- 1Department of Control and Instrumentation Engineering, King Fahd University of Petroleum and Minerals (KFUPM), Dhahran, Saudi Arabia
- 2Interdisciplinary Research Center for Sustainable Energy Systems (IRC-SES), KFUPM, Dhahran, Saudi Arabia
- 3Institute of Electrical, Electronics and Computer Engineering, University of the Punjab, Lahore, Pakistan
- 4Department of Industrial Engineering, King Khalid University, Abha, Saudi Arabia
- 5Electrical Engineering Department, KFUPM, Dhahran, Saudi Arabia
Effective energy management in grid-connected renewable energy systems is essential for achieving cost-efficiency and reliability. This work presents a versatile control technique to tackle power system difficulties in grid-connected and grid-failure modes. The concept employs a digital iterative approach to optimize the operation of a DC-DC converter, guaranteeing the attainment of maximum power output and stability. During a grid breakdown, the system injects carefully adjusted active power into the grid to ensure that voltage and current levels are maintained at appropriate levels, hence preventing overloading. The proposed strategy exhibits a 99% enhancement in system responsiveness and a 96% decrease in overshoot when compared to conventional methods, thus substantiating its efficacy in practical scenarios.
1 Introduction
Traditional methods use non-renewable resources such as coal to make electricity in power plants and cause significant pollution. Due to population and industry growth, electricity demand is rising significantly. A typical power plant cannot meet customer demand alone due to environmental concerns. Changing tactics to meet consumer demand is vital now. Most affluent nations focus on generating alternative resources and researching ways to maximize power. Solar, fuel cell, wind, and hydro/water energy sources are mostly eco-friendly. Only resource availability affects renewable energy power generation. Renewable energy sources vary daily due to natural resource fluctuations. The main renewable energy sources are photovoltaic (PV) and fuel cells (Nureddin et al., 2020). Photovoltaics, fuel cells, and energy storage. The Cuckoo search method uses three DC-DC boost converters for solar and fuel cell Maximum Power Point Tracking (MPPT). These converters boost source efficiency and output voltage with MPPT on a shared DC bus. A DC/AC inverter connects the hybrid power system to the proposed community’s AC loads. PSO-optimized PI controllers control converters (abed El-Raouf et al., 2018). Heat, hydrogen/oxygen partial pressure, and water membrane content affect fuel cell power (Ahmadi et al., 2017).
A fuzzy set theory-based maximum power point tracker can significantly boost energy conversion. Fuzzy algorithms optimize boost converter power point tracking by using linguistic rules in operator control techniques. Careful selection of fuzzy logic controller variables is crucial for effective DC-DC converter modulation (Garg et al., 2015). The research in (Padmanaban et al., 2019) discusses MPPT-generated PV and fuel cells, highlighting how MPPT optimizes system power. Most off-grid solar systems use MPPT to maximize battery charging making solar energy affordable and accessible. Although solar panels generate a lot of energy, they convert only a small amount of sunlight into power. Rotating traditional solar panels perpendicular to the sun maximizes efficiency, but this requires continuous adjustments as the sun moves throughout the day (Patel et al., 2016). Manual rotation is laborious and expensive, but solar tracking systems address this by automatically rotating PV panels toward the sun using motors. However, the electricity used by these motors can reduce overall system efficiency (Mousazadeh et al., 2009).
This study introduces a comprehensive method for determining the ideal size and energy management strategy for fuel-cell hybrid electric vehicles (FC-HEVs). The technique demonstrates substantial reductions in fuel usage and enhanced vehicle performance by utilizing a combination of different energy sources (Abdeldjalil et al., 2023). Additionally, the study assesses various optimization algorithms for a grid-connected hybrid energy system combining photovoltaic and wind turbine components with battery and supercapacitor storage. It emphasizes the exceptional performance of the CGO algorithm in achieving the most efficient system configurations and cost-effectiveness (Guven et al., 2024).
Furthermore, the study employs four Multi-Objective Optimization techniques to optimize a system that integrates Photovoltaic, Wind, Battery, and Electric Vehicle Charging Stations. The findings indicate that NSGA-II achieves the best results in minimizing total costs and ensuring a reliable supply. The study also confirms the economic feasibility and resilience of the system through thorough technical and sensitivity analyses (Barakat et al., 2024).
This study explores the integration of a Proton Exchange Membrane Fuel Stack (PEMFS) with a Wide Input Operation Single Switch Boost Converter and a Grey Wolf Algorithm Dependent Fuzzy Logic Methodology to optimize current supply and maintain operation near Maximum Power Point (Prashanth et al., 2024). This study examines metaheuristic optimization-based MPPT methods for Proton Exchange Membrane Fuel Stacks in electric vehicles, highlighting the effectiveness of the Continuous Different Slope Value-based Cuckoo Search Method (in improving power output and efficiency (Touti et al., 2024).
The system discussed in this paper is more reliable because fuel cells are efficient and durable. They are clean and emit less pollutants, making them ideal for the system. During peak demand and periods of system stress, fuel cells will generate power, ensuring that the system meets client needs even during high usage. Advances in energy technology have made power more widely available. Despite its many benefits, the system has downsides that must be addressed to ensure safety and energy reliability. An intelligent control method regulates grid connections both during faults and normal operation, maintaining system stability.
The rest of the paper is organized as follows: Section 2 outlines the problem statement addressed in this study. Section 3 details the system modeling, providing a concise overview of the key system components. Section 4 describes the methodology, including the system development and the process for obtaining results. Section 5 presents the results and offers a comprehensive discussion, while Section 6 concludes the paper.
2 Problem statement
Traditional energy management systems for renewable energy sources often struggle to efficiently handle the dual challenges of maximizing power output during grid-connected operations and maintaining stability during grid failures. Current approaches lack the adaptability needed to optimize power flow in dynamic environments, leading to inefficiencies and potential overloading during critical grid failure scenarios.
This research addresses these challenges by introducing a novel control strategy that operates seamlessly in both grid-connected and grid-failure modes. The proposed system employs an online iterative technique based on the Perturb and Observe (P&O) method to optimize the operation of a DC-DC converter. The control strategy ensures that power output is maximized during grid-connected conditions, while in grid-failure mode, it maintains the desired voltage and current levels for loads, preventing overloading and enhancing system reliability.
This work aims to demonstrate that the PV-integrated fuel cell system can effectively inject optimized active power into the grid during failures, ensuring continuous power supply and stability. The study’s results validate the efficacy of the proposed approach, highlighting significant improvements in system responsiveness and a substantial reduction in overshoot, compared to conventional methods.
3 System modeling
This section provides a brief description of the primary components of the modeling system. The PV-based fuel cell system is connected to the grid using a three-phase, three-level Voltage Source Converter (VSC) and a boost converter. A Simulink model employing the Perturb and Observe (P&O) technique is used to perform MPPT in the boost converter. The system’s inverter converts the DC-link voltage to AC voltage. Additionally, an isolation and voltage step-up transformer is incorporated, and inductive filters are used to eliminate switching harmonics at the output. The common coupling point is connected to adjustable resistive local loads. The control technique for the boost converter aims to achieve peak efficiency by utilizing voltages and currents from the PV-based fuel cell system, along with grid and system voltages and load currents. Figure 1 provides a detailed description of the system.
In the following sub-sections, the primary components of the modeling system are briefly described. These components include the fuel cell and photo-voltaic cell energy generation unit, the DC-DC boost converter, and the three-phase inverter.
3.1 Fuel cell
In hydrogen-based fuel cells, the reaction of hydrogen and oxygen produces electricity. Equation 1 represents the fuel cell’s electrochemical reaction by using the Ernest Equation application (Li et al., 2015).
Here,
3.2 Photo-voltaic cell
The use of PV systems has been increasing rapidly due to their many advantages. A typical module provides DC output, though modern systems include an inverter unit that converts DC to AC power for Maximum Power Point (MPP) operation and performs inversion tasks when needed, making them more efficient. A perfect PV cell can be modeled with a current source and a parallel-connected diode (Salman et al., 2018). The characteristic Equations 2 and 3 for the current and voltage of a solar cell is given as follows (Gergaud et al., 2002; Esram and Chapman, 2007):
The thermal voltage is denoted by
3.3 Boost converter
The fundamental components of a boost converter include the input voltage, an inductor (
Upon solving the provided Equation 4, the DC transfer function that connects the input and output voltages is stated as Equation 5:
3.4 Inverter
The primary purpose of an inverter is to convert DC energy into AC electrical energy (Inci, 2019). Additionally, it manages the transfer of electricity (Patra et al., 2015). To minimize switching ripples in the electrical power flow of the PV-based fuel cell system, inductive filters are employed at the output. Ignoring the losses, the Equation 6 represents the power of the system (Al-Shetwi et al., 2018; Hajizadeh et al., 2010)
To determine the value of the DC-link voltage, the DC power must be zero. Additionally, fluctuations in the system’s supplied power impact the rate at which the DC-link voltage responds. According to this condition, the DC-link voltage (Hajizadeh et al., 2010) can be expressed as Equation 8:
3.5 Power flow control
In addition to managing the DC-link voltage, it also controls the active power flow. A Phase-Locked Loop (PLL) uses the system voltage to provide information about the phase and synchronizes the voltage output with the grid voltage. Using the inner DC-link voltage and current of the system, the reference value (
4 Methodology
This section discusses the methodology of the system. The aim of the proposed control approach is to offer a contrast to the traditional method used in grid-connected PV-based fuel cell systems that operate in grid-failure mode.
4.1 Comparison
Traditional P&O MPPT maximizes PV-based fuel cell power extraction and feeds the grid via interface components. This method iteratively finds the operating point at maximal condition. Following the operational framework of traditional P&O MPPT, the approach compares instantaneous power and voltage to their preceding values to find the maximum power point. The biggest problem of this method is that it encourages excessive power. For instance, boost converters employ P&O MPPT to maximize system power and provide it to loads and grids. A system connected to the grid sends additional electricity into the grid as the load needs the necessary quantity of electricity. This phenomenon has positive outcomes (Becherif and Hissel, 2010). In case of Gird PV-based fuel cell system failure, electricity will be sent to the load. This can overload and lower load current and voltage (Ahmed and Salam, 2016).
4.2 Operational principle
The primary objective of the flexible control algorithm is to extract the maximum power output from a PV-based fuel cell system. The updated algorithm operates on the principle of detecting faults in the grid bus and is based on the P&O MPPT concept. In the initial stage, the grid-side voltage is monitored, and the controller determines the appropriate operating mode (Shitole et al., 2017). Equation 11 is utilized for the conversion of the
Grid voltage’s d-components and q-components are produced as orthogonal signals during the conversion process (Golestan and Guerrero, 2014). Furthermore, it is clear that the magnitude signal is produced by adding the squares of the d and q components (Hwang et al., 2012). According to the fault situation, the magnitude will decrease when a fault develops on the grid side. In this regard, the voltage magnitude is subtracted from the reference value to determine the depth of voltage reduction (Camacho et al., 2017).
The flexible control strategy’s flowchart can be found in Figure 2. The fault signal must equal zero in order to get the maximum power extraction. The grid connection mode is engaged when the fault signal (F) is equal to zero. The duty cycle of the switching process is ascertained by applying the P&O technique’s operating rules. In this mode, the polarity of the most recent perturbation and the polarity of the power shift decide which way the next apprehension will go (Bianconi et al., 2013). If the power difference between the current sample and the prior sample is positive,
where
In the event of a grid-side interruption, the control strategy switches from grid connection mode to grid failure mode. The main goal is to supply the most electricity possible to loads when the grid is disconnected. The value of the load impedance is initially calculated. Calculating the nominal power value
5 Results and discussion
This study thoroughly evaluates the performance of a proposed flexible control strategy for a grid-connected PV-based fuel cell system. The strategy’s effectiveness was tested under grid failure conditions. The system was modeled in Simulink, while the control algorithm was developed in Stateflow.
5.1 System operation
PV-based fuel cells can generate
During grid-connected operation, the voltages on the grid side stabilize into a steady state condition. This voltage interruption typically happens during the timeframe of
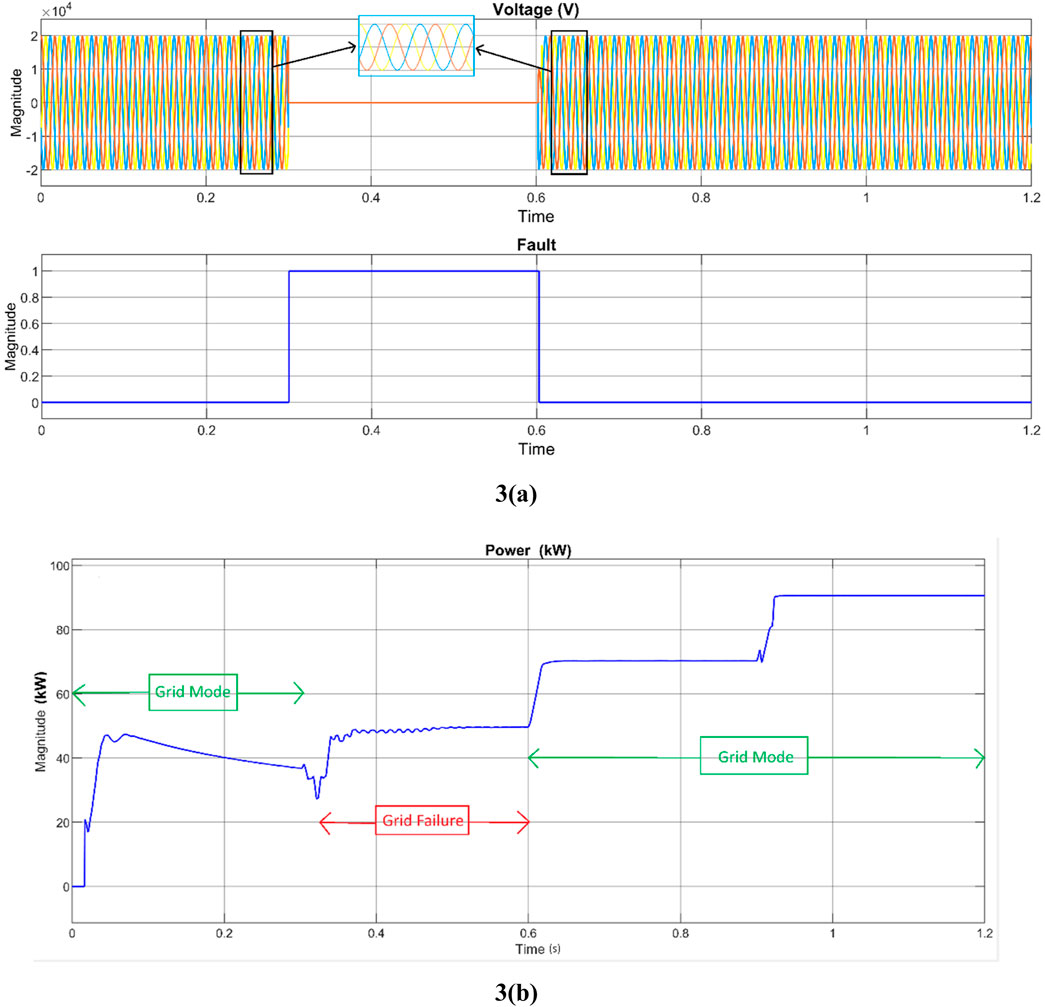
Figure 3. Grid Voltage and System Power Generation under Flexible P&O Control Methods: (A) Grid voltage and grid fault; (B) Results of flexible P&O Control Methods for System Power Generation.
Figure 3B illustrates the power output (in kW) of a PV-based fuel cell system over time, with distinct phases of “Grid Mode” and “Grid Failure.” Initially, the system operates in “Grid Mode,” where the power output rapidly increases and stabilizes around 50 kW, indicating normal operation with grid support. However, around the 0.3 s mark, a fault occurs, causing the system to enter “Grid Failure.” During this phase, the power output drops significantly, indicating a loss of grid support. The system’s output fluctuates, reflecting instability as it attempts to manage the fault condition.
Despite these challenges, the system recovers, and by approximately 0.9 s, it transitions back to “Grid Mode.” The power output stabilizes once again, gradually increasing and reaching a higher steady-state value above 80 kW by the 1.2 s mark. This recovery and subsequent stabilization demonstrate the system’s resilience and ability to maintain a consistent power supply, even in the face of faults. The fault introduced between 0.3 s and 0.6 s serves as a critical test of the system’s robustness and fault-handling capabilities.
The charts shown in Figures 4A, B provide a thorough performance comparison of the two algorithms in a visually appealing and instructive way. These graphs visually summarize the comparison analysis, making it easier to grasp the efficiency and capabilities of the algorithms.
5.2 Evaluation results
Analyzing voltage regulation requires considering rise time. As input voltage changes, this measures how quickly the system’s response achieves its final value. Flexible P&O speeds up this procedure. It rises in 0.00999 s, compared to 0.0243 s for the P&O algorithm. The Large discrepancy shows that the Flexible P&O algorithm can swiftly respond to voltage variations, improving system responsiveness. A shorter settling time of 0.4245 s makes the Flexible P&O algorithm stand out. It takes 0.9231 s for the P&O algorithm to settle. Fast stabilization via the Flexible P&O algorithm enhances voltage regulation stability and speeds steady-state achievement.
Flexible P&O also improves power management. Its rise time is 0.0565 s, compared to the P&O algorithm’s 0.3042 s. This signifies faster responsiveness to input power or disturbances, allowing faster output power level attainment. Compared to the P&O algorithm’s 0.9949 s, the Flexible P&O algorithm stabilizes faster at 0.923 s. This expedited stabilization is like a researcher, improving power system stability and dependability by balancing the system faster after interruptions. Overshoot is decreased to 0.0433 by the Flexible P&O method, compared to 0.2788 by the P&O algorithm. A reduced overshoot shows that the Flexible P&O algorithm keeps the power system’s output closer to the desired setpoint, resulting in low variations and consistent power supply.
This study evaluates the performance of a PV-based fuel cell system under various conditions of irradiance and fuel rate, focusing on the differences between the expected (reference) and actual (obtained) values of power output and voltage, as well as the system’s efficiency. The system was designed to deliver specific power outputs at a reference voltage, but the observed deviations under different operating conditions.
At an irradiance level of 500 W/m2 and fuel rate of 500 L per minute, the system was expected to produce 50 kW of power with a voltage of 300 V. However, the actual measurements showed a slightly lower power output of 49.82 kW and a voltage of 296.2 V, resulting in an impressive efficiency of 99.64%. Similarly, under an irradiance of 700 W/m2c (watts per square meter) and fuel rate of 700 L per minute, the system was supposed to output 75 kW at 300 V, and the recorded a power output of 70.27 kW and a voltage of 273.2 V, achieving an efficiency of 93.69%. At the highest irradiance level tested, 900 W/m2 and fuel rate of 900 L per minute, the expected power output was 100 kW with a corresponding voltage of 300 V. However, the system only produced 90.46 kW with a voltage of 272.5 V, maintaining a high efficiency of 90.46%.
These observations indicate that while the system’s actual performance varies with changing irradiance and fuel rate conditions, it consistently maintained high efficiency, suggesting robustness and resilience.
5.3 Discussion
The obtained results highlight several key findings related to the MPPT-based power management controller for PV-based fuel cells systems. The implementation of the improved MPPT algorithms has demonstrated a significant increase in the overall efficiency of the system, as evidenced by the higher energy yield compared to traditional methods.
5.3.1 Comparison with previous studies
The results align with findings from previous studies that emphasize the effectiveness of MPPT algorithms in optimizing energy extraction from hybrid systems. However, this study advances the understanding by incorporating a hybrid energy system with both PV and fuel cell sources. For instance, similar studies have primarily focused on standalone PV systems, while this research provides novel insights into the combined operation of PV and fuel cell technologies. The integration of fuel cells has shown to provide a reliable backup power source, thereby addressing the intermittency issues associated with solar energy.
5.3.2 Significance of findings
One of the significant contributions of this study is the demonstration of the system’s improved performance under various load conditions. The controller’s ability to dynamically adjust power distribution between the PV-based fuel cells sources based on load demand ensures optimal utilization of available resources. This adaptability is crucial for applications in remote and off-grid areas where energy resources can be scarce or highly variable.
5.3.3 Implications and future work
The implications of these findings are far-reaching, particularly in the context of sustainable energy solutions. The successful implementation of the MPPT-based power management controller in PV-based fuel cells systems can pave the way for more efficient and reliable renewable energy systems. Moreover, the reduced dependency on fossil fuels and the potential for decreased carbon emissions make this technology a viable option for addressing global energy challenges.
Future work could focus on exploring the integration of additional renewable energy sources, such as wind or geothermal energy, into the PV-based fuel cells system. Additionally, further research could investigate the long-term stability and durability of the system components, particularly the fuel cells, under continuous operation. Another area of interest could be the development of more sophisticated control algorithms that can handle multiple energy sources and storage options, potentially incorporating machine learning techniques for real-time optimization.
6 Conclusion
This paper presents an adaptable control strategy that effectively tackles the challenges encountered in power systems, whether they are in grid-connected or grid-failure modes. The adaptable control approach has a significant advantage in maximizing power output during grid-connected mode and ensuring optimal power flow in grid-failure scenarios. This proactive approach prevents overloading and significantly improves system stability. This study highlights the effectiveness of the adaptable control strategy, especially in grid-failure mode. This emphasizes the practicality of the proposed approach in real-world applications, addressing the urgent requirement for dependable and effective power management strategies in uncertain grid conditions. It aims to achieve robust performance and optimal power utilization.
Data availability statement
The raw data supporting the conclusions of this article will be made available by the authors, without undue reservation.
Author contributions
MG: Supervision, Project administration, Methodology, Writing–review and editing. MN: Writing–original draft, Software, Data curation, Investigation. KS: Supervision, Writing–review and editing. FN: Investigation, Writing–review and editing. MA: Writing–review and editing, Funding acquisition. MK: Writing–review and editing, Visualization, Formal analysis.
Funding
The author(s) declare that financial support was received for the research, authorship, and/or publication of this article. The author, MK, at King Fahd University of Petroleum and Minerals, would like to express their profound gratitude to King Abdullah City for Atomic and Renewable Energy (K.A.CARE) for their financial support in accomplishing this work. MA would like to acknowledge the support by the Deanship of Scientific Research through King Khalid University, Saudi Arabia funded by the Large Group Research Project RGP2/392/45.
Conflict of interest
The authors declare that the research was conducted in the absence of any commercial or financial relationships that could be construed as a potential conflict of interest.
Publisher’s note
All claims expressed in this article are solely those of the authors and do not necessarily represent those of their affiliated organizations, or those of the publisher, the editors and the reviewers. Any product that may be evaluated in this article, or claim that may be made by its manufacturer, is not guaranteed or endorsed by the publisher.
References
Abdeldjalil, D., Negrou, B., Youssef, T., and Samy, M. M. (2023). Incorporating the best sizing and a new energy management approach into the fuel cell hybrid electric vehicle design. Energy and Environ., 0958305X2311777. doi:10.1177/0958305X231177743
abed El-Raouf, M. O., Mosaad, M. I., Mallawany, A., AlAhmar, M. A., and El Bendary, F. M. (2018). “Mppt of pv-wind-fuel cell of off-grid hybrid system for a new community,” in 2018 Twentieth International Middle East Power Systems Conference (MEPCON), Cairo, Egypt, 18-20 December 2018 (IEEE), 480–487.
Ahmadi, S., Abdi, S., and Kakavand, M. (2017). Maximum power point tracking of a proton exchange membrane fuel cell system using pso-pid controller. Int. J. hydrogen energy 42 (32), 20430–20443. doi:10.1016/j.ijhydene.2017.06.208
Ahmed, J., and Salam, Z. (2016). A modified p&o maximum power point tracking method with reduced steady-state oscillation and improved tracking efficiency. IEEE Trans. Sustain. Energy 7 (4), 1506–1515. doi:10.1109/tste.2016.2568043
Al-Shetwi, A. Q., Sujod, M. Z., and Blaabjerg, F. (2018). Low voltage ride-through capability control for single-stage inverter-based grid-connected photovoltaic power plant. Sol. Energy 159, 665–681. doi:10.1016/j.solener.2017.11.027
Bai, H., and Shang, S. (2010). “A research of combined multifunctional three phase gridconnected inverter/active power filter for pv system,” in The 2nd International Symposium on Power Electronics for Distributed Generation Systems, Hefei, China, 16-18 June 2010 (IEEE), 224–228.
Barakat, S., Osman, A. I., Tag-Eldin, E., Telba, A. A., Mageed, H. M. A., and Samy, M. M. (2024). Achieving green mobility: multi-objective optimization for sustainable electric vehicle charging. Energy Strategy Rev. 53, 101351. doi:10.1016/j.esr.2024.101351
Becherif, M., and Hissel, D. (2010). Mppt of a pemfc based on air supply control of the motocompressor group. Int. J. Hydrogen Energy 35 (22), 12521–12530. doi:10.1016/j.ijhydene.2010.06.094
Bianconi, E., Calvente, J., Giral, R., Mamarelis, E., Petrone, G., Ramos-Paja, C. A., et al. (2013). Perturb and observe mppt algorithm with a current controller based on the sliding mode. Int. J. Electr. Power and Energy Syst. 44 (1), 346–356. doi:10.1016/j.ijepes.2012.07.046
Bjazic, T., Ban, Z., and Milanovic, M. (2012). Modeling of current mode controlled boost converter supplied by fuel cell suitable for controller design purposes. J. Power Sources 198, 203–217. doi:10.1016/j.jpowsour.2011.09.079
Camacho, A., Castilla, M., Miret, J., Garcia De Vicuna, L., and Guzman, R. (2017). Positive and negative sequence control strategies to maximize the voltage support in resistive–inductive grids during grid faults. IEEE Trans. Power Electron. 33 (6), 5362–5373. doi:10.1109/tpel.2017.2732452
Esram, T., and Chapman, P. L. (2007). Comparison of photovoltaic array maximum power point tracking techniques. IEEE Trans. energy Convers. 22 (2), 439–449. doi:10.1109/tec.2006.874230
Garg, A., Nayak, R. S., and Gupta, S. (2015). Comparison of p and o and fuzzy logic controller in mppt for photovoltaic (pv) applications by using matlab/simulink. IOSR J. Electr. Electron. Eng. 10 (4), 53–62. doi:10.9790/1676-10415362
Gergaud, O., Multon, B., and Ahmed, H. B. (2002). “Analysis and experimental validation of various photovoltaic system models,” in 7th International Electrimacs Congress, 1–6.
Golestan, S., and Guerrero, J. M. (2014). Conventional synchronous reference frame phaselocked loop is an adaptive complex filter. IEEE Trans. Industrial Electron. 62 (3), 1679–1682. doi:10.1109/tie.2014.2341594
Guven, A. F., Abdelaziz, A. Y., Samy, M. M., and Barakat, S. (2024). Optimizing energy Dynamics: a comprehensive analysis of hybrid energy storage systems integrating battery banks and supercapacitors. Energy Convers. Manag. 312, 118560. doi:10.1016/j.enconman.2024.118560
Hajizadeh, A., Golkar, M. A., and Feliachi, A. (2010). Voltage control and active power management of hybrid fuel-cell/energy-storage power conversion system under unbalanced voltage sag conditions. IEEE Trans. Energy Convers. 25 (4), 1195–1208. doi:10.1109/tec.2010.2062516
Hwang, S.-H., Liu, L., Li, H., and Kim, J.-M. (2012). Dc offset error compensation for synchronous reference frame pll in single-phase grid-connected converters. IEEE Trans. power Electron. 27 (8), 3467–3471. doi:10.1109/tpel.2012.2190425
Inci, M. (2019). Performance analysis of t-type inverter based on improved hysteresis current controller. Balkan J. Electr. Comput. Eng. 7 (2), 149–155. doi:10.17694/bajece.510412
Li, Q., Chen, W., Liu, Z., Zhou, G., and Ma, L. (2015). Active control strategy based on vector-proportion integration controller for proton exchange membrane fuel cell grid-connected system. IET Renew. Power Gener. 9 (8), 991–999. doi:10.1049/iet-rpg.2014.0245
Mousazadeh, H., Keyhani, A., Javadi, A., Mobli, H., Abrinia, K., and Ahmad, S. (2009). A review of principle and sun-tracking methods for maximizing solar systems output. Renew. Sustain. energy Rev. 13 (8), 1800–1818. doi:10.1016/j.rser.2009.01.022
Nureddin, A. A. M., Rahebi, J., and Ab-BelKhair, A. (2020). Power management controller for microgrid integration of hybrid pv/fuel cell system based on artificial deep neural network. Int. J. Photoenergy 2020, 1–21. doi:10.1155/2020/8896412
Padmanaban, S., Priyadarshi, N., Sagar Bhaskar, M., HolmNielsen, J. B., Hossain, E., and Azam, F. (2019). A hybrid photovoltaic-fuel cell for grid integration with jaya-based maximum power point tracking: experimental performance evaluation. IEEE Access 7, 82978–82990. doi:10.1109/access.2019.2924264
Patel, H., Gupta, M., and Kumar Bohre, A. (2016). “Mathematical modeling and performance analysis of mppt based solar pv system,” in 2016 International Conference on Electrical Power and Energy Systems (ICEPES), Bhopal, India, 14-16 December 2016 (IEEE), 157–162.
Patra, S., Ankur, A., Narayana, M., Mohanty, S. R., and Kishor, N. (2015). Power quality improvement in grid-connected photovoltaic–fuel cell based hybrid system using robust maximum power point tracking controller. Electr. Power Components Syst. 43 (20), 2235–2250. doi:10.1080/15325008.2015.1082680
Prashanth, V., Rafikiran, S., Hussaian Basha, C. H., Kumar, J. A., Dhanamjayulu, C., Kotb, H., et al. (2024). Implementation of high step-up power converter for fuel cell application with hybrid MPPT controller. Sci. Rep. 14 (1), 3342. doi:10.1038/s41598-024-53763-0
Salman, S., Ai, X., and Wu, Z. (2018). Design of a p-&-o algorithm based mppt charge controller for a stand-alone 200w pv system. Prot. Control Mod. Power Syst. 3 (1), 25–28. doi:10.1186/s41601-018-0099-8
Shitole, A. B., Suryawanshi, H. M., Talapur, G. G., Sathyan, S., Ballal, M. S., Borghate, V. B., et al. (2017). Grid interfaced distributed generation system with modified current control loop using adaptive synchronization technique. IEEE Trans. Industrial Inf. 13 (5), 2634–2644. doi:10.1109/tii.2017.2665477
Keywords: solar photovoltaic based fuel cell system, maximum power point tracking, perturb and observe, grid failure, energy surplus
Citation: Gulzar MM, Naeem MM, Shahid K, Naeem FA, Alqahtani M and Khalid M (2024) Strategic optimization of PV integrated fuel cell systems for energy surplus utilization in grid failure scenarios. Front. Energy Res. 12:1467820. doi: 10.3389/fenrg.2024.1467820
Received: 20 July 2024; Accepted: 23 August 2024;
Published: 12 September 2024.
Edited by:
Enrico Pons, Polytechnic University of Turin, ItalyReviewed by:
Kenneth E. Okedu, Melbourne Institute of Technology, AustraliaMohamed Samy, Beni-Suef University, Egypt
Copyright © 2024 Gulzar, Naeem, Shahid, Naeem, Alqahtani and Khalid. This is an open-access article distributed under the terms of the Creative Commons Attribution License (CC BY). The use, distribution or reproduction in other forums is permitted, provided the original author(s) and the copyright owner(s) are credited and that the original publication in this journal is cited, in accordance with accepted academic practice. No use, distribution or reproduction is permitted which does not comply with these terms.
*Correspondence: Muhammad Majid Gulzar, bXVoYW1tYWQuZ3VsemFyQGtmdXBtLmVkdS5zYQ==