06579
Hansen, R. N., Eliassen, J. L., Schmidt, J., Andersen, C. E., Weidema, B. P., Birgisdóttir, H., et al. (2024). Environmental consequences of shifting to timber construction: the case of Denmark. Sustain. Prod. Consum. 46, 54–67. doi:10.1016/j.spc.2024.02.014
Hauglustaine, D., Paulot, F., Collins, W., Derwent, R., Sand, M., and Boucher, O. (2022). Climate benefit of a future hydrogen economy. Commun. Earth Environ. 3 (1), 295. doi:10.1038/s43247-022-00626-z
Havlik, P., and Frank, S. (2023). Global Biosphere management model (GLOBIOM). Available at: https://iiasa.ac.at/models-tools-data/globiom.
ICAO (2022a). CORSIA methodology for calculating actual life cycle emissions values. Available at: https://www.icao.int/environmental-protection/CORSIA/Documents/CORSIA_Eligible_Fuels/ICAO%20document%2007%20-%20Methodology%20for%20Actual%20Life%20Cycle%20Emissions%20-%20June%202022.pdf.
ICAO (2022b). Corsia supporting document corsia eligible fuels-life cycle assessment methodology. Available at: https://www.icao.int/environmental-protection/CORSIA/Documents/CORSIA_Eligible_Fuels/CORSIA_Supporting_Document_CORSIA%20Eligible%20Fuels_LCA_Methodology_V5.pdf.
ICAO (2022c). Default life cycle emissions. Available at: https://www.icao.int/environmental-protection/CORSIA/Documents/CORSIA_Eligible_Fuels/ICAO%20document%2006%20-%20Default%20Life%20Cycle%20Emissions%20-%20June%202022.pdf.
IEA (2023). Tracking clean energy progress 2023. Available at: https://www.iea.org/reports/tracking-clean-energy-progress-2023.
IMO (2023b). Expert views on sustainability aspects in the imo lca framework, including possible approaches to operationalize a risk-based approach for indirect land use change (ILUC). Available at: https://www.wto.org/english/tratop_e/dispu_e/cases_e/ds593_e.htm.
IMO. (2023c). Review of existing practices on sustainability aspects/certification and third-party verification issues.
International Organization for Standardization (ISO). (2006a). EN ISO14040 Environmental management—life cycle assessment— principles and framework.
International Organization for Standardization (ISO). (2006b). EN ISO14044 Environmental management—life cycle assessment— requirements and guidelines.
International Organization for Standardization (ISO). (2018). EN ISO 14067 Greenhouse gases - carbon footprint of products - requirements and guidelines for quantification.
IPCC (2008). Climate change 2007: synthesis report. Geneva, Switzerland: Intergovernmental Panel on Climate Change.
IPCC. (2015). Climate change 2014: synthesis report. Geneva, Switzerland: Intergovernmental Panel on Climate Change.
IPCC. (2023). Climate change 2023: synthesis report. Geneva, Switzerland: Intergovernmental Panel on Climate Change. doi:10.59327/IPCC/AR6-9789291691647
Jeswani, H. K., Chilvers, A., and Azapagic, A. (2020). Environmental sustainability of biofuels: a review. Proc. R. Soc. A Math. Phys. Eng. Sci. 476 (Issue 2243), 20200351. doi:10.1098/rspa.2020.0351
Konradsen, F., Hansen, K. S. H., Ghose, A., and Pizzol, M. (2024). Same product, different score: how methodological differences affect EPD results. Int. J. Life Cycle Assess. 29 (2), 291–307. doi:10.1007/s11367-023-02246-x
Li, H. (2023). Advancing “carbon peak” and “carbon neutrality” in China: a comprehensive review of current global research on carbon capture, utilization, and storage technology and its implications. ACS Omega 8 (45), 42086–42101. doi:10.1021/acsomega.3c06422
Malins, C. (2019). Understanding the indirect land use change analysis for CORSIA. Available at: www.cerulogy.com.
Malins, C. (2021). Considerations for addressing indirect land use change in Danish biofuel regulation.
Muñoz, I., Schmidt, J., de Saxcé, M., Dalgaard, R., and Merciai, S. (2015). Inventory of country specific electricity in LCA - consequential scenarios. Available at: https://lca-net.com/files/Final-Energy-Methodology-Report_v3.0_20150827.pdf.
Muñoz, I., and Weidema, B. P. (2023). Example – marginal electricity in Denmark. Available at: https://consequential-lca.org/clca/marginal-suppliers/the-special-case-of-electricity/example-marginal-electricity-in-denmark/.
Oh, J., Kim, D., Roussanaly, S., and Lim, Y. (2024). Greenhouse gas emissions of shipping with onboard carbon capture under the FuelEU Maritime regulation: a well-to-wake evaluation of different propulsion scenarios. Chem. Eng. J. 498, 155407. doi:10.1016/j.cej.2024.155407
Plevin, R. J., Delucchi, M. A., and Creutzig, F. (2014). Using attributional life cycle assessment to estimate climate-change mitigation benefits misleads policy makers. J. Industrial Ecol. 18 (1), 73–83. doi:10.1111/jiec.12074
Repo, A., Böttcher, H., Kindermann, G., and Liski, J. (2015). Sustainability of forest bioenergy in Europe: land-use-related carbon dioxide emissions of forest harvest residues. GCB Bioenergy 7 (4), 877–887. doi:10.1111/gcbb.12179
Riahi, K., van Vuuren, D. P., Kriegler, E., Edmonds, J., O’Neill, B. C., Fujimori, S., et al. (2017). The Shared Socioeconomic Pathways and their energy, land use, and greenhouse gas emissions implications: an overview. Glob. Environ. Change 42, 153–168. doi:10.1016/j.gloenvcha.2016.05.009
Roux, M., Lodato, C., Laurent, A., and Astrup, T. F. (2024). A review of life cycle assessment studies of maritime fuels: critical insights, gaps, and recommendations. Sustain. Prod. Consum. 50, 69–86. doi:10.1016/j.spc.2024.07.016
Schmidt, J. H. (2015). Life cycle assessment of five vegetable oils. J. Clean. Prod. 87 (C), 130–138. doi:10.1016/j.jclepro.2014.10.011
Schmidt, J. H., and Brandão, M. (2013). LCA screening of biofuels - iLUC, biomass manipulation and soil carbon.
Schmidt, J. H., Weidema, B. P., and Brandão, M. (2015). A framework for modelling indirect land use changes in Life Cycle Assessment. J. Clean. Prod. 99, 230–238. doi:10.1016/j.jclepro.2015.03.013
Searchinger, T. D., James, O., Dumas, P., Kastner, T., and Wirsenius, S. (2022). EU climate plan sacrifices carbon storage and biodiversity for bioenergy. Nature 612, 27–30. doi:10.1038/d41586-022-04133-1
Shu, D. Y., Deutz, S., Winter, B. A., Baumgärtner, N., Leenders, L., and Bardow, A. (2023). The role of carbon capture and storage to achieve net-zero energy systems: trade-offs between economics and the environment. Renew. Sustain. Energy Rev. 178, 113246. doi:10.1016/j.rser.2023.113246
Sonnemann, G., and Vigon, B. (2011). Global guidance principles for life cycle assessment databases.
Tavakoli, S., Gamlem, G. M., Kim, D., Roussanaly, S., Anantharaman, R., Yum, K. K., et al. (2024). Exploring the technical feasibility of carbon capture onboard ships. J. Clean. Prod. 452, 142032. doi:10.1016/j.jclepro.2024.142032
Tjandra, S., Kraus, S., Ishmam, S., Grube, T., Linßen, J., May, J., et al. (2024). Model-based analysis of future global transport demand. Transp. Res. Interdiscip. Perspect. 23, 101016. doi:10.1016/j.trip.2024.101016
UKDfT (2023). Renewable transport fuel obligation: compliance guidance. Available at: www.nationalarchives.gov.uk/doc/opengovernment-licence/version/3/.
UKDfT (2024a). Renewable transport fuel obligation: compliance guidance. Available at: www.nationalarchives.gov.uk/doc/opengovernment-licence/version/3/.
UKDfT (2024b). RTFO guidance for renewable fuels of non-biological origin. Available at: www.gov.uk/government/organisations/.
UNEP/SETAC Life Cycle Initiative. (2011). Global guidance principles for life cycle assessment databases: a basis for greener processes and products.
University of Missouri (2023). FAPRI: food and agricultural policy research Institute at the university of Missouri. Available at: https://fapri.missouri.edu/.
USEPA (2010). Regulation of fuels and fuel additives: changes to renewable fuel standard program. Available at: https://www.regulations.gov/document/EPA-HQ-OAR-2005-0161-3187.
USEPA (2023a). Lifecycle analysis of greenhouse gas emissions under the renewable fuel standard. Available at: https://www.epa.gov/renewable-fuel-standard-program/lifecycle-analysis-greenhouse-gas-emissions-under-renewable-fuel.
USEPA (2023b). Lifecycle greenhouse gas results. Available at: https://www.epa.gov/fuels-registration-reporting-and-compliance-help/lifecycle-greenhouse-gas-results.
USEPA (2023c). Overview of the renewable fuel standard program. Available at: https://www.epa.gov/renewable-fuel-standard-program/overview-renewable-fuel-standard-program.
USEPA (2023d). Renewable fuel petition review process. Available at: https://www.epa.gov/renewable-fuel-standard-program/renewable-fuel-petition-review-process.
Valin, H., Peters, D., van den Berg, M., Frank, S., Havlik, P., Forsell, N., et al. (2015). The land use change impact of biofuels consumed in the EU. Available at: https://energy.ec.europa.eu/system/files/2016-03/Final%2520Report_GLOBIOM_publication_0.pdf.
Von Der Assen, N., Jung, J., and Bardow, A. (2013). Life-cycle assessment of carbon dioxide capture and utilization: avoiding the pitfalls. Energy Environ. Sci. 6 (9), 2721–2734. doi:10.1039/c3ee41151f
Warwick, N., Griffiths, P., Keeble, J., Archibald, A., and Pyle, J. (2022). Atmospheric implications of increased Hydrogen use.
Weidema, B. P., Ekvall, T., and Heijungs, R. (2009). Guidelines for application of deepened and broadened LCA.
Weidema, B. P., Pizzol, M., Schmidt, J., and Thoma, G. (2018). Attributional or consequential Life Cycle Assessment: a matter of social responsibility. J. Clean. Prod. 174, 305–314. doi:10.1016/j.jclepro.2017.10.340
Keywords: transportation fuels, renewable fuel standards, environmental impacts, fuel certification, regulatory frameworks, carbon footprint
Citation: Roux M, Ekvall T, Schmidt J and Croxatto Vega G (2024) Which rules to follow? how differences in renewable fuel standards obscure the potential climate impact of transportation fuels. Front. Energy Res. 12:1490137. doi: 10.3389/fenrg.2024.1490137
Received: 02 September 2024; Accepted: 28 October 2024;
Published: 07 November 2024.
Edited by:
Eddie Blanco-Davis, Liverpool John Moores University, United KingdomReviewed by:
Fan Yang, Southwest Petroleum University, ChinaHu Li, Sichuan University of Science and Engineering, China
Copyright © 2024 Roux, Ekvall, Schmidt and Croxatto Vega. This is an open-access article distributed under the terms of the Creative Commons Attribution License (CC BY). The use, distribution or reproduction in other forums is permitted, provided the original author(s) and the copyright owner(s) are credited and that the original publication in this journal is cited, in accordance with accepted academic practice. No use, distribution or reproduction is permitted which does not comply with these terms.
*Correspondence: Megan Roux, merou@dtu.dk
†These authors have contributed equally to this work