- Energy and Process Systems Engineering, ETH Zurich, Zurich, Switzerland
National energy systems require flexibility to accommodate increasing amounts of variable renewable energy. This flexibility can be provided by demand-side management (DSM) from industry. However, the flexibility potential depends on the characteristics of each industrial process. The enormous diversity of industrial processes makes it challenging to evaluate the total flexibility provision from industry to sector-coupled energy systems. In this work, we quantify the maximum cost reductions due to industrial DSM in the net-zero sector-coupled Swiss energy system, and the relationship between cost reductions and various industrial process characteristics. We analyze the flexibility of industrial processes using a generic, process-agnostic model. Our results show that industrial DSM can reduce total energy system costs by up to 4.4%, corresponding to 20% of industry-related energy costs. The value of flexibility from industrial DSM depends not only on the process characteristics but also on the system’s flexibility alternatives, particularly for flexibility over seasonal time horizons. As one specific option for industrial DSM, we find that thermal energy storage (TES) technologies available today could realize between 28% and 61% of the maximum cost reductions from industrial DSM, making TES a promising DSM solution and showing that industrial DSM is an accessible and cost-effective flexibility option.
1 Introduction
Countries are increasingly setting net-zero emissions targets to address climate change (United Nations, 2023). Meeting this target requires rapid decarbonization across sectors, mainly by replacing fossil fuels with renewable energy sources. Renewable energy can be extracted as electricity from natural resources, for example, through photovoltaics and wind turbines for harnessing sun and wind energy, respectively. However, sun and wind energy are subject to intermittency, making the associated electricity volatile (Ramsebner et al., 2021).
As non-power energy sectors transition, electrification becomes increasingly important as the medium for integrating renewables. For example, the heating sector can be electrified by heat pumps and the transportation sector can be electrified by battery electric vehicles (Sternberg and Bardow, 2015). The resulting reliance of all energy sectors on electricity leads to sector-coupling, where the electricity sector becomes the central pillar for the overall energy system (Bardow et al., 2023). However, systems with a high share of volatile renewable electricity require flexibility to ensure matching of electricity supply and demand at every point in time. Flexibility refers to an energy system’s ability to cope with the variability and unpredictability that variable renewable energy introduces on different time scales, while reliably supplying all the demanded energy to end users (Bardow et al., 2023). The need for flexibility increases rapidly for a share of renewable energy above 80% (Shaner et al., 2018).
Flexibility can be provided from the supply side through imports and exports, dispatchable production, and energy storage. However, each of these options has limitations: Electricity and fossil fuel imports are subject to availability abroad and geopolitics. Dispatchable carbon-neutral generation from fossil fuels requires carbon capture and storage (CCS), which is not widely accepted and can be difficult to implement at scale due to both social and physical constraints (Budinis et al., 2018). Other renewable forms of dispatchable generation, through hydropower, geothermal, or bio-based power plants are limited by resource availability. Regarding storage technologies, batteries for electricity storage are expensive and not suitable for long-duration applications due to their self-discharge characteristic (Albertus et al., 2020; Gabrielli et al., 2020; Sepulveda et al., 2021). Power-to-hydrogen is a storage option that is stable over long time horizons, but has a low round-trip efficiency (Pellow et al., 2015; Gabrielli et al., 2020). Pumped hydro storage, while efficient, is subject to high capital costs, topographic limitations, and environmental concerns regarding surrounding areas (Koohi-Fayegh and Rosen, 2020).
Flexibility can also be provided in the form of thermal energy storage (TES), where heating or cooling is stored for later use either directly as thermal energy or reconversion into electricity. While TES has a low round-trip efficiency back to electricity (Viswanathan et al., 2022), TES can have round-trip efficiencies greater than 95% for heat recovery (Murakoshi and Fushimi, 2022). Additionally, TES technologies are significantly cheaper than batteries (Victoria et al., 2019; IRENA, 2020), making TES particularly promising for industrial applications with a substantial heat demand (IRENA, 2020). However, as heat transport over long distances is limited (Hammond and Norman, 2014), TES for heat recovery should be employed on-site where the heat is consumed.
Limitations of the flexibility options described above can be alleviated by demand-side management (DSM). Demand-side management refers to the shifting of energy consumption patters to obtain a desired energy consumption profile (Meyabadi and Deihimi, 2017). Kachirayil et al. (2022) identify DSM as one of the most impactful flexibility levers for sector-coupled energy systems reliant on volatile renewable electricity. A good candidate for demand-side management is the industry sector due to its large energy demand in the form of both electricity and thermal energy, its potential for storing products over long time horizons, its already-existing metering infrastructure, and the avoidance of behavior change from individual end consumers (Zhang and Grossmann, 2016; Williams et al., 2023). Industrial DSM can be implemented through production flexibility, where production schedules shift to follow the availability of renewable electricity. For instance, an industrial process can over-produce during the daytime hours to take advantage of low prices caused by photovoltaic availability. The overproduction is stored and the process under-produces during night-time hours such that the overall production stays the same. Promising example processes for industrial DSM have been identified with high electricity demands and technical possibilities for load shifting (Arnold and Janssen, 2018). These processes include: aluminum electrolysis (Bao et al., 2020; Golmohamadi, 2022), cement and raw mills (Bohlayer et al., 2020; Golmohamadi, 2022), air separation (Caspari et al., 2019; Zhang and Pinto, 2022), electric arc furnace (Manana et al., 2021; Wachs et al., 2023), pulp and paper (Helin et al., 2017), and copper production (Röben et al., 2022; Wang et al., 2024).
Demand-side flexibility can also stem from the energy demands of industrial processes. As discussed above, thermal energy can be stored on-site with round-trip efficiencies greater than 95%. If heat production is electrified, such on-site TES enables the shifting of electricity consumption to follow the temporal availability of renewables without modifying the actual process operation (Arteconi et al., 2013). Thus, industrial TES is a promising DSM option that can be applied to a wide range of processes. According to Williams et al. (2023), industries with large thermal energy storage are well-suited for DSM applications. Cirocco et al. (2022) demonstrate significant cost savings via thermal energy storage on-site an industrial food processing plant as a demand-side management measure.
The extent of flexibility achievable from industrial DSM depends on the characteristics of the production processes (Arnold and Janssen, 2018; Schäfer et al., 2020; Bielefeld et al., 2023):
These process characteristics influence the potential value from industrial DSM for the overall system. Promising industrial candidates can be identified via favorable combinations of these characteristics. Additionally, some characteristics can be influenced via investments, e.g., the installation of larger storage capacities. Therefore, understanding the characteristics’ effects on industrial DSM can help to find promising processes and guide financial incentives.
Industrial DSM can affect the costs and environmental impacts of individual industrial sites, as shown for example, by (Klaucke et al., 2017; Schäfer et al., 2020; Torabi et al., 2020). However, these works measure the DSM contributions to the sites rather than to the overall energy system. Other studies consider the contributions of industrial DSM from a power-system perspective, rather than from an industrial site perspective (Paulus and Borggrefe, 2011; Papadaskalopoulos et al., 2018; Marañón-Ledesma and Tomasgard, 2019; Lu et al., 2021; He et al., 2023). However, the non-power energy sectors that require electricity also contribute to the energy system’s flexibility needs. Thus, an investigation of industrial DSM within a sector-coupled energy system is needed to capture the full flexibility potential. Still, within sector-coupled energy systems, only a subset of the industrial flexibility has been considered so far: Nebel et al. (2020) consider the flexibility provision from DSM of aluminum electrolysis to the German sector-coupled energy system. Cruz et al. (2023) consider the flexibility provision from DSM of industrial electricity demands for Sweden. However, as these works only consider a subset of industrial energy demands for their respective analyses, they do not resolve the total potential flexibility provision from industrial DSM. Additionally, a general understanding of the relationships between industrial process characteristics and the resulting potential flexibility provision is still missing. Thus far, to the best of the authors’ knowledge, no study has quantified the total potential contributions of industrial DSM to a net-zero sector-coupled national energy system, resolving the impact of varying industrial process characteristics.
In the present study, we evaluate how varying degrees of industrial DSM affect the costs and the needs for other flexibility options of the Swiss net-zero sector-coupled energy system. Varying degrees of industrial DSM are modelled by varying the industrial process characteristics listed above. We model the Swiss sector-coupled energy system using our in-house linear optimization framework SecMOD (Reinert et al., 2022), and employ a snapshot approach for the year 2050. That is, we constrain the energy system to net-zero emissions, and determine the system’s cost-optimal investments and operation for varying degrees of industrial DSM. To determine an upper bound on the potential cost reductions, we do not consider costs associated with industrial DSM. However, we include a sensitivity analysis that evaluates thermal energy storage (TES) as a concrete DSM measure while accounting for TES-related costs. We consider three scenarios varying the availability and operating costs of new dispatchable power plants to compare the contributions from industrial DSM across systems with varying flexibility alternatives. To represent industry, we create a generic, process-agnostic model comprised of the Swiss industry’s electricity and thermal energy requirements. Emissions associated with industrial production therefore arise from the energy system’s electricity and thermal energy supply. This set-up allows us to vary industrial process characteristics without the need to model specific industries and processes. Our approach allows us to quantify the maximum potential of industrial DSM as a flexibility provider to net-zero sector-coupled national energy systems. Furthermore, we can assess the extent to which the maximum potential can be harnessed through thermal energy storage.
In Section 2, we briefly introduce the energy system model and discuss the modeling of industrial DSM in detail. In Section 3, we present the results of the study and in Section 4 we highlight the key takeaways.
2 Modeling industrial DSM in sector-coupled energy systems
As the focus of this study is industrial DSM, we only briefly summarize the Swiss energy system model in Section 2.1. Further details, including all modelling assumptions and techno-economic parameters of the included technologies, are provided in detail in the Supplementary Material. We describe the modeling of industry and the industrial DSM characteristics in detail in Section 2.2. Section 2.3 introduces our three scenarios and associated sensitivities.
2.1 Swiss sector-coupled energy system
The Swiss sector-coupled energy system is modelled with our in-house open-source linear optimization framework SecMOD (Reinert et al., 2022), designed for flexible modelling of multi-sector energy systems. We consider the sectors: non-industrial electricity, industry, residential heat, and private transportation. We also consider storage technologies, power-to-X technologies, and carbon capture and storage (CCS) via direct air capture (DAC). We do not consider waste heat recovery from our power-to-X technologies as a conservative assumption in line with our aim of quantifying the maximum cost reductions from industrial DSM. However, we do not expect our results to be highly impacted by this assumption as power-to-X is mainly used for seasonal flexibility, maximizing production during the summer months when heat demand is low and when renewables availability is high for direct electrified heat production. We focus on the year 2050, adding an exogenous net-zero operational emissions constraint in line with Swiss policy targets (The Federal Council, 2019). For the industry sector, we consider the industrial electricity demand together with heat demands at three temperature levels (Section 2.2).
The optimization framework determines the cost-optimal investment and operation decisions to reach the net-zero emissions target, while ensuring that exogenous demands are met (Figure 1). Demands for non-industrial electricity, residential heat, and private transportation are provided separately (details in the Supplementary Material), while demands for industrial electricity and heat are provided in an aggregated fashion to represent Swiss industrial demands. We only consider operational emissions for the net-zero target corresponding to current accounting practice (Rypdal et al., 2006). To focus the analysis on the flexibility needs of a sector-coupled energy system with maximal renewable electricity penetration, we exclusively consider electrified technology options for the residential heat and private transportation sectors. The technology options included in the model are specified in Table 1.
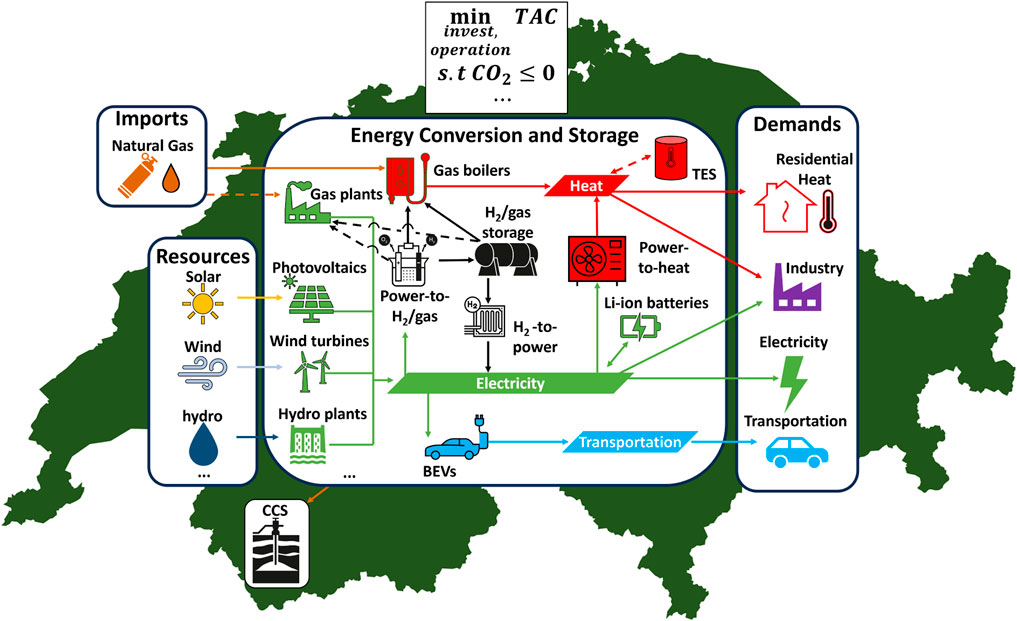
Figure 1. System boundaries, energy system setup, and simplified optimization formulation for the sector-coupled energy system model for Switzerland. The full optimization problem formulation can be found in Reinert et al. (2022). Dashed lines represent connections that exist for specific scenarios or sensitivities (Section 2.3). Not all technologies are depicted, see Table 1 for a comprehensive list of the technologies included in each sector. TAC: total annualized costs; CCS: carbon capture and storage; BEV: battery electric vehicle; TES: thermal energy storage;
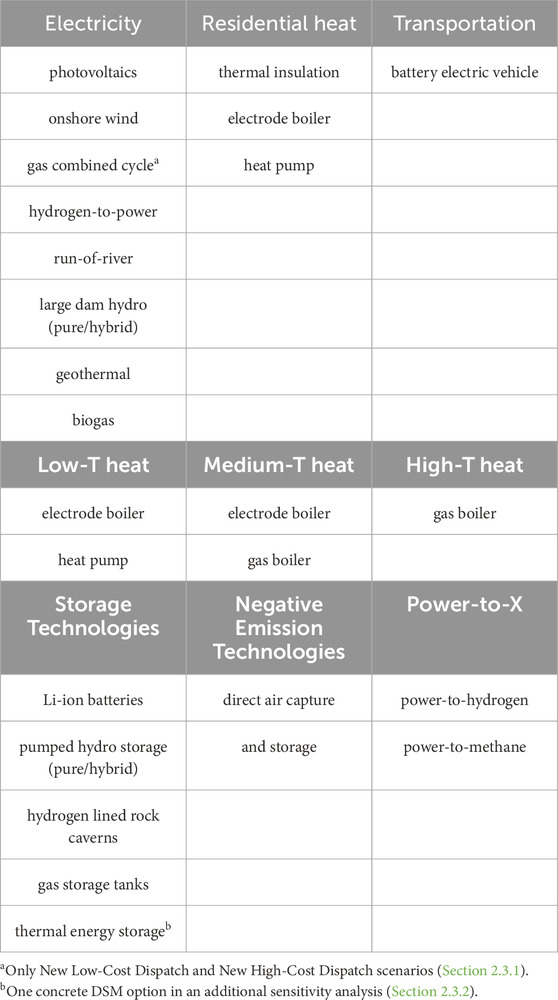
Table 1. Technology options provided for modeling the Swiss sector-coupled energy system. Further details are provided in the Supplementary Material.
The energy system is modelled as a 1-node system. By excluding spatial resolution in our system setup, we may underestimate the energy system’s flexibility needs (Pfenninger et al., 2014). However, as the contributions from industrial DSM can either increase or decrease depending on the spatial distribution of industry vs. the systems’ flexibility needs, we believe that our one-node representation provides a balanced approximation of the upper bound contributions from industrial DSM. Hourly time series are provided and aggregated with a temporal resolution of 25 typical days. The number of typical days was selected by running a sensitivity analysis, investigating how the number of typical days affects the total annualized system costs. We selected 25 typical days as the lowest number of typical days for which the total cost stabilizes (Supplementary Figure S4 in the Supplementary Material) to keep the computation time as low as possible (Supplementary Figure S5). To allow for seasonal storage, the typical days are interlinked using the method developed by Kotzur et al. (Kotzur et al., 2018). Note that Switzerland today has 8.8 TWh of seasonal storage from hydro reservoirs (Swiss Federal Office of Energy (SFOE), 2020a), which is substantial considering that it comprises 14% of the overall electricity demand in 2019 (Swissgrid, Feb. 2020). Modeling details of the Swiss sector-coupled energy system can be found in the Supplementary Material.
2.2 Implementation of industrial demand-side management
In this section, we discuss the representation of the industrial sector. We also discuss how industrial DSM is modelled using the three characteristics discussed in Section 1. The hourly electricity and heat demands of Swiss industry are aggregated into a generic industrial process. This aggregated process produces 1 “good/hour”, while consuming the hourly industrial energy demands for Switzerland. We use an industrial electricity demand of 6 TWh (Swiss Federal Office of Energy (SFOE), 2020b) and a heat demand of 20 TWh (Marcucci et al., 2021) projected in 2050. The heat demand refers to process heat and is split into three temperature levels (Table 2) (Swiss Federal Office of Energy (SFOE), 2020b).
The demands in Table 2 represent the base industrial energy demands,
The contributions from industrial DSM are evaluated by performing a parameterized study of the industrial process characteristics introduced in Section 1. An industrial goods storage tank is introduced to serve as a buffer for industrial over- and under-production (Figure 2). In order to obtain an upper-bound on cost reductions from industrial DSM, no investment or operating costs are associated with the industrial sector, including costs for production and storage of industrial goods. Still, the total amount of goods to be produced and the corresponding energy demands are fixed, limiting the overall potential of industrial DSM. The resulting upper-bound cost reductions can then be compared to the real costs associated with DSM measures, such as additional production and storage capacities. Such a comparison is conducted explicitly for thermal energy storage in Section 2.3.2.
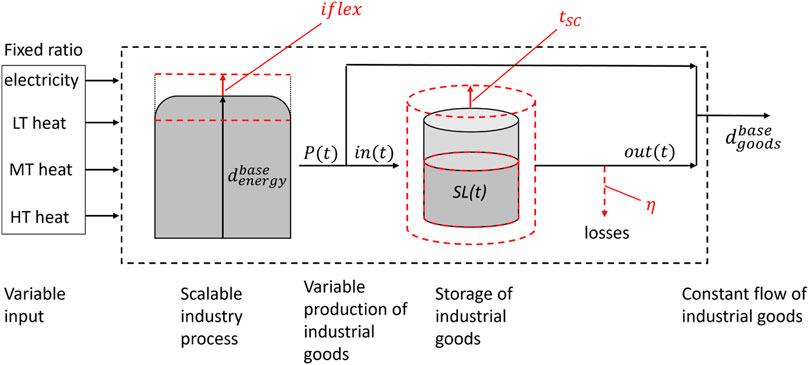
Figure 2. Schematic representation of the relationship between the parameterized industrial DSM process characteristics (
The three modelled process characteristics are described below:
An iflex value of 0 corresponds to no varying of the base load and an iflex of 1 corresponds to the ability to shift 100% of the base load at a given time step, ranging from a complete shutdown to the doubling of base production.
For example, with a
An
The relationship between the three parameters (
Eq. 5 constrains the production used at a given time step,
Similar to the implementation in Schäfer et al. (2020), the circularity constraint in Eq. 6 prevents the industry storage from acting as a source or sink for industrial product, where
Eq. 7 models the development of the stored product inventory
where
Eq. 8 shows how the demand of industrial goods is met at every time step with a combination of production,
Finally, Eq. 9 constrains the storage level with respect to the parameterized storage capacity,
2.3 Scenarios and sensitivities
2.3.1 Scenarios
Three scenarios represent energy systems with varying dispatchable flexibility alternatives. As a proxy for dispatchable flexibility, we consider gas power plants with carbon capture and storage (CCS). We vary the natural gas import prices and restrictions on the utilization of gas power plants with CCS. We expect comparable results when green fuels or electricity could be imported for flexibility. The three scenarios are described below.
2.3.2 Sensitivity analyses
Two sensitivity analyses were carried out for each scenario to serve as benchmarks against which to compare the upper bound cost reductions from industrial DSM.
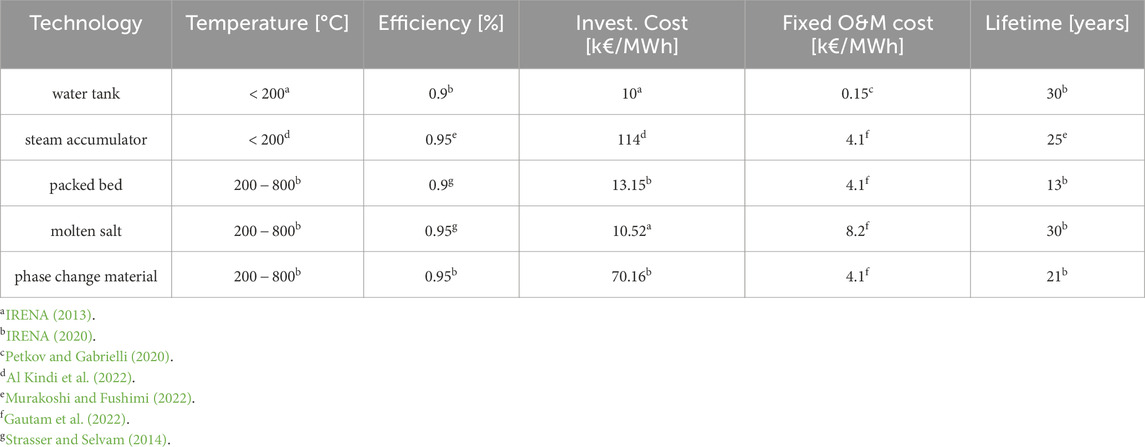
Table 3. Techno-economic parameters for thermal energy storage (TES) technologies included in the TES as a DSM measure sensitivity.
3 Results and discussion
The maximum system cost reductions from industrial DSM range from 2% to 4.4% of overall system costs across the three scenarios. These cost reductions make up between 12% and 20% of the industry-related energy costs (Figure 3). Thus, industry has a substantial incentive to contribute to DSM. Notably, thermal energy storage (TES) as a DSM measure can harness between 28% and 61% of the maximum cost reductions from industrial DSM. Overall, our study shows that industrial DSM reduces overall system costs, and that the cost reductions comprise up to 1/5 of industry-related energy costs. Additionally, TES provides a promising solution for flexibility provision from industry, showing that a large portion of the cost reduction potential from industrial DSM is achievable.
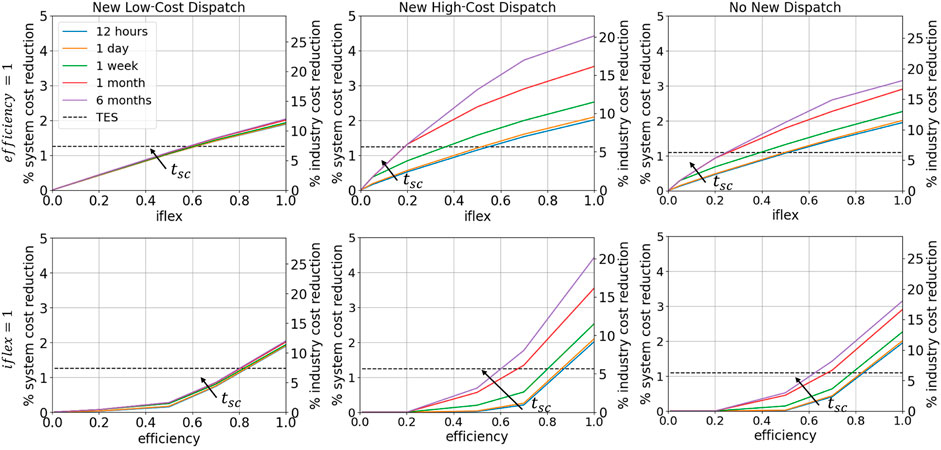
Figure 3. Top row: % cost reduction compared to a system with no industrial DSM (left axis) and compared to industry-related energy costs with no industrial DSM (right axis) as a function of load-varying potential, iflex, for 100% discharge efficiency
In the New Low-Cost Dispatch scenario, industrial DSM reduces costs by up to 2%. The maximum cost reduction remains around 2% regardless of the industrial storage time horizon (Figure 3). In the New High-Cost Dispatch scenario, the maximum reductions increase from 2% to 4.4% as more storage capacity becomes available to enable long-term flexibility. The comparison between the New Low-Cost Dispatch and the New High-Cost Dispatch scenarios shows that the value of long-term industrial storage depends on the system’s flexibility alternatives. More generally, our study shows that the contributions from industrial DSM depend not only on the industrial DSM characteristics, represented by the three parameters (
Industrial DSM can lead to 6 TWh of energy equivalents stored in the form of industrial goods for seasonal flexibility (Table 4). This energy equivalent is substantial compared to the already-existing seasonal flexibility options for Switzerland (8.8 TWh of hydro storage (Swiss Federal Office of Energy (SFOE), 2020a)). This finding indicates that the contributions from industrial DSM could be larger for countries with fewer seasonal flexibility options or more industry than Switzerland.
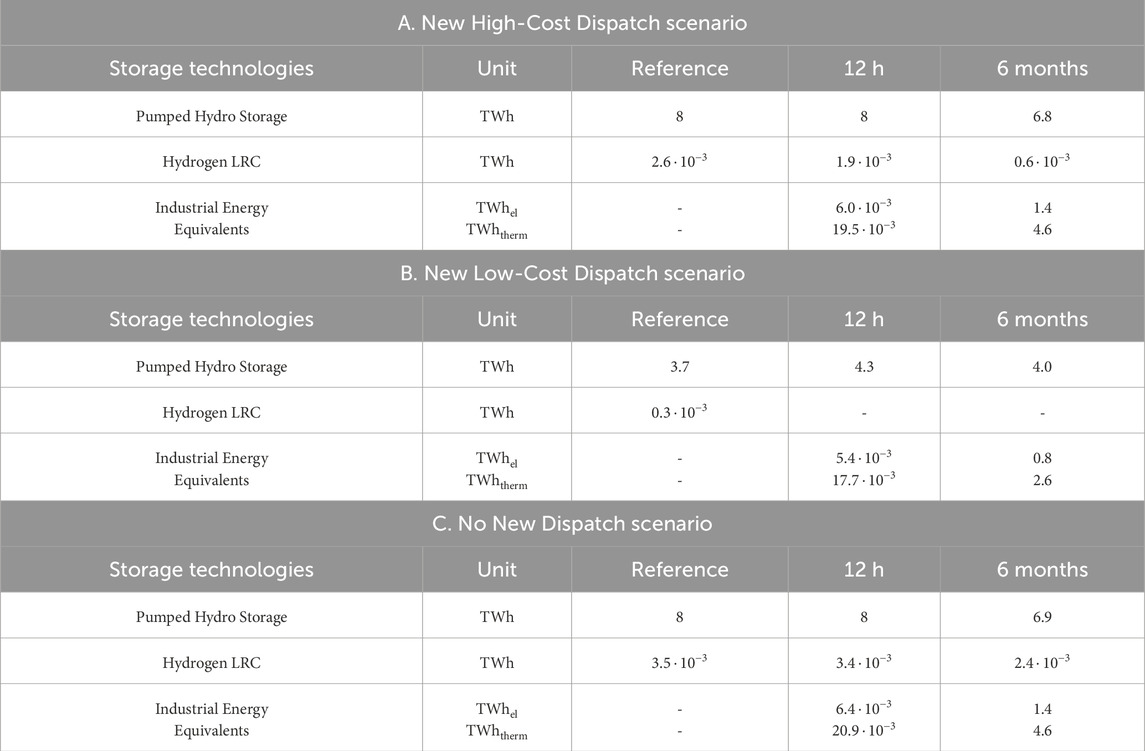
Table 4. Maximum used storage for different storage technologies and for varying storage capacities,
Section 3.1 discusses the system effects of industrial DSM. Section 3.2 presents the results of implementing thermal energy storage (TES) as a specific DSM measure. In Sections 3.3 and 3.4, we discuss findings regarding the effect of storage capacity and DSM losses on cost reductions from industrial DSM.
3.1 System responses to industrial DSM
Industrial DSM can decrease system costs for all scenarios (Figure 3, top row). However, if the alternative flexibility options are more expensive, potential for cost reductions is higher. In the New High-Cost Dispatch scenario, increasing storage capacity,
3.1.1 New High-Cost Dispatch scenario
We first discuss the New High-Cost Dispatch scenario, as industrial DSM shows the highest contributions under this system configuration. In the New High-Cost Dispatch scenario without industrial DSM, the system does not produce any electricity from natural gas due to the high natural gas prices. The system produces all electricity through renewable sources and relies on 2.6 GWh of hydrogen lined rock caverns (LRCs) and 8 TWh of pumped hydro storage for seasonal flexibility. In addition, the system relies on natural gas imports for medium and high temperature heat production mainly during the winter months, when renewable electricity availability is insufficient for synthetic methane production (Figure 4). 105 GWh of methane storage is used on a monthly time scale and helps provide some of the winter gas demand.
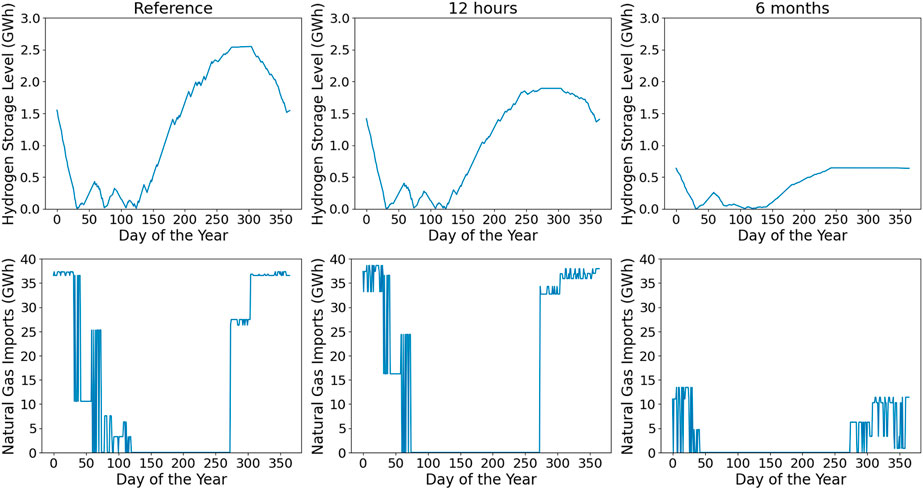
Figure 4. New High-Cost Dispatch scenario: yearly profiles for hydrogen storage level (top row) and natural gas imports (bottom row) for varying storage capacities (columns). The Reference case corresponds to no industrial DSM
Industrial DSM with a 12-h storage capacity (assuming
Industrial DSM with a 6-month storage capacity (assuming
In summary, in a scenario with expensive dispatchable flexibility alternatives to industrial DSM, the industrial DSM contributions are the largest across all of our studied scenarios, reducing system costs by up to 4.4% and industry-related energy costs by up to 20%. Additionally, the contributions grow with increasing flexibility provision time scale due to the displacement of long-duration flexibility alternatives.
3.1.2 No New Dispatch scenario
In the No New Dispatch scenario, similar to the New High-Cost Dispatch scenario, the system produces all electricity through renewable sources and relies on 3.5 GWh of hydrogen lined rock caverns (LRCs) and 8 TWh of pumped hydro storage for seasonal flexibility. However, contrary to the New High-Cost Dispatch scenario, no synthetic methane is produced due to the cheaper natural gas imports. Therefore, more renewable electricity can be stored in the form of hydrogen throughout the summer.
Industrial DSM with a 12-h storage capacity (assuming
Industrial DSM with a 6-month storage capacity (assuming
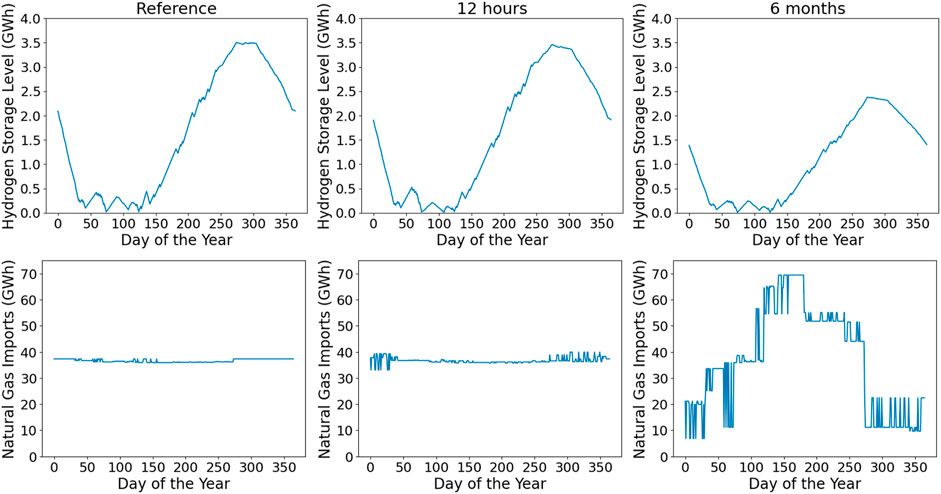
Figure 5. No New Dispatch scenario: yearly profiles for hydrogen storage level (top row) and natural gas imports (bottom row) for varying storage capacities (columns). The Reference case corresponds to no industrial DSM
In an extreme system configuration with limited dispatchable electricity production options, the contributions from industrial DSM grow with increasing storage capacity. Counterintuitively, however, industrial DSM decreases the installed capacities of renewable power generation technologies. Regardless of the amount of flexibility from industrial DSM, natural gas imports for the production of industrial heat remain constant, while investments in renewables decrease.
3.1.3 New Low-Cost Dispatch scenario
In the New Low-Cost Dispatch scenario without industrial DSM, dispatchable electricity from natural gas in combination with carbon capture and storage (CCS) is used to balance both the intraday and the seasonal fluctuations in renewables availability. To help with the seasonal imbalance of PV availability, which peaks in the summer, direct air capture (DAC) with CCS is deployed flexibly, maximizing its
Industrial DSM with a 12-h storage capacity (assuming
Industrial DSM with a 6-month storage capacity (assuming
In a scenario with inexpensive dispatchable flexibility alternatives, contributions from industrial DSM are the smallest across all of our studied scenarios, reducing system costs by up to 2% and industry-related energy costs by up to 12%. The maximum contributions from industrial DSM are obtained with flexibility provision at a daily time scale and the contributions do not increase when flexibility is provided over longer time horizons.
In summary, our findings across all three scenarios show that the magnitude of the cost reduction from industrial DSM depends on the system’s alternative flexibility options, particularly over time horizons longer than 12 h. Industrial DSM contributes most in systems with expensive long-duration flexibility alternatives. Across our studied scenarios, the maximum system cost reduction is 4.4%. This cost reduction represents an upper bound on contributions from industrial DSM since it considers no costs, losses, or technical constraints regarding DSM implementation. When considering cost reductions relative to industry-related energy costs, the maximum cost reduction ranges between 12% and 20%, indicating high incentives for industrial DSM implementation from an industry perspective. Moreover, the system cost reduction from industrial DSM might be higher in countries with higher shares of industrial energy demands than in Switzerland. The upper-bound system cost reductions relative to no industrial DSM may differ depending on actual industrial energy demand profiles, which we assume constant, and on the spatial distribution of energy supply and demand throughout Switzerland, which we aggregate for the purposes of our study.
3.2 Thermal energy storage as a DSM measure
The previous analysis assumes a generic industrial process to establish the maximal potential of industrial DSM. To explore whether this potential can be reached in practice, we study a concrete industrial DSM option: thermal energy storage (TES) for low and medium temperature heat (Section 2.3). As a process-independent DSM measure, TES can already harness between 28% and 61% of the maximum cost reductions from industrial DSM depending on the scenario (Figure 3). These findings are substantial considering that the cost reductions from TES take into account realistic costs and losses, as opposed to the upper-bound cost reductions from the generic industrial DSM implementation without costs, losses, or technical limitations. All three scenarios implement only TES for low temperature heat via water tanks, which is the cheapest among all TES options (Table 3).
In the New Low-Cost Dispatch scenario, TES harnesses 61% of the upper bound cost reductions from industrial DSM. The system invests in 38 GWh storage capacity for low temperature (LT) heat and nearly 5 times more capacity for electrified LT heat production than in the reference case without DSM. Similar to the results of the generic DSM implementation (Section 3.1.3), natural gas imports decrease by 12%, driving 40% of the cost reduction compared to no DSM.
In the New High-Cost Dispatch and No New Dispatch scenarios, the cost reductions arising from TES correspond to 28% and 35% of the maximum cost reductions from industrial DSM, respectively. The systems invest in 48 GWh and 35 GWh storage capacity for low temperature heat while also installing nearly 5 times more capacity for electrified LT heat production. While the savings from TES in these two scenarios realize less of the upper bound DSM potential than in the New Low-Cost Dispatch scenario, the cost reductions from TES are comparable to the upper bound savings under a 20% load-varying potential
Our implementation of TES as a concrete DSM measure, considering today’s costs and losses, shows that TES can harness between 28% and 61% of the upper bound cost reductions from industrial DSM. TES provides a promising and process-independent industrial DSM measure, showing that the potential benefits of industrial DSM are obtainable.
3.3 Storage capacity of industrial goods
As discussed in Section 3.1, the effect of storage capacity on cost reductions from industrial DSM differs between scenarios and depends on whether industrial DSM provides a preferred alternative for long-duration flexibility. In the following, we analyze the storage capacity actually used and show that all scenarios exhibit a maximum useful storage capacity beyond which cost reductions from industrial DSM stagnate.
In the New Low-Cost Dispatch scenario, which is characterized by inexpensive dispatchable flexibility alternatives, cost reductions already stagnate at a 12-h storage capacity (Section 3.1.3). In the New High-Cost Dispatch and No New Dispatch scenarios, representative of systems with expensive or missing dispatchable flexibility alternatives, costs decrease for increasing storage capacities beyond 1 month (Sections 3.1.1, 3.1.2). However, given a storage capacity availability of 6 months, both of these system configurations only use up to 3 months-worth of storage capacity. Hence, even systems that benefit from long-duration flexibility from industrial DSM reach a storage capacity beyond which cost reductions plateau.
To put the storage of industrial goods into perspective, we compare the magnitude of the energy stored across storage technologies (Table 4): in the New High-Cost Dispatch scenario with no industrial DSM, 8 TWh are stored in pumped hydro storage and 2.6 GWh are stored in hydrogen lined rock caverns. With maximum industrial DSM implementation, the 3 months-worth storage of industrial goods (assuming
Our findings indicate that there is a maximum useful storage capacity for industrial goods, above-which no additional industrial DSM flexibility provision is obtained. Hence, depending on the system configuration, small storage capacities can be sufficient to harvest the maximum benefits from industrial DSM. Additionally, the contributions from industrial DSM can be greater in countries with fewer seasonal flexibility options and a higher share of industry than Switzerland.
3.4 DSM losses
The discussion in the previous sections focuses on industrial DSM without efficiency losses. However, losses reduce the achievable cost reductions from industrial DSM. The DSM efficiency,
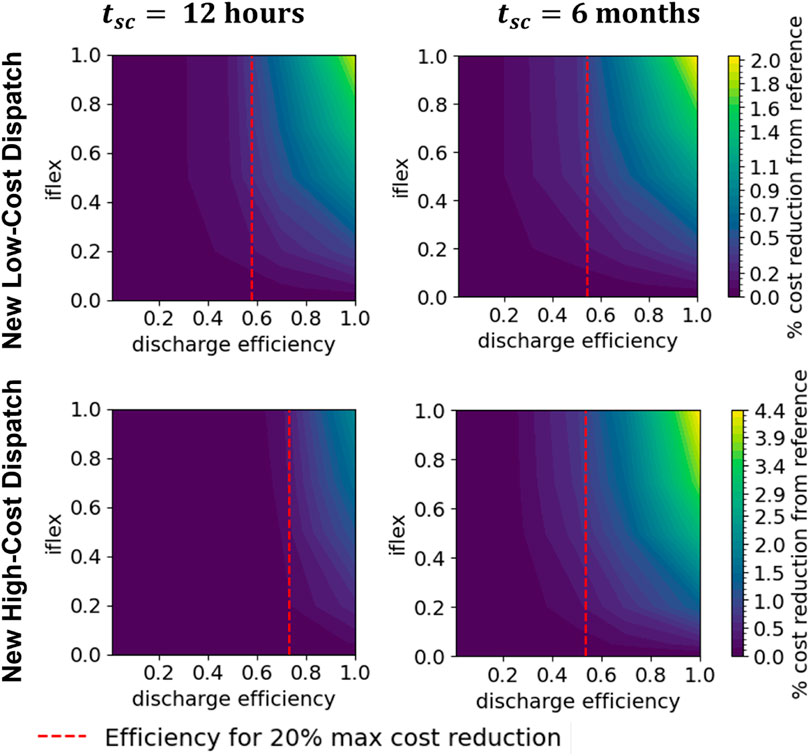
Figure 6. % cost reduction for the net-zero sector-coupled system for different values of load-varying potential,
In the New Low-Cost Dispatch scenario, the threshold efficiency is 58% for a storage capacity of 12 h and 55% for a storage capacity of 6 months. The small change in threshold efficiency across storage capacities reemphasizes the small influence of flexibility time horizon on cost reductions (Section 3.1.3), even when considering DSM losses. Nonetheless, efficiencies as low as 55% can yield 1/5 of the 2% maximum savings for this system configuration.
In the New High-Cost Dispatch scenario, the threshold efficiency decreases from 73% to 54% as storage capacity increases from 12 h to 6 months (Figure 6), emphasizing the benefits from longer storage duration. In the No New Dispatch scenario, the threshold efficiency decreases from 69% to 52% (Supplementary Figure S7 in the Supplementary Material). As shown for both scenarios, increasing storage capacity decreases the threshold efficiency. Therefore, when considering losses, larger storage capacities may be needed to harness the potential contributions from industrial DSM. However, under these system configurations, the value of long-duration flexibility from industrial DSM is so large that even DSM efficiencies around 50%–55% can provide 1/5 of the maximum cost reduction.
Our results show that while DSM efficiencies need to be above a certain threshold for industrial DSM to reduce system costs, the threshold can be as low as 52% to still obtain 1/5 of the savings. Particularly for system configurations with expensive or missing dispatchable flexibility options, more DSM losses are tolerated for larger industrial storage capacities due to the high value of long-duration flexibility from industrial DSM.
4 Conclusion
In this study, we investigate the potential of industrial demand-side management (DSM) as a flexibility provider for the Swiss sector-coupled energy system. We find that industrial DSM reduces costs for all three of our considered system configurations, with maximum system cost reductions ranging from 2% to 4.4%. These savings comprise between 12% and 20% of industry-related energy costs. Industrial DSM can lead to 6 TWh of energy equivalents stored in the form of industrial goods for seasonal flexibility, and thus reach a similar importance to hydro storage.
The magnitude of the cost reduction depends on the system’s alternative flexibility options and is greatest for systems with expensive or limited dispatchable flexibility alternatives. Under these system configurations, savings increase for longer flexibility provision time horizons due to the displacement of alternative long-duration flexibility options. This effect becomes magnified when considering DSM losses, in which case larger storage capacities are needed as losses increase in order to obtain the benefits of industrial DSM.
The maximum system cost reductions from industrial DSM represent an upper bound on the potential savings since they consider 100% over-sizing of industrial processes, no costs or losses associated with industrial DSM, and no technical constraints regarding DSM implementation. Still, as a concrete DSM option, thermal energy storage (TES) can harness between 28% and 61% of the upper bound industrial DSM savings while considering the associated costs and losses. Thus, TES provides a promising and process-independent DSM measure.
Overall, the maximum cost reductions from industrial DSM depend on both industrial process characteristics and on the system’s characteristics. Therefore, the value of industrial DSM must be evaluated within the context of the overall energy system. Particularly, industrial DSM is most beneficial when industrial products can be stored seasonally and long-duration flexibility options are expensive or missing. Additionally, we find large incentives for industrial DSM implementation from an industry perspective. Finally, we show that thermal energy storage can harness a large portion of the potential contributions from industrial DSM, making it a promising DSM measure and showing that economic benefits from industrial DSM are obtainable. Note that while we asses the value of industrial DSM from an economic perspective, industrial DSM might have additional benefits on power grid stability (Santecchia et al., 2022; Wang and Milanović, 2022) and environmental impacts (Yang et al., 2022; Nilges et al., 2024), potentially compounding the contributions from industrial DSM. Additionally, given the large energy equivalents stored in the form of industrial goods, we expect even larger contributions from industrial DSM in countries with fewer flexibility options or a higher share of industry than Switzerland.
Data availability statement
The original contributions presented in the study are included in the article/Supplementary Material. Further inquiries can be directed to the corresponding author.
Author contributions
PM: Conceptualization, Investigation, Methodology, Supervision, Visualization, Writing–original draft, Writing–review and editing. MH: Investigation, Methodology, Validation, Writing–review and editing. DS: Data curation, Supervision, Writing–review and editing. NZ: Data curation, Investigation, Writing–review and editing. LL: Methodology, Supervision, Writing–review and editing. FB: Conceptualization, Methodology, Supervision, Writing–review and editing. AB: Conceptualization, Funding acquisition, Supervision, Writing–review and editing.
Funding
The author(s) declare that financial support was received for the research, authorship, and/or publication of this article. This work was funded by the Swiss Federal Office of Energy’s SWEET program as part of the project PATHFNDR. Open access funding by ETH Zurich.
Acknowledgments
We thank Behnam Akbari for his help in gathering data for the hydrogen and methane storage technologies and we thank Fabian Leuenberger for his help in gathering data for the thermal energy storage technologies.
Conflict of interest
AB has ownership interests in firms that render services to industry, some of which may offer flexibility. AB has served on review committees for research and development at ExxonMobil and TotalEnergies, oil and gas companies that might also offer flexibility.
The remaining authors declare that the research was conducted in the absence of any commercial or financial relationships that could be construed as a potential conflict of interest.
Publisher’s note
All claims expressed in this article are solely those of the authors and do not necessarily represent those of their affiliated organizations, or those of the publisher, the editors and the reviewers. Any product that may be evaluated in this article, or claim that may be made by its manufacturer, is not guaranteed or endorsed by the publisher.
Supplementary material
The Supplementary Material for this article can be found online at: https://www.frontiersin.org/articles/10.3389/fenrg.2024.1443506/full#supplementary-material
References
Albertus, P., Manser, J. S., and Litzelman, S. (2020). Long-duration electricity storage applications, economics, and technologies. Joule 4, 21–32. doi:10.1016/j.joule.2019.11.009
Al Kindi, A. A., Sapin, P., Pantaleo, A. M., Wang, K., and Markides, C. N. (2022). Thermo-economic analysis of steam accumulation and solid thermal energy storage in direct steam generation concentrated solar power plants. Energy Convers. Manag. 274, 116222. doi:10.1016/j.enconman.2022.116222
Arnold, K., and Janssen, T. (2018). Demand side management in industry: necessary for a sustainable energy system or a backward step in terms of improving efficiency?.
Arteconi, A., Hewitt, N. J., and Polonara, F. (2013). Domestic demand-side management (dsm): role of heat pumps and thermal energy storage (tes) systems. Appl. Therm. Eng. 51, 155–165. doi:10.1016/j.applthermaleng.2012.09.023
Bao, P., Zhang, W., Cheng, D., and Liu, M. (2020). Hierarchical control of aluminum smelter loads for primary frequency support considering control cost. Int. J. Electr. Power and Energy Syst. 122, 106202. doi:10.1016/j.ijepes.2020.106202
Bardow, A., Fiorentini, M., Heer, P., Kämper, A., Koirala, B., Knoeri, C., et al. (2023). Flexibility and sector coupling in energy systems: definitions and metrics: synthesis report. Zurich: ETH Zurich.
Bielefeld, S., Cvetković, M., and Ramírez, A. (2023). Should we exploit flexibility of chemical processes for demand response? differing perspectives on potential benefits and limitations. Front. Energy Res. 11, 1190174. doi:10.3389/fenrg.2023.1190174
Bohlayer, M., Fleschutz, M., Braun, M., and Zöttl, G. (2020). Energy-intense production-inventory planning with participation in sequential energy markets. Appl. Energy 258, 113954. doi:10.1016/j.apenergy.2019.113954
Budinis, S., Krevor, S., Mac Dowell, N., Brandon, N., and Hawkes, A. (2018). An assessment of ccs costs, barriers and potential. Energy strategy Rev. 22, 61–81. doi:10.1016/j.esr.2018.08.003
Caspari, A., Pérez, Y. M., Offermanns, C., Schäfer, P., Ecker, A.-M., Peschel, A., et al. (2019). Economic nonlinear model predictive control of multi-product air separation processes. Comput. Aided Chem. Eng. (Elsevier) 46, 1–6. doi:10.1016/b978-0-12-818634-3.50001-1
Cirocco, L., Pudney, P., Riahi, S., Liddle, R., Semsarilar, H., Hudson, J., et al. (2022). Thermal energy storage for industrial thermal loads and electricity demand side management. Energy Convers. Manag. 270, 116190. doi:10.1016/j.enconman.2022.116190
Cruz, I., Ilić, D. D., and Johansson, M. T. (2023). Using flexible energy system interactions amongst industry, district heating, and the power sector to increase renewable energy penetration. Energy Effic. 16, 53. doi:10.1007/s12053-023-10134-4
Gabrielli, P., Poluzzi, A., Kramer, G. J., Spiers, C., Mazzotti, M., and Gazzani, M. (2020). Seasonal energy storage for zero-emissions multi-energy systems via underground hydrogen storage. Renew. Sustain. Energy Rev. 121, 109629. doi:10.1016/j.rser.2019.109629
Gautam, K. R., Andresen, G. B., and Victoria, M. (2022). Review and techno-economic analysis of emerging thermo-mechanical energy storage technologies. Energies 15, 6328. doi:10.3390/en15176328
Golmohamadi, H. (2022). Demand-side management in industrial sector: a review of heavy industries. Renew. Sustain. Energy Rev. 156, 111963. doi:10.1016/j.rser.2021.111963
Hammond, G., and Norman, J. (2014). Heat recovery opportunities in UK industry. Appl. Energy 116, 387–397. doi:10.1016/j.apenergy.2013.11.008
He, R., Dong, P., Liu, M., Huang, S., and Li, J. (2023). Research on large-scale wind power consumption in the electricity market considering demand response and energy storage systems. Front. Energy Res. 10, 1025152. doi:10.3389/fenrg.2022.1025152
Helin, K., Käki, A., Zakeri, B., Lahdelma, R., and Syri, S. (2017). Economic potential of industrial demand side management in pulp and paper industry. Energy 141, 1681–1694. doi:10.1016/j.energy.2017.11.075
IRENA (2013). Thermal energy storage: technology brief. IEA-ETSAP and IRENA- Technology Brief. E17-January.
Kachirayil, F., Weinand, J. M., Scheller, F., and McKenna, R. (2022). Reviewing local and integrated energy system models: insights into flexibility and robustness challenges. Appl. energy 324, 119666. doi:10.1016/j.apenergy.2022.119666
Klaucke, F., Karsten, T., Holtrup, F., Esche, E., Morosuk, T., Tsatsaronis, G., et al. (2017). Demand response potentials for the chemical industry. Chem. Ing. Tech. 89, 1133–1141. doi:10.1002/cite.201600073
Koohi-Fayegh, S., and Rosen, M. A. (2020). A review of energy storage types, applications and recent developments. J. Energy Storage 27, 101047. doi:10.1016/j.est.2019.101047
Kotzur, L., Markewitz, P., Robinius, M., and Stolten, D. (2018). Time series aggregation for energy system design: modeling seasonal storage. Appl. Energy 213, 123–135. doi:10.1016/j.apenergy.2018.01.023
Lu, P., Lv, H., Liu, N., Wang, T., Han, J., Zhang, W., et al. (2021). Optimal flexibility dispatch of demand side resources with high penetration of renewables: a stackelberg game method. Glob. Energy Interconnect. 4, 28–38. doi:10.1016/j.gloei.2021.03.003
Manana, M., Zobaa, A., Vaccaro, A., Arroyo, A., Martinez, R., Castro, P., et al. (2021). Increase of capacity in electric arc-furnace steel mill factories by means of a demand-side management strategy and ampacity techniques. Int. J. Electr. Power and Energy Syst. 124, 106337. doi:10.1016/j.ijepes.2020.106337
Marañón-Ledesma, H., and Tomasgard, A. (2019). Analyzing demand response in a dynamic capacity expansion model for the european power market. Energies 12, 2976. doi:10.3390/en12152976
Marcucci, A., Guidati, G., and Giardini, D. (2021). Swiss energy scope–ETH: Swiss energy scope with hourly resolution. Zurich: ETH Zurich.
Meyabadi, A. F., and Deihimi, M. H. (2017). A review of demand-side management: reconsidering theoretical framework. Renew. Sustain. Energy Rev. 80, 367–379. doi:10.1016/j.rser.2017.05.207
Murakoshi, R., and Fushimi, C. (2022). Integration of a steam accumulator with a biomass power-generation system for flexible energy storage and discharge: effect of the initial steam pressure on the steam discharge profile and levelized cost of storage. J. Energy Storage 55, 105586. doi:10.1016/j.est.2022.105586
Nebel, A., Krüger, C., Janßen, T., Saurat, M., Kiefer, S., and Arnold, K. (2020). Comparison of the effects of industrial demand side management and other flexibilities on the performance of the energy system. Energies 13, 4448. doi:10.3390/en13174448
Nilges, B., Reinert, C., and von der Assen, N. (2024). Is demand-side management environmentally beneficial? analyzing the greenhouse gas emissions due to load shifting in electric power systems. J. Clean. Prod. 434, 140062. doi:10.1016/j.jclepro.2023.140062
Papadaskalopoulos, D., Moreira, R., Strbac, G., Pudjianto, D., Djapic, P., Teng, F., et al. (2018). Quantifying the potential economic benefits of flexible industrial demand in the european power system. IEEE Trans. Industrial Inf. 14, 5123–5132. doi:10.1109/tii.2018.2811734
Paulus, M., and Borggrefe, F. (2011). The potential of demand-side management in energy-intensive industries for electricity markets in Germany. Appl. energy 88, 432–441. doi:10.1016/j.apenergy.2010.03.017
Pellow, M. A., Emmott, C. J., Barnhart, C. J., and Benson, S. M. (2015). Hydrogen or batteries for grid storage? A net energy analysis. Energy and Environ. Sci. 8, 1938–1952. doi:10.1039/c4ee04041d
Petkov, I., and Gabrielli, P. (2020). Power-to-hydrogen as seasonal energy storage: an uncertainty analysis for optimal design of low-carbon multi-energy systems. Appl. Energy 274, 115197. doi:10.1016/j.apenergy.2020.115197
Pfenninger, S., Hawkes, A., and Keirstead, J. (2014). Energy systems modeling for twenty-first century energy challenges. Renew. Sustain. Energy Rev. 33, 74–86. doi:10.1016/j.rser.2014.02.003
Ramsebner, J., Haas, R., Ajanovic, A., and Wietschel, M. (2021). The sector coupling concept: a critical review. Wiley Interdiscip. Rev. energy Environ. 10, e396. doi:10.1002/wene.396
Reinert, C., Schellhas, L., Mannhardt, J., Shu, D. Y., Kämper, A., Baumgärtner, N., et al. (2022). Secmod: an open-source modular framework combining multi-sector system optimization and life-cycle assessment. Front. Energy Res. 10, 884525. doi:10.3389/fenrg.2022.884525
Röben, F. T., Liu, D., Reuter, M. A., Dahmen, M., and Bardow, A. (2022). The demand response potential in copper production. J. Clean. Prod. 362, 132221. doi:10.1016/j.jclepro.2022.132221
Rypdal, K., Paciornik, N., Eggleston, S., Goodwin, J., Irving, W., Penman, J., et al. (2006). 2006 IPCC guidelines for national greenhouse gas inventories: chapter 1. Hayama, Kanagawa, Japan: Institute for Global Environmental Strategies.
Santecchia, A., Kantor, I., Castro-Amoedo, R., and Marechal, F. (2022). Industrial flexibility as demand side response for electrical grid stability. Front. Energy Res. 10, 831462. doi:10.3389/fenrg.2022.831462
Schäfer, P., Daun, T. M., and Mitsos, A. (2020). Do investments in flexibility enhance sustainability? a simulative study considering the German electricity sector. AIChE J. 66, e17010. doi:10.1002/aic.17010
Sepulveda, N. A., Jenkins, J. D., Edington, A., Mallapragada, D. S., and Lester, R. K. (2021). The design space for long-duration energy storage in decarbonized power systems. Nat. Energy 6, 506–516. doi:10.1038/s41560-021-00796-8
Shaner, M. R., Davis, S. J., Lewis, N. S., and Caldeira, K. (2018). Geophysical constraints on the reliability of solar and wind power in the United States. Energy and Environ. Sci. 11, 914–925. doi:10.1039/c7ee03029k
Sternberg, A., and Bardow, A. (2015). Power-to-what? environmental assessment of energy storage systems. Energy and Environ. Sci. 8, 389–400. doi:10.1039/c4ee03051f
Strasser, M. N., and Selvam, R. P. (2014). A cost and performance comparison of packed bed and structured thermocline thermal energy storage systems. Sol. Energy 108, 390–402. doi:10.1016/j.solener.2014.07.023
Swissgrid (2020). Energy Statistic Switzerland 2019. Available at: https://www.swissgrid.ch/en/home/operation/grid-data/generation.html
Swiss Federal Office of Energy (SFOE) (2020b). Analyse des schweizerischen Energieverbrauchs 2000-2019 nach Verwendungszwecken. Tech. Rep.
The Federal Council (2019). Federal Council aims for a climate-neutral Switzerland by 2050. (Accessed May 5, 2021).
Trading Economics (2023). Natural Gas. Available at: https://tradingeconomics.com/commodity/eu-natural-gas.
Torabi, R., Gomes, A., Lobo, D., and Morgado-Dias, F. (2020). Modelling demand flexibility and energy storage to support increased penetration of renewable energy resources on porto santo. Greenh. Gases Sci. Technol. 10, 1118–1132. doi:10.1002/ghg.2005
United Nations (2023). For a livable climate: net-zero commitments must be backed by credible action. Available at: https://www.un.org/en/climatechange/net-zero-coalition
Victoria, M., Zhu, K., Brown, T., Andresen, G. B., and Greiner, M. (2019). The role of storage technologies throughout the decarbonisation of the sector. Energy Convers. Manag. 201, 111977. doi:10.1016/j.enconman.2019.111977
Viswanathan, V., Mongird, K., Franks, R., Li, X., and Sprenkle, V. (2022). “Energy storage grand challenge cost and performance assessment 2022,” in Pacific Northwest national laboratory, US Departmat of energy, technical report, publication, PNNL-33283.
Wachs, L., McMillan, C., and Reese, S. B. (2023). Reviewing flexibility in industrial electrification: focusing on green ammonia and steel in the United States. Energy Res. Soc. Sci. 103, 103202. doi:10.1016/j.erss.2023.103202
Wang, M., and Milanović, J. V. (2022). The impacts of demand side management on combined frequency and angular stability of the power system. IEEE Trans. Power Syst., 1–12. doi:10.1109/tpwrs.2022.3203887
Wang, X., Chen, Z., Xie, H., Liao, S., Ye, X., and Chen, G. (2024). Demand-side response power control strategy considering load production sequence requirements. Front. Energy Res. 12, 1370301. doi:10.3389/fenrg.2024.1370301
Williams, B., Bishop, D., Gallardo, P., and Chase, J. G. (2023). Demand side management in industrial, commercial, and residential sectors: a review of constraints and considerations. Energies 16, 5155. doi:10.3390/en16135155
Yang, C., He, B., Liao, H., Ruan, J., and Zhao, J. (2022). Price-based low-carbon demand response considering the conduction of carbon emission costs in smart grids. Front. Energy Res. 10, 959786. doi:10.3389/fenrg.2022.959786
Zhang, Q., and Grossmann, I. E. (2016). Enterprise-wide optimization for industrial demand side management: fundamentals, advances, and perspectives. Chem. Eng. Res. Des. 116, 114–131. doi:10.1016/j.cherd.2016.10.006
Zhang, Q., and Pinto, J. M. (2022). Energy-aware enterprise-wide optimization and clean energy in the industrial gas industry. Comput. Chem. Eng. 165, 107927. doi:10.1016/j.compchemeng.2022.107927
Nomenclature
Keywords: demand response, flexibility, sector-coupling, net-zero, industry, Switzerland, thermal energy storage, optimization
Citation: Mayer P, Heer M, Shu DY, Zielonka N, Leenders L, Baader FJ and Bardow A (2024) Flexibility from industrial demand-side management in net-zero sector-coupled national energy systems. Front. Energy Res. 12:1443506. doi: 10.3389/fenrg.2024.1443506
Received: 04 June 2024; Accepted: 18 July 2024;
Published: 14 August 2024.
Edited by:
Francesco Liberato Cappiello, University of Naples Federico II, ItalyReviewed by:
Yi Zong, Technical University of Denmark, DenmarkWei He, University of Warwick, United Kingdom
Copyright © 2024 Mayer, Heer, Shu, Zielonka, Leenders, Baader and Bardow. This is an open-access article distributed under the terms of the Creative Commons Attribution License (CC BY). The use, distribution or reproduction in other forums is permitted, provided the original author(s) and the copyright owner(s) are credited and that the original publication in this journal is cited, in accordance with accepted academic practice. No use, distribution or reproduction is permitted which does not comply with these terms.
*Correspondence: André Bardow, YWJhcmRvd0BldGh6LmNo