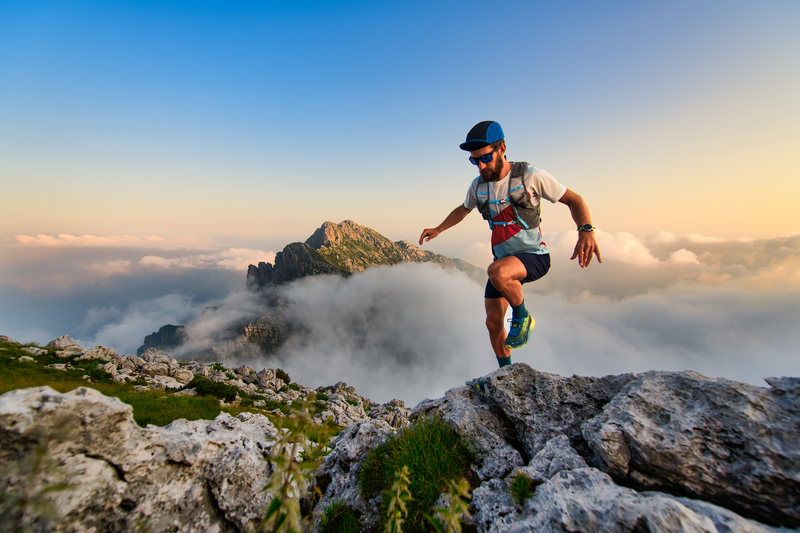
95% of researchers rate our articles as excellent or good
Learn more about the work of our research integrity team to safeguard the quality of each article we publish.
Find out more
EDITORIAL article
Front. Energy Res. , 21 August 2024
Sec. Sustainable Energy Systems
Volume 12 - 2024 | https://doi.org/10.3389/fenrg.2024.1444585
This article is part of the Research Topic Smart Energy System for Carbon Reduction and Energy Saving: Planning, Operation and Equipments View all 42 articles
Editorial on the Research Topic
Smart energy system for carbon reduction and energy saving: planning, operation and equipments
Energy conservation and emission reduction techniques are the best ways to achieve sustainable development and energy usage given the dire circumstances of resource restrictions, ecological deterioration, and environmental damage. Achieving energy conservation and emission reduction strategies as well as guaranteeing sustainable development are inextricably linked to the implementation of the “smart energy” plan. Nonetheless, the smart energy system places greater emphasis on the coupling relationship between various energy systems than does a single energy system. High coupling enables the smart energy system to satisfy complex energy demands, but it also presents new difficulties for managing power, maximizing operating and maintenance expenses, and figuring out functioning status. Thus, it is anticipated that research into cutting-edge machinery and technology would enhance the sustainability and economy of smart energy systems, which is crucial for the effective use of energy.
Using the complementary qualities of each energy resource in time or space, this Research Topic aims to fully integrate and mobilize various energy resources, such as electric energy, heat energy, and natural gas, between regions, giving full play to each energy resource’s advantages to meet the region’s energy use demand.
The objective is to apply Internet-savvy thinking and cunning methods to change the conventional single energy supply scenario. By creating a novel energy system with vertical “source-network-load-storage” coordination, horizontal multi-source complementarity, and high integration of energy and information to reduce carbon emissions and improve energy efficiency.
The Research Topic currently includes 41 papers. Eight papers pertain to distribution network and grid planning, six papers focus on energy storage and control strategies, five papers delve into renewable energy integration and prediction, six papers explore carbon emission and environmental impacts, while 16 papers center on technological innovation and optimization. All contributions to this Research Topic focus on one or more of the research areas listed in this Research Topic.
In order to address the escalating energy and environmental issues, a macro perspective on the evolution of diverse electrical systems is required to enhance overall energy efficiency and environmental sustainability. JL et al. offer a distribution network planning methodology that incorporates multi-agent game theory and the distribution system operator (DSO) in order to address this issue. This method recognizes how supply and demand dynamics in the power market impact market prices, which are dynamic in nature. Grid planning and distributed generation (DG) operation are first included as decision variables in decision models for distribution network companies and electricity users. The DSO is then given a game-theoretic structure that includes a distribution locational marginal pricing (DLMP)-based price accounting model. The gaming habits and patterns of contact between distribution companies, power users, and the DSO are then analyzed. Finally, an iterative search approach is used to solve the multi-agent game planning model for distribution networks that consider price signals in the power market (Li et al., 2023a).
JL et al. have made another contribution that offers a comprehensive planning method that considers the policy imperatives for a wide-area Integrated Energy System (IES) as well as the comprehensive energy consumption behavior (CECB) of consumers. Originally, a decision-making model for changing the energy supply for regional users is developed based on the analysis of CECB. Next, a game-theoretic model enables cooperative design of an integrated natural gas and electrical energy system that takes into account the various profit models of market players. An evaluation model that considers both supply and demand side pollution emissions supports the planning process that is directed by the government. Additionally, to take into consideration the interactions between users, the government, the IES, and other market organizations, a two-level dynamic game planning model is constructed. The wide-area IES optimal solutions are obtained from the iterative solution of this model (Li et al., 2023b).
To manage fluctuation in active loads, MH et al. propose a two-phase resilient planning process for storage of energy in distribution infrastructure. First, a Bi-LSTM model with mutual information techniques is created via clustering techniques for short-term load forecasting. The findings of load forecasting are then used to create a full norm-constrained uncertainty set, which serves as the foundation for a two-phase resilient planning process for storage of energy in distribution infrastructure (Yuan et al., 2024).
JL et al. provide a two-phase static resilient distribution network development technique that considers autocorrelation issues in order to address the challenges brought on by load uncertainties in distribution network design. The Spearman rank association coefficient is used to quantify the correlation, while independent transformation and Cholesky decomposition are used to convert the correlated random variables into independent ones. The suggested approach uses Benders decomposition to express source-load uncertainty and treats the design of active distribution networks as a fluid mutually exclusive game. Mathematical modeling validation shows how accurate and effective the process is (Song and Zhang, 2021).
A multi-agent planning technique for gas and electricity systems is proposed by WL et al. taking into account consumers’ overall energy consumption behavior. An evolutionary game is used to analyze overall energy consumption behavior, and a utility model for consumers’ energy consumption characteristics is developed using both objective and subjective weighting. The findings provide guidance for creating income models that investors in gas and electricity networks can use. To account for a wide range of energy consumption patterns, a dynamic game model of multi-agent electricity-gas planning is solved iteratively (Liu Wentao et al., 2024).
To lessen power congestion in networks and increase trading channel utilization, CW et al. offer a technique for determining trading channel available transfer capabilities. They use a histogram-based gradient tree boosting (HGTB) model to estimate the likelihood and intensity of power network congestion, enabling proactive congestion reduction. The obtainable transferability of trading routes is determined by taking into consideration the supply-demand balance in the power market, the sensitivity of generating units and power trading networks to transmission lines, and other factors (Wei et al., 2024).
A confidence-based scenario cluster technique and a co-planning model for generators, networks, loads, and energy storage (GNLS) are described by SM et al. Consolidating and analyzing load, solar, and wind data facilitates the process of developing a scenario cluster with confidence that accounts for uncertainties. The GNLS co-planning model includes flexible assessment indices and is solved as a bi-level probabilistic framework on ongoing time periods using Benders decomposition, with a focus on load and battery collaboration (Ma et al., 2024).
In a paper by CX et al. an approach to analyzing the effects of distributed and centralized sources of clean energy on the electrical grid system is presented. The bus state trajectory index is generated with the help of a basic model for the coupling relationship between renewable energy permeability along with access demonstrate electricity and a holomorphic implementing model for non-global fluctuation. This index analyzes the effects of both distributed and centralized renewable energy access on voltage and power system stability under different conditions and suggests configuration options for improving system receptivity (Xu et al., 2023).
These contributions encompass various aspects of distribution network and grid planning, addressing challenges such as uncertainty, correlation concerns, congestion management, and comprehensive energy consumption behavior, thereby advancing the understanding and development of efficient and sustainable energy systems.
In response to the multifaceted challenges posed by intricate system dynamics, emergency faults, and the inadequacy of frequency regulation reserves, FT et al. propose an Islanding with Controlled Islanding (ICI) strategy aimed at ensuring island frequency stability while integrating wind power. First, they work together to optimize load shedding, generator tripping, and the deliberate islanding border in order to create a basic ICI model. They then create a frequency response model with primary frequency management of wind power for the islanded system. Finally, they propose a corresponding linear iterative algorithm to implement the strategy effectively (Tang Fei et al., 2023).
JH et al. introduce an adaptive control technique for Variable Speed Generators (VSGs) considering the limitations of Energy Storage Units’ (ESUs) State of Charge (SOC) to maximize the benefits of flexible parameters in VSG management while addressing concerns about service life loss resulting from operation at limit states. This adaptive approach dynamically adjusts damping factors and inertia in response to system frequency perturbations, aiming to optimize performance. Simulation comparisons using PLECS evaluate the proposed technique against traditional VSG control methods (Han et al., 2023).
From a quadratic frequency modulation approach, SZ et al. present a quick Q-learning based Automatic Generation Control (AGC) technique. They integrate complete sampling with full expectation for multi-area coordination and offer a balance parameter σ to improve convergence accuracy. They also accelerate convergence by using fast Q-learning to replace historical estimation functions with current state estimates. The effectiveness of the technique in attaining optimal multi-area coordination and enhancing frequency deviation management is verified by simulations employing IEEE two-region models and Hubei power grid models (Zhang Shengxi et al., 2023).
ZS et al. offer a hybrid bilevel optimization method to maximize the interaction between PV microgrids and distribution grids. This method uses second-order cone programming and an upgraded consensus approach. Demand response models based on pricing and incentives are developed to manage differential supply and demand dynamics. The combined multilevel strategy for optimization employs centralized optimization at the upper level to decrease grid lost in the distribution grid and distributed optimization at the lower level to iteratively optimize the electrical generated by PV microgrids in order to increase convergence and system efficiency (Shao et al., 2023).
An improved seagull algorithm-based segmented compensation strategy is put out by HC et al. to reduce fluctuations in distributed solar power generation. Using wavelet packet decomposition and state-of-charge adaptation, the method modifies the internal power distribution in a system for energy storage that is made up of lithium-ion cells and supercapacitors. In order to maximize system efficiency while keeping costs down, charging and discharging schedules are optimized based on usage time, power price, state-of-charge information, and the magnitude of nocturnal demand (Chen et al., 2023).
In order to preserve system frequency stability during emergency faults, YL et al. offer a Centralized Frequency Emergency Control (FEC) solution that takes Energy Storage Cluster (ESC) participation into account. They present a generic design approach for optimal droop coefficients to balance power between generators and ESCs, alongside an optimal-droop-based frequency regulation framework to complement traditional techniques. The optimality of droop coefficients is thoroughly demonstrated, ensuring efficient system control (Liu Yang et al., 2024).
When renewable energy resources are integrated into electrical transmission lines, two primary challenges are generated: accurate brief wind turbine forecasts and efficient electricity integration control. These intricacies highlight how crucial it is to have consistent power flow convergence and accurate power system scheduling.
SJ et al. offer a succinct model for short-term wind power generation prediction using an element selection-based Convolutional in nature Neural Network-Bidirectional Long Short-Term Memory Network (CNN-BiLSTM) architecture. One of their methods is an autonomous power flow convergence adjustment model that makes use of the D3QN (Double Dueling Q Network) reinforcement learning algorithm. This novel approach resolves power imbalance problems that result in non-convergence of the flow, enabling efficient control and flexible modification of the operating modes. The model guarantees wind power generation predictions that are accurate by screening multidimensional feature datasets and selecting feature parameters optimally (Jin et al., 2023).
To solve the problem of non-ideal stiffness in PVESS brought on by inaccurate voltage control parameters, GL et al. develop an Equivalent Reduced-Order Model (EROM). This model incorporates low-frequency filter-based simulated inertia loops for regulation together with strategies for controlling voltage and current. By increasing the system’s perceived inertia through the low-pass filter and simplifying inertia analysis through the EROM’s output impedance, this technique enhances system stability. A switch-based representation of PVESS built on the RT-BOX hardware-in-the-loop experimentation system is utilized to thoroughly investigate and verify how voltage control parameters and low-pass filter bandwidth affect system inertia (Li Guanyang et al., 2023).
To enhance fault diagnosis accuracy in scenarios with limited samples or imbalanced problem categories, BW et al. leverage the Glow model to augment wind turbine bearing failure samples. They develop a multi-input fault diagnostic model capable of detecting faults across multiple locations and categories, validated experimentally under various conditions (Wang Bin et al., 2024).
In their thorough analysis of the state-of-the-art ultra-short-term wind power forecasting methods, GY et al. point out several issues, including the lack of new wind farm data and the limited improvements in numerical weather forecast accuracy. They examine integrated power forecasting methods in inclement weather, providing case studies to illustrate their usefulness and suggestions for further research (Yu et al., 2024).
Additionally, WL et al. improve the effectiveness of the Transformer model in time series processing to deal with fluctuations and irregularities resulting from a greater integration of renewable energy resources. Their approach makes use of the mechanism of self-attention and nonlinear simulation capabilities of the Transformer model, and it increases the model’s adaptability in load forecasting by utilizing Data-Slicing and Channel-Independence stages (Lu and Chen, 2024).
Reducing the carbon emissions from power that urban dwellers (CDs) use becomes essential in the quest of low-carbon development and sustainable energy transformation. However, because there is little incentive for emission reduction activities, CDs’ power-related carbon emissions have increased despite the growing attention on reducing carbon emissions. DT et al. offer a model of electricity-related carbon emission quota trading that incorporates a credit rating and scoring system in answer to this difficulty. Using historical power bill payment data, they cluster CDs using the DBSCAN technique. Discrete clusters sensitive to different carbon emission quota values are produced by this clustering procedure. Then, based on the power company’s electricity pricing tiers and overall carbon emission quotas, incentive rules are developed, taking into account the credit scores and clustering results of the CDs. To ascertain the base price of the carbon emission limit, a leader-follower Stackelberg game is also constructed between CDs and the authority firm under specific conditions. At the final settlement, CDs are issued floating greenhouse gas permit prices based on their credit scores (Tang Donglai et al., 2023).
Similarly, DP et al. deploy a multi-objective particle swarm algorithm-based optimal scheduling technique for residential microgrids to handle household load management difficulties and focused usage of electric cars in low-cost electricity periods. By considering time-sharing rates and the travel habits of electric car users, their residential microgrid optimization approach minimizes grid-side energy supply variability while optimizing daily household power expenses. Variations in the battery capacity of energy storage devices and electric vehicles are also assessed by the model as optimization variables. Through the simulation of arithmetic operations inside the Pareto optimal solution set, the model determines the optimal approach to minimize the amount of energy input and output variations on the economic side (Pan et al., 2024).
Furthermore, XX et al. use the Long-Range Energy Alternatives Planning System (LEAP) to develop a fishing model for Zhoushan City in order to study energy usage and greenhouse gas emission trends in the fishing industry under various circumstances. Their study compares three scenarios—low-carbon, reinforced low-carbon, and business as usual—in order to assess energy consumption and emission levels in Zhoushan City’s fishing industry (Xu et al., 2024).
Furthermore, BK et al. investigate user energy characteristics by leveraging key family feature factors for quadratic clustering. They collect and preprocess user energy consumption data, apply an integrated clustering algorithm to analyze energy consumption curves, and conduct two-layer clustering of users within the same group based on questionnaire surveys. The outcomes of one-layer clustering are refined using nonlinear mapping and the Support Vector Machine (SVM) (Kang et al., 2024).
Moreover, LQ et al. propose an integrated energy system environmentally friendly operation optimization methodology that aligns with China’s “dual carbon” targets. Their strategy comprises developing a linked trading mechanism for carbon trade and environmental credits in order to optimize the daily operating expenditures of integrated energy systems and satisfy low-carbon targets (Qin et al., 2024).
Finally, RJ et al. support a charging station planning approach that takes carbon emission patterns into account in relation to the contribution of electric vehicles to carbon-peak targets. In order to predict trends in carbon emissions, they combine the LSTM network with the STIRPAT model. Next, utilizing actual road network data from a particular Shanghai neighborhood, a multi-phase charging station planning model is created to determine the best station placement strategy, balancing the benefits of emission reduction and cost-efficiency (Jia et al., 2024).
JL et al. propose a wildfire catastrophe detection system for power line corridors using Fengyun-3E (FY-3E) satellite data. This system addresses challenges such as significant variations in the angle of solar zenith and shifts in satellite views that affect the detection of infrared channels. By accurately identifying fire areas under challenging air conditions, the program minimizes false alerts. The sensitivity of FY-3E’s fire-spot detection is demonstrated to be four times higher than that of geostationary meteorological satellites through hybrid pixel linear spectrum analysis. The effectiveness of the algorithm is illustrated using the Shanxi provincial electricity grid of the State Grid in China (Li J. et al., 2023).
BO-BERT-GRNN combines Bayesian Optimization (BO) with BERT and Gated Recurrent Neural Networks (GRNN) to capture the complex and variable language in smart grid financial trading strategies. By adopting the BO-BERT-GRNN model, MZ et al. address the innovation management difficulty in financial trading strategies for power system planning. This model predicts power system planning, optimizes hyperparameters, and extracts features from historical data by combining the BERT model, gated recurrent neural network, and Bayesian optimization. According to experimental results, the BO-BERT-GRNN model, which aims to maximize income, control market risk, and allocate power assets efficiently, performs better than current methods in power system planning price prediction, energy transaction risk management, and energy asset allocation (Zhang Mengzhu et al., 2023).
CL et al. suggest a data-driven surrogate-assisted method to solve the Combined Economic/Emission Dispatch (CEED) problems. In order to update improved Kriging-based surrogate models, they suggest using an infilling sampling technique. This technique replaces the objective functions for fuel cost and emissions online, greatly cutting down on the time required to evaluate the objective functions (Lin et al., 2023).
ZJ et al. provide a probabilistic model that uses Mixture Density Network (MDN) and Multitask Learning (MTL) to anticipate tropical cyclone (TC) tracks. The forecasting work is divided into two parts using this model: the probabilistic prediction of the residual between the actual TC location and the causal assessment of a subsequent TC position. According to experimental results, the model that trains MDN on residuals using a unique MTL training technique outperforms existing methods (Zhou et al., 2023).
YW et al. use stacking ensemble learning to provide an online load-loss risk evaluation method for electrical systems. They use RFECV to pick features and build risk samples using traditional load-loss risk evaluation methodologies. Using PSO techniques for optimizing parameters and training four machine learning models results in an efficient online load-loss risk assessment (Wang et al., 2023a).
A generator status monitoring method based on stacked autoencoder (SAE) networks, adaptive thresholding, and multi-source data fusion technology is proposed by CX et al. Using auto-coding technology and the Supervisory Control And Data Acquisition-Power System Monitoring Unit (SCADA-PMU) data fusion method, they build a defect detection model. They then integrate trend change in monitoring quantity with smoothed reconstruction error to achieve fault judgment (Xing et al., 2023).
In the Hail region of Saudi Arabia, SY et al. study the efficiency of wind-solar generators (WSG) using a fractional order Proportional Integral (PI) controller (FOPI) installed on the grid connection line. The performance of FOPI controllers, which are designed to control line currents, is evaluated against that of traditional PI controllers (Younsi et al., 2023).
In response to uncertainty, JL et al. suggest a cooperative configuration technique for renewable energy and energy storage. They determine the best mixes of renewable energy and energy storage by leveraging the wind-to-solar ratio (W/S) and energy-to-power ratio (E/P) and building a model with hourly granularity (Liao and Wu, 2024).
A decentralized control method for air conditioning load involvement in power system primary frequency management is presented by BJ et al. By guaranteeing that air conditioning loads react to system frequency changes in a methodical manner without requiring communication, this technique improves the impact of frequency regulation (Jiang Baofeng et al., 2024).
YY et al. use a Cooling, Heating, and Power (CCHP) system with energy storage to study the planning of day-ahead supply and demand for energy in the summer. They take into account the integration of renewable energy and use the Gurobi solver to optimize energy supply and demand under different scenarios (Yufeng et al., 2024).
A multi-objective planning technique based on Condition Value at Risk (CvaR) is put out by YH et al. for integrated energy systems with a carbon trading mechanism. This approach takes into account the costs and risks associated with carbon trading while optimizing economic and environmental goals (Huang et al., 2024).
LL et al. introduce a dual-model predictive control strategy for regulating fuel cell stack temperature, demonstrating its effectiveness through comparative analysis with traditional PID control (Liu Lidong et al., 2024).
ZZ et al. have devised an assessment method and statistical index system for new energy storage power facilities. The method evaluates several stages of development using indices for economic, regulatory, energy-efficient, reliable, and environmental protection (Zhang et al., 2024).
WJ et al. construct a physical verification platform for power inspection robot manipulator control algorithms, verifying the effectiveness of PID controllers and expert PID controllers (Jiang W. et al., 2024).
By considering the average production order of loads, XW et al. provide an electrical management technique that improves grid regulating flexibility by balancing the requirements for safe production order and load response capacity (Wang X. et al., 2024).
A stacking ensemble learning-based online load-loss risk assessment method for electrical systems is proposed by YW et al. They create a conventional power flow analysis-based load-loss risk assessment technique and use recursive removal of features by cross-validation (RFECV) for feature selection. then they build an ensemble-based learning algorithm by combining four distinct machine learning models, and then use particle swarm optimization (PSO) techniques to fine-tune the model’s parameters for even better performance (Wang et al., 2023b).
WF: Writing–original draft, Writing–review and editing. NY: Writing–original draft, Writing–review and editing. ZL: Writing–original draft, Writing–review and editing.
The author(s) declare that no financial support was received for the research, authorship, and/or publication of this article.
The authors declare that the research was conducted in the absence of any commercial or financial relationships that could be construed as a potential conflict of interest.
All claims expressed in this article are solely those of the authors and do not necessarily represent those of their affiliated organizations, or those of the publisher, the editors and the reviewers. Any product that may be evaluated in this article, or claim that may be made by its manufacturer, is not guaranteed or endorsed by the publisher.
Chen, H., Cheng, J., Li, Z., Abu-Siada, A., and Li, H. (2023). Distributed photovoltaic power fluctuation flattening strategy based on hybrid energy storage. Front. Energy Res. 11, 1303522. doi:10.3389/fenrg.2023.1303522
Han, J., Feng, X., Zhao, H., Hu, P., and He, C. (2023). Adaptive VSG control strategy considering energy storage SOC constraints. Front. Energy Res. 11, 1278648. doi:10.3389/fenrg.2023.1278648
Huang, Yu, He, G., Pu, Z., Zhang, Y., Luo, Q., and Ding, C. (2024). Multi-objective particle swarm optimization for optimal scheduling of household microgrids. Front. Energy Res. 11, 1354869. doi:10.3389/fenrg.2023.1354869
Jia, R., Xia, X., Xuan, Y., Sun, Z., Gao, Y., and Qin, S. (2024). Low-carbon planning of urban charging stations considering carbon emission evolution characteristics and dynamic demand. Front. Energy Res. 12, 1359824. doi:10.3389/fenrg.2024.1359824
Jiang, B., Yang, Z., Xiong, W., Chen, L., Wang, P., and Liao, S. (2024a). Decentralized control strategy of air-conditioning loads for primary frequency regulation based on environment information. Front. Energy Res. 11, 1347789. doi:10.3389/fenrg.2023.1347789
Jiang, W., Wang, X., and Liu, Z. (2024b). Control and physical verification of 6-DOF manipulator for power inspection robots based on expert PID algorithm. Front. Energy Res. 12, 1367903. doi:10.3389/fenrg.2024.1367903
Jin, S., Liu, Q., Zhang, W., He, Z., He, Y., Zhang, L., et al. (2023). Data-driven methods for situation awareness and operational adjustment of sustainable energy integration into power systems. Front. Energy Res. 11, 1253206. doi:10.3389/fenrg.2023.1253206
Kang, B., Xu, Z., He, W., Ding, G., Han, W., Sun, M., et al. (2024). Research on the double-layer clustering method of residential energy use characteristics under the background of energy system energy savings and carbon reduction. Front. Energy Res. 11, 1326257. doi:10.3389/fenrg.2023.1326257
Li, G., Wang, J., Wang, X., and Zhang, L. (2023c). Virtual inertia analysis of photovoltaic energy storage systems based on reduced-order model. Front. Energy Res. 11, 1276273. doi:10.3389/fenrg.2023.1276273
Li, J., Chen, J., Yu, H., Jiang, M., Lu, Z., Zhou, Y., et al. (2023d). Wildfire monitoring technologies of transmission line corridors based on Fengyun-3E satellite imaging. Front. Energy Res. 11, 1265516. doi:10.3389/fenrg.2023.1265516
Li, J., Wang, T., Huang, G., Ou, M., Zhao, W., and Mao, T. (2023b). Research on joint planning method of supply and demand considering users’ comprehensive energy consumption behavior and policy orientation. Front. Energy Res. 11, 1294287. doi:10.3389/fenrg.2023.1294287
Li, J., Wang, T., Tang, S., Jiang, J., and Chen, S. (2023a). Planning distribution network using the multi-agent game and distribution system operators. Front. Energy Res. 11, 1244394. doi:10.3389/fenrg.2023.1244394
Liao, J., and Wu, G. (2024). Collaborative configuration of renewable energy and energy storage under fixed investment in the decarbonization process. Front. Energy Res. 12, 1345780. doi:10.3389/fenrg.2024.1345780
Lin, C., Liang, H., Pang, A., and Zhong, J. (2023). Data-driven method of solving computationally expensive combined economic/emission dispatch problems in large-scale power systems: an improved kriging-assisted optimization approach. Front. Energy Res. 11, 1273760. doi:10.3389/fenrg.2023.1273760
Liu, L., Zhang, M., and Gong, J. (2024c). Enhancing fuel cell performance through a dual MPC strategy for coordinated temperature management. Front. Energy Res. 12, 1358468. doi:10.3389/fenrg.2024.1358468
Liu, W., Zhou, B., Ou, M., Zhao, W., Huang, G., and Mao, T. (2024a). Electricity–gas multi-agent planning method considering users’ comprehensive energy consumption behavior. Front. Energy Res. 11, 1341400. doi:10.3389/fenrg.2023.1341400
Liu, Y., Xie, P., Wu, G., Chen, Y., Lin, X., and Lu, Q. (2024b). Frequency emergency control strategy in power systems considering the participation of energy storage clusters. Front. Energy Res. 12, 1355344. doi:10.3389/fenrg.2024.1355344
Lu, W., and Chen, X. (2024). Short-term load forecasting for power systems with high-penetration renewables based on multivariate data slicing transformer neural network. Front. Energy Res. 12, 1355222. doi:10.3389/fenrg.2024.1355222
Ma, Su, Liu, Lu, and Cheng, H. (2024). Power generation–network–load–energy storage co-planning under uncertainty. Front. Energy Res. 12, 1355047. doi:10.3389/fenrg.2024.1355047
Pan, D., Zhang, L., Wang, B., Jia, J., Song, Z., and Zhang, X. (2024). Multi-objective planning of integrated energy system based on CVaR under carbon trading mechanism. Front. Energy Res. 12, 1310301. doi:10.3389/fenrg.2024.1310301
Qin, L., Ma, H., Huang, C., Li, H., Wu, S., and Wang, G. (2024). Low-carbon operation optimization of integrated energy system considering CCS-P2G and multi-market interaction. Front. Energy Res. 11, 1337164. doi:10.3389/fenrg.2023.1337164
Shao, Z., Liu, C., Yao, R., Wang, C., Li, L., Liu, Z., et al. (2023). Hybrid bilevel optimization-based interaction between the distribution grid and PV microgrids with differentiated demand response. Front. Energy Res. 11, 1297650. doi:10.3389/fenrg.2023.1297650
Song, L., and Zhang, Y. (2021). Research on dynamic robust planning method of active distribution network considering correlation. Power Syst. Prot. Control 49 (10), 178–187.
Tang, D., Li, Q., Zhang, J., Chen, Y., Liu, Y., and Song, W. (2023b). Credit rating-and credit score-based carbon emission quota trading model of city dwellers. Front. Energy Res. 11, 1250717. doi:10.3389/fenrg.2023.1250717
Tang, F., Guo, Y., Wei, X., Chen, M., Sun, J., and Deng, H. (2023a). An intentional controlled islanding strategy considering island frequency stability for power system with wind-power integrated. Front. Energy Res. 11, 1247412. doi:10.3389/fenrg.2023.1247412
Wang, B., Wang, F., Zou, Y., Fang, S., Luan, H., and Zhang, L. (2024a). Proposed multi-signal input fault diagnosis of wind turbine bearing based on glow model. Front. Energy Res. 11, 1342811. doi:10.3389/fenrg.2023.1342811
Wang, X., Chen, Z., Xie, H., Liao, S., Ye, X., and Chen, G. (2024b). Demand-side response power control strategy considering load production sequence requirements. Front. Energy Res. 12, 1370301. doi:10.3389/fenrg.2024.1370301
Wang, Y., Sun, Y., Dan, Y., Li, Y., Cao, J., and Han, X. (2023a). Online load-loss risk assessment based on stacking ensemble learning for power systems. Front. Energy Res. 11, 1281368. doi:10.3389/fenrg.2023.1281368
Wang, Y., Sun, Y., Dan, Y., Li, Y., Cao, J., and Han, X. (2023b). Online load-loss risk assessment based on stacking ensemble learning for power systems. Front. Energy Res. 11, 1281368. Response Letter for Editoral. doi:10.3389/fenrg.2023.1281368
Wei, C., Jia, L., Wang, X., Cai, Y., Huang, Y., Huang, Y., et al. (2024). Calculation of the available transfer capability of trading channels based on power network congestion forecasting. Front. Energy Res. 12, 1351306. doi:10.3389/fenrg.2024.1351306
Xing, C., Xi, X., He, X., and Liu, M. (2023). Generator condition monitoring method based on SAE and multi-source data fusion. Front. Energy Res. 11, 1308957. doi:10.3389/fenrg.2023.1308957
Xu, C., He, S., Wang, L., and Yu, H. (2023). Difference analysis method of grid connection influence between CRE and DRE based on bus state trajectory. Front. Energy Res. 11, 1266252. doi:10.3389/fenrg.2023.1266252
Xu, X., Gui, F., and Zhao, S. (2024). Simulation of fishery energy consumption and carbon emissions in Zhoushan City based on the long-range energy alternatives planning system model. Front. Energy Res. 11, 1319915. doi:10.3389/fenrg.2023.1319915
Younsi, S., Kahouli, O., Alsaif, H., Ali, N. B., and Chaabane, M. (2023). Grid interface control of wind–solar generator in Hail region of Saudi Arabia using FOPI controller. Front. Energy Res. 11, 1309889. doi:10.3389/fenrg.2023.1309889
Yu, G., Shen, L., Dong, Q., Cui, G., Wang, S., Xin, D., et al. (2024). Ultra-short-term wind power forecasting techniques: comparative analysis and future trends. Front. Energy Res. 11, 1345004. doi:10.3389/fenrg.2023.1345004
Yuan, M., Zhang, H., Ji, K., Liu, Y., Tang, X., Tao, B., et al. (2024). Two-stage robust planning method for distribution network energy storage based on load forecasting. Front. Energy Res. 12, 1327857. doi:10.3389/fenrg.2024.1327857
Yufeng, Y., Zhicheng, Z., Xubing, X., Yaxin, P., and Linjun, S. (2024). Intelligent day-ahead optimization scheduling for multi-energy systems. Front. Energy Res. 11, 1349194. doi:10.3389/fenrg.2023.1349194
Zhang, M., Shen, L., and Guo, J. (2023b). Analysis on innovation management of power financial transaction strategy integrating BO-BERT-GRNN model. Front. Energy Res. 11, 1269059. doi:10.3389/fenrg.2023.1269059
Zhang, S., Lan, F., Xue, B., Chen, Q., and Qiu, X. (2023a). A novel automatic generation control method with hybrid sampling for multi-area interconnected girds. Front. Energy Res. 11, 1280724. doi:10.3389/fenrg.2023.1280724
Zhang, Z., Ma, G., Song, N., Wang, Y., Xia, J., Xu, X., et al. (2024). A performance evaluation method for energy storage systems adapted to new power system interaction requirements. Front. Energy Res. 12, 1365419. doi:10.3389/fenrg.2024.1365419
Keywords: operation, smart grids, control, planning, energy system, power system
Citation: Fu W, Yang N and Li Z (2024) Editorial: Smart energy system for carbon reduction and energy saving: planning, operation and equipments. Front. Energy Res. 12:1444585. doi: 10.3389/fenrg.2024.1444585
Received: 05 June 2024; Accepted: 24 July 2024;
Published: 21 August 2024.
Edited and reviewed by:
Michael Carbajales-Dale, Clemson University, United StatesCopyright © 2024 Fu, Yang and Li. This is an open-access article distributed under the terms of the Creative Commons Attribution License (CC BY). The use, distribution or reproduction in other forums is permitted, provided the original author(s) and the copyright owner(s) are credited and that the original publication in this journal is cited, in accordance with accepted academic practice. No use, distribution or reproduction is permitted which does not comply with these terms.
*Correspondence: Nan Yang, eW55eWF5eUBjdGd1LmVkdS5jbg==
Disclaimer: All claims expressed in this article are solely those of the authors and do not necessarily represent those of their affiliated organizations, or those of the publisher, the editors and the reviewers. Any product that may be evaluated in this article or claim that may be made by its manufacturer is not guaranteed or endorsed by the publisher.
Research integrity at Frontiers
Learn more about the work of our research integrity team to safeguard the quality of each article we publish.