- 1Processes, Energy, Environment and Electrical Systems, National Engineering School of Gabes, University of Gabes, Gabes, Tunisia
- 2MEU Research Unit, Middle East University, Amman, Jordan
- 3The Private Higher School of Applied Sciences and Technology of Gabes (ESSAT), University of Gabes, Gabes, Tunisia
- 4Applied Science Research Center, Applied Science Private University, Amman, Jordan
- 5Electrical Engineering Department, College of Engineering, King Saud University, Riyadh, Saudi Arabia
- 6Advanced High Voltage Engineering Research Centre, Cardiff University, Cardiff, United Kingdom
- 7Department of Electrical Engineering, Bayeh Institute, Amchit, Lebanon
The increasing number of electric Vehicles (EVs) and their influence on the power grid present difficulties that this article addresses by suggesting optimal planning methods for EV charging and discharging. EV charging and discharging operations are effectively managed by creating both locally and globally optimal planning schemes. Future transportation could be changed by the widespread adoption of dynamic wireless power transfer systems in conjunction with EVs, as they would enable speedier travel and continuous EV battery recharging. Dynamic wireless power transfer is a practical answer to problems with electric vehicles. The electrification of automobiles will have a significant influence on the power infrastructure due to the increase in demand for electricity. In this study, we provide an optimal planning method worldwide and a locally optimal strategy for EV charging and discharging. To minimize the total cost of all EVs that charge and discharge during the day, we propose an optimization problem for global planning in which the charging powers are optimized. The simulation results demonstrate that the proposed planning schemes can effectively reduce the total electricity cost for EV owners while also minimizing the impact on the power grid. The globally optimal planning scheme achieves the lowest electricity cost, while the locally optimal scheme provides a good balance between cost reduction and computational complexity.
1 Introduction
With almost one-third of the world’s electricity use going to the transportation industry, it is one of the biggest users of energy in the world (Manzolli et al., 2022). Furthermore, the internal combustion engine (ICE) based current transportation system is the main contributor to air pollution and greenhouse gas emissions. There has been a noticeable surge in interest in electrifying the transportation system in recent years to lessen these negative effects and lessen reliance on fossil fuels. According to a study, electric vehicles have a special niche as a safe alternative (Zhang L. et al., 2023). One claim is that the development of EVs has been hampered by the high cost and dearth of charging stations. As a result, most countries have implemented laws and policies to lower these obstacles and encourage the widespread utilization of electric vehicles.
About this subject, energy transmission by induction has been adapted to this system. Indeed, considering the geopolitical and environmental background, this recharge mechanism has emerged as a very attractive field of study. The charging tool’s best and most effective solutions may produce several profitable outcomes for electric vehicles. This method could reduce the price, weight, and volume of electrochemical batteries used in automobiles if it proves to be productive and energy efficient (Mohamed A. A. S. et al., 2022; Mohamed N. et al., 2022).
This type of dynamic charging is one of the ways that EVs’ autonomy is expected to be increased without significantly raising battery capacity (Laporte et al., 2019; Mohamed et al., 2021). This view pertains to one of these methods, namely to the transfer of energy through induction. Fuel efficiency, traffic restrictions brought on by the requirement for integration in the car and on the road, and adherence to electromagnetic emission regulations are the key issues with this mode of energy transfer. The connection of the two coils affects the transfer’s energy efficiency (Mou et al., 2019).
Essentially, this charging system consists of a transmitter coil that is mounted on the road or in the parking lot and is coupled to an alternating current (AC) or direct current (DC) power supply (Zhang J. et al., 2023; Ju et al., 2022). The lack of these energy sources on a highway restricts the usefulness of this charging option. For this reason, one practical way to solve the problem of charging this device is to use alternate power sources (Manousakis et al., 2023).
Considerable advances in the field of EV management are presented in this article. It offers the best planning strategies for charging and discharging electric vehicles on a local and worldwide scale. The two planning approaches it proposes are focused on local optimization, while the other seeks a worldwide optimal. By using two separate approaches, one can respond to various operational restrictions and situations with flexibility in application. About the complexities of organizing the charging and discharging of electric vehicles, it develops an extensive optimization model. To efficiently control energy in EV systems, this model considers several variables, including demand response, energy costs, and operational limits. The proposed planning strategy aims to improve the efficiency of the electric vehicle charging infrastructure, which has the potential to reduce operating costs and improve grid stability. This is particularly important as the adoption of electric vehicles continues to increase and requires more sophisticated management techniques. The simulation results included in this document confirm the effectiveness of the proposed scheduling scheme. These results demonstrate the potential advantages in terms of cost savings and efficiency improvements compared to traditional planning methods.
The goal of this work is to find solutions for the problems brought about by the growing use of EVs in the electrical grid. The stability and security of the smart grid are significantly impacted by the growing number of electric vehicles and their charging requirements. To address these issues, this paper emphasizes the necessity of efficient management techniques for charging and unplugging electric vehicles.
Numerous advantages are highlighted in this paper, including:
• Electric vehicles are a global industry center point due to their clean and environmentally favorable attributes.
• The rising demand for EV charging creates uncertainty in terms of charge durations and energy needs, which can interfere with the smart grid’s regular operation.
• EVs can function as mobile energy storage units, offering the system a supplemental energy source when needed. A deliberate approach to their charging and discharging characteristics is necessary for this dual functionality.
The scope of the document establishes a planning model that integrates the grid, charging infrastructure, and EVs. The goal is to optimize charging and discharging operations based on real-time information and user preferences.
This model consists of three primary components:
• Real-time information on electricity supply and costs is provided by the electrical grid.
• Charging Equipment controls the delivery and purchasing of electricity for EVs. Considering that the framework in which EVs function permits them to charge or discharge by market rates and demand.
• There are three phases in the planning process: - Gathering data on vehicle conditions and electricity pricing. - Making decisions in real-time and utilizing gathered data to identify the best charge and discharge plans. - Order execution by sending the electricity required to finish the schedule.
The novelties in this article can be shown in Table 1 that compares them to earlier research:
Vehicle-to-grid (V2G) technology allows an EV to supply electricity to the grid by draining its battery (Khalid et al., 2021). The EV charging patterns can be optimally planned by an intelligent planning method to flatten the electric system’s demand profile efficiently. Both possible capital costs and operating expenses will be decreased as a result. Implementing a smart grid now requires intelligent EV charging and discharging planning (Aghajan-Eshkevari et al., 2022). The basic idea behind intelligent planning is to reshape the load profile by charging the EV battery from the grid if demands are low and discharging it to the grid when demands are high (Wang S. et al., 2023). Planning EV charging and discharging patterns optimally is difficult, though. First off, particularly when there is a big EV population, it can be challenging to identify the globally ideal planning strategy that can lower the overall cost of charging. The planning strategy must be able to manage random EV arrivals efficiently (Khalid and Peng, 2020; Feng et al., 2024a).
Recent research has offered several planning strategies for EV charging and discharging (Kraiem et al., 2024). However, the planning plans in (Zhang and Fan, 2020) only addressed battery charging in the absence of V2G functionality. While previous studies on V2G planning (Hadian et al., 2020; Ke et al., 2020) attempted to optimize the charging and discharging powers to minimize prices, their approaches which are essentially centralized algorithms may not be appropriate for EV charging and discharging systems that have a high population density and fluctuating arrivals (Lei et al., 2023).
Because of the use of several energy sources in this work, it is necessary to manage energy. Planning is necessary for the times when electric vehicles are charged and discharged (Amir et al., 2023; Ouyang et al., 2024). This paper presents a locally and globally optimal planning for EV charging and discharging. It summarizes the following contributions:
• The paper’s goal is to reduce the overall cost of charging every EV each day by formulating a problem of optimization for global planning. The kind of optimization issue that is amenable to an effective solution is convex optimization. By resolving a single global planning optimization issue, the globally optimal planning strategy finds the globally least total cost and, consequently, the ideal charging powers for all EVs for all intervals.
• This article suggests a local planning optimization challenge for the EV in the local group. It creates a locally optimal planning technique based on the local planning optimization issue, and it is carried out in a distributed and independent manner. For EV charging and discharging systems with a high population density and fluctuating arrivals, the locally optimum planning technique is highly suitable. The globally optimal planning scheme performs better than the locally optimal planning system, but it is extremely close to it.
The strategy with the lowest total cost is the globally optimal planning approach. However, the globally optimal planning strategy is not practicable as it necessitates the data on the base loads in the future, along with the EVs that will arrive later in the day, their arrival times, and their charging duration. The locally optimal scheduling scheme is an acceptable strategy that can effectively manage a large EV population and dynamic EV arrivals, even if it performs slightly worse than the globally optimal planning strategy. As a result, the paper’s final recommendation is the locally optimal scheduling method. Using the lowest overall total cost that the globally optimal planning approach provides, it is possible to calculate the optimality gap between the two strategies.
The rest of the article is structured as follows: in the second section, the battery charging techniques will be presented, in the third section the control structures of the EV charging and discharging system will be presented, in section four the charging system and its components will be described, in section five there is a problem description of the global planning optimization and its solution, and in the sixth section there is a detailed description of the problem of local planning optimization and its solution, then there is a discussion of the simulation to seventh section, finally, in the last section there is the conclusion.
2 Techniques and different methods for EV battery charging
Grid charging is necessary for two types of electric vehicles: plug-in hybrids (PHEV) and batteries electric vehicles (BEVs) (Zheng et al., 2019; Zhang et al., 2022a). BEVs solely use the electrical energy stored in the battery for propulsion, whereas PHEVs have the option of using fossil fuels. Batteries for electric vehicles can be charged using three different methods: conductive, inductive, and battery swap. This section outlines the three loading techniques. However, since conductive charging is the most popular way to charge batteries for electric vehicles, this article looks at the features and effects of this kind of charging from several angles.
2.1 Conductive charging
“Conductive charging” is the method of physically attaching an EV to the electrical grid to charge it. The two types of chargers that can be utilized for conductive charging of EVs are off-board and on-board chargers. An EV may be charged anywhere it is connected to an electrical outlet because it has an on-board charger installed, which eliminates the need for additional equipment to connect to the grid. However, because of the charger’s limited power transfer capability, charging an EV using this method takes longer. Off-board chargers, on the other hand, are usually located in fast-charging stations, commercial parking lots, and highways and are not part of the design of electric vehicles (Khalid et al., 2019). EVs receive more power from off-board chargers, which results in a quicker charging time.
A standard for varying EV charging levels has been established by the Society for Automatic Engineers (SAE) (Kongjeen and Bhumkittipich, 2018). Three charge levels are specified for each AC and DC charge in this standard. Table 2 provides a summary of these charge levels.
2.2 Inductive charging
The inductive charging technique, sometimes referred to as wireless charging, transfers electricity between an EV and the power grid via an electromagnetic field rather than a physical connection. Due to the power transmission through the air gap, one benefit of inductive charging is a decreased risk of electric shocks and related damages; however, the charging efficiency decreases in this scenario due to the relatively large air gap and non-compliance of the windings (Khalid et al., 2023). There are two methods to apply inductive charging: static and dynamic. Figures 1A shows the static wireless charging of the EV, this type of charging takes place when the electric vehicle is in position parked and the engine is turned off to fully charge. It is the usual charging method in public parking lots or homes. Some function Complex chargers can be integrated into these chargers to minimize misalignment coils. Figure 1B shows the dynamic wireless charging of the EV that allows an electric vehicle to charge wirelessly while driving on the road. In this case, some sections of the road are equipped with wireless power transmitters (WPT) and electronic equipment power to activate WPT for electric vehicles. This type of charging favors road-powered electric vehicles. Although the cost of infrastructure is high, the benefits of this infrastructure should be notable due to the limited number of charging stations offered on highways (Wang T. et al., 2023).
2.3 Change of battery
The battery of EVs is crucial to ensure its proper functioning and autonomy. According to manufacturers’ recommendations, the battery of EVs generally needs to be replaced every 5 years. Electric vehicle batteries, mainly composed of lithium-ion, store charged energy and power the electric motor while traveling. In the case of battery discharge, it can use the method of swapping your EV’s battery is a quick way to have it fully charged. The owner of an electric vehicle uses a battery-switching facility to replace the empty battery with a fully charged one (Ahmad et al., 2020). This technology helps the battery swapping station by managing the charging, draining, and battery switching, and it significantly reduces the time it takes for the owner of the EV to charge (Liu et al., 2023).
Table 3 presents a comparison of inductive charging, capacitive charging, and battery swapping for EVs.
Practically, the best method depends on the current state of development. Inductive charging is the most common option today. This method offers a charging system that does not require any mechanical contact, but capacitive charging is catching up, and the battery swapping requires specific stations.
3 The power system’s various EV charge and discharge control structures
As seen in Figure 2, aggregators are typically used to interchange electricity between EVs and the grid because each EV has a limited capacity. In contrast, EV charging and discharging processes can be directly or indirectly regulated by aggregators.
The control structure specifies three general approaches (centralized, decentralized, and hierarchical) that can be utilized to implement electric vehicle charging and discharging management in the power grid. A comparison of these three categories of approaches is presented below, looking at them from various angles.
3.1 Centralized control structure
EV owners lose control over the charging and discharging processes of their vehicles under a centralized control system, with aggregators managing and controlling EV behavior directly. Aggregators initially compile each EV data and charging specifications using this technique. Every time, The EV charge or discharge rate is determined by the aggregator based on predetermined variables and network conditions (Sobrinho et al., 2023).
3.2 Decentralized control structure
Owners of electric vehicles (EVs) can choose whether to charge or drain the battery according to their own needs, which are usually to save on charging expenses, as opposed to centralized control.
Therefore, the system operator or aggregator can move the charging load of EVs from peak to off-peak hours by employing pricing mechanisms and providing appropriate price incentives, so indirectly regulating the charging and discharging behavior of EVs (Shirkhani et al., 2023). The structure of decentralized control is presented in Figure 3.
3.3 Hierarchical control structure
In comparison, the hierarchical control structure has advantages over centralized and decentralized control systems concerning computing load and communication network requirements. Two levels usually make up hierarchical control. At the higher layer, all of the EV aggregators are scheduled by a central controller, like a Distribution System Operator (DSO). On the lowest layer, each aggregator is responsible for overseeing several EVs and scheduling when each EV needs to be charged and released. This control structure is hierarchical, as seen in Figure 4 (Nimalsiri et al., 2019).
A comparison of the centralized, decentralized, and hierarchical control systems is shown in Table 4.
The complexity of infrastructure necessitates the selection of a central control structure that ensures network stability, eliminates power fluctuations, and optimizes energy usage while enabling quick response to changes in network conditions.
4 Specification of the wireless charging system
4.1 General point of view
This work focuses on the dynamic wireless charging system using exhaustible energies such as (electricity grid, solar energy, wind energy, and piezoelectric energy). This charging system is made up of two coils; the first one is installed on the ground of the road which is called the transmitter coil, and the second one is installed under the vehicle which is called the receiver coil. Power converters are used to connect the many energy sources that this system can use to power the transmitter coil. The 50 KW of power generated by this coil powers the reception coil by producing a magnetic field that transforms magnetic energy into electrical energy. A power converter connects this coil to the battery of the electric car. A description of the charging system is shown in Figure 5:
This study emphasizes on a multi-lane highway, but one-way, with wireless charging in the rightmost lane. This road is divided into several sections, each measuring length L. Along this length, the highway is equipped with a power station that can generate energy, each power station being placed in a section to recharge electric vehicles. It is also equipped with charging coils that have a length of 1.2 m and a space between them equal to 0.8 m. Each 50 m section generates a maximum power transfer rate of 100 KW that can power two electric vehicles (Kong and Devetsikiotis, 2016). Figure 6 shows the infrastructure model of wireless charging in highways.
4.2 Mathematical model
After the last presentation of the dimensioning of the highway, and that of the spacing between the charging coils as well as the spacing between the electric vehicle to allow it to recharge properly.
The current problem is solved by the proposed mathematical model. This content includes an explanation of the data, a list of assumptions made before running the mathematical model, definitions of the notations, and finally the formulation.
4.2.1 Hypotheses
Several hypotheses were made to solve the problem of this study, the following hypotheses can be mentioned:
• All drivers must use the same speed.
• Electric vehicle ranges are continuous.
• The system for charging electricity is unlimited and uninterrupted.
• No queues form in front of the charging areas.
• Don’t stop at charging coils.
4.2.2 Parameters
Sections S of highways S = {1, 2, 3, . . ., n},
Nc: maximum number of recharge coils that can be placed on highway section S,
DS: charging demand on highway section S,
XS = {1, if the highway section S is selected to implement the charging coils for electric vehicles; 0, else},
NVES: Number of EVs on highway section S,
UDS: Number of unsatisfied charging demands on highway section S,
NDS: Number of updated demands on highway section S.
4.2.3 Formulation
➢ Constraints
The constraint Equation 1 stops the model from choosing a site as a charging area devoid of charging power. This constraint guarantees that this choice variable XS if the charges are present, it accepts the value 1. However, it also catalogs and archives the selected sites.
Constraints Equations 2, 3 deal with updated procedures and demand limits. These constraints specify unsatisfied charge demands and the values that these constraints provide as outputs are stored as findings in a database. When the entire demand for the last segment of the highway is not satisfied, constraint Equation 4 modifies the demand.
The objective of these constraints is to manage energy to ensure reliable and optimal production, thus minimizing the cost of recharging. A charging plan must be created in this situation so that the battery can be charged if it is discharged and discharged to the grid if it is charged (Zhang X. et al., 2023), (Duan et al., 2023).
5 Global planning optimization: principle and flowchart description
Based on a real-time pricing model, we develop a global planning optimization for EV charging and discharging in this part. The optimization problem’s solution offers a globally optimal planning plan that lowers the total cost (Feng et al., 2024b).
5.1 Global planning optimization model
This paper examines the equal division of daily EV battery charging and discharging into a series of intervals. O indicates the interval set. The length of an interval is given by δ. It is predicated on the idea that power usage for charging and discharging can be adjusted over time.
This article divides the day into 24 equal-length segments, each lasting 1 h. Every electric vehicle, that charges and discharges during the day (Li et al., 2021), are noted by N. The EV N set consists of two sets; set NCH: charging only the EV, which can charge EV batteries but does not feed energy from the batteries into the grid and sets NV2G: the set of charging vehicles to grid, which includes EV that can charge their battery, and both can discharge the battery (Zhang et al., 2022b). We have N = NCH + NV2G.
Since the EVs in the charging-only set NCH never drain their batteries, they constantly meet
Conversely, the EVs in the V2G set NV2G may have a positive, zero, or negative charging power
Since the time is divided into several intervals, as Figure 7 illustrates, we define the Tn load period of EV n as the collection of intervals between arrival time
The battery capacity of EV n is noted
In this scenario, the controller locally can automatically determine the beginning energy, battery capacity, and EV arrival time when the vehicle is attached to the charging coil.
The final energy report and departure time of the EV n are given by the EV n user before the load starts. From the
The planning of EV charging and discharging in a limited geographic area is the subject of this research. Our real-time pricing methodology operates under two suppositions: Two things are true: 1- there is no transmission congestion, and 2- the losses between nodes are tiny and hence insignificant. For these two reasons, it is vital to overlook the regional variations in electricity prices. Regardless of where the charging is taking place, the cost of electricity is constant in real-time. The EV charging optimizations found in (Ma et al., 2011) are solely dependent on the price’s temporal volatility, not its spatial variation. According to (Ma et al., 2011), the instant load is the basis for modeling the price of electricity and is represented as follows Equation 5:
When
The two elements that together comprise the overall load in interval j: are the charging load
The charging load
Total load
This study defines Cj, the charging cost, as the total amount of money customers spend charging and discharging their electric vehicles at a given interval of j. The pricing model provides
The charging cost Cj can be positive or negative, as Equation 7 illustrates. The charging cost Cj is positive if the charging load, denoted by
5.2 Problem formulation and solution
The following assumptions determine a globally optimal planning strategy for EVs that are used for daytime charging and discharging: The EV set N comprises of the following: known arrival and departure times for each EV, known battery initial and final energies, known base load for each day’s interval, and scheduling optimization carried out by a central controller that compiles and analyzes all the data.
The total cost is the sum of charging expenses across O intervals. The total cost is then determined by:
To reduce the total cost of the EVs that execute charging and discharging during the day, the global planning optimization problem might be defined as total load
The (Equation 8a) is a function that minimizes the total cost of charging and discharging EVs during the day. The link between the charging power of a single EV and the charging load in an interval is shown by (Equation 8b):
Equation 8c presents the instant energy constraints and the final energy constraints, respectively:
Where
(Equation 8d) defines load power constraints, where the first equation has the lower limit 0 and the upper limit
For EV charging and discharging during the day, the globally optimal planning strategy is provided by the solution to the optimization issue (Equation 8).
5.3 Related flowchart
The exposed flowchart is related to the global planning optimization protocol. Seven steps resume the function of the process. In the first step, it is important to know the necessary information on EVs, as the state of charge, the desired recharge time and the vehicle number then it is important to know the integrated constraints, such as the charging demand; if it is satisfied (
6 Local planning optimization: principle and flowchart description
6.1 Local planning optimization model
The planning strategy that is globally optimal has the lowest total cost. But the globally optimal planning approach is not practical for the reasons listed below: it is not scalable for a centralized planning strategy where a high number of EVs may overload the central controller, it is currently uncertain how many EVs will come at a future time of day, the base load at a future time of day is also unknown. In this section, we propose a local planning optimization problem, which relaxes the assumptions from the global planning optimization problem (Equation 8).
This part develops a local planning optimization problem, which loosens the assumptions of the global planning optimization problem (Equation 8). A locally optimal planning strategy that can perform comparably to the globally optimal planning strategy is a solution to the local planning optimization challenge. Compared to the globally optimal planning method, the locally optimal planning system is more practical and scalable.
6.2 Problem formulation and solution
The globally optimal planning strategy assumes that we have a global awareness of the data regarding EVs and the base load during the day, thus the ideal charging powers at each interval can be found by solving the global planning optimization problem (Equation 8) only once. In the locally optimal planning technique, there is no information about the future demand for electric vehicles. We present a locally optimal planning technique to find the optimal charging powers for the local EVs in the next period using a sliding window mechanism. We optimize based on groups in the locally optimal planning technique. An EV group consists of all the EVs in one or more adjacent locations. For example, there are distinct categories of electric vehicles based on whether they are charged and discharged in a private garage or a parking lot. There is a local controller for each group. Communication links connect the utility company’s central controller and the charging coils to the local controller. The local controller receives the anticipated loads for the day from the central controller. EV data is collected through real-time communication between the local controller and every charging zone. Using this information, the local controller then optimizes planning and provides each local EV with instructions on the most efficient way to charge or discharge the battery.
The group set is denoted as D. Since planning is done independently by each local controller, we will only be examining group
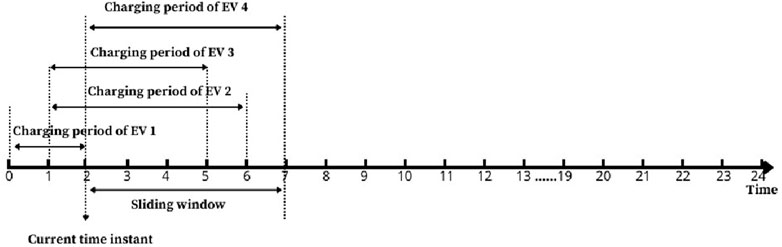
Figure 9. An example of the sliding window and the ongoing EV set in the locally optimal planning strategy.
We can forecast the base loads in the sliding window
We design the local planning optimization problem for the current instant in group u based on the current ongoing EV set
The Equation 9a minimizes the total cost of EVs in the current ongoing EV group
Equation 9c presents the formulas of the energies needed to load the EVs, such that the first equation shows the initial energy in the interval j, and the other equation shows the final energy:
Where:
Equation 9d defines load power constraints, where the first equation has the lower limit 0 and the upper limit
The local planning optimization problem (Equation 9) at the start of interval j is convex and can be easily solved using interior point methods (Simonetto et al., 2020). The optimal charging powers
6.3 Related flowchart
The exposed flowchart is related to the local planning optimization protocol. Eight steps resume the function of the process. In the first step, it is important to know the necessary information on EVs, as the state of charge, the desired recharge time, and the vehicle number then it is important to know the integrated constraints, such as the charging demand; if it is satisfied (
7 Simulation and results
We studied the charging and discharging of electric vehicles for a day. Considering that a day is divided into 24 intervals, and each interval represents 1 h. The base load at each interval is simulated using actual load data in Stuttgart in December 2023, and the electricity cost is defined in €/KWh. The battery settings of the electric vehicle are based on the characteristics of the BMW i3. The battery capacity is 37.9 kWh with an electric range of up to 308 km. We assume the same characteristics for each electric vehicle. The battery energy must reach at least 90% of its capacity at the end of the charging period. The maximum charging power for all-electric vehicles is 50 KW. Considering the total number of electric vehicles is set by default to 100. The arrival times of electric vehicles are distributed evenly throughout the day and the percentage of vehicles arriving at a given time is less than 20%. Charging periods for electric vehicles are evenly distributed between 6 and 18 h. The initial energy levels of electric vehicles are evenly distributed between 20% and 80% of the battery capacity.
The simulation conditions are summarized in Table 5.
In Figure 11, the charging and discharging of VE all over a day are examined by contrasting the actual base load with the forecasted base load. In this case, the real base load is determined by scaling charge in Stuttgart in December 2023. The average charge for the 8 days in Stuttgart for the week of December 7–15 is the forecast base load.
We are contrasting three planning strategies: equal allocation, locally optimal, and globally optimal, which distribute an electric vehicle’s charging power over a given time interval according to the following standards: 2) The absolute value of the charging power remains constant during each interval, and 1) the charging or discharging of EVs within a period is depending on the price of electricity for the previous day. Here are the simulation parameters that are used for the comparison: There are 100 EVs in total, and they are all capable of charging and discharging. There are two groups of 50 EVs each, based on the overall number of EVs. The overall expenses for each of the three options are determined using actual baseline prices to ensure a fair comparison. Figure 12 shows the change in charging and total load for each of the three techniques in each interval. As demonstrated in Figure 12A, to achieve lower overall costs, both the locally and globally optimal planning strategies charge the battery from the grid during times of lower demand and discharge it to the grid during times of higher demand. To minimize the overall cost, the globally optimal planning technique flattens the whole load profile in intervals 1-7, 8-9, 10, 11, and 12-24, as shown in Figure 12B. The globally optimal planning approach solves a single global planning optimization issue to find the best charging powers for every EV in every interval, resulting in the lowest possible total cost on a global scale. The locally optimal planning approach solves the local planning optimization problem to find the best charging powers for a set of EVs inside the interval.
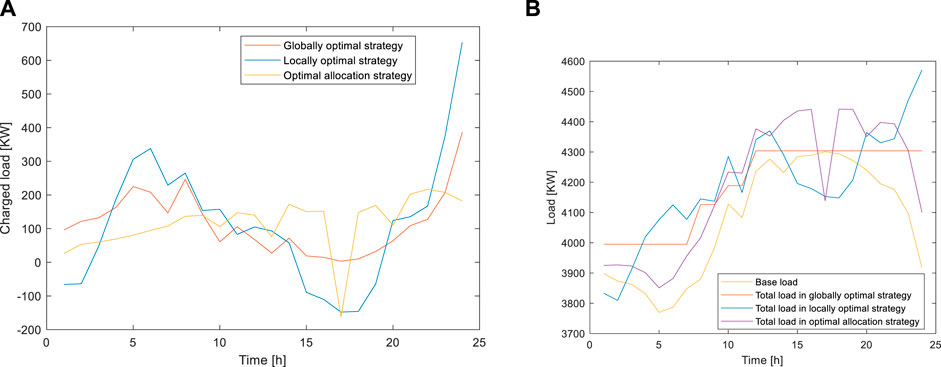
Figure 12. Variation of charging load and total load in each interval: (A) Charging load, (B) Total load.
The power variation of five electric vehicles within each interval is shown in Figure 13A. We may analyze the charging power planning of an EV (EV 20, for example) at random using this graphic. For the EV 20, the charging time spans from interval 15 to interval 24. The allocation technique uses intervals 16 to 24 to charge the EV 20 battery after it has been discharged in period 15. The variation of five EVs during each period is shown in Figure 13B. From this figure, it is observed that all three strategies enable EV 20 to reach the same final energy level.
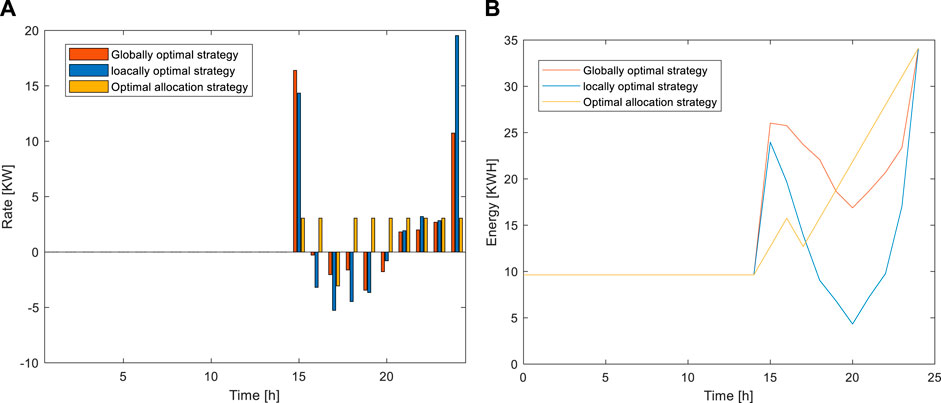
Figure 13. Variation of charging power and energy of EV 5 in each interval: (A) Charging power, (B) Energy.
Before charging, every EV has the option to discharge its battery to the grid. As such, each EV is classified as either the charging-only set NCH or the V2G set NV2G. The charging-only ratio is the ratio of EVs in the charging-only set NCH to total EVs. Figure 14 shows how the charging-only ratio affects the total cost. Figure 14 illustrates how a higher charging-only ratio leads to more EVs in the charging-only set NCH and fewer EVs in the V2G set NV2G. This increases the three strategies’ total cost.
The locally optimal planning technique is employed by the local controller to distribute and plan the EVs in the local group in an autonomous method. We specify the group size as the total number of EVs. Figure 15 shows the average group sizes for the group. Evaluate each member’s performance. A fixed 100 EVs are present altogether. When the average group size is bigger, there are consequently fewer groups. To determine the ideal charging powers for a set of EVs for interval
8 Conclusion
In this work, we presented the EV battery charging techniques in the electrical system, and the different control structures for charging and discharging of electric vehicles in the electrical system. Then we presented the wireless charging system using inexhaustible energies, the use of several energy sources lead us to look for a solution to manage energy and minimize the total cost of charging. Next, we examine the planning optimization problem for EV charging and discharging. Initially, we propose an optimization problem for global planning where the charging powers are adjusted to reduce the total cost of all EVs that are used during charging and discharging. The globally minimum total cost is provided by the globally optimal solution. However, because it relies on the assumption that all EV arrivals and base load arrivals during the day are known in advance, the globally optimal planning strategy is not feasible. We propose a local planning optimization problem, which attempts to reduce the overall cost of the EVs in the present continuing EV set in the local group, to develop a practical planning strategy. The locally optimal planning system is robust to dynamic EV arrivals and operates in a large EV population. So, this study introduces optimization algorithms that enhance the efficiency of charging and discharging operations. These algorithms are designed to minimize costs and maximize the utility of EVs as mobile energy storage devices, thus contributing to grid stability. And it highlights the significant role of EVs in supporting grid management. By optimizing their charging and discharging schedules, EVs can help alleviate peak demand periods, and stabilize the grid. The results of the simulation showed that, in comparison to the globally optimal planning strategy, the locally optimal planning strategy can attain a close performance. Finally, this study concludes with recommendations for future research, including the exploration of advanced machine learning techniques to further enhance the predictive capabilities of the scheduling model and the integration of more complex grid dynamics.
9 Future endeavors for this work
As an extension of this work, it can be mentioned that it is possible to Incorporate more realistic constraints: The current models could be extended to consider additional real-world constraints, such as battery degradation, user preferences, and grid-level impacts.
Also, it is possible to Explore more advanced optimization techniques, and more sophisticated optimization methods, such as deep reinforcement learning, to handle the complexity of EV scheduling problems.
Extending to large-scale, multi-agent scenarios is also possible. The proposed schemes could be scaled up to handle scenarios with many EVs and charging stations, potentially involving multiple stakeholders and grid operators.
Validating of the models through real-world demonstrations can be also an interesting future work. Implementing and testing the proposed scheduling schemes in real-world pilot projects would help validate the practical applicability and benefits of the approaches.
By addressing these future endeavors, the research on optimal EV scheduling can continue to evolve and provide more comprehensive solutions to the challenges faced in the integration of electric vehicles into the power grid.
Data availability statement
The original contributions presented in the study are included in the article/supplementary material, further inquiries can be directed to the corresponding author.
Author contributions
AB: Investigation, Writing–original draft, Formal Analysis, Supervision, Validation. AF: Investigation, Writing–original draft, Methodology, Project administration, Writing–review and editing. AA: Conceptualization, Data curation, Validation, Writing–original draft. RU: Conceptualization, Data curation, Project administration, Writing–original draft. CE-B: Conceptualization, Funding acquisition, Project administration, Writing–original draft.
Funding
The author(s) declare that no financial support was received for the research, authorship, and/or publication of this article.
Acknowledgments
authors declare that this work is under Researchers Supporting Project number (RSP2024R258), King Saud University, Riyadh, Saudi Arabia.
Conflict of interest
The authors declare that the research was conducted in the absence of any commercial or financial relationships that could be construed as a potential conflict of interest.
Publisher’s note
All claims expressed in this article are solely those of the authors and do not necessarily represent those of their affiliated organizations, or those of the publisher, the editors and the reviewers. Any product that may be evaluated in this article, or claim that may be made by its manufacturer, is not guaranteed or endorsed by the publisher.
References
Aghajan-Eshkevari, S., Azad, S., Nazari-Heris, M., Ameli, M. T., and Asadi, S. (2022). Charging and discharging of electric vehicles in power systems: an updated and detailed review of methods, control structures, objectives, and optimization methodologies. Sustainability 14 (4), 2137. doi:10.3390/su14042137
Ahmad, F., Saad Alam, M., Saad Alsaidan, I., and Shariff, S. M. (2020). Battery swapping station for electric vehicles: opportunities and challenges. IET Smart Grid 3 (3), 280–286. doi:10.1049/iet-stg.2019.0059
Amir, M., Deshmukh, R. G., Khalid, H. M., Said, Z., Raza, A., Muyeen, S., et al. (2023). Energy storage technologies: an integrated survey of developments, global economical/environmental effects, optimal scheduling model, and sustainable adaption policies. J. Energy Storage 72, 108694. doi:10.1016/j.est.2023.108694
Chow, J. H., Wu, F. F., and Momoh, J. A. (2005). Applied mathematics for restructured electric power systems. Springer. doi:10.1007/0-387-23471-3_1
Das, P., and Kayal, P. (2024). An advantageous charging/discharging scheduling of electric vehicles in a PV energy enhanced power distribution grid. Green Energy Intell. Transp. 3 (2), 100170. doi:10.1016/j.geits.2024.100170
Duan, Y., Zhao, Y., and Hu, J. (2023). An initialization-free distributed algorithm for dynamic economic dispatch problems in microgrid: modeling, optimization and analysis. Sustain. Energy, Grids Netw. 34, 101004. doi:10.1016/j.segan.2023.101004
El-Bayeh, C. Z., Alzaareer, K., Aldaoudeyeh, A.-M. I., Brahmi, B., and Zellagui, M. (2021). Charging and discharging strategies of electric vehicles: a survey. World Electr. Veh. J. 12 (1), 11. doi:10.3390/wevj12010011
Feng, J., Wang, Y., and Liu, Z. (2024b). Joint impact of service efficiency and salvage value on the manufacturer’s shared vehicle-type strategies. RAIRO-Oper. Res. 58, 2261–2287. doi:10.1051/ro/2024082
Feng, J., Yao, Y., Liu, Z., and Liu, Z. (2024a). Electric vehicle charging stations’ installing strategies: considering government subsidies. Appl. Energy 370, 123552. doi:10.1016/j.apenergy.2024.123552
Hadian, E., Akbari, H., Farzinfar, M., and Saeed, S. (2020). Optimal allocation of electric vehicle charging stations with adopted smart charging/discharging schedule. IEEE Access 8, 196908–196919. doi:10.1109/access.2020.3033662
Ju, Y., Liu, W., Zhang, Z., and Zhang, R. (2022). Distributed three-phase power flow for AC/DC hybrid networked microgrids considering converter limiting constraints. IEEE Trans. Smart Grid 13 (3), 1691–1708. doi:10.1109/tsg.2022.3140212
Ke, B.-R., Lin, Y.-H., Chen, H.-Z., and Fang, S.-C. (2020). Battery charging and discharging scheduling with demand response for an electric bus public transportation system. Sustain. Energy Technol. Assessments 40, 100741. doi:10.1016/j.seta.2020.100741
Khalid, H., Mekhilef, S., Mubin, M., and Seyedmahmoudian, M. (2023). Advancements in inductive power transfer: overcoming challenges and enhancements for static and dynamic electric vehicle applications. Energy Rep. 10, 3427–3452. doi:10.1016/j.egyr.2023.10.008
Khalid, H. M., Flitti, F., Muyeen, S. M., Elmoursi, M. S., Tha’er, O. S., and Yu, X. (2021). Parameter estimation of vehicle batteries in V2G systems: an exogenous function-based approach. IEEE Trans. Ind. Electron. 69 (9), 9535–9546. doi:10.1109/tie.2021.3112980
Khalid, H. M., and Peng, J. C.-H. (2020). Bidirectional charging in V2G systems: an in-cell variation analysis of vehicle batteries. IEEE Syst. J. 14 (3), 3665–3675. doi:10.1109/jsyst.2019.2958967
Khalid, M. R., Alam, M. S., Sarwar, A., and Asghar, M. S. J. (2019). A Comprehensive review on electric vehicles charging infrastructures and their impacts on power-quality of the utility grid. ETransportation 1, 100006. doi:10.1016/j.etran.2019.100006
Kong, C., and Devetsikiotis, M. (2016). “Optimal charging framework for electric vehicles on the wireless charging highway,” in 2016 IEEE 21st international workshop on computer aided modelling and design of communication links and networks (CAMAD), 89–94.
Kongjeen, Y., and Bhumkittipich, K. (2018). Impact of plug-in electric vehicles integrated into power distribution system based on voltage-dependent power flow analysis. Energies 11 (6), 1571. doi:10.3390/en11061571
Kraiem, H., Gadri, W., and Flah, A. (2024). Efficient energy management with emphasis on EV charging/discharging strategy. Eng. Technol. \and Appl. Sci. Res. 14 (2), 13143–13147. doi:10.48084/etasr.6807
Laporte, S., Coquery, G., Deniau, V., De Bernardinis, A., and Hautiere, N. (2019). Dynamic wireless power transfer charging infrastructure for future evs: from experimental track to real circulated roads demonstrations. World Electr. Veh. J. 10 (4), 84. doi:10.3390/wevj10040084
Lei, Y., Yanrong, C., Hai, T., Ren, G., and Wenhuan, W. (2023). DGNet: an adaptive lightweight defect detection model for new energy vehicle battery current collector. IEEE Sens. J. 23, 29815–29830. doi:10.1109/jsen.2023.3324441
Li, P., Hu, J., Qiu, L., Zhao, Y., and Ghosh, B. K. (2021). A distributed economic dispatch strategy for power--water networks. IEEE Trans. Control Netw. Syst. 9 (1), 356–366. doi:10.1109/tcns.2021.3104103
Liang, J., Feng, J., Fang, Z., Lu, Y., Yin, G., Mao, X., et al. (2023). An energy-oriented torque-vector control framework for distributed drive electric vehicles. IEEE Trans. Transp. Electrif. 9, 4014–4031. doi:10.1109/tte.2022.3231933
Liu, Z., Wu, Y., and Feng, J. (2023). Competition between battery switching and charging in electric vehicle: considering anticipated regret. Environ. Dev. Sustain. 26, 11957–11978. doi:10.1007/s10668-023-03592-4
Ma, Z., Callaway, D. S., and Hiskens, I. A. (2011). Decentralized charging control of large populations of plug-in electric vehicles. IEEE Trans. Control Syst. Technol. 21 (1), 67–78. doi:10.1109/tcst.2011.2174059
Manousakis, N. M., Karagiannopoulos, P. S., Tsekouras, G. J., and Kanellos, F. D. (2023). Integration of renewable energy and electric vehicles in power systems: a review. Processes 11 (5), 1544. doi:10.3390/pr11051544
Manzolli, J. A., Trovão, J. P. F., and Antunes, C. H. (2022). Electric bus coordinated charging strategy considering V2G and battery degradation. Energy 254, 124252. doi:10.1016/j.energy.2022.124252
Mohamed, A. A. S., Shaier, A. A., Metwally, H., and Selem, S. I. (2022a). An overview of dynamic inductive charging for electric vehicles. Energies 15 (15), 5613. doi:10.3390/en15155613
Mohamed, N., Aymen, F., Issam, Z., Bajaj, M., Ghoneim, S. S. M., and Ahmed, M. (2021). The impact of coil position and number on wireless system performance for electric vehicle recharging. Sensors 21 (13), 4343. doi:10.3390/s21134343
Mohamed, N., Proceeds, E., Boukhchana, A., Lassaad, S., and Aymen, F. (2022b). Sizing of a dynamic wireless power transfer system for electric vehicles in highway. IEEE International Conference on Electrical Sciences and Technologies in Maghreb (CISTEM). doi:10.1109/CISTEM55808.2022.10043868
Mohith, B., Manoj, V., and Nithin, K. (2023). Exploring charging-dispatch approaches and vehicle-to-grid technologies for electric vehicles in distribution networks. IJSREM. doi:10.55041/IJSREM27309
Mou, X., Zhang, Y., Jiang, J., and Sun, H. (2019). Achieving low carbon emission for dynamically charging electric vehicles through renewable energy integration. IEEE Access 7, 118876–118888. doi:10.1109/access.2019.2936935
Nimalsiri, N. I., Mediwaththe, C. P., Ratnam, E. L., Shaw, M., Smith, D. B., and Halgamuge, S. K. (2019). A survey of algorithms for distributed charging control of electric vehicles in smart grid. IEEE Trans. Intell. Transp. Syst. 21 (11), 4497–4515. doi:10.1109/tits.2019.2943620
Ouyang, D., Liu, B., Huang, J., and Wang, Z. (2024). Degradation and safety performance of lithium-ion cells under high-rate charging/discharging scenarios. Process Saf. Environ. Prot. 185, 76–85. doi:10.1016/j.psep.2024.03.064
Ozkan, H. A., and Erol-Kantarci, M. (2022). A novel Electric Vehicle Charging/Discharging Scheme with incentivization and complementary energy sources. J. Energy Storage 51, 104493. doi:10.1016/j.est.2022.104493
Shirkhani, M., Tavoosi, J., Danyali, S., Sarvenoee, A. K., Abdali, A., Mohammadzadeh, A., et al. (2023). A review on microgrid decentralized energy/voltage control structures and methods. Energy Rep. 10, 368–380. doi:10.1016/j.egyr.2023.06.022
Simonetto, A., Dall’Anese, E., Paternain, S., Leus, G., and Giannakis, G. B. (2020). Time-varying convex optimization: time-structured algorithms and applications. Proc. IEEE 108 (11), 2032–2048. doi:10.1109/jproc.2020.3003156
Sobrinho, D. M., Almada, J. B., Tofoli, F. L., Leão, R. P. S., and Sampaio, R. F. (2023). Distributed control based on the consensus algorithm for the efficient charging of electric vehicles. Electr. Power Syst. Res. 218, 109231. doi:10.1016/j.epsr.2023.109231
Wang, S., Lu, T., Hao, R., Li, J., Guo, Y., He, X., et al. (2023a). An identification method for anomaly types of active distribution network based on data mining. IEEE Trans. Power Syst. 39, 5548–5560. doi:10.1109/tpwrs.2023.3288043
Wang, T., Zhang, J., and He, J. (2023b). Dynamic wireless charging lane reversal for connected and automated electric vehicles in highway. Sustain. Energy Technol. Assessments 57, 103206. doi:10.1016/j.seta.2023.103206
Xu, X., Liu, W., and Yu, L. (2022). Trajectory prediction for heterogeneous traffic-agents using knowledge correction data-driven model. Inf. Sci. (Ny) 608, 375–391. doi:10.1016/j.ins.2022.06.073
Yilmaz, M., and Krein, P. T. (2012). Review of battery charger topologies, charging power levels, and infrastructure for plug-in electric and hybrid vehicles. IEEE Trans. Power Electron. 28 (5), 2151–2169. doi:10.1109/tpel.2012.2212917
Yin, H., Alsabbagh, A., and Ma, C. (2021). A decentralized power dispatch strategy in an electric vehicle charging station. IET Electr. Syst. Transp. 11 (1), 25–35. doi:10.1049/els2.12002
Zhang, J., Li, H., Kong, X., Zhou, J., Shi, G., Zang, J., et al. (2023b). A novel multiple-medium-AC-port power electronic transformer. IEEE Trans. Ind. Electron. 71, 6568–6578. doi:10.1109/tie.2023.3301550
Zhang, L., Yin, Q., Zhu, W., Lyu, L., Jiang, L., Hai Koh, L., et al. (2023a). Research on the orderly charging and discharging mechanism of electric vehicles considering travel characteristics and carbon quota. IEEE Trans. Transp. Electrif. doi:10.1109/TTE.2023.3296964
Zhang, M., and Fan, X. (2020). Review on the state of charge estimation methods for electric vehicle battery. World Electr. Veh. J. 11 (1), 23. doi:10.3390/wevj11010023
Zhang, X., Gong, L., Zhao, X., Li, R., Yang, L., and Wang, B. (2023c). Voltage and frequency stabilization control strategy of virtual synchronous generator based on small signal model. Energy Rep. 9, 583–590. doi:10.1016/j.egyr.2023.03.071
Zhang, X., Wang, Y., Yuan, X., Shen, Y., and Lu, Z. (2022a). Adaptive dynamic surface control with disturbance observers for battery/supercapacitor-based hybrid energy sources in electric vehicles. IEEE Trans. Transp. Electrif. 9 (4), 5165–5181. doi:10.1109/tte.2022.3194034
Zhang, X., Wang, Z., and Lu, Z. (2022b). Multi-objective load dispatch for microgrid with electric vehicles using modified gravitational search and particle swarm optimization algorithm. Appl. Energy 306, 118018. doi:10.1016/j.apenergy.2021.118018
Zheng, Y., Niu, S., Shang, Y., Shao, Z., and Jian, L. (2019). Integrating plug-in electric vehicles into power grids: a comprehensive review on power interaction mode, scheduling methodology and mathematical foundation. Renew. Sustain. Energy Rev. 112, 424–439. doi:10.1016/j.rser.2019.05.059
Nomenclature
Keywords: electric vehicle, dynamic wireless charging, inductive power transfer, optimal planning, charging and discharging smart grid, vehicle-to-grid (V2G), vehicle battery, state of charge (SOC)
Citation: Boukhchana A, Flah A, Alkuhayli A, Ullah R and El-Bayeh CZ (2024) Optimal planning strategy for charging and discharging an electric vehicle connected to the grid through wireless recharger. Front. Energy Res. 12:1453711. doi: 10.3389/fenrg.2024.1453711
Received: 23 June 2024; Accepted: 20 August 2024;
Published: 05 September 2024.
Edited by:
Arsalan Najafi, Wrocław University of Science and Technology, PolandReviewed by:
Nikolaos Manousakis, University of West Attica, GreeceHaris M. Khalid, University of Dubai, United Arab Emirates
Copyright © 2024 Boukhchana, Flah, Alkuhayli, Ullah and El-Bayeh. This is an open-access article distributed under the terms of the Creative Commons Attribution License (CC BY). The use, distribution or reproduction in other forums is permitted, provided the original author(s) and the copyright owner(s) are credited and that the original publication in this journal is cited, in accordance with accepted academic practice. No use, distribution or reproduction is permitted which does not comply with these terms.
*Correspondence: Rahmat Ullah, dWxsYWhyMUBjYXJkaWZmLmFjLnVr