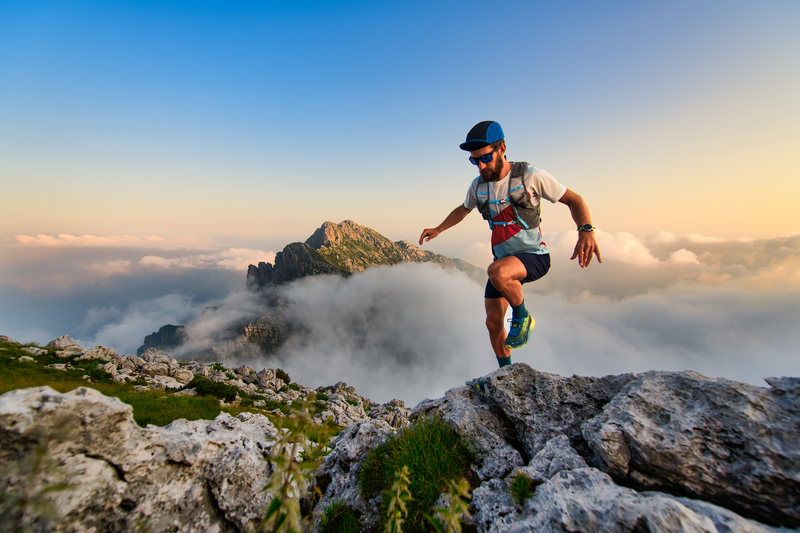
95% of researchers rate our articles as excellent or good
Learn more about the work of our research integrity team to safeguard the quality of each article we publish.
Find out more
ORIGINAL RESEARCH article
Front. Energy Res. , 02 September 2024
Sec. Smart Grids
Volume 12 - 2024 | https://doi.org/10.3389/fenrg.2024.1458115
This article is part of the Research Topic AI-based Energy Storage Systems View all 11 articles
The presented work addresses the growing need for efficient and reliable DC microgrids integrating renewable energy sources. However, for the sake of practicality, implementing complex control strategies can increase system complexity. Thus, efficient methodologies are required to provide efficient energy management of microgrids while increasing the integration of renewable energy sources. The primary contribution of this work is to investigate the issues related to operating a DC microgrid with conventional control designed to power DC motors using readily available, non-advanced control strategies with the objective of achieving stable and reliable grid performance without resorting to complex control schemes. The proposed microgrid integrates a combination of uncontrollable renewable distributed generators (DGs) alongside controllable DGs and energy storage systems, including batteries and supercapacitors, connected via DC links. The Incremental Conductance (InCond) algorithm is employed for maximum power point tracking to maximize power output from the PV system. The energy management strategy prioritizes the solar system as the primary source, with the battery and supercapacitor acting as backup power sources to ensure overall system reliability and sustainability. The effectiveness of the microgrid under various operating conditions is evaluated through extensive simulations conducted using MATLAB. These simulations explore different power generation scenarios, including normal operation with varying load levels and operation under Standard Test Conditions (STC). Moreover, fault analysis of the DC microgrid is performed to examine system reliability. The system performance is evaluated using real-time simulation software (OPAL-RT) to validate the effectiveness of the approach under real-time conditions. This comprehensive approach demonstrates the efficacy of operating a DC microgrid with conventional controllers, ensuring grid stability and reliability across various operating conditions and fault scenarios while prioritizing the use of renewable energy sources. The results illustrated that system efficiency increases with load, but fault tolerance measures, can introduce trade-offs between reliability and peak efficiency.
The modern power grid is moving toward utilizing distributed energy resources (DERs). The shifting trend from conventional generation based on fossil fuels to dispersed renewable generation aims to reduce greenhouse gas emissions, which have raised many global environmental concerns (Wu et al., 2024). In addition, the shortage and the increasing cost of fossil fuels are causing security concerns regarding the balance between energy supply and demand. In order to meet the need for electrical energy, the utilization of renewable energy sources (RES) is considered a solution to the problem of the deficit of energy supply. The low generation costs and maximum power point tracking (MPPT) capability of solar and wind energy make them the most promising RES technologies (Hossain and Ali, 2016; Kumar et al., 2024). According to the International Renewable Energy Agency (IREA), RES will supply 66% of global energy consumption by 2050 (Gielen et al., 2019). Microgrids (MGs) have recently received significant concentration from many researchers as a promising solution to better utilizing large penetration of RES. Thus, the future distribution grids may consist of a group of microgrids that generate, deliver and store energy. It is an autonomous energy system that serves a particular geographic region. The configuration of the microgrids depends on different types of DERs, such as solar panels, wind turbines, microturbines, and energy storage systems (ESSs), e.g., battery energy systems (BES) and supercapacitors (Marwali et al., 2009; Gowtham et al., 2018). Based on their voltage characteristics, microgrids are categorized into AC microgrids, DC microgrids, or Hybrid AC /DC microgrids. MGs can operate in grid-connected modes, exchanging power with the distribution grid, or operate in islanding modes supplying energy to their local energy demands. In case of catastrophic events, MGs can operate in islanding modes to restore power to some critical loads, hence improving the availability of energy supply. Besides being environmentally friendly, MGs have several advantages, such as providing grid isolation during disturbances, reducing peak load on the grid to prevent failures, and enhancing energy efficiency. However, they face some operational challenges, including maintaining acceptable voltage and frequency, ensuring power quality standards, and difficulty in resynchronization with the utility grid. In islanded green microgrids that consist of only renewable energy sources, the problems related to voltage regulation, power sharing, and battery management are even more severe and require more sophisticated control strategies.
DC microgrids are becoming increasingly popular compared to conventional AC microgrids due to their compatibility with renewable energy sources and electronically controlled loads. DC microgrids offer several advantages, including the absence of conflicts with harmonics or frequencies, eliminating the need for synchronization in islanded mode, and not requiring reactive power control. (Gandhi and Gupta, 2021; Saleh Al-Ismail, 2024). In such a DC microgrid, uncontrollable DGs based on renewable technologies, controllable DGs, and energy storage systems are coupled via DC links. Microgrids with energy storage devices, such as BES and supercapacitors, can enhance system reliability. On the one hand, the stored energy can supply the electric demand during peak hours; on the other hand, the BES and supercapacitors can be recharged during off-peak hours.
An energy management system (EMS) is used to monitor energy usage and control and optimize the generation units of the grid. The EMS generally involves instruments to monitor energy usage, predict future energy demands, control generation assets, and report on the interventions performed. It provides utilities and building management services with real-time data and the ability to tune energy utilization, enabling to minimize energy expenses and promote effectiveness. During periods of peak demands, the EMS may respond to signals from the energy grid to temporarily reduce energy consumption by shedding non-critical loads to stabilize the grid and prevent blackouts. Overall, the EMS aims to ensure optimal energy management of the grid, minimize energy costs, and reduce environmental impact resulting from major power outages.
Several research studies have proposed energy management systems for microgrids in the literature. An effective energy management system for the DC microgrid with PV systems was proposed in (Awaji et al., 2022), which consists of a photovoltaic system with BES to enhance power generation when the power output of the photovoltaic (PV) system is insufficient and a DC shunt motor as a dynamic load. The DC microgrid was studied in three distinct scenarios of weather conditions to analyze the behaviour of the BESS under the variations of power generation and highlight the contributions of BES in stabilizing the system under the stochastic nature of RES and demand variability in the microgrid. The paper (Alarbidi et al., 2023) introduced modelling and control strategies for a fixed-speed wind turbine powered by a permanent magnet synchronous machine (PMSM). Additionally, a case study was conducted to illustrate the impact of wind energy on the short circuit level. The torque-controlled PMSM was regulated using the field orientation approach, which involved the modelling and control approach for the frequency converter on the generator-rotor side. Reference (Rana and Abido, 2017) presented a strategy for energy management of a DC microgrid with PV systems. Here, a model predictive controller (MPC) was proposed to control the power flow between the bidirectional voltage source converter (VSC) and the grid. The implementation of the proposed controller was assessed and compared with that of the classical PI controller, and the results showed that the MPC outperformed the classical PI control. The hybrid ESS’s frequency control, consisting of a battery and a supercapacitor, was used in the microgrid to eliminate frequency power variation by exchanging power with the DC bus. In the study (Rashid et al., 2021), an efficient approach for home energy management of community microgrids was developed. Here, the microgrid considers RES, distributed battery energy systems, one centralized battery storage system, and demand response programs. The results show potential financial returns for residential customers and facilitate sustainable energy generation options in a community. The positive aspects of leveraging RES, BES, and central BES with the proposed optimal power-sharing algorithm were assessed. Reference (Ahmed et al., 2019) presented a control methodology for the DC microgrid energy managing utilizing hybrid ESS. The microgrid comprised RES, variable load, hybrid ESS, and diesel generator. The implementation of the proposed microgrid was validated using simulation, where the voltage level of DC under load variation and intermittent RER never exceeded the allowable tolerance, i.e., ± 5%. A coordinated control methodology for the energy management of a microgrid in a grid-connected mode with solar PV and BES was proposed in (Zhang et al., 2024a). The algorithm coordinated the VSC and bidirectional DC-DC converter relied on the battery’s state of charge (SOC). It also compensated for the imaginary power of the load and minimized the unbalanced neutral current. Reference (Alahmed et al., 2019) proposed a dynamic load prioritization methodology built on the artificial neural network (ANN) for microgrid reconfiguration. The proposed method prioritized the microgrid demands relied on the current time, available energy supply, and system reliability factors to provide flexible load restoration under different hours and system conditions. An energy management method using fuzzy logic was applied in (Athira and Pandi, 2017) to ensure generation demand balance in the islanded DC microgrid. The system was evaluated under different load conditions to ensure proper operation of the microgrid with PV panels and a hybrid energy storage system composed of a battery and an ultracapacitor to enhance the battery life. A DC microgrid with a standalone solar PV system was proposed in (El-Shahat and Sumaiya, 2019), aiming to enhance the power conversion efficiency and ensure system permanency. The proposed model reduced the component losses and increased the system efficiency by improving the dynamic response, minimizing the harmonic losses, and obtaining a stable maximum power point value using sum-of-squares optimization for the PV system. Reference (Chahal et al., 2023) developed a model for inverter-based distributed generation technology. Here, a control strategy was employed to enhance disturbance rejection and incorporate an extended range of frequency modes through an analogous internal model. Particle swarm optimization (PSO), focused on minimizing the error in the actual power, was used to discover the best controller parameters. A microgrid operating in grid-connected and autonomous modes was described in (Hussaini et al., 2022) using linear and nonlinear models. An optimization algorithm was proposed for designing various controllers, filters, and power-sharing coefficients. The outcomes validated the efficiency of the suggested PSO approach in tuning the PI regulator, filter, and power-sharing coefficient settings to attain adequate system performance under several disturbances. The microgrid’s stability was examined using nonlinear time-domain simulations in a project to construct a nonlinear model of a self-controlled microgrid published in (Wang S. et al., 2024). The PSO methodology was utilized to ascertain the optimal values for the tuned parameters. The outcomes attest to the strength and efficacy of the suggested PSO-based methodology. The real-time digital simulator (RTDS) outcomes validated the suggested controllers’ efficacy for the system under consideration under various disturbances and operating situations. In (Hassan et al., 2018), the influence of active load on the microgrid’s dynamic stability is investigated. The accompanying controllers are developed for an autonomous microgrid with three DGs based on inverters and an active load. The outcomes showed that the suggested controller has the potential to increase the microgrid’s stability and offer effective dampening properties. Additionally, it demonstrated good performance with the autonomous microgrid under consideration having effective damping properties. When used on MG, the study in (Naderi et al., 2023) examined several System of Systems (SOS) control mechanisms. The study concluded that a networked control system allows for improved microgrid control. The (Keerthisinghe and Kirschen, 2020) microgrid concept featured solar systems, a battery, a backup generator, loads, and an electric vehicle charging station with grid connectivity. The performance of the microgrid was evaluated under three operating scenarios: grid-connected mode, islanding mode microgrid using only the battery, and islanding mode microgrid operating using the backup generator. A microgrid constructed by Snohomish PUD in Arlington, Washington, was simulated in real-time using the real-time digital simulator OPAL-RT. Methods for detecting local and remote microgrid islanding were provided in the paper (Rami Reddy et al., 2024). In each class, various detection techniques were investigated, and the benefits and drawbacks of each technique were reviewed based on some assessment indices, such as non-detection zone, detection time, fault detection rate, and power quality indices. The use of signal processing approaches and intelligent classifiers to modify islanding procedures recently was also covered. The control and sharing of real and reactive power outputs of the inverter-based DGs was suggested using an optimal PQ control technique (Ahmad et al., 2023). Here, the study was conducted on two instances of microgrids with various structures, and various disturbances were used to study how well the microgrid performed. The research study found that the dynamic stability of the microgrid was enhanced using the suggested optimal control. A powerful control technique for voltage balancing, grid synchronization, and power management was developed in (Worku et al., 2021) to enhance the stability and dependability of a microgrid based on DERs. The system model and control approach were created in an RTDS. The outcomes demonstrated the efficacy of the suggested control strategy in grid-connected microgrids, islanding microgrids, and microgrid resynchronization.
In short, an energy management system constantly monitors energy use by analyzing real-time data and weather patterns; it predicts future demand and controls generation, including renewables, for efficient power production, as explored in (Lei et al., 2023). Moreover, it provides utilities with valuable data for cost-saving and environmentally friendly grid improvements while integrating renewable energy (Xie et al., 2016). Recent advancements offer promising solutions to microgrid challenges. Research is shifting towards more sophisticated models that capture the dynamic behavior of DERs within the microgrid, as proposed in (Kumar et al., 2024). Additionally, exploring novel control strategies using artificial intelligence and machine learning for optimized energy management and enhanced system resilience holds significant potential, moving beyond approaches such as particle swarm optimization (PSO) (Chahal et al., 2023; Hussaini et al., 2022), as demonstrated in research on a DC microgrid energy management system (Awaji et al., 2022). This paper dives deeper into the challenges faced by isolated, renewable-powered microgrids (MGs). To ensure alignment with current research, we comprehensively review recent literature, including microgrid dynamics and control (Gandhi and Gupta, 2021), virtual inertia control for DC microgrids (Saleh Al-Ismail, 2024), advanced control strategies like optimal PQ control and networked control systems for MGs (Naderi et al., 2023; Ahmad et al., 2023), and islanding detection methods (Rami Reddy et al., 2024). Table 1 summarizes the existing work on microgrid dynamics and control strategies.
Several studies on real-time energy management simulations have examined renewable energy integration in microgrids, leading to many approaches to enhancing their efficiency and stability. In (Shirkhani et al., 2023), an extensive survey of decentralized energy management and voltage controls for microgrids is presented and highlights some crucial operational challenges. An online reinforcement learning-based energy management for centrally controlled microgrids is introduced in (Meng et al., 2024), highlighting how adaptive learning can be useful in real-time optimization. Moreover, Ref. (Zhou et al., 2024). proposes a multi-stage adaptive stochastic-robust scheduling approach for hydrogen-based multi-energy microgrids, incorporating stochastic programming and robust optimization to enhance system resilience against uncertainties. Furthermore, (Zhang et al., 2024b), emphasizes embedded DC power flow regulators based on full-bridge modular multilevel converters, which control power flow and improve reliability. Ju et al. (2022) proposes a distributed three-phase power flow for AC/DC hybrid microgrids while considering converter limiting constraints aiming at tackling complexities associated with hybrid AC/DC microgrids.
This presented paper investigates the issues related to operation of a DC microgrid powered by solar and controllable DGs, using readily available batteries and supercapacitor storage connected via DC links. The well-established InCond algorithm has been employed to maximize solar output, while batteries and supercapacitors operate as backups. The energy management strategy for the proposed DC microgrid is based on the system operators’ experience, where the solar system is the primary source, while the battery and supercapacitor act as backup power sources. The proposed model will be evaluated through various scenarios, i.e., normal operation and load changes, to ensure effectiveness considering the utilization of the non-advanced controller. All results are tested in a real-time condition using simulation software (OPAL-RT). The combined BES and supercapacitor approach addresses the limitations of BES alone, improving power stability and system efficiency and offering a more comprehensive performance evaluation by considering diverse weather conditions. This demonstrates the feasibility of a reliable and efficient DC microgrid maximizing renewable energy with conventional controls. The contribution of the presented paper can be summarized as follows:
• Analyzing a DC microgrid combining RES and energy storage technologies, such as BES and supercapacitors, to overcome the limitations of battery-based microgrids and leveraging the InCond method for the MPPT of the PV system.
• Evaluating the implementation of the DC microgrid with several practical tests to evaluate the stability of the grid under different operating conditions, including steady-state scenarios, and providing fault analysis on the DC microgrid to assess system reliability.
• Conducting a real-time simulation using the real-time simulator (OPAL-RT) to validate the performance of the suggested DC microgrid.
By addressing these aspects, this paper not only advances the field of energy management in DC microgrids but also offers practical profits like improved energy efficiency, minimized emissions, cost savings, and validation through rigorous simulations. The structure of this article is as follows: Section 2 provides an explanation of the DC Microgrid, while Section 3 contains the system model. Section 4 includes simulation results and a discussion on energy management. Section 5 provides a concise overview of the research findings and explores potential avenues for future research.
A microgrid is a small-scale grid that comprises DGs and ESSs to serve local loads (Dawoud et al., 2021). The microgrid may operate in conjunction with the main grid, referred to as grid-connected mode, or operate independently in an islanded mode, and it can be AC, DC, or hybrid AC-DC based on the voltage characteristics (Fani et al., 2022). The microgrids operating in grid-connected mode can offer ancillary services to the main grid, such as voltage and frequency control, to improve the system’s flexibility and reliability by trading energy based on the available energy supply and current energy demands. In this grid-connected mode, DERs operate in an MPPT mode to supply the maximum available power to the grid. The microgrid operating in islanding mode, i.e., isolated from the main grid, can serve its local load from the DERs (Worku et al., 2019). In these microgrid operational modes, the power generation and demand balance is the most critical issue in the energy management of the microgrids. In AC microgrids, DC sources such as PV systems are coupled with the AC system using DC/AC converters. In DC microgrids, DC sources are directly linked to the DC bus, and AC sources are coupled with the DC bus via AC/DC converters. Therefore, DC microgrids have higher system efficiency than AC microgrids due to less power. Converters are required and hence, less investment and operating costs. Hybrid microgrids can supply energy to AC and DC loads; however, they are more complex than DC microgrids. The DC Microgrid can facilitate the utilization of large RES with less complexity and operating costs compared with its counterparts. However, the optimal operation of microgrids should be addressed to ensure efficient performance of both grid-connected and islanding modes and promote sustainable power generation (Jirdehi et al., 2020).
Figure 1 displays the configuration of the DC microgrid. The physical layer is comprised of PV, battery and super capacitor connected to the DC bus through a DC-DC converter to supply a local load. The power production of the photovoltaic (PV) system is influenced by the diurnal cycle, encompassing both day and night periods. Consequently, it has an adverse impact on the DC motor’s ability to meet the necessary power demand. Hence, using Battery Energy Storage (BES) in conjunction with the Photovoltaic (PV) system is imperative for a streamlined energy management system to maximize system performance during critical scenarios. The connection in a direct current (DC) system is regarded as simpler compared to an alternating current (AC) system due to the sole requirement of regulating the voltage in a DC bus. In the DC microgrid, distributed generators, ESSs, and local loads are linked via a single DC bus using line regulating converters or are directly connected to the DC bus depending on the operating voltage.
The MPPT algorithm can be employed to maximize the power output of the PV panel by regulating the output voltage and current, considering the variations in irradiance (Elbarbary and Alranini, 2021). Several methods exist to achieve this goal. Among them, the Incremental Conductance (InCond) algorithms are widely used (Seyedmahmoudian et al., 2014). Other approaches for the MPPT of the PV systems leverage heuristic algorithms, such as fuzzy logic control and neural networks. However, these methods provide a local maximum or approximated solution for the MPPT. The V-P curve of the PV panel under normal conditions contains merely one maximum value. However, partial shading of the PV panel may lead to multiple maxima in the curve. The InCond method for the MPPT of the PV system is developed based on the “hill-climbing” principle (Seyedmahmoudian et al., 2014), which involves adjusting the operation point of the PV panel in a direction that can increase the output power of the PV panel. The hill-climbing approach is prevalent for the MPPT of the PV system because of its straightforward implementation and satisfactory performance, particularly during constant solar irradiation. Although this method offers simplicity and requires low computational power, it also has some drawbacks. Oscillations can occur around the MPP, and during rapidly changing weather conditions, the algorithm may fail to track the MPP and move in the wrong direction.
In this paper, the InCond method will be utilized for the MPPT of the PV panels, primarily because of its advantages over other techniques mentioned earlier. The flowchart presented in Figure 2 outlines the process of the InCond MPPT method (Seyedmahmoudian et al., 2014). This method tracks MPP through a simultaneous comparison of InCond (
Figure 2. Flowchart of InCond Algorithm obtained from (Seyedmahmoudian et al., 2014).
Herein, the DC microgrid system model is presented. The model comprises a PV system, power converters, BES, supercapacitors, and a DC shunt motor. The following subsections will explain each component in the proposed DC microgrid.
In the PV system utilizes solar cells composed of semiconductor materials capable of converting solar energy into electrical energy. The photovoltaic (PV) system produces a specific quantity of electrical power determined by the characteristics of voltage (V) and current (I) known as P-V and I-V properties (Zhang et al., 2021). The cells in the PV system are interconnected in either series, parallel, or a hybrid configuration to create a PV array, which is determined by the specific purpose of the system. Equation 1 describes the correlation between the current and voltage of the photovoltaic system.
where
In order to compensate for the fluctuation in solar irradiance and maintain a consistent output power, the PV system employs a DC-DC boost converter. This converter is responsible for regulating the output voltage to achieve the desired level, as depicted in Figure 4. In addition, the MPPT algorithm is employed to ascertain the optimal power output of the photovoltaic (PV) system by regulating the voltage and current (Hashim et al., 2018).
There are several techniques to determine the maximum power of the PV system in the literature (Elbarbary and Alranini, 2021; Abdel-Salam et al., 2018). In this paper, the incremental conductance (InCond) for MPPT of the PV system is used. The output DC voltage of the PV system is then stepped-up using power converters to a higher voltage level to match the operating voltage. The choice of switching device is determined by the particular use case, with options including MOSFETs and other semiconductor switching devices. This study utilizes the DC-DC boost converter. The DC-DC boost converter is comprised of two semiconductors, namely a diode and a MOSFET, along with a series inductor and a shunt capacitor. These components are connected to the terminals of the PV system. Upon activation of the MOSFET, the inductor experiences a short circuit, resulting in the accumulation of a substantial charge. This charge is subsequently amplified using the boost DC-DC converter located at the DC bus. Upon deactivation of the MOSFET, the capacitor is connected in parallel with the DC bus in order to maintain a consistent operating voltage. The parameter of the boost DC-DC converter can be approximated using Equations 2–6 as stated in reference (Wang and Zhang, 2023).
where
The BES comprises of a battery storage unit, where the size of the battery is calculated according to the size of DC loads, and a bidirectional buck-boost system DC-DC, illustrated in Figure 5. A bidirectional buck-boost converter is utilized to allow a bidirectional power flow of the BES during the charging and discharging modes.
In this setup, the terminals of both the Battery Energy Storage and the supercapacitors are linked to a buck-boost DC-DC converter, which includes a diode connected in series with the load. A parallel connection is made between the switch and the diode using an inductor, while a shunt capacitor is connected to the DC bus. The buck-boost DC-DC converter has the capability to function in either buck mode, where it charges the Battery Energy Storage, or boost mode, where it releases the stored energy from the BES to the grid. During the off period, the buck-boost DC-DC converter produces an output voltage that has a polarity opposite to that of the input voltage. When the switch is activated, an electric current passes through both the switch and the inductor for a specific duration. During this period, the induced voltage has the same polarity as the input voltage, meaning that the rate of change of current over time (di/dt) is positive. When the switch is in the OFF position, the current in the inductor will drop, causing the induced voltage to change polarity. The rate of change of current (di/dt) is negative, resulting in the output voltage having the opposite polarity of the input voltage. The shunt capacitor will ensure a consistent output voltage.
The Battery Energy Storage (BES) system comprises a series of lithium-ion batteries connected to a bidirectional DC-DC buck-boost converter. There are various types of batteries include lithium-ion, lead-acid, and Ni-Cd batteries. This paper utilizes lithium-ion batteries due to their superior energy density compared to lead-acid and Ni-Cd batteries. In this battery variant, the electrodes are made thicker in order to counterbalance the formation of a passivation film resulting from the interaction between the lithium electrodes and the electrolytes during each cycle of charging and discharging. Nevertheless, due to the presence of denser electrodes, lithium-ion batteries incur higher costs compared to Ni-Cd batteries. This article utilizes Lithium-ion batteries and a bidirectional DC-DC buck-boost converter. The battery capacity is determined based on the DC load. The battery’s characteristics, namely the terminal voltage and state of charge (SOC), can be determined using Equations 7, 8 (Akram et al., 2018).
here, the open-circuit voltage has been named
During a critical situation, the battery can serve as a contingency. The battery is replenished from the photovoltaic (PV) source when the generated power of the PV system exceeds the power required. The battery can be utilized in discharge mode when the power supply of the microgrid is insufficient, specifically when the PV source is inadequate to meet the load requirement (Mohan et al., 2002) as shown in Equation 9 and Equation 10.
where
It is imperative that the control, operation, and energy management of the microgrid be carried out in an appropriate manner in order for a smart electrical grid to work efficiently (Sweidan, 2017). The microgrid has a power imbalance, particularly when it is running in the islanded mode, as a result of the considerable integration of intermittent renewable energy sources (RES) and changeable load swings. In the literature, several solutions have been offered to overcome this issue. Some of these options include the support of conventional generation and the sharing of energy amongst smaller microgrids that are located nearby (Wang et al., 2017). The use of energy storage systems is one of the effective methods that can be utilized to lessen the fluctuations in power and improve the stability of microgrids (Zia et al., 2018). In addition, energy storage has the capability of reducing peak demand, enhancing the grid’s transient stability, and storing extra energy for use at a later time. A number of different energy storage technologies, such as batteries, supercapacitors, flywheels, and superconducting magnetic energy storage, have been utilized in order to enhance the stability of microgrids. An extremely high-energy density storage solution for long-term energy sources is the combination of supercapacitors and batteries, which is the most promising option (Nazaripouya et al., 2019).
A DC shunt motor is one example of a dynamic load that may be supplied by the DC microgrid. DC shunt motors are able to transform the electrical DC power that is fed into them into the mechanical power that is produced, which can be seen in the motor’s speed and torque. The armature winding of the DC shunt motor is connected in parallel with the field winding of the motor that is being used. When the DC power source is applied to the DC shunt motor, a magnetic flux is produced in the stator, which induces the voltage in the armature winding. Advanced controller can be applied to control the DC shunt motor as in (Wang et al., 2024b) to address the current and speed loop. The nonlinear dynamic model of the DC shunt motor can be represented by (Equations 11–14) (Kumar et al., 2022).
here, the rotor armature current has been named
The performance of the Microgrid is validated by using a real-time simulation (Opal-RT), as shown in Figure 6. The proposed DC microgrid and the proposed algorithms are implemented using MATLAB Simulink. Subsequently, we compiled the algorithmic code in Opal-RT (OP4510) system. The primary objective is to assess the microgrid’s dynamic behaviour and evaluate the proposed control strategy’s effectiveness under various operating conditions. This research proposes a non-sophisticated control strategy for a DC microgrid powered by solar and controllable DGs, using readily available battery and supercapacitor storage connected via DC links. The control method is based on prioritizing the available sources in the DC microgrid. The power output of the PV system is of high priority, as it will supply the DC motor and charge the BES. When solar irradiation is low, the BES and supercapacitors will kick in to supply the DC motors. The control signal will be sent by the system operator from the control system that is considered to be a centralized controller who has full access to the available power sources. The well-established InCond algorithm maximizes solar output, while batteries and supercapacitors act as backup. Three main power generation scenarios for the energy management strategy are conducted:
• Full System: Utilizing all available sources - PV system as primary sources, while energy storge system BES and supercapacitors as backup sources.
• Standalone PV: Evaluating system performance solely with the PV system as the primary power source.
• Backup Power Only: Assessing the microgrid’s ability to operate solely on BES and supercapacitors, simulating a critical scenario where the PV system is unavailable.
The DC microgrid is tested under these power generation scenarios. Firstly, power sources include the PV system, BES, and supercapacitors used to form the DC microgrid. The second scenario considers a standalone PV microgrid, and the last scenario is the operation of the DC microgrid consisting solely of the backup power sources, i.e., BES and supercapacitors. These different power source scenarios are used to test the dynamics of the microgrid and evaluate the performance of the proposed method under Standard Test Conditions (STC). The following cases are performed to identify the system behaviour under steady-state conditions. Moreover, the case study evaluates the performance of the ESS under different operating conditions. Lastly, the fault analysis on the DC microgrid is performed. It is worth noting that the power rating of the system under study is considered to be 20 kW. These cases have been listed as follows:
1. Steady-State Scenarios:
• Case 1: Testing under no load to establish baseline performance.
• Case 2: Testing under full load to evaluate system capability.
2. Fault Scenarios:
• Case 3: Simulating a PV system fault to assess system response and recovery.
It is necessary to do the steady-state analysis of the DC microgrid both with the full load and without any load in order to guarantee the DC microgrid’s operation that is both stable and dependable. This analysis aims to identify the system’s steady-state behaviour when subjected to different load conditions. To evaluate the steady-state performance, the simulation of the DC microgrid with the full load and no loads are conducted to observe how the system responds over time. The electrical torque values have been chosen in the nominal case with the full load.
To determine the motor’s rotational speed or “no-load speed” and other parameters, this test is conducted by running the motor without any load or external resistance connected to its shaft. The purpose of a no-load test is to evaluate the power flow in the DC microgrid with three power generation scenarios, i.e., the DC microgrid with all power sources, the standalone PV microgrid, and the DC microgrid operating using BES and supercapacitors. Figure 7 shows the torque of the DC motor with no load. Figure 8 shows the output power of the DC microgrid under the three power generation scenarios.
This test is similar to Case 1, but the DC microgrid is tested when running the motor with a nominal load. The electrical torque (Te) is constant when the motor’s shaft is connected to external resistance and equal to 40 N.m. As shown in Figure 8, the battery and the supercapacitor are charged from the PV system when there is no load in Case 1. In addition, if we sum the power of both the battery and supercapacitor, it will give us the PV’s power without the motor effective at no load. On the other hand, in Case 2, with the full load, we have a similar behaviour compared with Case 1. The PV system can supply all the load in the DC microgrid and charge the backup sources, and the motor operates more effectively than in Case 1. By comparing the torque of the DC motor in Case1, shown in Figure 7, with Case 2, shown in Figure 9, it can be noticed that the performance of the DC motor in Case 2 is better than in Case 1 due to the presence of the full load in Case 2. Moreover, the comparison reveals that the absence of load initially creates instability issues and slow transient responses, as shown in Figure 7.
B. FAULT OPERATION
In the following case, fault analysis is performed to evaluate the reliability of the DC microgrid. In this case, a fault test is applied to the PV system with the short voltage type. Figure 10 shows the DC motor torque, and Figure 11 shows the power output of the power sources during the fault in the PV system. As Figure 11 shows, when the PV fault occurs, the BES supplies the DC motor. It is illustrated that the battery fluctuated in the beginning and then supplied the whole system with the desired output.
Table 2 describes the load/no-load, and fault tolerance on the system efficiency based on the results obtained in the case study. As can be seen, Comparing the load and no-load conditions, the system efficiency in Case 2 is higher efficiency than in Case 1. Hence, operating with the DC microgrid in full load conditions reduces loss, leading to higher system efficiency. Moreover, performing fault analysis Case 3 shows that BES enhances the system’s efficiency and ensures uninterrupted power during faults.
Sever studies have focused on energy management strategies in DC microgrids. Furthermore, all these studies have agreed on efficient power management and system reliability are crucial. However, these studies have employed different in methods as well as areas of focus. While (Li et al., 2023; Duan et al., 2023) utilized optimization focused decision-making process to improve the performance of their systems (Li et al., 2023), relies on using artificial intelligence (AI) whereas bidirectional converters are used by (Duan et al., 2023). Conversely (Luo et al., 2024), focused on using model predictive control for enhanced power flow whereas (Li et al., 2023; Duan et al., 2023) discuss energy losses based on their observation. Nevertheless, these studies serve the same objective of energy management in a DC microgrid. It is obvious that the performance could be slightly different as a result of complex control methodology. The presented paper showed adequate performance with a conventional controller.
For future work, one potential area involves investigating the influence of frequency on the microgrid’s behaviour. Additionally, incorporating advanced control systems capable of predicting changes in solar power output and adjusting power supply accordingly could further reduce power fluctuations and enhance stability. Overall, this study provides a valuable foundation for developing and optimizing reliable DC microgrids that effectively integrate renewable energy sources like solar power. A valid extension of the presented work is performing an experimental validation.
The proposed paper develops an energy management system (EMS) for a DC microgrid, aiming to ensure stable and dependable power delivery for DC motors with conventional control strategies. The microgrid integrates a photovoltaic (PV) system, battery energy storage (BES), and supercapacitors to provide a robust power supply. To optimize PV power generation, the Incremental Conductance (InCond) technique is employed for maximum power point tracking (MPPT). Furthermore, this work investigates the microgrid’s performance under various operating scenarios, including diverse power generation conditions and fluctuating solar irradiation. The focus is on maintaining grid stability and reliability. Furthermore, the study examines the performance of the BES in both charging and discharging modes, highlighting its crucial role in power management. Additionally, a fault analysis is conducted to assess the system’s response to potential failures and its overall dependability. Further validation of the proposed microgrid is achieved through real-time simulation using OPAL-RT and MATLAB. The results demonstrate that under standard test conditions (1000 W/m2 solar irradiation and 25°C), the DC motors operate efficiently across various power generation scenarios. The fault analysis emphasizes the importance of the BES in mitigating instability caused by fault conditions. This highlights the BES’s role in enhancing the microgrid’s overall reliability and ensuring consistent power delivery. The load condition of a system greatly affects its efficiency. This is observable when examining full-load and no-load situations. Conversely, fault tolerance has the potential to affect efficiency through systems such as BES. Even though BES guarantees the constant supply of power during faults, there are energy conversions needed that might lower overall efficiency. Consequently, this gives rise to a trade-off between the reliability of a system and its peak efficiency thus necessitating careful analysis for specific fault conditions. This research has a few limitations. First, the repeated use of the InCond algorithm might limit adaptability to advanced strategies. Additionally, while the study covers various weather conditions, it does not address extreme events or long-term climate impacts.
The original contributions presented in the study are included in the article/supplementary material, further inquiries can be directed to the corresponding authors.
HH: Conceptualization, Data curation, Formal Analysis, Investigation, Methodology, Resources, Software, Validation, Visualization, Writing–original draft, Writing–review and editing. AA (2nd author): Conceptualization, Data curation, Formal Analysis, Funding acquisition, Investigation, Methodology, Resources, Software, Validation, Visualization, Writing–original draft, Writing–review and editing. AA (3rd author): Conceptualization, Methodology, Validation, Visualization, Writing–original draft, Writing–review and editing. SuA: Conceptualization, Funding acquisition, Investigation, Methodology, Project administration, Resources, Software, Supervision, Validation, Visualization, Writing–original draft, Writing–review and editing. SaA: Conceptualization, Funding acquisition, Investigation, Project administration, Methodology, Resources, Software, Supervision, Validation, Visualization, Writing–original draft, Writing–review and editing. MA: Conceptualization, Methodology, Validation, Visualization, Writing–original draft, Writing–review and editing.
The author(s) declare that financial support was received for the research, authorship, and/or publication of this article. The authors would like to express their profound gratitude to King Abdullah City for Atomic and Renewable Energy (K.A.CARE) for their financial support in accomplishing this work. The authors also extend their appreciation to the Deanship of Scientific Research at Northern Border University, Arar, KSA for funding this research work through the project number NBU-FFR-2024-2124-05.
The authors declare that the research was conducted in the absence of any commercial or financial relationships that could be construed as a potential conflict of interest.
All claims expressed in this article are solely those of the authors and do not necessarily represent those of their affiliated organizations, or those of the publisher, the editors and the reviewers. Any product that may be evaluated in this article, or claim that may be made by its manufacturer, is not guaranteed or endorsed by the publisher.
Abdel-Salam, M., El-Mohandes, M. T., and Goda, M. (2018). An improved perturb-and-observe based MPPT method for PV systems under varying irradiation levels. Sol. Energy 171, 547–561. doi:10.1016/j.solener.2018.06.080
Ahmed, M., Kuriry, S., Shafiullah, M. D., and Abido, M. A. (2019). “DC microgrid energy management with hybrid energy storage systems,” in 2019 23rd international conference on mechatronics technology (ICMT) Salerno, Italy, October 23–October 26, 2019 (IEEE), 1–6.
Ahmad, S., Shafiullah, M., Ahmed, C. B., and Alowaifeer, M. (2023). A review of microgrid energy management and control strategies. IEEE Access 11, 21729–21757. doi:10.1109/access.2023.3248511
Akram, U., Khalid, M., and Shafiq, S. (2018). Optimal sizing of a wind/solar/battery hybrid grid connected microgrid system. IET Renew. Power Gener. 12 (1), 72–80. doi:10.1049/iet-rpg.2017.0010
Alahmed, A. S., Taiwo, S. U., Abido, M. A., and Almuhaini, M. M. (2019). “Intelligent flexible priority list for reconfiguration of microgrid demands using deep neural network,” in 2019 IEEE innovative smart grid technologies-asia (ISGT asia), Chengdu, China, May 21–May 24, 2019 (IEEE), 3490–3495. doi:10.1109/ISGT-Asia.2019.8881363
Alarbidi, O. M. F., Awaji, H. H. H., Althobiti, A. A., Alhussainy, A. A., Alghamd, A., Abusorrah, A. M., et al. (2023). “Power quality of DQ synchronous frame for wind connected to grid,” in 2023 13th International Conference on Power, Energy and Electrical Engineering (CPEEE) Tokyo, Japan, 59–64. doi:10.1109/CPEEE56777.2023.10217705
Athira, G. R., and Pandi, V. R. (2017). “Energy management in islanded DC microgrid using fuzzy controller to improve battery performance,” in 2017 international conference on technological advancements in power and energy (TAP energy) Kollam, India, December 21–December 23 2017 (IEEE), 1–6.
Awaji, H. H., Alsulami, A. A. G., Althobiti, A. A., Alhussainy, A. A., Alghamd, A., Milyan, A. H., et al. (2022). “Energy Management System for Direct current (DC) Microgrid,” in 2023 13th International Conference on Power, Energy and Electrical Engineering (CPEEE) Tokyo, Japan, 240–245. doi:10.1109/CPEEE56777.2023.10217378
Chahal, M., Tiwari, G., and Saini, S. (2023). Supervisory control of an inverter-based microgrid using PSO algorithm. SONIPAT, India: 2023 9th IEEE India international conference on power electronics (IICPE), 1–6. doi:10.1109/IICPE60303.2023.10474934
Dawoud, N. M., Megahed, T. F., and Kaddah, S. S. (2021). Enhancing the performance of multi-microgrid with high penetration of renewable energy using modified droop control. Electr. Power Syst. Res. 201, 107538. doi:10.1016/j.epsr.2021.107538
Duan, Y., Zhao, Y., and Hu, J. (2023). An initialization-free distributed algorithm for dynamic economic dispatch problems in microgrid: modeling, optimization and analysis. Sustain. Energy, Grids Netw. 34, 101004. doi:10.1016/j.segan.2023.101004
Elbarbary, Z. M. S., and Alranini, M. A. (2021). Review of maximum power point tracking algorithms of PV system. Front. Eng. Built Environ. 1, 68–80. doi:10.1108/febe-03-2021-0019
El-Shahat, A., and Sumaiya, S. (2019). DC-microgrid system design, control, and analysis. Electronics 8 (2), 124. doi:10.3390/electronics8020124
Fani, B., Shahgholian, G., Alhelou, H. H., and Siano, P. (2022). “Inverter-based islanded microgrid: a review on technologies and control,” in E-prime-advances in electrical engineering, electronics and energy 2, 100068. doi:10.1016/j.prime.2022.100068
Gandhi, K., and Gupta, S. K. (2021). Operational strategies and electricity market structure of microgrid: a critical review. Renew. Energy Focus 39 (39), 163–171. doi:10.1016/j.ref.2021.09.001
Gielen, D., Gorini, R., Wagner, N., Leme, R., Gutierrez, L., Prakash, G., et al. (2019). Global energy transformation: a roadmap to 2050.
Gowtham, K., Sivaramadurai, C. V., Hariprasath, P., and Indurani, B. (2018). “A management of power flow for DC microgrid with solar and wind energy sources,” in 2018 international conference on computer communication and informatics (ICCCI) Coimbatore, India, January 04–January 06, 2018 (IEEE), 1–5.
Hashim, N., Salam, Z., Johari, D., and Ismail, N. F. N. (2018). DC-DC boost converter design for fast and accurate MPPT algorithms in stand-alone photovoltaic system. Int. J. Power Electron. Drive Syst. 9 (3), 1038. doi:10.11591/ijpeds.v9.i3.pp1038-1050
Hassan, M. A., Worku, M. Y., and Abido, M. A. (2018). Optimal design and real time implementation of autonomous microgrid including active load. Energies 11 (5), 1109. doi:10.3390/en11051109
Hossain, M. K., and Ali, M. H. (2016). Transient stability augmentation of PV/DFIG/SG-based hybrid power system by parallel-resonance bridge fault current limiter. Electr. Power Syst. Res. 130, 89–102. doi:10.1016/j.epsr.2015.08.016
Hussaini, H., Yang, T., Gao, Y., Wang, C., Bai, Ge, and Bozhko, S. (2022) “Droop coefficient design and optimization using genetic algorithm-A case study of the more electric aircraft DC microgrid,” in Iecon 2022 – 48th annual conference of the. IEEE Industrial Electronics Society, 1–6.
Jirdehi, M. A., Tabar, V. S., Ghassemzadeh, S., and Tohidi, S. (2020). Different aspects of microgrid management: a comprehensive review. J. Energy Storage 30, 101457. doi:10.1016/j.est.2020.101457
Ju, Y., Liu, W., Zhang, Z., and Zhang, R. (2022). Distributed three-phase power flow for AC/DC hybrid networked microgrids considering converter limiting constraints. IEEE Trans. Smart Grid 13 (3), 1691–1708. doi:10.1109/TSG.2022.3140212
Keerthisinghe, C., and Kirschen, D. S. (2020). 1 real-time digital simulation of microgrid control strategies. 2020 IEEE power and energy society innovative smart grid technologies conference (ISGT) (IEEE), 1–5.
Kumar, A., Ibraheem, S., Gupta, R. K., Nguyen, T. A., and Yasin, G. (2022). “Battery-supercapacitor hybrid systems: an introduction,” in Nanotechnology in the automotive industry (Elsevier), 453–458.
Kumar, K., Kumar, P., and Kar, S. (2024). A review of microgrid protection for addressing challenges and solutions. Renew. Energy Focus 49, 100572. doi:10.1016/j.ref.2024.100572
Lei, C., Bu, S., Wang, Q., Chen, Q., Yang, L., and Chi, Y. (2023). Look-ahead rolling economic dispatch approach for wind-thermal-bundled power system considering dynamic ramping and flexible load transfer strategy. IEEE Trans. Power Syst. 39, 186–202. doi:10.1109/TPWRS.2023.3238035
Li, B., Wang, J., Nassani, A. A., Binsaeed, R. H., and Li, Z. (2023). The future of Green energy: a panel study on the role of renewable resources in the transition to a Green economy. Energy Econ. 127, 107026. doi:10.1016/j.eneco.2023.107026
Luo, J., Zhuo, W., Liu, S., and Xu, B. (2024). The optimization of carbon emission prediction in low carbon energy economy under big data. IEEE Access 12, 14690–14702. doi:10.1109/ACCESS.2024.3351468
Marwali, M. N., Keyhani, A., and Dai, M. (2009). Integration of green and renewable energy in electric power systems. John Wiley and Sons.
Meng, Q., Hussain, S., Luo, F., Wang, Z., and Jin, X. (2024). An online reinforcement learning-based energy management strategy for microgrids with centralized control. IEEE Trans. Industry Appl., 1–10. doi:10.1109/TIA.2024.3430264
Mohan, N., Undeland, T. M., and Robbins, W. P. (2002). Power electronics: converters, applications, and design (3rd ed.). John Wiley & Sons.
Naderi, M., Khayat, Y., Shafiee, Q., Blaabjerg, F., and Bevrani, H. (2023). Dynamic modeling, stability analysis and control of interconnected microgrids: a review. Appl. Energy 334 (2023), 120647. doi:10.1016/j.apenergy.2023.120647
Nazaripouya, H., Chung, Y. W., and Akhil, A. (2019). Nergy storage in microgrids: challenges, applications and research need. Int. J. Energy Smart Grid 3 (2), 60–70. doi:10.23884/ijesg.2018.3.2.02
Rami Reddy, C., Choi, J.-H., Sekhar, O. C., Colak, I., and Khalid, M. (2024). State of the art review of islanding detection methods for integrated distributed generation system. Electr. Power Components Syst. 52, 1906–1935. doi:10.1080/15325008.2024.2314197
Rana, M. J., and Abido, M. A. (2017). Energy management in DC microgrid with energy storage and model predictive controlled AC–DC converter. IET Generation, Transm. and Distribution 11 (15), 3694–3702. doi:10.1049/iet-gtd.2016.1934
Rashid, M. M. U., Alotaibi, M. A., Chowdhury, A. H., Rahman, M., Alam, M. S., Hossain, M. A., et al. (2021). Home energy management for community microgrids using optimal power sharing algorithm. Energies 14 (4), 1060. doi:10.3390/en14041060
Saleh Al-Ismail, F. (2024). A critical review on DC microgrids voltage control and power management. IEEE Access 12, 30345–30361. doi:10.1109/access.2024.3369609
Seyedmahmoudian, M., Mohamadi, A., Kumary, S., Oo, A. M. T., and Stojcevski, A. (2014). A comparative study on procedure and state of the art of conventional maximum power point tracking techniques for photovoltaic system. Int. J. Comput. Electr. Eng. 6 (5), 402–414. doi:10.17706/ijcee.2014.v6.859
Shirkhani, M., Tavoosi, J., Danyali, S., Sarvenoee, A. K., Abdali, A., Mohammadzadeh, A., et al. (2023). A review on microgrid decentralized energy/voltage control structures and methods. Energy Rep. 10, 368–380. doi:10.1016/j.egyr.2023.06.022
Sweidan, T. (2017). Dynamical analysis of DC shunt motor powered by PV generator using perturbation and observation as MPPT tracking technique. Energy Power Eng. 9 (1), 55–69. doi:10.4236/epe.2017.91005
Wang, C., Wang, Y., Wang, K., Dong, Y., Yang, Y., and Hanne, T. (2017). An improved hybrid algorithm based on biogeography/complex and metropolis for many-objective optimization. Math. Problems Eng. 2017, 2462891. doi:10.1155/2017/2462891
Wang, R., Gu, Q., Lu, S., Tian, J., Yin, Z., Yin, L., et al. (2024b). FI-NPI: exploring optimal control in parallel platform systems. Electronics 13 (7), 1168. doi:10.3390/electronics13071168
Wang, R., and Zhang, R. (2023). Techno-economic analysis and optimization of hybrid energy systems based on hydrogen storage for sustainable energy utilization by a biological-inspired optimization algorithm. J. Energy Storage 66, 107469. doi:10.1016/j.est.2023.107469
Wang, S., Lu, T., Hao, R., Li, J., Guo, Y., He, X., et al. (2024a). An identification method for anomaly types of active distribution network based on data mining. IEEE Trans. Power Syst. 39 (4), 5548–5560. doi:10.1109/TPWRS.2023.3288043
Worku, M. Y., Hassan, M. A., and Abido, M. A. (2019). Real time energy management and control of renewable energy based microgrid in grid connected and island modes. Energies 12 (2), 276. . doi:10.3390/en12020276
Worku, M. Y., Hassan, M. A., and Abido, M. A. (2021). Power management, voltage control and grid synchronization of microgrids in real time. Arabian J. Sci. Eng. 46, 1411–1429. . doi:10.1007/s13369-020-05062-9
Wu, Y., Cui, J. Z., and Liu, C. (2024). State-of-the-art review on energy management systems, challenges and top trends of renewable energy based microgrids. EAI Endorsed Trans. Energy Web 10. doi:10.4108/ew.4124
Xie, K., Dong, J., Singh, C., and Hu, Bo (2016). Optimal capacity and type planning of generating units in a bundled wind–thermal generation system. Appl. Energy 164 (2016), 200–210. doi:10.1016/j.apenergy.2015.12.004
Zhang, J., Cho, H., and Mago, P. J. (2021). “Energy conversion systems and energy storage systems,” in Energy services fundamentals and financing. Editor D. Borge-Diez (Academic Press), 155–179. doi:10.1016/B978-0-12-820592-1.00007-5
Zhang, J., Liu, Y., Zang, J., Liu, Z., Zhou, J., Wang, J., et al. (2024b). An embedded DC power flow controller based on full-bridge modular multilevel converter. IEEE Trans. Industrial Electron. 71 (3), 2556–2566. doi:10.1109/TIE.2023.3265041
Zhang, J., Liu, Y., Zhou, J., Zang, J., Shi, G., Wang, J., et al. (2024a). A novel multiport transformer-less unified power flow controller. IEEE Trans. Power Electron. 39 (4), 4278–4290. doi:10.1109/TPEL.2023.3347900
Zhou, Y., Zhai, Q., Xu, Z., Wu, L., and Guan, X. (2024). Multi-stage adaptive stochastic-robust scheduling method with affine decision policies for hydrogen-based multi-energy microgrid. IEEE Trans. Smart Grid 15 (3), 2738–2750. doi:10.1109/TSG.2023.3340727
Zia, M. F., Elbouchikhi, E., and Benbouzid, M. (2018). Microgrids energy management systems: a critical review on methods, solutions, and prospects. Appl. energy 222, 1033–1055. doi:10.1016/j.apenergy.2018.04.103
Keywords: renewable energy sources (RES), battery energy storage (BES), direct current (DC), microgrid (MG), solar photovoltaic (PV)
Citation: Hadi H. Awaji H, Alhussainy AA, Alobaidi AH, Alghamdi S, Alghamdi S and Alruwaili M (2024) Real-time energy management simulation for enhanced integration of renewable energy resources in DC microgrids. Front. Energy Res. 12:1458115. doi: 10.3389/fenrg.2024.1458115
Received: 01 July 2024; Accepted: 19 August 2024;
Published: 02 September 2024.
Edited by:
Flah Aymen, École Nationale d'Ingénieurs de Gabès, TunisiaReviewed by:
Rajeev Kumar, KIET Group of Institutions, IndiaCopyright © 2024 Hadi H. Awaji, Alhussainy, Alobaidi, Alghamdi, Alghamdi and Alruwaili. This is an open-access article distributed under the terms of the Creative Commons Attribution License (CC BY). The use, distribution or reproduction in other forums is permitted, provided the original author(s) and the copyright owner(s) are credited and that the original publication in this journal is cited, in accordance with accepted academic practice. No use, distribution or reproduction is permitted which does not comply with these terms.
*Correspondence: Mohammed Alruwaili, bW9oYW1tZWQuYWxydXdhaWxpckBuYnUuZWR1LnNh; Sultan Alghamdi, c21hbGdhbWRpMUBrYXUuZWR1LnNh
Disclaimer: All claims expressed in this article are solely those of the authors and do not necessarily represent those of their affiliated organizations, or those of the publisher, the editors and the reviewers. Any product that may be evaluated in this article or claim that may be made by its manufacturer is not guaranteed or endorsed by the publisher.
Research integrity at Frontiers
Learn more about the work of our research integrity team to safeguard the quality of each article we publish.